


Introducing a large domestic open source MoE model, its performance is comparable to Llama 2-7B, while the calculation amount is reduced by 60%
The open source MoE model finally welcomes its first domestic player!
Its performance is not inferior to the dense Llama 2-7B model, but the calculation amount is only 40%.
This model can be called a 19-sided warrior, especially in terms of math and coding capabilities, crushing Llama.
It is the Deep Search team’s latest open source 16 billion parameter expert model DeepSeek MoE.
In addition to its excellent performance, DeepSeek MoE's main focus is to save computing power.
In this performance-activation parameter diagram, it "singles out" and occupies a large blank area in the upper left corner.
Only one day after its release, the DeepSeek team’s tweet on X received a large number of retweets and attention.
Maxime Labonne, a machine learning engineer at JP Morgan, also said after testing that the chat version of DeepSeek MoE performs slightly better than Microsoft's "small model" Phi-2.
At the same time, DeepSeek MoE also received 300 stars on GitHub and appeared on the homepage of the Hugging Face text generation model rankings.
So, what is the specific performance of DeepSeek MoE?
The amount of calculation is reduced by 60%
The current version of DeepSeek MoE has 16 billion parameters, and the actual number of activated parameters is about 2.8 billion.
Compared with its own 7B dense model, the performance of the two on the 19 data sets has different advantages and disadvantages, but the overall performance is relatively close.
Compared with Llama 2-7B, which is also a dense model, DeepSeek MoE also shows obvious advantages in mathematics, code, etc.
But the calculation amount of both dense models exceeds 180TFLOPs per 4k tokens, while DeepSeek MoE only has 74.4TFLOPs, which is only 40% of the two.
Performance tests conducted at 2 billion parameters show that DeepSeek MoE can also achieve the performance of the same MoE model with 1.5 times the number of parameters with less calculation. GShard 2.8B has comparable or even better results.
In addition, the Deep Seek team also fine-tuned the Chat version of DeepSeek MoE based on SFT, and its performance was also close to its own intensive version and Llama 2-7B.
In addition, the DeepSeek team also revealed that a 145B version of the DeepSeek MoE model is under development.
Phased preliminary tests show that the 145B DeepSeek MoE has a huge lead over the GShard 137B, and can achieve equivalent performance to the dense version of the DeepSeek 67B model with 28.5% of the calculation amount.
After the research and development is completed, the team will also open source the 145B version.
Behind the performance of these models is DeepSeek’s new self-developed MoE architecture.
Self-developed MoE new architecture
First of all, compared to the traditional MoE architecture, DeepSeek has a more fine-grained expert division.
When the total number of parameters is fixed, the traditional model can classify N experts, while DeepSeek may classify 2N experts.
At the same time, the number of experts selected each time a task is performed is twice that of the traditional model, so the overall number of parameters used remains the same, but the degree of freedom of selection increases.
This segmentation strategy allows for a more flexible and adaptive combination of activation experts, thereby improving the accuracy of the model on different tasks and the pertinence of knowledge acquisition.
In addition to the differences in expert division, DeepSeek also innovatively introduces the "shared expert" setting.
These shared experts activate tokens for all inputs and are not affected by the routing module. The purpose is to capture and integrate common knowledge that is needed in different contexts.
By compressing these shared knowledge into shared experts, parameter redundancy among other experts can be reduced, thereby improving the parameter efficiency of the model.
The setting of shared experts helps other experts focus more on their unique knowledge areas, thereby improving the overall level of expert specialization.
#Ablation experiment results show that both solutions play an important role in the "cost reduction and efficiency increase" of DeepSeek MoE.
Paper address: https://arxiv.org/abs/2401.06066.
Reference link: https://mp.weixin.qq.com/s/T9-EGxYuHcGQgXArLXGbgg.
The above is the detailed content of Introducing a large domestic open source MoE model, its performance is comparable to Llama 2-7B, while the calculation amount is reduced by 60%. For more information, please follow other related articles on the PHP Chinese website!
