A seed was planted in 2021
Students who have read the BEV obstacle story should know that our group started doing BEV obstacles around October 21 material. At that time, I didn’t dare to think about doing BEV lane markings because there was no manpower. But I remember that around December, we met with a candidate. During the interview, we heard that they had been working on BEV lane markings for more than half a year. The entire technical route was used as a BEV lane marking network through high-precision maps. Train the true value and say that the effect is not bad. Unfortunately, that candidate did not come to us in the end. Combined with the content of lane markings taught at Telsa AI day in 2021, the seed of making BEV lane markings was planted in the group.
Taking the right first step in 2022
Throughout 2022, the manpower in our team was very tight. I remember that in June and July, We just have the manpower to explore the BEV lane lines. But at that time, there was only one classmate in our group (let’s call him Xiaoxuan for now) who had 2 months to do this. Then the seeds of 2021 began to sprout. We were going to start with the data. Student Xiaoxuan was still very good (very imaginative, and Xiaoxuan also made more things that surprised everyone in the future), and it was almost used. In February, we can extract lane line data around the corresponding car through high-speed high-precision maps. When it was made, I remember everyone was still very excited.
Figure 1: The effect of high-precision map lane lines projected onto the image system
As you can see from Figure 1, there are still some fitting problems problem, so Xiaoxuan made a series of optimizations. Two months later, Xiaoxuan went to do other tasks. Looking back now, we have taken the right step in exploring BEV lane lines. Because in 2021 and 22, many excellent BEV lane line papers and codes have been gradually open sourced. Seeing this, you may think that there must be a perfect story about the implementation of BEV lane lines in 2023. However, ideals are often very fulfilling, but the reality is very cruel.
23 years of stumbling
Because our BEV obstacles have proven that BEV can go down this road, and it has also shown good results in road tests. The group began to have more resources to consider lane lines. Note that this is not BEV. why? Because at this time, we were facing a lot of pressure to go online, and we did not have enough experience in BEV lane lines. In other words, there were almost no people in the entire group who had done mass production of 2D lane lines. In the first half of 2023, it can really be described as stumbling. We had many heated discussions internally, and finally decided to form two lines, one of which is the 2D lane line: most of the manpower is on the 2D lane line, and the focus is on the 2D lane