


Existing large model alignment methods include examplebased supervised fine-tuning (SFT) and score feedbackbased reinforcement learning (RLHF). However, the score can only reflect the quality of the current response and cannot clearly indicate the shortcomings of the model. In contrast, we humans typically learn and adjust our behavioral patterns from verbal feedback. Just like the review comments are not just a score, but also include many reasons for acceptance or rejection.
So, can large language models also use language feedback to improve themselves like humans?
Researchers from the Chinese University of Hong Kong and Tencent AI Lab recently proposed an innovative research called Contrastive Unlikelihood Learning (CUT). The research uses language feedback to adjust language models so that they can learn and improve from different criticisms, just like humans. This research aims to improve the quality and accuracy of language models to make them more consistent with the way humans think. By comparing non-likelihood training, researchers hope to enable the language model to better understand and adapt to diverse language usage situations, thereby improving its performance in natural language processing tasks. This innovative research is expected to provide a simple and effective method for language models
#CUT. By using only 1317 pieces of language feedback data, CUT was able to significantly improve the winning rate of LLaMA2-13b on AlpacaEval, soaring from 1.87% to 62.56%, and successfully defeated 175B DaVinci003. What’s exciting is that CUT can also perform iterative cycles of exploration, criticism, and improvement like other reinforcement learning and reinforcement learning reinforcement feedback (RLHF) frameworks. In this process, the criticism stage can be completed by the automatic evaluation model to achieve self-evaluation and improvement of the entire system.
The author conducted four rounds of iterations on LLaMA2-chat-13b, gradually improving the model's performance on AlpacaEval from 81.09% to 91.36%. Compared with alignment technology based on score feedback (DPO), CUT performs better under the same data size. The results reveal that language feedback has great potential for development in the field of alignment, opening up new possibilities for future alignment research. This finding has important implications for improving the accuracy and efficiency of alignment techniques and provides guidance for achieving better natural language processing tasks.
- ##Paper title: Reasons to Reject? Aligning Language Models with Judgments
- Paper link: https://arxiv.org/abs/2312.14591
- Github link: https://github.com/ wwxu21/CUT
Alignment of large models
Based on existing work , researchers summarized two common large model alignment methods:
1. Learning from Demonstration: Based on ready-made instructions - Reply Yes, using supervised training methods to align large models.
