


Applying physically coupled graph neural networks to improve precipitation forecasting skills at the Institute of Atmospheric Physics, Chinese Academy of Sciences
Editor | ScienceAI
In the era of large models, the effects of purely data-driven meteorological and climate models have gradually caught up with or even surpassed numerical models.
However, existing large-scale meteorological and climate models still have some problems. For example, the physical consistency in the model is not high enough, which limits the ability to predict complex weather and climate phenomena such as precipitation. In addition, the forecast effect of divergent wind is not satisfactory. These issues require further research and improvement to improve the prediction accuracy and reliability of the model.
At present, combining physics, atmospheric dynamics and deep learning models is an important way to solve the bottleneck problem.
Recently, the team of researcher Huang Gang from the Institute of Atmospheric Physics, Chinese Academy of Sciences, based on the data and computing power support of the Earth System Numerical Simulation Device (Huan), starting from the perspective of the coupling relationship of physical variables, combined with the figure The neural network imposes physical soft constraints on multiple variables, improves the precipitation forecasting skills of numerical models, and makes some attempts and explorations in the integration of physics and AI.
The research was titled "Coupling Physical Factors for Precipitation Forecast in China With Graph Neural Network" and was published in "Geophysical Research Letters" on January 18.
Paper link: https://doi.org/10.1029/2023GL106676
In response to the difficult issues of precipitation forecasting, especially the forecasting of heavy precipitation, Our team solved the problem by starting from the influencing factors and occurrence mechanism of precipitation, combining the omega equation and the water vapor equation, etc., to screen variables and build a variable coupling graph network.
The Omega equation and the water vapor equation describe vertical movement and water vapor changes respectively, both of which are important factors affecting precipitation. From the perspective of graph network, the aforementioned equation reflects the relationship between the nonlinear combination of basic physical quantities (temperature, wind, humidity, etc.) and the key elements of precipitation. Therefore, it can be abstracted into a graph network, and through the variables between the graph network (nodes) and the relationships between variables to represent the combination and coupling between different physical variables.
At the same time, taking into account the impact of climate factors on weather scales, especially the systematic differences in model errors under different climate backgrounds, this study combined sparse data such as season, ENSO and other climate factors and reporting time Use entity embedding technology to embed the correction model to distinguish errors in different backgrounds.
In addition, for the precipitation process, this study made local improvements to the graph neural network ChebNet, so that it can basically maintain the effect while avoiding global operations and greatly reducing the computational complexity.
Figure 1: Schematic of the omega-GNN model. (Source: paper)
Model comparison results show that the two physically constrained models omega-GNN and omega-EGNN proposed in this study significantly improve the precipitation forecasting skills of each category compared with the numerical model, and at the same time their performance Better than the current mainstream deep learning models without physical constraints (such as U-NET, 3D-CNN, etc.).