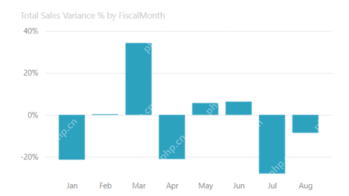
Harnessing the Power of Data Visualization with Microsoft Power BI Charts In today's data-driven world, effectively communicating complex information to non-technical audiences is crucial. Data visualization bridges this gap, transforming raw data i
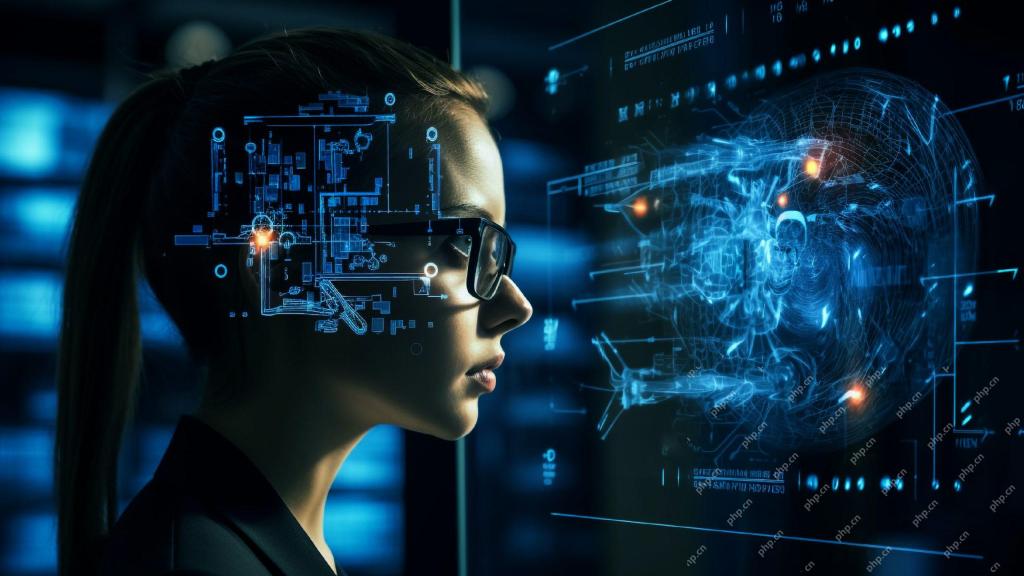
Expert Systems: A Deep Dive into AI's Decision-Making Power Imagine having access to expert advice on anything, from medical diagnoses to financial planning. That's the power of expert systems in artificial intelligence. These systems mimic the pro