Advantages: stable training; simple implementation.- Disadvantages: The cost of collecting high-quality and diverse example data is high; it is impossible to learn from error responses; example data is often irrelevant to the model.
- 2. Learning from Rewards: Score the command-reply pair and use reinforcement learning to train the model to maximize its response score.
Advantages: Correct responses and error responses can be used simultaneously; feedback signals are related to the model.
- Disadvantages: The feedback signal is sparse; the training process is often complicated.
- This study focuses on learning from Language feedback (Learning from Judgments): giving instructions - replying to writing comments, based on the language feedback Improve the defects of the model and maintain the advantages of the model, thereby improving the model performance.
It can be seen that language feedback inherits the advantages of score feedback. Compared with score feedback, verbal feedback is more informative: instead of letting the model guess what it did right and what it went wrong, verbal feedback can directly point out detailed deficiencies and directions for improvement. Unfortunately, however, researchers have found that there is currently no effective way to fully utilize verbal feedback. To this end, researchers have proposed an innovative framework, CUT, designed to take full advantage of language feedback.
Contrastive non-likelihood training
The core idea of CUT is to learn from contrast. Researchers compare the responses of large models under different conditions to find out which parts are satisfactory and should be maintained, and which parts are flawed and need to be modified. Based on this, researchers use maximum likelihood estimation (MLE) to train the satisfactory part, and use unlikelihood training (UT) to modify the flaws in the reply.
1. Alignment scenario: As shown in the figure above, the researchers considered two alignment scenarios:
a): This is a commonly understood alignment scenario where a reply needs to faithfully follow instructions and be consistent with human expectations and values.
b): This scenario introduces verbal feedback as an additional condition. In this scenario, the response must satisfy both instructions and verbal feedback. For example, when receiving a negative feedback, the large model needs to make mistakes based on the issues mentioned in the corresponding feedback.
2. Alignment data: As shown in the figure above, based on the above two alignment scenarios, researchers constructed three types of alignment data:
a) Align-P: The large model generated satisfactory responses and thus received positive feedback. Obviously, Align-P satisfies alignment in both and
scenarios.
b) Align-N: The large model generated flawed (blue bold) replies and therefore received negative feedback. For Align-N, alignment is not satisfied in . But after considering this negative feedback, Align-N is still aligned in the