In addition, this study performed ten sets of perturbations on all deep learning models, allowing them to perform ensemble forecasts. Combining diagnosis and case analysis, it is found that the model with physical constraints is significantly better than the model without physical constraints. For the prediction of heavy precipitation, the consistency between the omega-GNN model and the omega-EGNN model ensemble is higher, and the forecasting skills are better.
Figure 2: Each model’s (a) TS score, (b-g) spatial distribution of TS differences relative to the numerical model (precipitation above the 20mm/6h threshold). (Source: paper)
Researcher Huang Gang, the corresponding author of the paper, said: "Our team has accumulated a lot in the direction of climate dynamics. In recent years, we have made some attempts to use AI to improve weather and climate predictions, and have achieved many related results. Won relevant competition awards for the first time. In the era of large AI models, how to integrate physics with AI is a big issue, and there are many ways and ideas for integration. We combined some thoughts on atmosphere and climate dynamics to soft-soften the model from the perspective of physical coupling. Constraints, some attempts have been made in this direction, hoping to provide some incremental information for related fields."
The research was conducted by master students Chen Yutong, Dr. Wang Ya, Researcher Huang Gang and the Institute of Atmospheric Physics, Chinese Academy of Sciences Completed in collaboration with Dr. Tian Qun from the Guangzhou Institute of Tropical Marine Meteorology, China Meteorological Administration.
Reference content: https://iap.cas.cn/gb/xwdt/kyjz/202401/t20240119_6959543.html
The above is the detailed content of Applying physically coupled graph neural networks to improve precipitation forecasting skills at the Institute of Atmospheric Physics, Chinese Academy of Sciences. For more information, please follow other related articles on the PHP Chinese website!
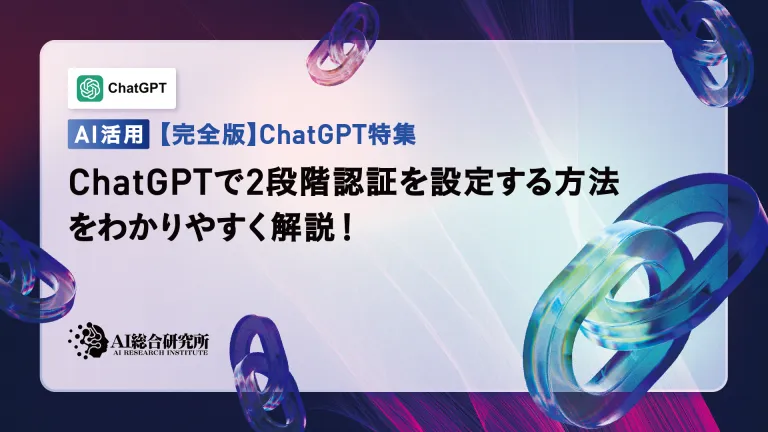
ChatGPT Security Enhanced: Two-Stage Authentication (2FA) Configuration Guide Two-factor authentication (2FA) is required as a security measure for online platforms. This article will explain in an easy-to-understand manner the 2FA setup procedure and its importance in ChatGPT. This is a guide for those who want to use ChatGPT safely. Click here for OpenAI's latest AI agent, OpenAI Deep Research ⬇️ [ChatGPT] What is OpenAI Deep Research? A thorough explanation of how to use it and the fee structure! table of contents ChatG
![[For businesses] ChatGPT training | A thorough introduction to 8 free training options, subsidies, and examples!](https://img.php.cn/upload/article/001/242/473/174704251871181.jpg?x-oss-process=image/resize,p_40)
The use of generated AI is attracting attention as the key to improving business efficiency and creating new businesses. In particular, OpenAI's ChatGPT has been adopted by many companies due to its versatility and accuracy. However, the shortage of personnel who can effectively utilize ChatGPT is a major challenge in implementing it. In this article, we will explain the necessity and effectiveness of "ChatGPT training" to ensure successful use of ChatGPT in companies. We will introduce a wide range of topics, from the basics of ChatGPT to business use, specific training programs, and how to choose them. ChatGPT training improves employee skills
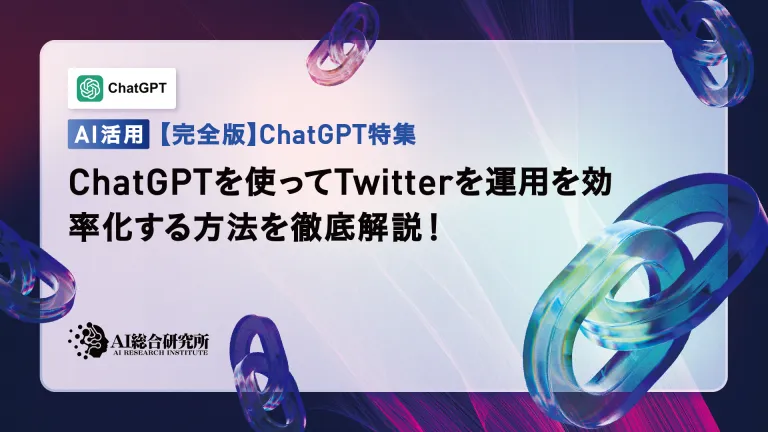
