Introduction to Transformer positional encoding and how to improve it
Transformer is a deep learning model widely used in natural language processing tasks. It uses a self-attention mechanism to capture the relationship between words in the sequence, but ignores the position order of words in the sequence, which may lead to information loss. To solve this problem, Transformer introduces positional encoding. The basic principle of positional encoding is to assign each word in the sequence a position vector, which contains information about the position of the word in the sequence. This way, the model can take into account the positional information of the word by adding the position vector to the word embedding vector. A common method of position encoding is to use sine and cosine functions to generate position vectors. Specifically, for each position and each dimension, the value of the position vector is composed of a sine function and a cosine function. This encoding method allows the model to learn the relationship between different locations. In addition to traditional position encoding methods, some improved methods have been proposed. For example, one can use learned position encoding, where position vectors are learned through a neural network. This method can adaptively adjust the position vector during the training process to better capture the position information in the sequence. In short, the Transformer model uses positional encoding to consider the order of words
1. Basic principles
In Transformer, positional encoding is to convert position information Encoded into a vector. It is added with the word’s embedding vector to get the final representation of each word. The specific calculation method is as follows:
PE_{(i,2j)}=sin(\frac{i}{10000^{2j/d_{model}}})
PE_{(i,2j 1)}=cos(\frac{i}{10000^{2j/d_{model}}})
Among them, i is the position of the word, j is the dimension of the position encoding vector, and d_{model} is the dimension of the Transformer model. With this formula, we can calculate the position encoding value for each position and each dimension. We can combine these values into a positional encoding matrix and then add it to the word embedding matrix to obtain a positional encoding representation of each word.
2. Improvement methods
Although Transformer's positional encoding performs well in many tasks, there are still some improvements that can be used.
1. Learning positional encoding