line. Post-processing, light model, and accumulation of lane line post-processing mass production experience through 2D lane lines. One line is the BEV lane line: there is only a small number of manpower (actually only 1-2 manpower), focusing on the model design of the BEV lane line and accumulating model experience. There are already many BEV lane marking networks. I will post two papers that have a great impact on us here for your reference. "HDMapNet: An Online HD Map Construction and Evaluation Framework" and "MapTR: Structured Modeling and Learning for Online Vectorized HD Map Construction"
However, a story often has twists and turns. We can only use high-speed high-precision maps to produce lane line data. What to do about the city? There are still so many bad cases that need to be solved. At this time, the important person will finally appear. Let's call him Classmate Xiaotang (the big steward of our data group). Classmate Xiaotang and the others used point cloud reconstruction to reconstruct the clip for us ( This process was quite painful. I remember those two months were the most stressful time for them, haha. Of course, classmate Xiaotang and we often fell in love and killed each other. , after all, I often say that there is no data again during meetings. ). Then how to label after reconstruction? Looking at the suppliers at the time, none of them had such labeling tools, let alone labeling experience. Together with Xiaotang and others, after a long month, the annotation tool was finally polished with the supplier. (We often joke that we are empowering the entire self-driving annotation industry. This process is really painful, and rebuilding clips is really slow to load). However, the whole labeling is still relatively slow or expensive. At this time, Xiaoxuan made his debut with his large model of lane line pre-labeling (the effect of the large model of lane line pre-labeling is still outstanding), and everyone looked at him with amazement. In sparkling. After this set of combinations, our lane line data production is finally almost ready. In August, our BEV lane line control lane line has been iterated well, which is suitable for simple high-speed piloting functions. Now Xiaoxuan is still bringing us more surprises in the pre-marked direction of the large model. We and Xiaotang are still in love with each other.
However, a story does not end so easily. In September, we started working on multi-modal (Lidar, camera, Radar) multi-task (lane lines, obstacles, Occ) pre-fusion models. It will also subsequently support City Navigation (NCP), a so-called solution that emphasizes perception and ignores maps. Based on the experience of BEV obstacles and BEV lane lines, we will soon deploy the converged network on vehicles, probably by the end of September. Many subtasks have also been added to lane lines, such as road