


Use pandas to read Excel files and easily implement data import and analysis
Use pandas to read Excel files and easily implement data import and analysis
pandas is a powerful tool for data analysis in Python. It can analyze various formats Data is processed flexibly and efficiently. In data analysis, Excel is a commonly used data format, and pandas provides a convenient interface that allows us to quickly import Excel files into data and analyze and process the data.
This article will introduce how to use the pandas library to read Excel files, and how to use pandas for data analysis, while providing code examples.
1. Reading Excel files
To read Excel files, you can use the read_excel function provided by pandas. This function can directly read the Excel file and convert it to the DataFrame data type. The following is a code example for reading an Excel file:
import pandas as pd # 读取Excel文件 filename = 'data.xlsx' df = pd.read_excel(filename) # 查看数据前5行 print(df.head())
In the above code, we first imported the pandas library and specified the alias as pd. Then use the pd.read_excel function to read the file data.xlsx and store the read data in a DataFrame named df. Finally, use the head method to view the first 5 rows of data.
2. Data analysis
- Data preprocessing
After the data is imported, we need to perform data preprocessing. Data preprocessing includes operations such as cleaning data, filling missing values, deduplication, and converting data types. The following is a sample code for data preprocessing:
# 删除含有缺失值的行 df = df.dropna() # 删除重复行 df = df.drop_duplicates() # 转换数据类型为float df['column1'] = df['column1'].astype(float) # 查看数据信息 print(df.info())
In the above code, we first use the dropna method to delete all rows containing missing values, and then use the drop_duplicates method to delete duplicate rows. Next, use the astype method to convert the data type of column1 to float type. Finally, use the info method to view the data information.
- Statistical analysis
Statistical analysis is one of the key steps in data analysis. Pandas provides a variety of methods to implement statistical analysis of data.
The following is a data analysis sample code:
# 计算各列的平均值、标准差、最大/最小值 print(df.mean()) print(df.std()) print(df.max()) print(df.min()) # 按照一列的值进行分组,并计算每组中数据的平均值 print(df.groupby('column1').mean()) # 绘制柱状图 df['column1'].plot(kind='bar')
In the above code, we use mean, std, max, and min to calculate the average, standard deviation, and maximum/minimum values of each column respectively. Then use the groupby method to group by the value of column1 and calculate the average of the data in each group. Finally, use the plot method to draw a histogram.
3. Summary
This article introduces how to use pandas to read Excel files and process and analyze the data. Pandas provides many convenient operations to make data analysis easier and more efficient. For data analysis and mining work, learning pandas will be very useful.
The above is the detailed content of Use pandas to read Excel files and easily implement data import and analysis. For more information, please follow other related articles on the PHP Chinese website!
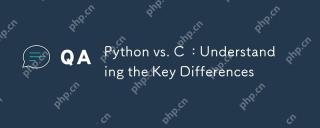
Python and C each have their own advantages, and the choice should be based on project requirements. 1) Python is suitable for rapid development and data processing due to its concise syntax and dynamic typing. 2)C is suitable for high performance and system programming due to its static typing and manual memory management.
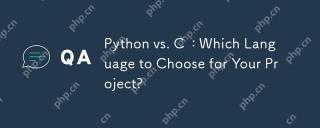
Choosing Python or C depends on project requirements: 1) If you need rapid development, data processing and prototype design, choose Python; 2) If you need high performance, low latency and close hardware control, choose C.
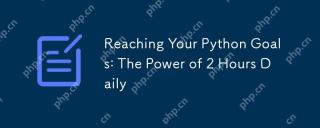
By investing 2 hours of Python learning every day, you can effectively improve your programming skills. 1. Learn new knowledge: read documents or watch tutorials. 2. Practice: Write code and complete exercises. 3. Review: Consolidate the content you have learned. 4. Project practice: Apply what you have learned in actual projects. Such a structured learning plan can help you systematically master Python and achieve career goals.
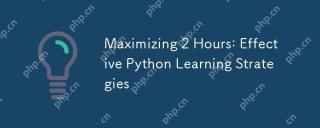
Methods to learn Python efficiently within two hours include: 1. Review the basic knowledge and ensure that you are familiar with Python installation and basic syntax; 2. Understand the core concepts of Python, such as variables, lists, functions, etc.; 3. Master basic and advanced usage by using examples; 4. Learn common errors and debugging techniques; 5. Apply performance optimization and best practices, such as using list comprehensions and following the PEP8 style guide.
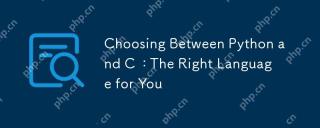
Python is suitable for beginners and data science, and C is suitable for system programming and game development. 1. Python is simple and easy to use, suitable for data science and web development. 2.C provides high performance and control, suitable for game development and system programming. The choice should be based on project needs and personal interests.
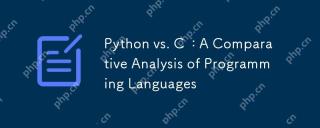
Python is more suitable for data science and rapid development, while C is more suitable for high performance and system programming. 1. Python syntax is concise and easy to learn, suitable for data processing and scientific computing. 2.C has complex syntax but excellent performance and is often used in game development and system programming.
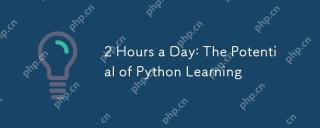
It is feasible to invest two hours a day to learn Python. 1. Learn new knowledge: Learn new concepts in one hour, such as lists and dictionaries. 2. Practice and exercises: Use one hour to perform programming exercises, such as writing small programs. Through reasonable planning and perseverance, you can master the core concepts of Python in a short time.
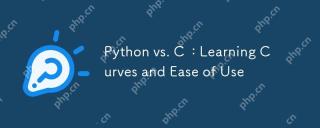
Python is easier to learn and use, while C is more powerful but complex. 1. Python syntax is concise and suitable for beginners. Dynamic typing and automatic memory management make it easy to use, but may cause runtime errors. 2.C provides low-level control and advanced features, suitable for high-performance applications, but has a high learning threshold and requires manual memory and type safety management.


Hot AI Tools

Undresser.AI Undress
AI-powered app for creating realistic nude photos

AI Clothes Remover
Online AI tool for removing clothes from photos.

Undress AI Tool
Undress images for free

Clothoff.io
AI clothes remover

Video Face Swap
Swap faces in any video effortlessly with our completely free AI face swap tool!

Hot Article

Hot Tools
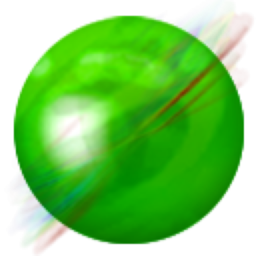
ZendStudio 13.5.1 Mac
Powerful PHP integrated development environment
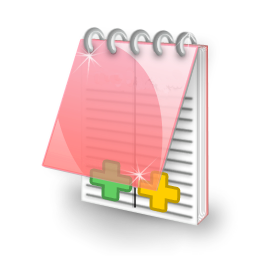
EditPlus Chinese cracked version
Small size, syntax highlighting, does not support code prompt function

MinGW - Minimalist GNU for Windows
This project is in the process of being migrated to osdn.net/projects/mingw, you can continue to follow us there. MinGW: A native Windows port of the GNU Compiler Collection (GCC), freely distributable import libraries and header files for building native Windows applications; includes extensions to the MSVC runtime to support C99 functionality. All MinGW software can run on 64-bit Windows platforms.

SublimeText3 Chinese version
Chinese version, very easy to use

Notepad++7.3.1
Easy-to-use and free code editor