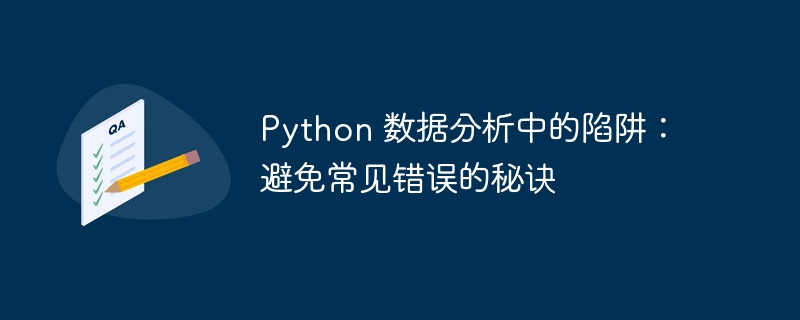
Data collection and preparation
-
Poor Data Quality: It is important to ensure that your data is accurate, complete, and consistent. Appropriate cleaning and transformation of data to eliminate errors and outliers.
-
Data Bias: Consider the source and collection method of the data. Ensure the sample is representative to avoid biasing the results.
-
Data preparation errors: Be careful of errors in data conversion when converting data to a different format or structure. Review data transformation and processing steps to ensure accuracy.
data analysis
-
Wrong Assumptions: Always verify assumptions and determine whether they are reasonable and based on evidence before conducting an analysis.
-
Overfitting: The model is too complex, causing it to overfit the training data and fail to generalize well on new data. Use regularization techniques or cross-validation to prevent overfitting.
-
Feature engineering errors: Errors in the feature selection and transformation process may cause model performance to degrade. Use appropriate feature selection and transformation techniques and carefully evaluate feature importance.
Model selection and evaluation
-
Model Wrong Selection: Selecting an appropriate model based on the type of problem and the nature of the data is critical. Avoid using complex models that are not suitable for the task.
-
Stop training prematurely: The model may be overfitted if the training time is too long. Find the optimal training time for optimal performance.
-
Model evaluation errors: Use evaluation metrics appropriate for the task to evaluate model performance. Avoid focusing on a single metric and consider multiple metrics.
Result Interpretation and Visualization
-
Error interpretation: Interpret results carefully and avoid drawing inappropriate conclusions. Consider model limitations and potential biases in the data.
-
Chart distortion: When creating data visualizations, avoid using distorted scales, colors, or chart types. Make sure Visualization is accurate and easy to understand.
-
Misleading Charts: Be careful using charts to illustrate a specific point or to hide information. Make sure the chart presents the data in a fair and unbiased way.
Other Traps
-
Handling large data sets: When processing large data sets, Optimize your code and use appropriate data structures to avoid out of memory or long processing times .
-
Library version conflicts: Manage python package and library versions to avoid compatibility issues and errors.
-
Debugging difficulties: Add appropriate comments and documentation to your code to make it easy to debug and maintain. Use debugging Tools to find and fix errors.
Following these tips can help data analysts avoid common pitfalls, thereby improving the accuracy and reliability of analysis results. Additionally, continuous learning, engaging with the community, and learning from mistakes is critical to improving your data analysis skills and avoiding future pitfalls.
The above is the detailed content of Pitfalls in Python Data Analysis: Tips to Avoid Common Mistakes. For more information, please follow other related articles on the PHP Chinese website!