sign recognition, intersection topology, etc. In this process, we upgraded the post-processing of BEV lane lines, abandoned the lane line cubic spline fitting, and adopted a point tracking scheme. The output of the point tracking scheme and our lane line model can be easily Good combination. This process was also painful. We held a special meeting once a week for 2 consecutive months. After all, we have done well based on the fitting plan, but in order to reach a higher limit, we can only suffer from pain and happiness. Finally, we have already put the basic functions into road testing.
Let me briefly explain Figure 4. The left side is the effect of lane line point tracking. Currently, the perception range of our model is only the first 80 meters. You can see that there are some points behind the car, which are left by tracking. On the right is the real-time perceptual map we have established. Of course, it is still in a rapid iteration process, and there are still many problems being solved.
The new beginning of 24 years
At this moment, standing in 24 years and looking back at our growth and accumulation from 21 years to now, I am very fortunate to be at that point in 21 years , I have the opportunity to do BEV, and I am very fortunate to have a group of like-minded friends who can help each other along the way. In 24 years, there are many things for us to pursue, including the mass production of pre-fusion models, efforts in data direction, exploration of timing models, end-to-end imagination, etc.
The above is the detailed content of A little bit about the implementation of BEV lane lines. For more information, please follow other related articles on the PHP Chinese website!
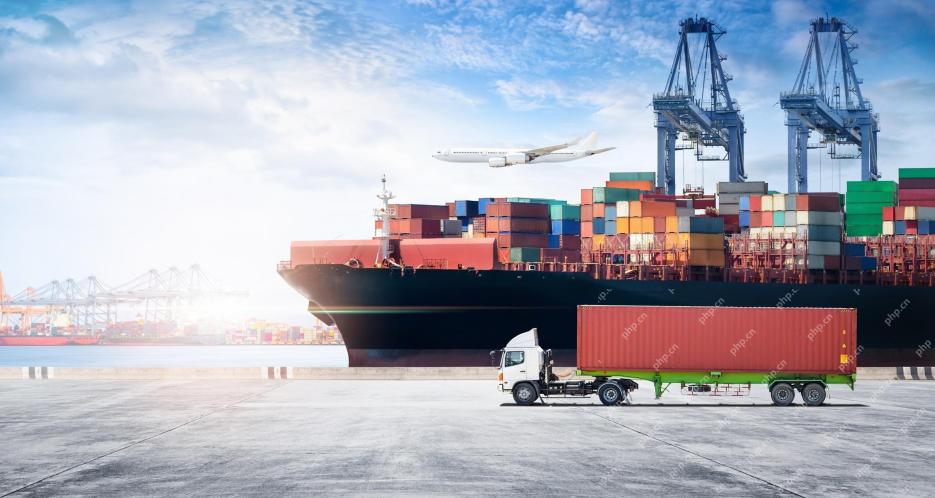
The term "AI-ready workforce" is frequently used, but what does it truly mean in the supply chain industry? According to Abe Eshkenazi, CEO of the Association for Supply Chain Management (ASCM), it signifies professionals capable of critic
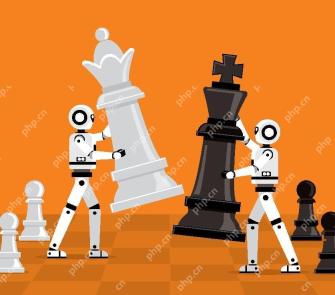
The decentralized AI revolution is quietly gaining momentum. This Friday in Austin, Texas, the Bittensor Endgame Summit marks a pivotal moment, transitioning decentralized AI (DeAI) from theory to practical application. Unlike the glitzy commercial
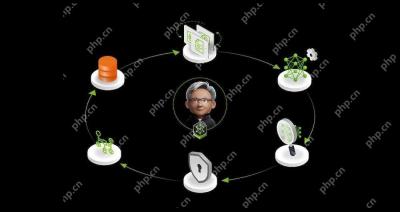
Enterprise AI faces data integration challenges The application of enterprise AI faces a major challenge: building systems that can maintain accuracy and practicality by continuously learning business data. NeMo microservices solve this problem by creating what Nvidia describes as "data flywheel", allowing AI systems to remain relevant through continuous exposure to enterprise information and user interaction. This newly launched toolkit contains five key microservices: NeMo Customizer handles fine-tuning of large language models with higher training throughput. NeMo Evaluator provides simplified evaluation of AI models for custom benchmarks. NeMo Guardrails implements security controls to maintain compliance and appropriateness