In the traditional Transformer model, positional encoding is calculated based on a fixed formula, which cannot adapt to different tasks and specific needs of different data sets. Therefore, researchers have proposed some methods to learn positional encoding. One approach is to use neural networks to learn positional encodings. Specifically, researchers use autoencoders or convolutional neural networks to learn positional encoding so that the positional encoding can be adapted to the specific needs of the task and data set. The advantage of this method is that the position encoding can be adaptively adjusted, thereby improving the generalization ability of the model.
2. Random position encoding
Another improvement method is to use random position encoding. This method replaces the fixed position encoding formula by randomly sampling a set of position encoding vectors. The advantage of this method is that it can increase the diversity of the model, thereby improving the robustness and generalization ability of the model. However, since the random position encoding is randomly generated at each training time, more training time is required.
3. Multi-scale position encoding
Multi-scale position encoding is a method to improve position by combining multiple position encoding matrices Coding method. Specifically, the researchers added position encoding matrices at different scales to obtain a richer position encoding representation. The advantage of this method is that it can capture position information at different scales, thereby improving the performance of the model.
4. Local positional encoding
Local positional encoding is a method to improve positional encoding by limiting positional encoding to a local area. Specifically, the researchers limited the calculation of positional encoding to a certain range around the current word, thereby reducing the complexity of positional encoding. The advantage of this approach is that it can reduce computational costs while also improving model performance.
In short, Transformer positional encoding is an important technique that can help the model capture the positional information between words in the sequence, thereby improving the performance of the model. Although traditional positional encoding performs well in many tasks, there are some improvements that can be used. These improvement methods can be selected and combined according to the needs of the task and data set, thereby improving the performance of the model.
The above is the detailed content of Introduction to Transformer positional encoding and how to improve it. For more information, please follow other related articles on the PHP Chinese website!
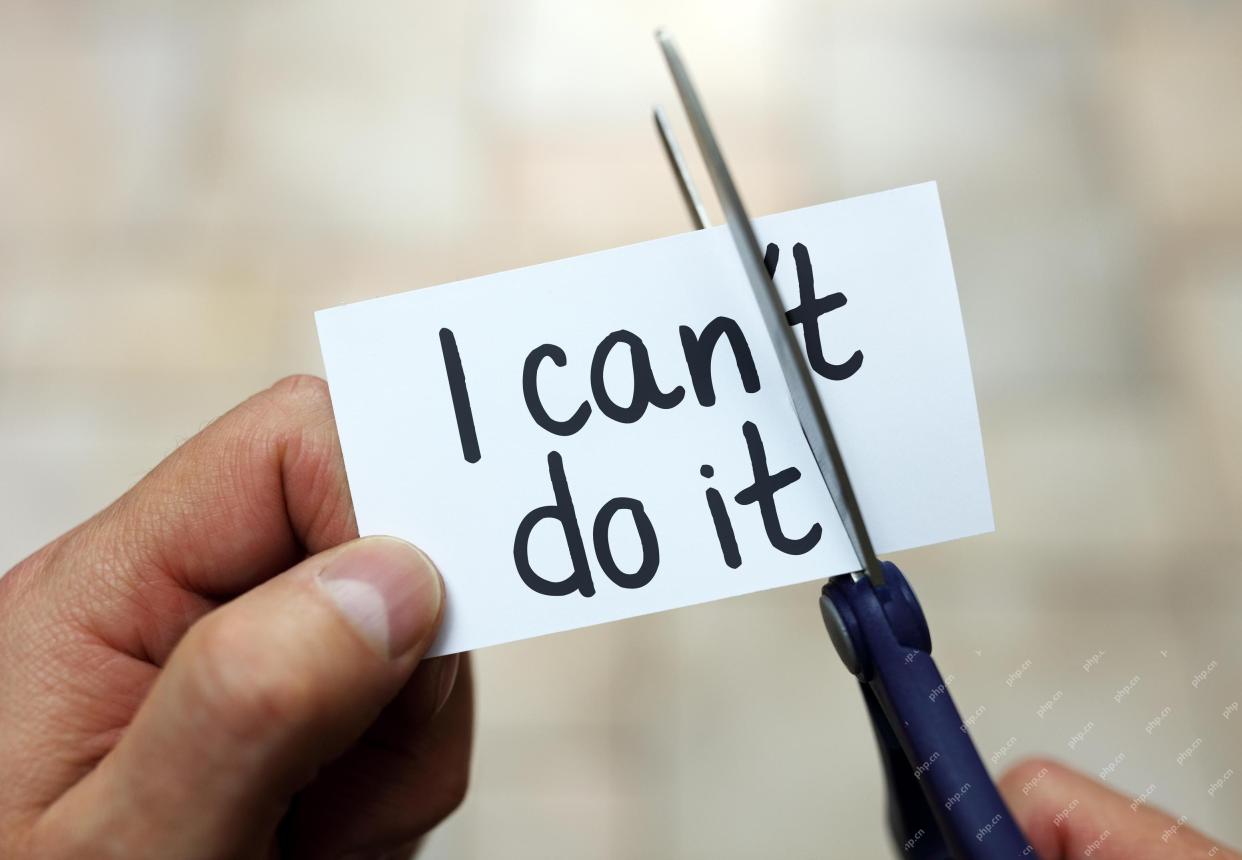