scenario.
c) Misalign: Real negative feedback in Align-N is replaced with a fake positive feedback. Obviously, Misalign does not satisfy alignment in both and
scenarios.
3. Learn from contrast:
a) Align-N vs. Misalign: The difference between the two is mainly the degree of alignment under . Given the powerful in-context learning capabilities of large models, the alignment polarity flip from Align-N to Misalign is usually accompanied by a significant change in the generation probability of specific words, especially those words that are closely related to real negative feedback. . As shown in the figure above, under the condition of Align-N (left channel), the probability of large model generating "a" is significantly higher than Misalign (right channel). And the place where the probability changes significantly is where the big model makes a mistake.
In order to learn from this comparison, the researchers input Align-N and Misalign data to the large model at the same time to obtain the generation probabilities of the output words under the two conditionsand
. Words that have a significantly higher generation probability in the
condition than in the
condition are marked as inappropriate words. Specifically, researchers used the following criteria to quantify the definition of inappropriate words:
where is Hyperparameters that trade off precision and recall during inappropriate word recognition.
The researchers used unlikelihood training (UT) on these identified inappropriate words, thereby forcing the large model to explore more satisfactory responses. For other reply words, researchers still use maximum likelihood estimation (MLE) to optimize:
where is a hyperparameter that controls the proportion of non-likelihood training,
is the number of reply words.
b) Align-P v.s. Align-N: The difference between the two mainly lies in the degree of alignment under . Essentially, the large model controls the quality of the output reply by introducing language feedback of different polarities. Therefore, the comparison between the two can inspire large models to distinguish satisfactory responses from defective responses. Specifically, we learn from this set of comparisons via the following maximum likelihood estimation (MLE) loss:
where is an indicator function that returns 1 if the data satisfies
alignment, otherwise it returns 0.
CUT’s final training goal combines the above two sets of comparisons: .
Experimental evaluation
1. Offline alignment
In order to save Qian, the researchers first tried to use existing language feedback data to align large models. This experiment was used to demonstrate CUT's ability to utilize language feedback.
a) General model
As shown in the table above, for general model alignment, the researchers used 1317 alignment data provided by Shepherd to compare CUT under cold start (LLaMA2) and hot start (LLaMA2-chat) conditions. versus existing methods for learning from linguistic feedback.