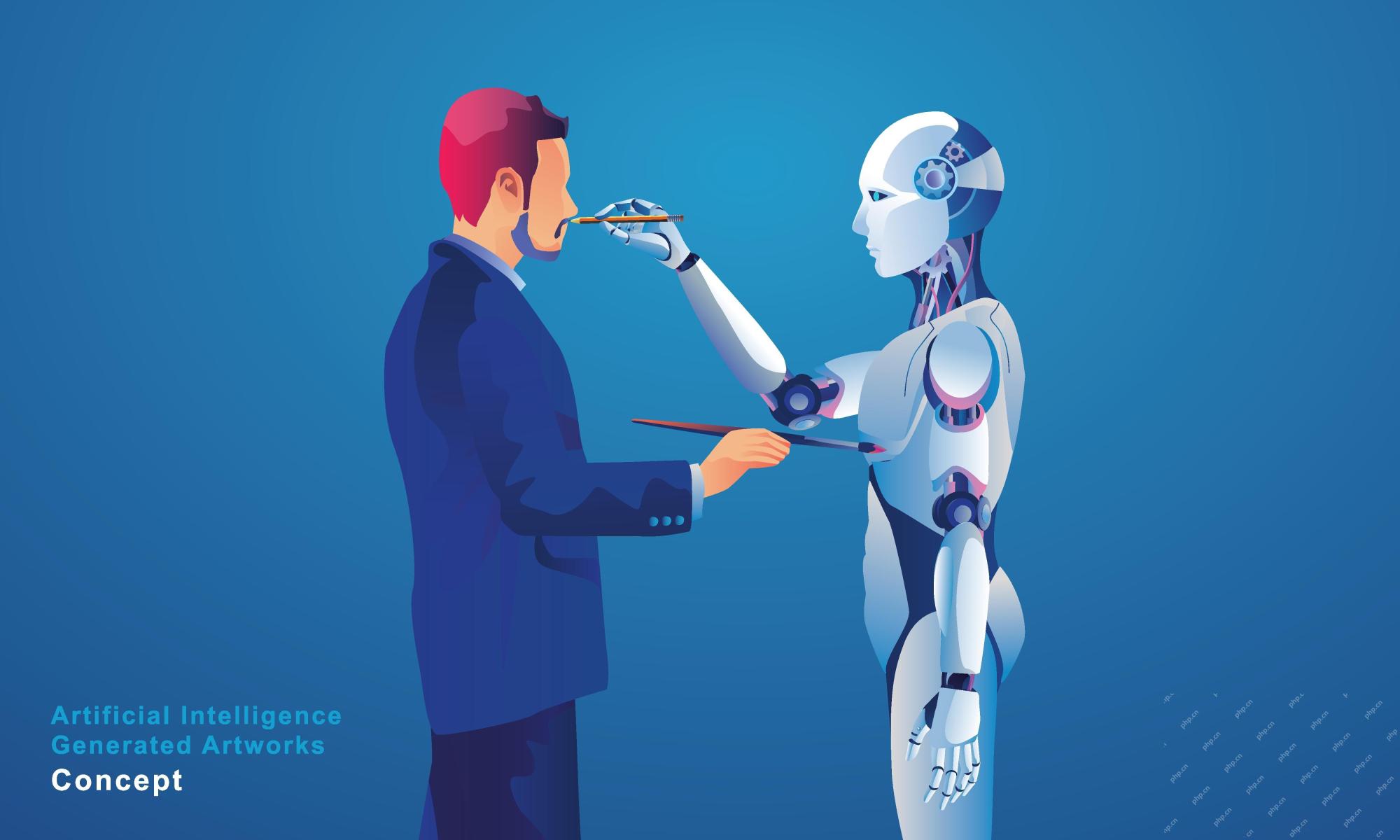
AI: The Future of Art and Design Artificial intelligence (AI) is changing the field of art and design in unprecedented ways, and its impact is no longer limited to amateurs, but more profoundly affecting professionals. Artwork and design schemes generated by AI are rapidly replacing traditional material images and designers in many transactional design activities such as advertising, social media image generation and web design. However, professional artists and designers also find the practical value of AI. They use AI as an auxiliary tool to explore new aesthetic possibilities, blend different styles, and create novel visual effects. AI helps artists and designers automate repetitive tasks, propose different design elements and provide creative input. AI supports style transfer, which is to apply a style of image
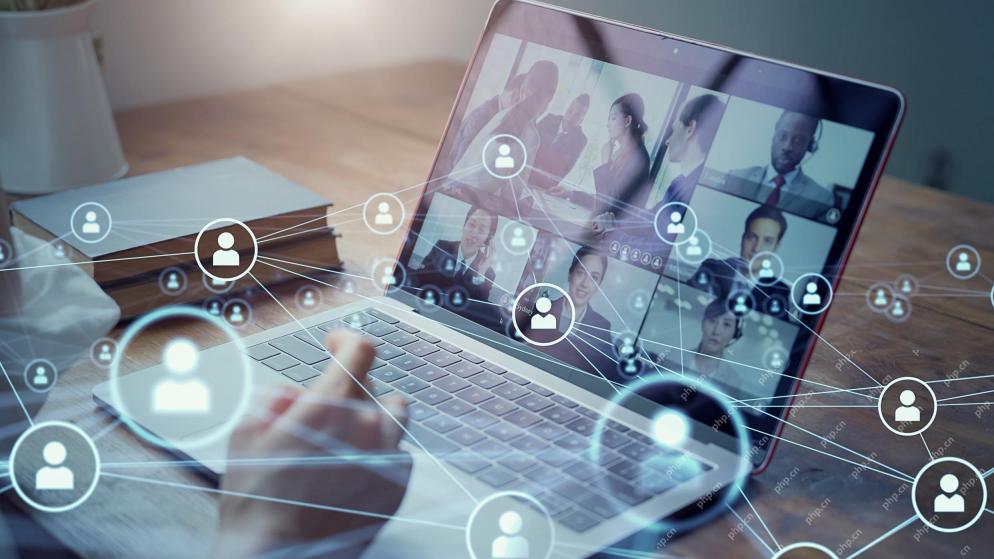
Zoom, initially known for its video conferencing platform, is leading a workplace revolution with its innovative use of agentic AI. A recent conversation with Zoom's CTO, XD Huang, revealed the company's ambitious vision. Defining Agentic AI Huang d
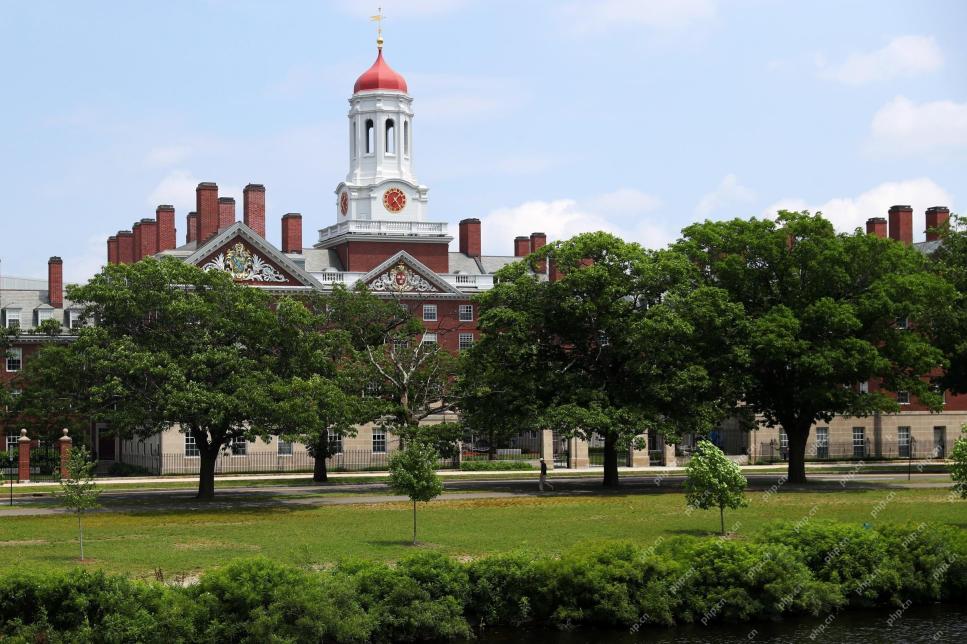
Will AI revolutionize education? This question is prompting serious reflection among educators and stakeholders. The integration of AI into education presents both opportunities and challenges. As Matthew Lynch of The Tech Edvocate notes, universit
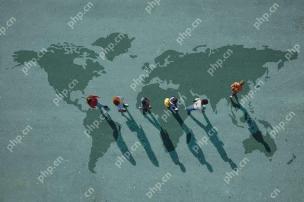
The development of scientific research and technology in the United States may face challenges, perhaps due to budget cuts. According to Nature, the number of American scientists applying for overseas jobs increased by 32% from January to March 2025 compared with the same period in 2024. A previous poll showed that 75% of the researchers surveyed were considering searching for jobs in Europe and Canada. Hundreds of NIH and NSF grants have been terminated in the past few months, with NIH’s new grants down by about $2.3 billion this year, a drop of nearly one-third. The leaked budget proposal shows that the Trump administration is considering sharply cutting budgets for scientific institutions, with a possible reduction of up to 50%. The turmoil in the field of basic research has also affected one of the major advantages of the United States: attracting overseas talents. 35
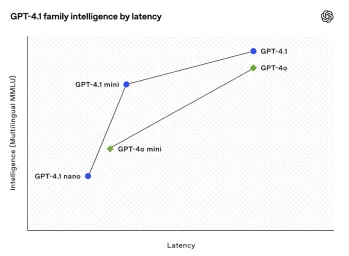
OpenAI unveils the powerful GPT-4.1 series: a family of three advanced language models designed for real-world applications. This significant leap forward offers faster response times, enhanced comprehension, and drastically reduced costs compared t


