


Diffusion model overcomes algorithmic problems, AGI is not far away! Google Brain finds the shortest path in a maze
「擴散模型」也能攻克演算法難題?
圖片
一位博士研究人員做了一個有趣的實驗,用「離散擴散」尋找用圖像表示的迷宮中的最短路徑。
圖片
作者介紹,每個迷宮都是透過重複添加水平和垂直牆生成的。
其中,起始點和目標點隨機選取。
從起點到目標點的最短路徑中,隨機取樣一條作為解的路徑。最短路徑是透過精確演算法算出來的。
圖片
然後使用離散擴散模型和U-Net。
將起點和目標的迷宮被編碼在一個通道中,而模型在另一個通道中用解來消除迷宮的雜訊。
圖片
再難一點的迷宮,也能做的很好。
圖片
為了估算去雜訊步驟p(x_{t-1} | x_t),演算法會估算p( x_0 | x_t)。在這個過程中可視化這一估計值(底行),顯示“當前假設”,最終聚焦在結果上。
圖片
英偉達資深科學家Jim Fan表示,這是一個有趣的實驗,擴散模型可以「渲染」演算法。它可以只從像素實現迷宮遍歷,甚至使用了比Transforme弱得多的U-Net。
我一直認為擴散模型是渲染器,而Transformer是推理引擎。看起來,渲染器本身也可以編碼非常複雜的順序演算法。
圖片
這個實驗簡直驚呆了網友,「擴散模型還能做什麼?!」
圖片
也有人表示,一旦有人在足夠好的資料集上訓練擴散Transformer,AGI就解決了。
圖片
不過這項研究尚未正式發布,作者表示稍後更新在arxiv上。
值得一提的是,在這個實驗中,他們採用了Google腦團隊曾在2021年提出的離散擴散模型。
圖片
就在最近,這項研究重新更新了一版。
離散擴散模型
「生成模型」是機器學習中的核心問題。
它既可用於衡量我們擷取自然資料集統計資料的能力,也可用於需要產生影像、文字和語音等高維度資料的下游應用程式。
GAN, VAE, large autoregressive neural network models, normalized flow and other methods have their own advantages in sample quality, sampling speed, log likelihood, and training stability.
Recently, the "diffusion model" has become the most popular alternative for image and audio generation.
It can achieve sample quality comparable to GAN and log-likelihood comparable to autoregressive models with fewer inference steps.
Picture
Paper address: https://www.php.cn/link/46994a3cd8d943d03b44b8fc9792d435
Although diffusion models for discrete and continuous state spaces have been proposed, recent research has mainly focused on Gaussian diffusion processes operating in continuous state spaces (such as real-valued images and waveform data).