Improved efficiency and quality in social media operations are essential. Particularly on platforms where real-time is important, such as Twitter, requires continuous delivery of timely and engaging content. In this article, we will explain how to operate Twitter using ChatGPT from OpenAI, an AI with advanced natural language processing capabilities. By using ChatGPT, you can not only improve your real-time response capabilities and improve the efficiency of content creation, but you can also develop marketing strategies that are in line with trends. Furthermore, precautions for use
![[For Mac] Explaining how to get started and how to use the ChatGPT desktop app!](https://img.php.cn/upload/article/001/242/473/174704239752855.jpg?x-oss-process=image/resize,p_40)
ChatGPT Mac desktop app thorough guide: from installation to audio functions Finally, ChatGPT's desktop app for Mac is now available! In this article, we will thoroughly explain everything from installation methods to useful features and future update information. Use the functions unique to desktop apps, such as shortcut keys, image recognition, and voice modes, to dramatically improve your business efficiency! Installing the ChatGPT Mac version of the desktop app Access from a browser: First, access ChatGPT in your browser.
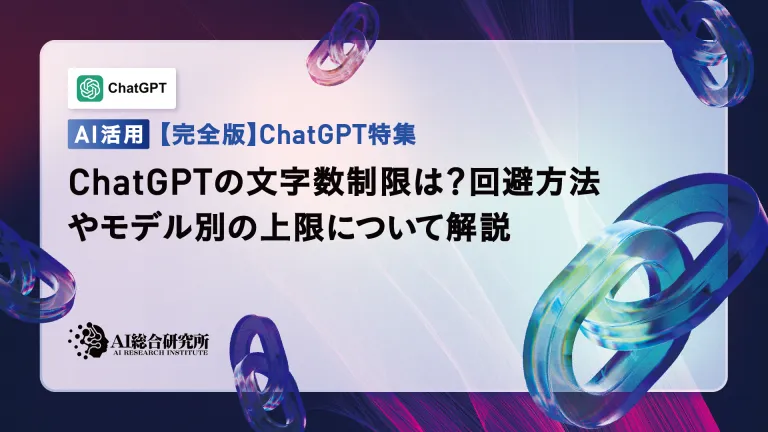
When using ChatGPT, have you ever had experiences such as, "The output stopped halfway through" or "Even though I specified the number of characters, it didn't output properly"? This model is very groundbreaking and not only allows for natural conversations, but also allows for email creation, summary papers, and even generate creative sentences such as novels. However, one of the weaknesses of ChatGPT is that if the text is too long, input and output will not work properly. OpenAI's latest AI agent, "OpenAI Deep Research"
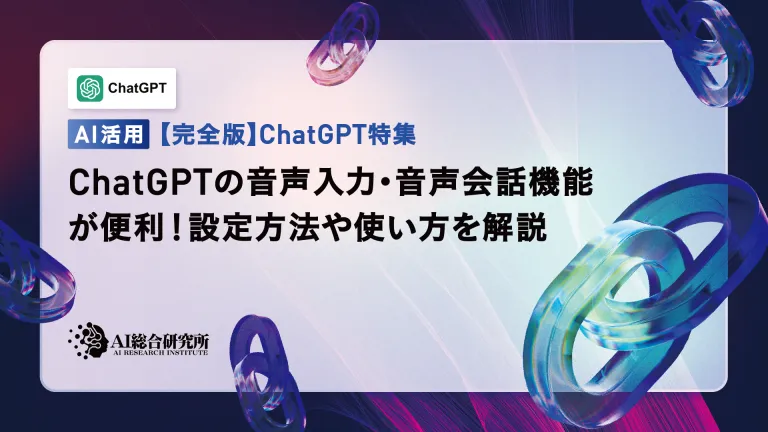
ChatGPT is an innovative AI chatbot developed by OpenAI. It not only has text input, but also features voice input and voice conversation functions, allowing for more natural communication. In this article, we will explain how to set up and use the voice input and voice conversation functions of ChatGPT. Even when you can't take your hands off, ChatGPT responds and responds with audio just by talking to you, which brings great benefits in a variety of situations, such as busy business situations and English conversation practice. A detailed explanation of how to set up the smartphone app and PC, as well as how to use each.
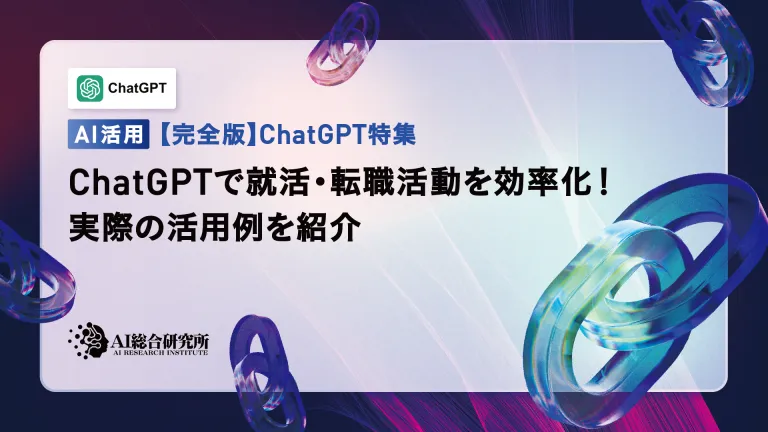
The shortcut to success! Effective job change strategies using ChatGPT In today's intensifying job change market, effective information gathering and thorough preparation are key to success. Advanced language models like ChatGPT are powerful weapons for job seekers. In this article, we will explain how to effectively utilize ChatGPT to improve your job hunting efficiency, from self-analysis to application documents and interview preparation. Save time and learn techniques to showcase your strengths to the fullest, and help you make your job search a success. table of contents Examples of job hunting using ChatGPT Efficiency in self-analysis: Chat
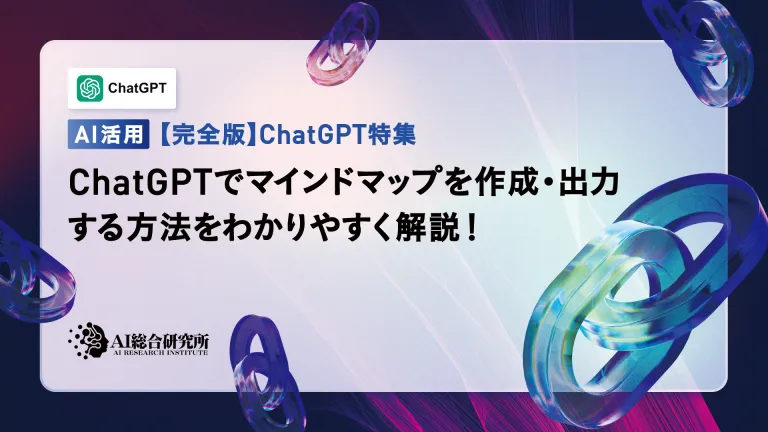
Mind maps are useful tools for organizing information and coming up with ideas, but creating them can take time. Using ChatGPT can greatly streamline this process. This article will explain in detail how to easily create mind maps using ChatGPT. Furthermore, through actual examples of creation, we will introduce how to use mind maps on various themes. Learn how to effectively organize and visualize your ideas and information using ChatGPT. OpenAI's latest AI agent, OpenA