Hot AI Tools

Undresser.AI Undress
AI-powered app for creating realistic nude photos

AI Clothes Remover
Online AI tool for removing clothes from photos.

Undress AI Tool
Undress images for free

Clothoff.io
AI clothes remover

Video Face Swap
Swap faces in any video effortlessly with our completely free AI face swap tool!

Hot Article

Hot Tools

MinGW - Minimalist GNU for Windows
This project is in the process of being migrated to osdn.net/projects/mingw, you can continue to follow us there. MinGW: A native Windows port of the GNU Compiler Collection (GCC), freely distributable import libraries and header files for building native Windows applications; includes extensions to the MSVC runtime to support C99 functionality. All MinGW software can run on 64-bit Windows platforms.

PhpStorm Mac version
The latest (2018.2.1) professional PHP integrated development tool

SublimeText3 Linux new version
SublimeText3 Linux latest version

mPDF
mPDF is a PHP library that can generate PDF files from UTF-8 encoded HTML. The original author, Ian Back, wrote mPDF to output PDF files "on the fly" from his website and handle different languages. It is slower than original scripts like HTML2FPDF and produces larger files when using Unicode fonts, but supports CSS styles etc. and has a lot of enhancements. Supports almost all languages, including RTL (Arabic and Hebrew) and CJK (Chinese, Japanese and Korean). Supports nested block-level elements (such as P, DIV),
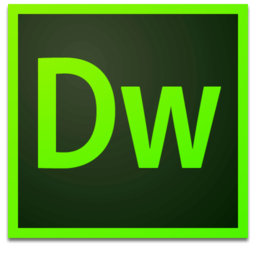
Dreamweaver Mac version
Visual web development tools