This article explores the growing concern of "AI agency decay"—the gradual decline in our ability to think and decide independently. This is especially crucial for business leaders navigating the increasingly automated world while retainin
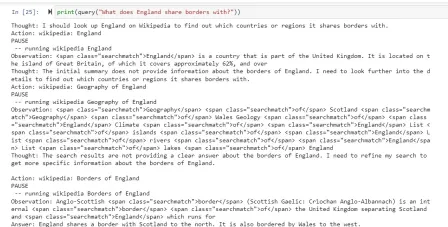
Ever wondered how AI agents like Siri and Alexa work? These intelligent systems are becoming more important in our daily lives. This article introduces the ReAct pattern, a method that enhances AI agents by combining reasoning an
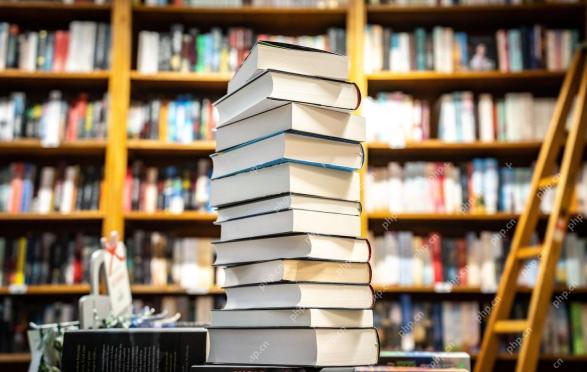
"I think AI tools are changing the learning opportunities for college students. We believe in developing students in core courses, but more and more people also want to get a perspective of computational and statistical thinking," said University of Chicago President Paul Alivisatos in an interview with Deloitte Nitin Mittal at the Davos Forum in January. He believes that people will have to become creators and co-creators of AI, which means that learning and other aspects need to adapt to some major changes. Digital intelligence and critical thinking Professor Alexa Joubin of George Washington University described artificial intelligence as a “heuristic tool” in the humanities and explores how it changes
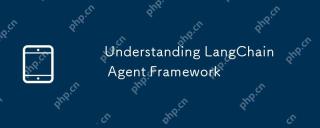
LangChain is a powerful toolkit for building sophisticated AI applications. Its agent architecture is particularly noteworthy, allowing developers to create intelligent systems capable of independent reasoning, decision-making, and action. This expl
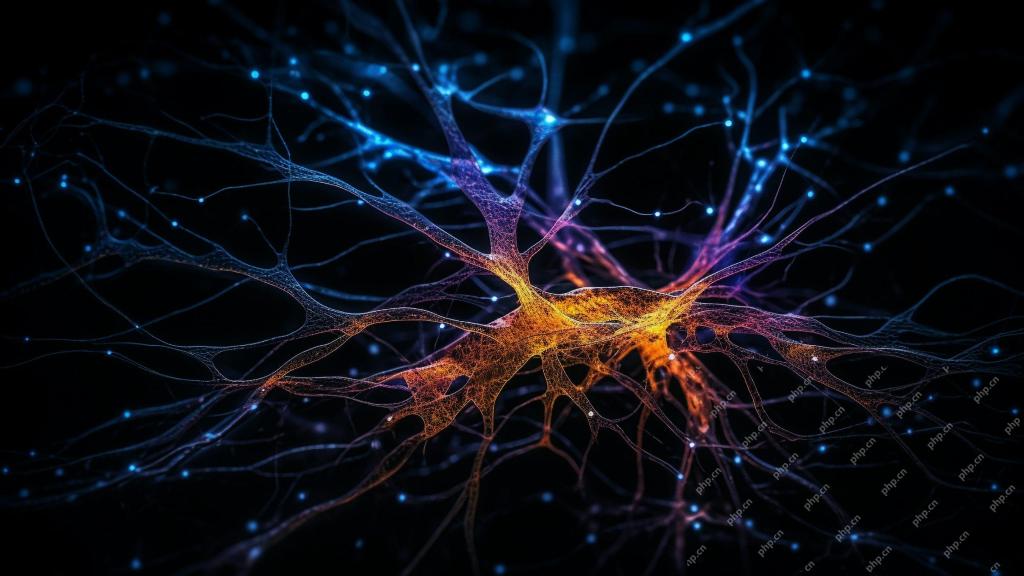
Radial Basis Function Neural Networks (RBFNNs): A Comprehensive Guide Radial Basis Function Neural Networks (RBFNNs) are a powerful type of neural network architecture that leverages radial basis functions for activation. Their unique structure make
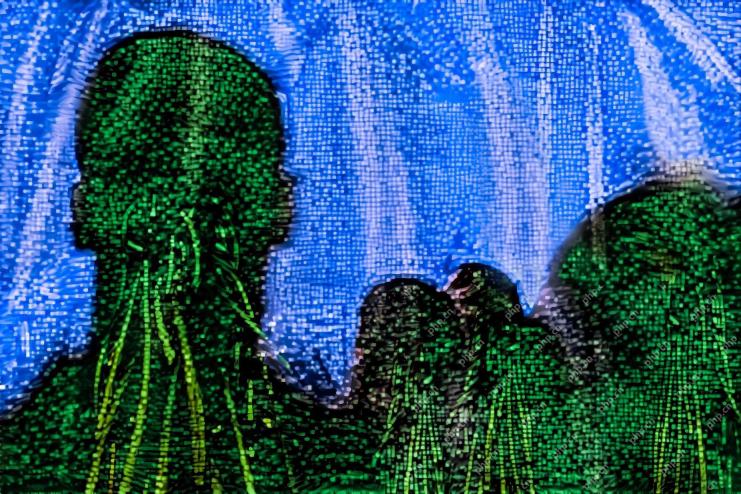
Brain-computer interfaces (BCIs) directly link the brain to external devices, translating brain impulses into actions without physical movement. This technology utilizes implanted sensors to capture brain signals, converting them into digital comman
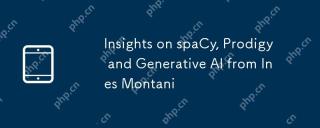
This "Leading with Data" episode features Ines Montani, co-founder and CEO of Explosion AI, and co-developer of spaCy and Prodigy. Ines offers expert insights into the evolution of these tools, Explosion's unique business model, and the tr
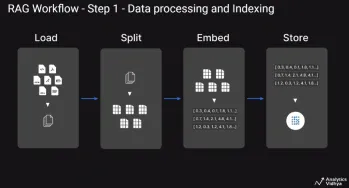
This article explores Retrieval Augmented Generation (RAG) systems and how AI agents can enhance their capabilities. Traditional RAG systems, while useful for leveraging custom enterprise data, suffer from limitations such as a lack of real-time dat