Under the cold start experiment based on LLaMA2, CUT significantly surpassed existing alignment methods on the AlpacaEval test platform, fully proving its advantages in utilizing language feedback. Moreover, CUT has also achieved significant improvements in TruthfulQA compared to the base model, which reveals that CUT has great potential in alleviating the hallucination problem of large models.
In the hot start scenario based on LLaMA2-chat, existing methods perform poorly in improving LLaMA2-chat and even have negative effects. However, CUT can further improve the performance of the base model on this basis, once again verifying the great potential of CUT in utilizing language feedback.
b) Expert model
The researchers also tested on specific expert tasks (text abstract ) on the CUT alignment effect. As shown in the table above, CUT also achieves significant improvements compared to existing alignment methods on expert tasks.
2. Online alignment
Research on offline alignment has successfully demonstrated the powerful alignment performance of CUT. Now, researchers are further exploring online alignment scenarios that are closer to practical applications. In this scenario, researchers iteratively annotate the responses of the target large model with language feedback so that the target model can be more accurately aligned based on the language feedback associated with it. The specific process is as follows:
-
Step 1: Collect instructions
, and obtain the reply from the target large model
.
-
Step 2: In response to the above instruction-reply pair, mark the language feedback
.
-
Step 3: Use CUT to fine-tune the target large model based on the collected triplet data
.
As shown in the figure above, after four rounds of online alignment iterations, CUT has only Under the conditions of 4000 training data and a small 13B model size, it can still achieve an impressive score of 91.36. This achievement further demonstrates CUT’s excellent performance and huge potential.
3. AI comment model
Annotation taking into account language feedback To reduce the cost, researchers try to train a judgment model to automatically annotate language feedback for the target large model. As shown in the figure above, the researchers used 5,000 pieces (AI Judge-5000) and 3,000 pieces (AI Judge-3000) of language feedback data to train two review models. Both review models have achieved remarkable results in optimizing the target large-scale model, especially the effect of AI Judge-5000.
This proves the feasibility of using AI comment models to align target large models, and also highlights the importance of comment model quality in the entire alignment process. This set of experiments also provides strong support for reducing annotation costs in the future.
4. Language feedback vs. score feedback