Discrete state space diffusion models have been explored in the field of text and image segmentation, but have not yet proven to be a competitive solution in large-scale text and image generation tasks. model.
The Google research team proposed a new discrete denoising diffusion probability model (D3PM).
In the study, the authors demonstrate that the choice of transition matrix is an important design decision that can improve results in both image and text domains.
Additionally, they proposed a new loss function that combines a variational lower bound and an auxiliary cross-entropy loss.
In terms of text, this model achieves good results in character-level text generation and can be extended to the large vocabulary LM1B dataset.
On the CIFAR-10 image dataset, the latest model approaches the sample quality of the continuous space DDPM model and exceeds the log-likelihood of the continuous space DDPM model.
Picture
Project Author
Arnaud Pannatier
Arnaud Pannatier started studying for his PhD in March 2020 in the machine learning group of his supervisor François Fleuret.
He recently developed HyperMixer, which uses a super network to enable MLPMixer to handle inputs of various lengths. This enables the model to process the input in a permutation-invariant manner and has been shown to give the model an attentional behavior that scales linearly with the length of the input.
At EPFL, he earned a bachelor’s degree in physics and a master’s degree in computer science and engineering (CSE-MASH).
Reference:
The above is the detailed content of Diffusion model overcomes algorithmic problems, AGI is not far away! Google Brain finds the shortest path in a maze. For more information, please follow other related articles on the PHP Chinese website!
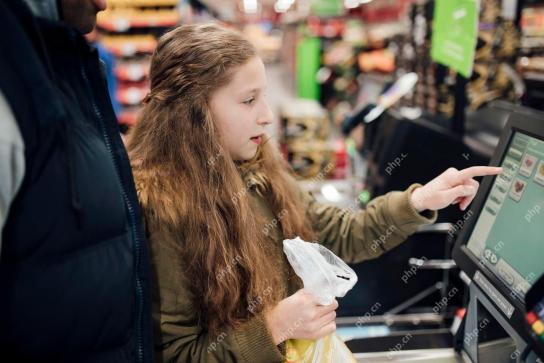
Revolutionizing the Checkout Experience Sam's Club's innovative "Just Go" system builds on its existing AI-powered "Scan & Go" technology, allowing members to scan purchases via the Sam's Club app during their shopping trip.
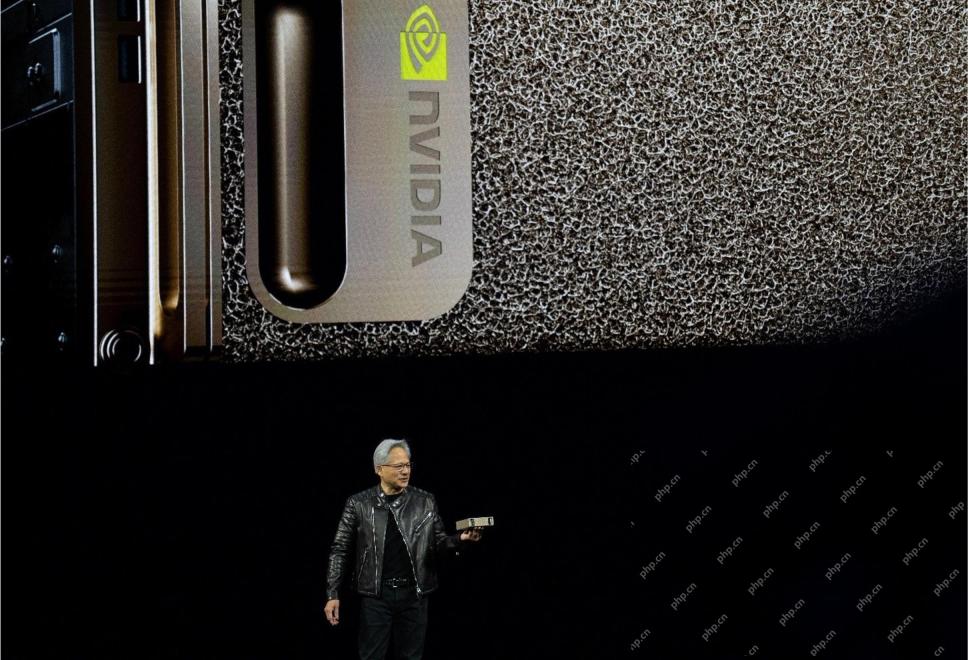
Nvidia's Enhanced Predictability and New Product Lineup at GTC 2025 Nvidia, a key player in AI infrastructure, is focusing on increased predictability for its clients. This involves consistent product delivery, meeting performance expectations, and
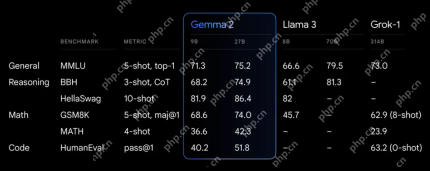
Google's Gemma 2: A Powerful, Efficient Language Model Google's Gemma family of language models, celebrated for efficiency and performance, has expanded with the arrival of Gemma 2. This latest release comprises two models: a 27-billion parameter ver
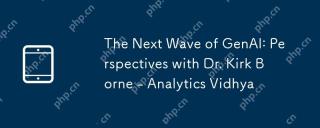
This Leading with Data episode features Dr. Kirk Borne, a leading data scientist, astrophysicist, and TEDx speaker. A renowned expert in big data, AI, and machine learning, Dr. Borne offers invaluable insights into the current state and future traje
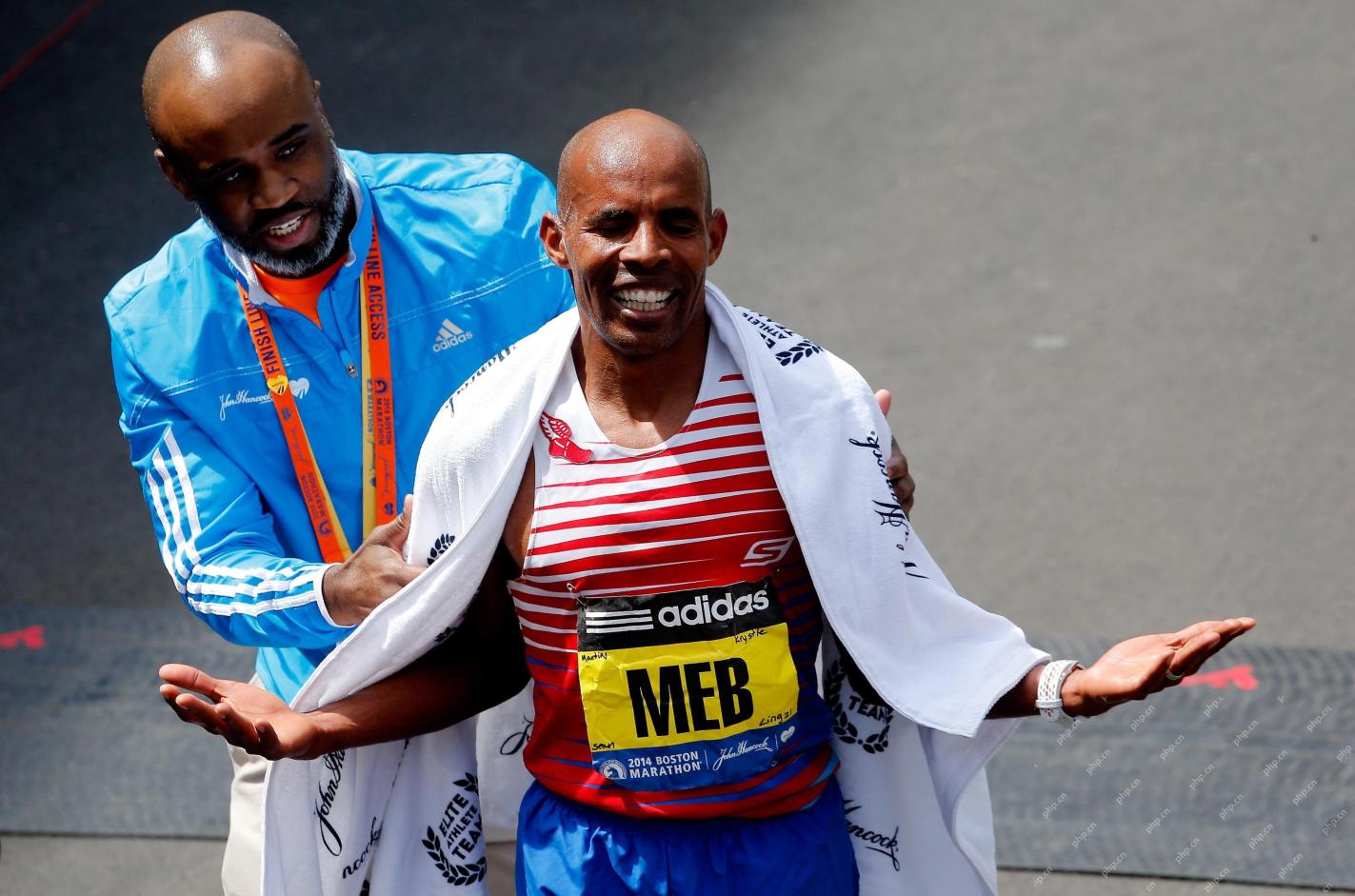
