


The Art of Data Analysis with Python: Exploring Advanced Tips and Techniques
Optimization of data preprocessing
Missing value handling:
-
interpolate()
Function: Use interpolation method to fill missing values. -
KNNImputer()
Module: Estimating missing values through K nearest neighbor algorithm . -
MICE
Method: Create multiple data sets through multiple imputation and combine the results.
Outlier detection and processing:
-
IQR()
Method: Identify outliers outside the interquartile range. -
Isolat<strong class="keylink">io</strong>n Forest
Algorithm: Isolate data points with abnormal behavior. -
DBSCAN
Algorithm: Detect outliers based on density clustering.
Feature Engineering
Feature selection:
-
SelectKBest
Function: Selects the best features based on the chi-square test or ANOVA statistic. -
SelectFromModel
Module: Use Machine Learning models (such as decision trees) to select features. -
L1 Regularization
: Penalize the weight of features in the model to select the most important features.
Feature transformation:
-
Standardization
andNormalization
: Ensure that features are within the same range and improve model performance. -
Principal Component Analysis (PCA)
: Reduce the feature dimension and remove redundant information. -
Local Linear Embedding (LLE)
: A nonlinear dimensionality reduction technique that preserves local structure.
Optimization of machine learning models
Hyperparameter tuning:
-
GridSearchCV
Function: Automatically search for the best hyperparameter array combination. -
RandomizedSearchCV
Module: Use random search algorithms to explore hyperparameter space more efficiently. -
Bayesian<strong class="keylink">Optimization</strong>
: Use probabilistic models to guide hyperparameter searches.
Model evaluation and selection:
-
Cross-validation
: Split the data set into multiple subsets to evaluate the generalization ability of the model. -
ROC/AUC Curve
: Evaluate the performance of the classification model. -
PR Curve
: Evaluate the trade-off between precision and recall of binary classification models.
Visualization and interactivity
Interactive Dashboard:
-
Plotly
andDash
libraries: Create interactive charts that allow users to explore data and tune models. -
Streamlit
Framework: Build fast, simple WEB applications to share data insights.
Geospatial Analysis:
-
Geo<strong class="keylink">pandas</strong>
Library: Process geospatial data such as shape files and raster data. -
Folium
Module: Create Visualization with a map. -
OpenStreetMap
Datasets: Provides free and open data for geospatial analysis.
Advanced Tips
Machine Learning Pipeline:
- Combine data preprocessing, feature engineering, and modeling steps into reusable pipelines.
- Simplify workflow and improve repeatability and maintainability.
Parallel processing:
- Use
multiprocessing
andjoblib
libraries for parallel processing of data-intensive tasks. - Shorten running time and improve processing efficiency of large data sets.
cloud computing:
- Use cloud platforms such as
AWS
,<strong class="keylink">GC</strong>P
or<strong class="keylink">Azure</strong>
for large-scale data analyze. - Expand computing resources to process extremely large geodata sets and accelerate the analysis process.
The above is the detailed content of The Art of Data Analysis with Python: Exploring Advanced Tips and Techniques. For more information, please follow other related articles on the PHP Chinese website!
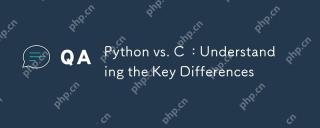
Python and C each have their own advantages, and the choice should be based on project requirements. 1) Python is suitable for rapid development and data processing due to its concise syntax and dynamic typing. 2)C is suitable for high performance and system programming due to its static typing and manual memory management.
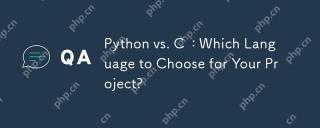
Choosing Python or C depends on project requirements: 1) If you need rapid development, data processing and prototype design, choose Python; 2) If you need high performance, low latency and close hardware control, choose C.
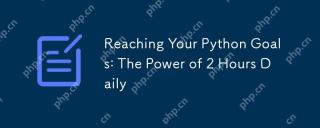
By investing 2 hours of Python learning every day, you can effectively improve your programming skills. 1. Learn new knowledge: read documents or watch tutorials. 2. Practice: Write code and complete exercises. 3. Review: Consolidate the content you have learned. 4. Project practice: Apply what you have learned in actual projects. Such a structured learning plan can help you systematically master Python and achieve career goals.
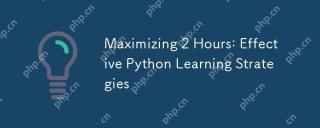
Methods to learn Python efficiently within two hours include: 1. Review the basic knowledge and ensure that you are familiar with Python installation and basic syntax; 2. Understand the core concepts of Python, such as variables, lists, functions, etc.; 3. Master basic and advanced usage by using examples; 4. Learn common errors and debugging techniques; 5. Apply performance optimization and best practices, such as using list comprehensions and following the PEP8 style guide.
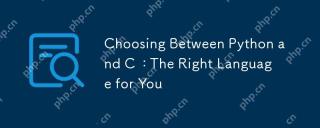
Python is suitable for beginners and data science, and C is suitable for system programming and game development. 1. Python is simple and easy to use, suitable for data science and web development. 2.C provides high performance and control, suitable for game development and system programming. The choice should be based on project needs and personal interests.
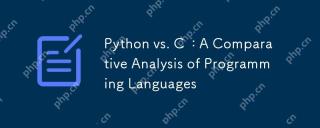
Python is more suitable for data science and rapid development, while C is more suitable for high performance and system programming. 1. Python syntax is concise and easy to learn, suitable for data processing and scientific computing. 2.C has complex syntax but excellent performance and is often used in game development and system programming.
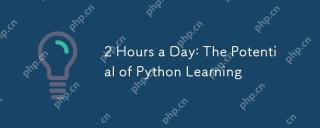
It is feasible to invest two hours a day to learn Python. 1. Learn new knowledge: Learn new concepts in one hour, such as lists and dictionaries. 2. Practice and exercises: Use one hour to perform programming exercises, such as writing small programs. Through reasonable planning and perseverance, you can master the core concepts of Python in a short time.
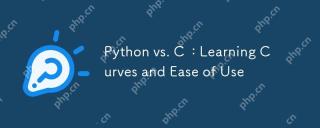
Python is easier to learn and use, while C is more powerful but complex. 1. Python syntax is concise and suitable for beginners. Dynamic typing and automatic memory management make it easy to use, but may cause runtime errors. 2.C provides low-level control and advanced features, suitable for high-performance applications, but has a high learning threshold and requires manual memory and type safety management.