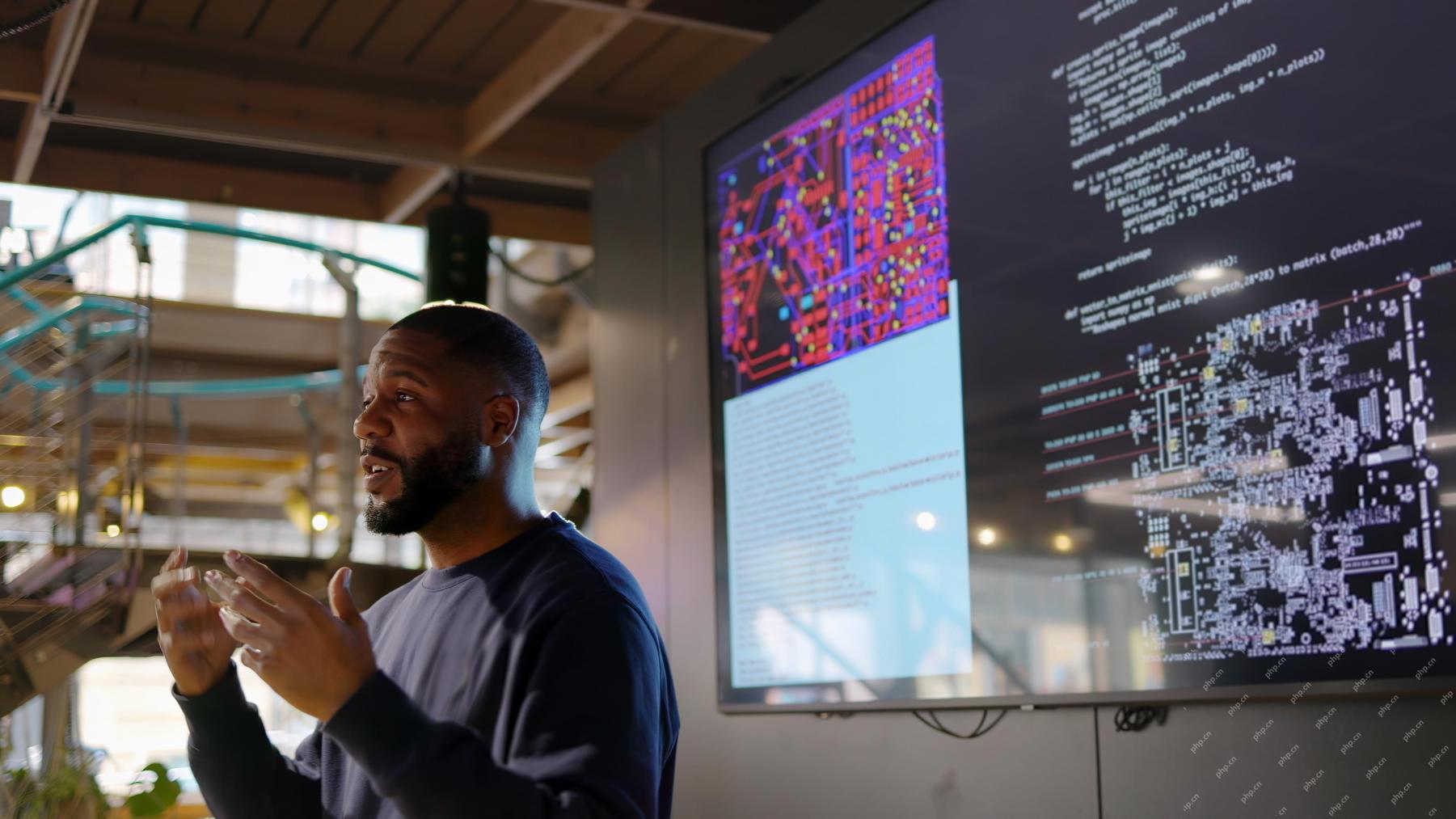
First of all, it’s apparent that this is happening quickly. Various companies are talking about the proportions of their code that are currently written by AI, and these are increasing at a rapid clip. There’s a lot of job displacement already around
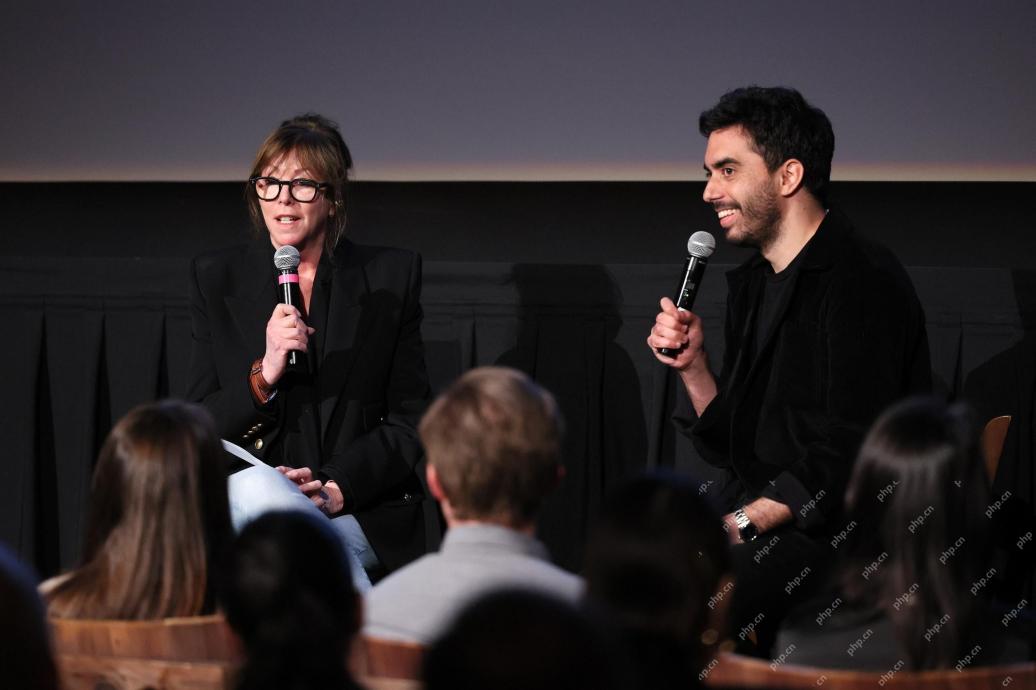
The film industry, alongside all creative sectors, from digital marketing to social media, stands at a technological crossroad. As artificial intelligence begins to reshape every aspect of visual storytelling and change the landscape of entertainment