In order to deeply explore the huge potential of language feedback in large model alignment, researchers compared CUT based on language feedback with the method based on score feedback (DPO). In order to ensure a fair comparison, the researchers selected 4,000 sets of the same instruction-response pairs as experimental samples, allowing CUT and DPO to learn from the score feedback and language feedback corresponding to these data respectively.
As shown in the table above, CUT performed significantly better than DPO in the cold start (LLaMA2) experiment. In the hot start (LLaMA2-chat) experiment, CUT can achieve results comparable to DPO on tasks such as ARC, HellaSwag, MMLU, and TruthfulQA, and is significantly ahead of DPO on the AlpacaEval task. This experiment confirmed the greater potential and advantages of linguistic feedback compared to fractional feedback during large model alignment.
Summary and Challenges
In this work, the researchers systematically explored the current status and innovation of language feedback in large model alignment We proposed an alignment framework CUT based on language feedback, revealing the great potential and advantages of language feedback in the field of large-scale model alignment. In addition, there are some new directions and challenges in the research of language feedback, such as:
1. The quality of the comment model: Although research The researchers have successfully demonstrated the feasibility of training a review model, but when observing the model output, they still find that the review model often gives less than accurate reviews. Therefore, improving the quality of the review model is of great significance for large-scale use of language feedback for alignment in the future.
2. Introduction of new knowledge: When language feedback involves knowledge that the large model lacks, even if the large model can accurately Errors were identified, but there was no clear direction for correction. Therefore, it is very important to supplement the knowledge that the large model lacks while aligning.
3. Multi-modal alignment: The success of language models has promoted the research of multi-modal large models, such as language, speech, A combination of images and videos. In these multi-modal scenarios, studying language feedback and feedback of corresponding modalities has ushered in new definitions and challenges.
The above is the detailed content of Learn and grow from criticism like humans, 1317 comments increased LLaMA2's winning rate by 30 times. For more information, please follow other related articles on the PHP Chinese website!
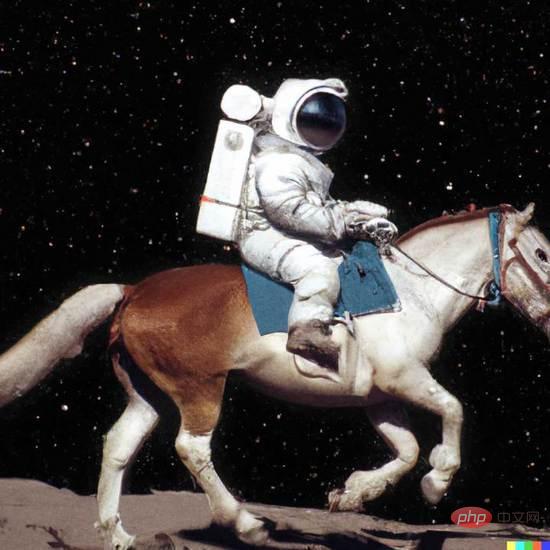
1 前言在发布DALL·E的15个月后,OpenAI在今年春天带了续作DALL·E 2,以其更加惊艳的效果和丰富的可玩性迅速占领了各大AI社区的头条。近年来,随着生成对抗网络(GAN)、变分自编码器(VAE)、扩散模型(Diffusion models)的出现,深度学习已向世人展现其强大的图像生成能力;加上GPT-3、BERT等NLP模型的成功,人类正逐步打破文本和图像的信息界限。在DALL·E 2中,只需输入简单的文本(prompt),它就可以生成多张1024*1024的高清图像。这些图像甚至
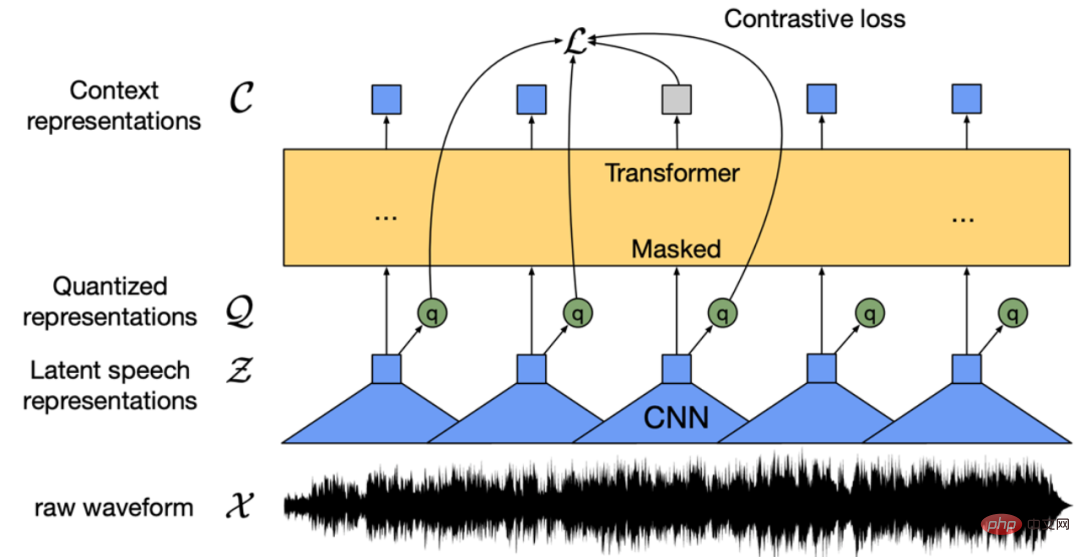