Hot AI Tools

Undresser.AI Undress
AI-powered app for creating realistic nude photos

AI Clothes Remover
Online AI tool for removing clothes from photos.

Undress AI Tool
Undress images for free

Clothoff.io
AI clothes remover

Video Face Swap
Swap faces in any video effortlessly with our completely free AI face swap tool!

Hot Article

Hot Tools

SecLists
SecLists is the ultimate security tester's companion. It is a collection of various types of lists that are frequently used during security assessments, all in one place. SecLists helps make security testing more efficient and productive by conveniently providing all the lists a security tester might need. List types include usernames, passwords, URLs, fuzzing payloads, sensitive data patterns, web shells, and more. The tester can simply pull this repository onto a new test machine and he will have access to every type of list he needs.
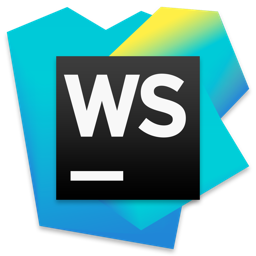
WebStorm Mac version
Useful JavaScript development tools

Atom editor mac version download
The most popular open source editor
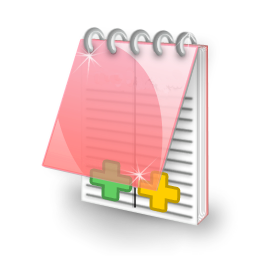
EditPlus Chinese cracked version
Small size, syntax highlighting, does not support code prompt function

DVWA
Damn Vulnerable Web App (DVWA) is a PHP/MySQL web application that is very vulnerable. Its main goals are to be an aid for security professionals to test their skills and tools in a legal environment, to help web developers better understand the process of securing web applications, and to help teachers/students teach/learn in a classroom environment Web application security. The goal of DVWA is to practice some of the most common web vulnerabilities through a simple and straightforward interface, with varying degrees of difficulty. Please note that this software