There were some very insightful perspectives in this speech—background information about engineering that showed us why artificial intelligence is so good at supporting people’s physical exercise. I will outline a core idea from each contributor’s perspective to demonstrate three design aspects that are an important part of our exploration of the application of artificial intelligence in sports. Edge devices and raw personal data This idea about artificial intelligence actually contains two components—one related to where we place large language models and the other is related to the differences between our human language and the language that our vital signs “express” when measured in real time. Alexander Amini knows a lot about running and tennis, but he still
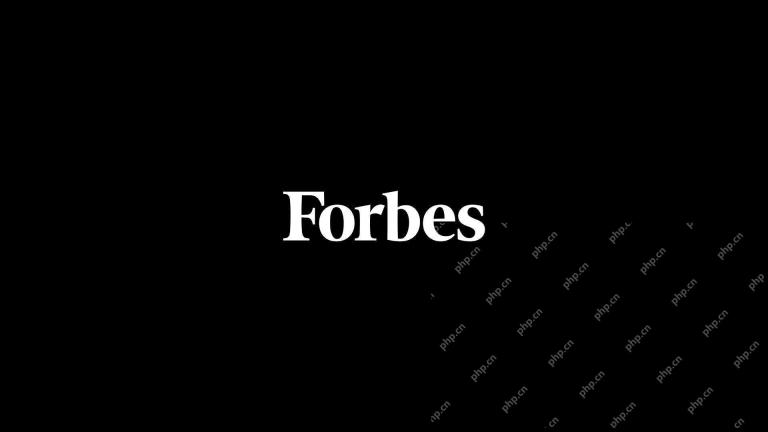
Caterpillar's Chief Information Officer and Senior Vice President of IT, Jamie Engstrom, leads a global team of over 2,200 IT professionals across 28 countries. With 26 years at Caterpillar, including four and a half years in her current role, Engst
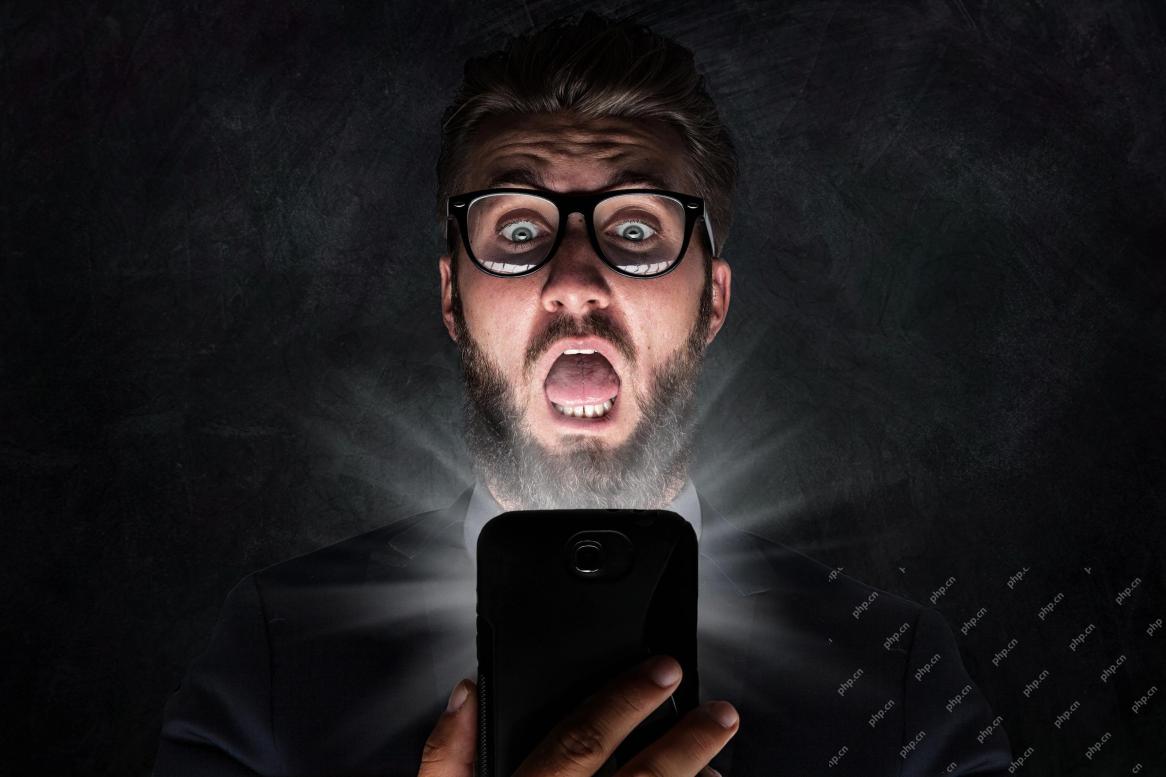
Google Photos' New Ultra HDR Tool: A Quick Guide Enhance your photos with Google Photos' new Ultra HDR tool, transforming standard images into vibrant, high-dynamic-range masterpieces. Ideal for social media, this tool boosts the impact of any photo,
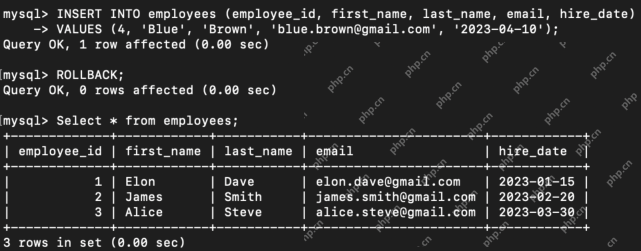
Introduction Transaction Control Language (TCL) commands are essential in SQL for managing changes made by Data Manipulation Language (DML) statements. These commands allow database administrators and users to control transaction processes, thereby