Hot AI Tools

Undresser.AI Undress
AI-powered app for creating realistic nude photos

AI Clothes Remover
Online AI tool for removing clothes from photos.

Undress AI Tool
Undress images for free

Clothoff.io
AI clothes remover

Video Face Swap
Swap faces in any video effortlessly with our completely free AI face swap tool!

Hot Article

Hot Tools

SecLists
SecLists is the ultimate security tester's companion. It is a collection of various types of lists that are frequently used during security assessments, all in one place. SecLists helps make security testing more efficient and productive by conveniently providing all the lists a security tester might need. List types include usernames, passwords, URLs, fuzzing payloads, sensitive data patterns, web shells, and more. The tester can simply pull this repository onto a new test machine and he will have access to every type of list he needs.
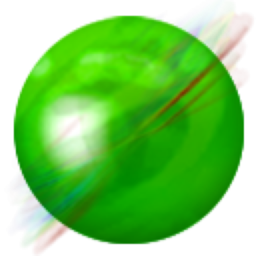
ZendStudio 13.5.1 Mac
Powerful PHP integrated development environment

MantisBT
Mantis is an easy-to-deploy web-based defect tracking tool designed to aid in product defect tracking. It requires PHP, MySQL and a web server. Check out our demo and hosting services.

MinGW - Minimalist GNU for Windows
This project is in the process of being migrated to osdn.net/projects/mingw, you can continue to follow us there. MinGW: A native Windows port of the GNU Compiler Collection (GCC), freely distributable import libraries and header files for building native Windows applications; includes extensions to the MSVC runtime to support C99 functionality. All MinGW software can run on 64-bit Windows platforms.

SublimeText3 Linux new version
SublimeText3 Linux latest version