Hot AI Tools

Undresser.AI Undress
AI-powered app for creating realistic nude photos

AI Clothes Remover
Online AI tool for removing clothes from photos.

Undress AI Tool
Undress images for free

Clothoff.io
AI clothes remover

Video Face Swap
Swap faces in any video effortlessly with our completely free AI face swap tool!

Hot Article

Hot Tools

MantisBT
Mantis is an easy-to-deploy web-based defect tracking tool designed to aid in product defect tracking. It requires PHP, MySQL and a web server. Check out our demo and hosting services.
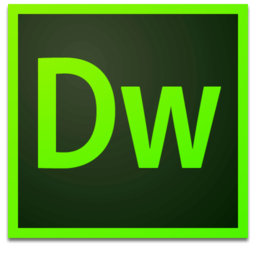
Dreamweaver Mac version
Visual web development tools
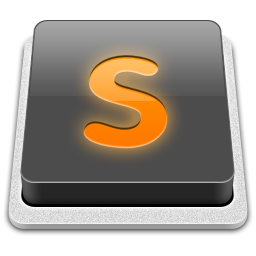
SublimeText3 Mac version
God-level code editing software (SublimeText3)

PhpStorm Mac version
The latest (2018.2.1) professional PHP integrated development tool
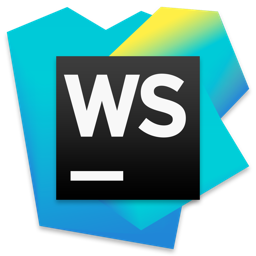
WebStorm Mac version
Useful JavaScript development tools