Hot AI Tools

Undresser.AI Undress
AI-powered app for creating realistic nude photos

AI Clothes Remover
Online AI tool for removing clothes from photos.

Undress AI Tool
Undress images for free

Clothoff.io
AI clothes remover

Video Face Swap
Swap faces in any video effortlessly with our completely free AI face swap tool!

Hot Article

Hot Tools

SublimeText3 English version
Recommended: Win version, supports code prompts!

mPDF
mPDF is a PHP library that can generate PDF files from UTF-8 encoded HTML. The original author, Ian Back, wrote mPDF to output PDF files "on the fly" from his website and handle different languages. It is slower than original scripts like HTML2FPDF and produces larger files when using Unicode fonts, but supports CSS styles etc. and has a lot of enhancements. Supports almost all languages, including RTL (Arabic and Hebrew) and CJK (Chinese, Japanese and Korean). Supports nested block-level elements (such as P, DIV),
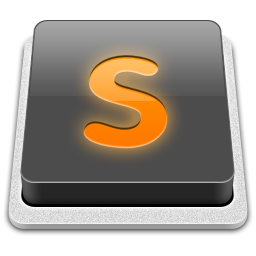
SublimeText3 Mac version
God-level code editing software (SublimeText3)

MinGW - Minimalist GNU for Windows
This project is in the process of being migrated to osdn.net/projects/mingw, you can continue to follow us there. MinGW: A native Windows port of the GNU Compiler Collection (GCC), freely distributable import libraries and header files for building native Windows applications; includes extensions to the MSVC runtime to support C99 functionality. All MinGW software can run on 64-bit Windows platforms.

Atom editor mac version download
The most popular open source editor