Python Data Analysis: An Essential Guide for Data Scientists
introduction python is a powerful programming language that is highly respected in the field of data science. Its extensive library and tools package makes data processing, analysis and visualization a breeze. This article will delve into all aspects of Pythondata analysis to provide a comprehensive guide for data scientists.
Data processing and preparation Data processing is a key step in data analysis. Python provides a series of libraries, such as NumPy and pandas, for data structures, operations and cleaning. These libraries allow you to easily do the following:
import numpy as np import pandas as pd # 读取CSV文件 data = pd.read_csv("data.csv") # 清除缺失值 data.fillna(data.mean(), inplace=True) # 转换数据类型 data["Age"] = data["Age"].astype("int64")
data visualization Data Visualization is essential for revealing patterns and trends in data. Python provides libraries such as Matplotlib and Seaborn for creating various types of charts and graphs.
import matplotlib.pyplot as plt # 柱状图 data.Age.value_counts().plot.bar() plt.title("年龄分布") plt.show() # 散点图 plt.scatter(data["Age"], data["Salary"]) plt.xlabel("年龄") plt.ylabel("工资") plt.show()
Machine Learning Machine learning is an important part of data analysis, used to learn patterns from data and predict future outcomes. Python has libraries such as Scikit-learn, which can easily implement various machine learning algorithms.
from sklearn.linear_model import LinearRegression # 创建线性回归模型 model = LinearRegression() # 拟合模型 model.fit(data[["Age"]], data["Salary"]) # 预测工资 predicted_salary = model.predict([[30]])
deep learning Deep Learning is an advanced form of machine learning that is particularly suitable for processing large amounts of complex data. Python has libraries such as Tensorflow and PyTorch for building and training deep learning models.
import tensorflow as tf # 创建序列模型 model = tf.keras.Sequential([ tf.keras.layers.Dense(128, activation="relu"), tf.keras.layers.Dense(1) ]) # 编译模型 model.compile(optimizer="adam", loss="mean_squared_error") # 训练模型 model.fit(data[["Age"]], data["Salary"], epochs=100) # 评估模型 loss, mse = model.evaluate(data[["Age"]], data["Salary"])
in conclusion Python provides powerful tools and libraries for data science. By mastering the techniques outlined in this article, data scientists can effectively process, analyze, and visualize data, and leverage machine learning and deep learning algorithms to derive valuable insights from the data.
The above is the detailed content of Python Data Analysis: An Essential Guide for Data Scientists. For more information, please follow other related articles on the PHP Chinese website!
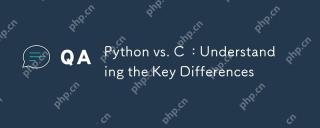
Python and C each have their own advantages, and the choice should be based on project requirements. 1) Python is suitable for rapid development and data processing due to its concise syntax and dynamic typing. 2)C is suitable for high performance and system programming due to its static typing and manual memory management.
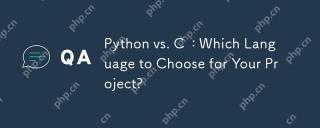
Choosing Python or C depends on project requirements: 1) If you need rapid development, data processing and prototype design, choose Python; 2) If you need high performance, low latency and close hardware control, choose C.
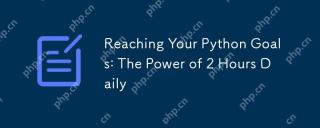
By investing 2 hours of Python learning every day, you can effectively improve your programming skills. 1. Learn new knowledge: read documents or watch tutorials. 2. Practice: Write code and complete exercises. 3. Review: Consolidate the content you have learned. 4. Project practice: Apply what you have learned in actual projects. Such a structured learning plan can help you systematically master Python and achieve career goals.
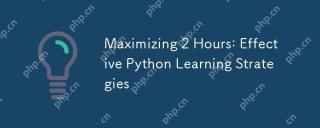
Methods to learn Python efficiently within two hours include: 1. Review the basic knowledge and ensure that you are familiar with Python installation and basic syntax; 2. Understand the core concepts of Python, such as variables, lists, functions, etc.; 3. Master basic and advanced usage by using examples; 4. Learn common errors and debugging techniques; 5. Apply performance optimization and best practices, such as using list comprehensions and following the PEP8 style guide.
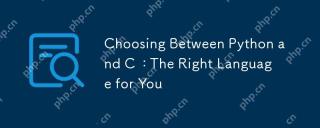
Python is suitable for beginners and data science, and C is suitable for system programming and game development. 1. Python is simple and easy to use, suitable for data science and web development. 2.C provides high performance and control, suitable for game development and system programming. The choice should be based on project needs and personal interests.
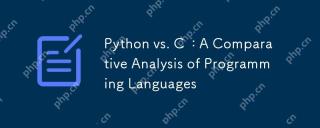
Python is more suitable for data science and rapid development, while C is more suitable for high performance and system programming. 1. Python syntax is concise and easy to learn, suitable for data processing and scientific computing. 2.C has complex syntax but excellent performance and is often used in game development and system programming.
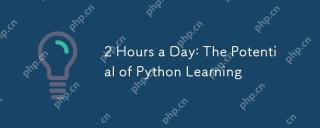
It is feasible to invest two hours a day to learn Python. 1. Learn new knowledge: Learn new concepts in one hour, such as lists and dictionaries. 2. Practice and exercises: Use one hour to perform programming exercises, such as writing small programs. Through reasonable planning and perseverance, you can master the core concepts of Python in a short time.
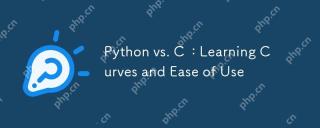
Python is easier to learn and use, while C is more powerful but complex. 1. Python syntax is concise and suitable for beginners. Dynamic typing and automatic memory management make it easy to use, but may cause runtime errors. 2.C provides low-level control and advanced features, suitable for high-performance applications, but has a high learning threshold and requires manual memory and type safety management.


Hot AI Tools

Undresser.AI Undress
AI-powered app for creating realistic nude photos

AI Clothes Remover
Online AI tool for removing clothes from photos.

Undress AI Tool
Undress images for free

Clothoff.io
AI clothes remover

Video Face Swap
Swap faces in any video effortlessly with our completely free AI face swap tool!

Hot Article

Hot Tools

Atom editor mac version download
The most popular open source editor

SublimeText3 Linux new version
SublimeText3 Linux latest version

mPDF
mPDF is a PHP library that can generate PDF files from UTF-8 encoded HTML. The original author, Ian Back, wrote mPDF to output PDF files "on the fly" from his website and handle different languages. It is slower than original scripts like HTML2FPDF and produces larger files when using Unicode fonts, but supports CSS styles etc. and has a lot of enhancements. Supports almost all languages, including RTL (Arabic and Hebrew) and CJK (Chinese, Japanese and Korean). Supports nested block-level elements (such as P, DIV),

Zend Studio 13.0.1
Powerful PHP integrated development environment

SecLists
SecLists is the ultimate security tester's companion. It is a collection of various types of lists that are frequently used during security assessments, all in one place. SecLists helps make security testing more efficient and productive by conveniently providing all the lists a security tester might need. List types include usernames, passwords, URLs, fuzzing payloads, sensitive data patterns, web shells, and more. The tester can simply pull this repository onto a new test machine and he will have access to every type of list he needs.