Wav2vec 2.0 [1],HuBERT [2] 和 WavLM [3] 等语音预训练模型,通过在多达上万小时的无标注语音数据(如 Libri-light )上的自监督学习,显著提升了自动语音识别(Automatic Speech Recognition, ASR),语音合成(Text-to-speech, TTS)和语音转换(Voice Conversation,VC)等语音下游任务的性能。然而这些模型都没有公开的中文版本,不便于应用在中文语音研究场景。 WenetSpeech [4] 是
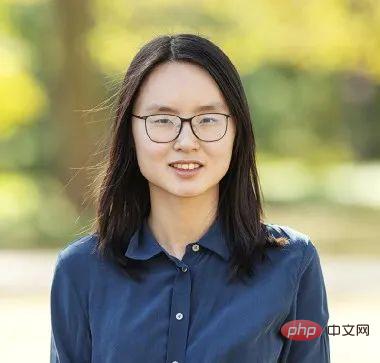
“Making large models smaller”这是很多语言模型研究人员的学术追求,针对大模型昂贵的环境和训练成本,陈丹琦在智源大会青源学术年会上做了题为“Making large models smaller”的特邀报告。报告中重点提及了基于记忆增强的TRIME算法和基于粗细粒度联合剪枝和逐层蒸馏的CofiPruning算法。前者能够在不改变模型结构的基础上兼顾语言模型困惑度和检索速度方面的优势;而后者可以在保证下游任务准确度的同时实现更快的处理速度,具有更小的模型结构。陈丹琦 普
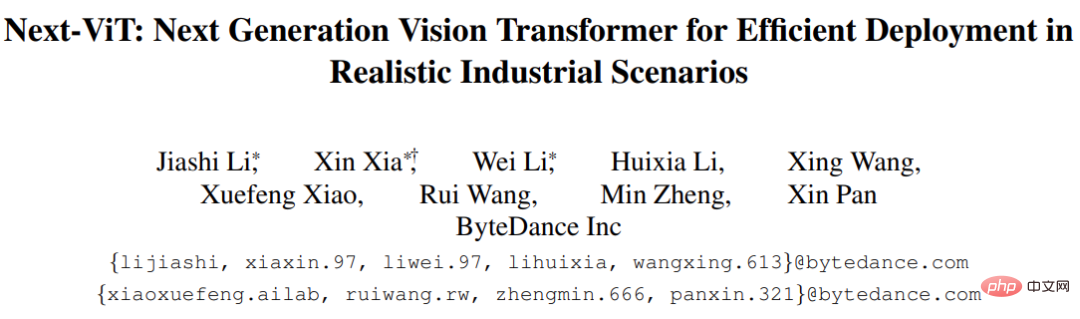
由于复杂的注意力机制和模型设计,大多数现有的视觉 Transformer(ViT)在现实的工业部署场景中不能像卷积神经网络(CNN)那样高效地执行。这就带来了一个问题:视觉神经网络能否像 CNN 一样快速推断并像 ViT 一样强大?近期一些工作试图设计 CNN-Transformer 混合架构来解决这个问题,但这些工作的整体性能远不能令人满意。基于此,来自字节跳动的研究者提出了一种能在现实工业场景中有效部署的下一代视觉 Transformer——Next-ViT。从延迟 / 准确性权衡的角度看,
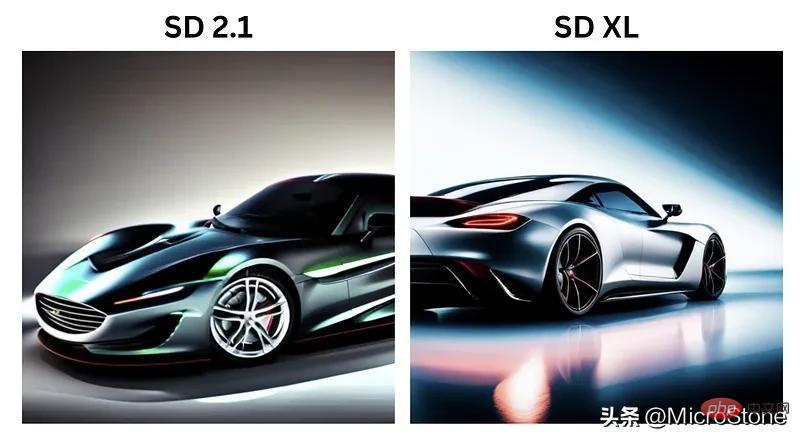
3月27号,Stability AI的创始人兼首席执行官Emad Mostaque在一条推文中宣布,Stable Diffusion XL 现已可用于公开测试。以下是一些事项:“XL”不是这个新的AI模型的官方名称。一旦发布稳定性AI公司的官方公告,名称将会更改。与先前版本相比,图像质量有所提高与先前版本相比,图像生成速度大大加快。示例图像让我们看看新旧AI模型在结果上的差异。Prompt: Luxury sports car with aerodynamic curves, shot in a
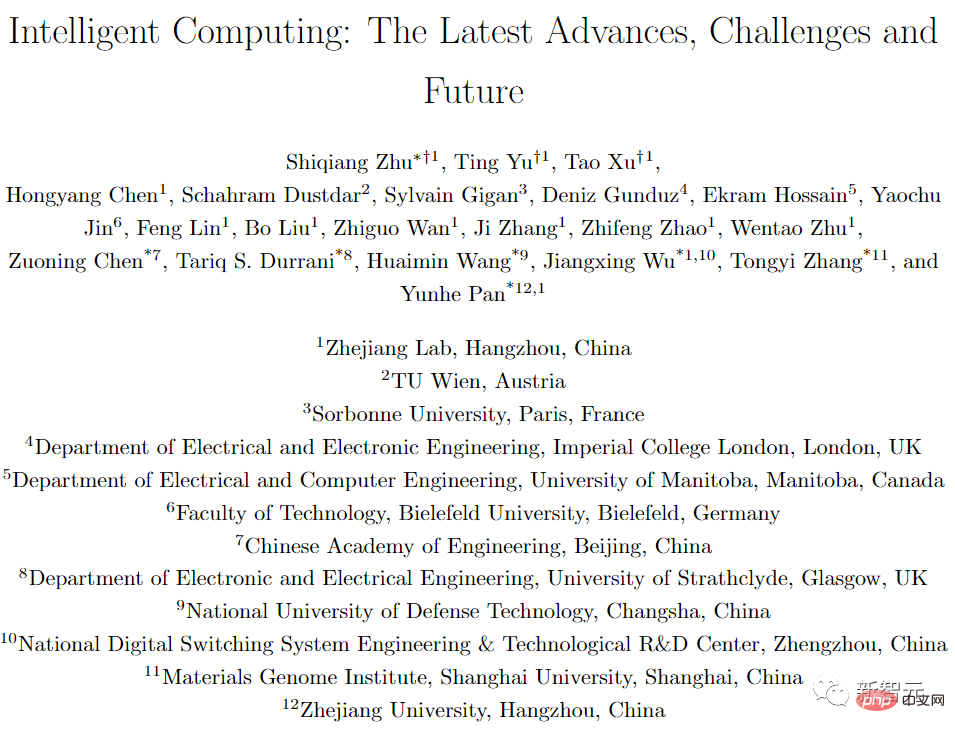
人工智能就是一个「拼财力」的行业,如果没有高性能计算设备,别说开发基础模型,就连微调模型都做不到。但如果只靠拼硬件,单靠当前计算性能的发展速度,迟早有一天无法满足日益膨胀的需求,所以还需要配套的软件来协调统筹计算能力,这时候就需要用到「智能计算」技术。最近,来自之江实验室、中国工程院、国防科技大学、浙江大学等多达十二个国内外研究机构共同发表了一篇论文,首次对智能计算领域进行了全面的调研,涵盖了理论基础、智能与计算的技术融合、重要应用、挑战和未来前景。论文链接:https://spj.scien
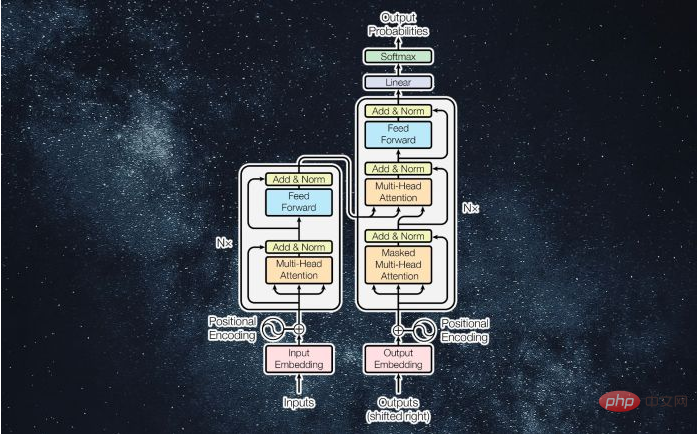
译者 | 李睿审校 | 孙淑娟近年来, Transformer 机器学习模型已经成为深度学习和深度神经网络技术进步的主要亮点之一。它主要用于自然语言处理中的高级应用。谷歌正在使用它来增强其搜索引擎结果。OpenAI 使用 Transformer 创建了著名的 GPT-2和 GPT-3模型。自从2017年首次亮相以来,Transformer 架构不断发展并扩展到多种不同的变体,从语言任务扩展到其他领域。它们已被用于时间序列预测。它们是 DeepMind 的蛋白质结构预测模型 AlphaFold
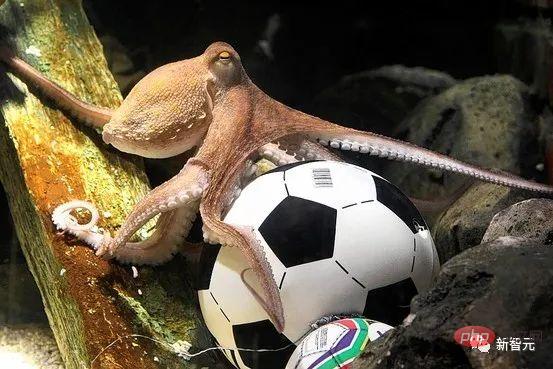
说起2010年南非世界杯的最大网红,一定非「章鱼保罗」莫属!这只位于德国海洋生物中心的神奇章鱼,不仅成功预测了德国队全部七场比赛的结果,还顺利地选出了最终的总冠军西班牙队。不幸的是,保罗已经永远地离开了我们,但它的「遗产」却在人们预测足球比赛结果的尝试中持续存在。在艾伦图灵研究所(The Alan Turing Institute),随着2022年卡塔尔世界杯的持续进行,三位研究员Nick Barlow、Jack Roberts和Ryan Chan决定用一种AI算法预测今年的冠军归属。预测模型图


Hot AI Tools

Undresser.AI Undress
AI-powered app for creating realistic nude photos

AI Clothes Remover
Online AI tool for removing clothes from photos.

Undress AI Tool
Undress images for free

Clothoff.io
AI clothes remover

AI Hentai Generator
Generate AI Hentai for free.

Hot Article

Hot Tools

Atom editor mac version download
The most popular open source editor
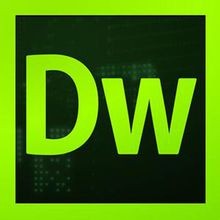
Dreamweaver CS6
Visual web development tools

Safe Exam Browser
Safe Exam Browser is a secure browser environment for taking online exams securely. This software turns any computer into a secure workstation. It controls access to any utility and prevents students from using unauthorized resources.

MantisBT
Mantis is an easy-to-deploy web-based defect tracking tool designed to aid in product defect tracking. It requires PHP, MySQL and a web server. Check out our demo and hosting services.

Zend Studio 13.0.1
Powerful PHP integrated development environment