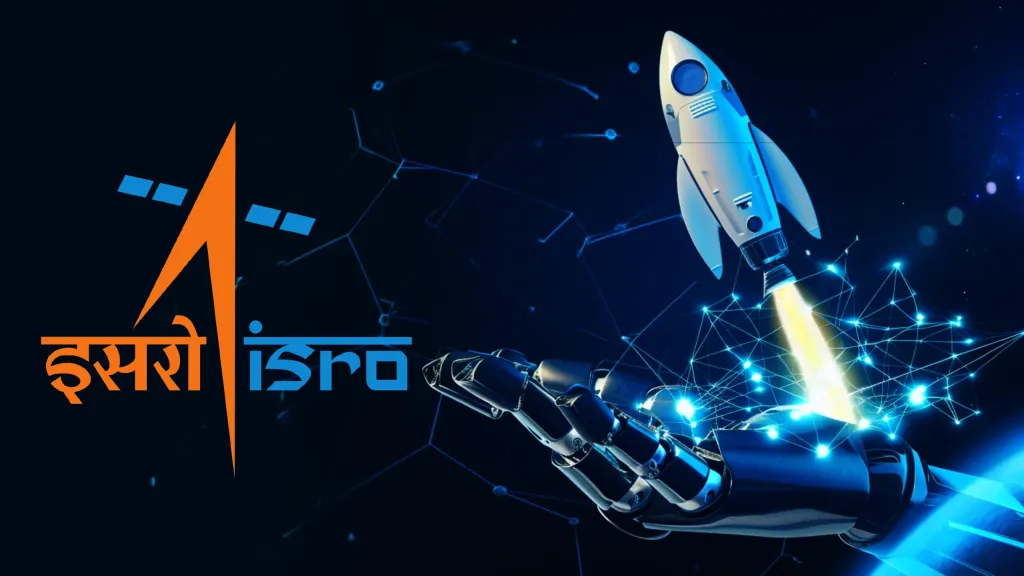
ISRO's Free AI/ML Online Course: A Gateway to Geospatial Technology Innovation The Indian Space Research Organisation (ISRO), through its Indian Institute of Remote Sensing (IIRS), is offering a fantastic opportunity for students and professionals to
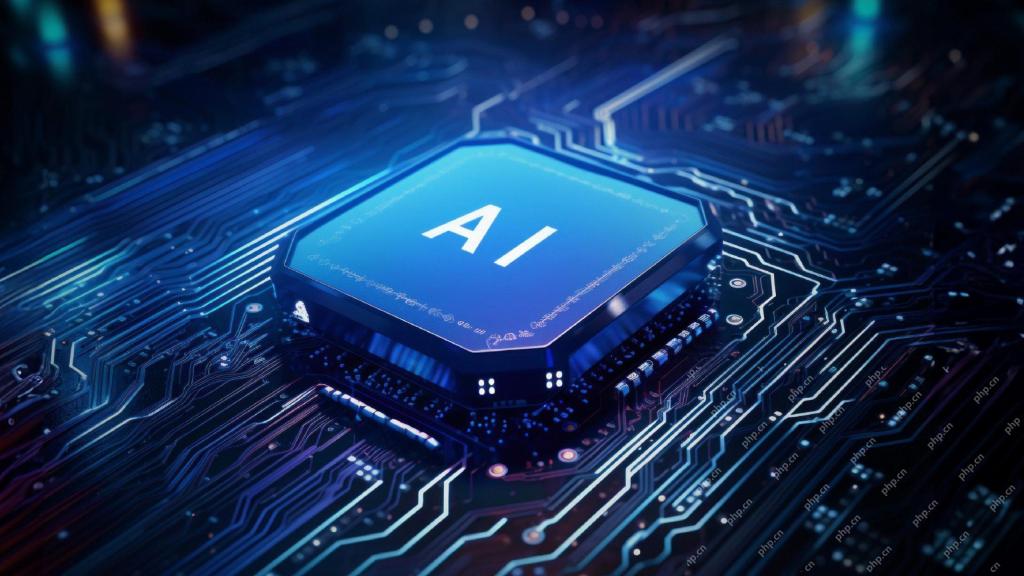
Local Search Algorithms: A Comprehensive Guide Planning a large-scale event requires efficient workload distribution. When traditional approaches fail, local search algorithms offer a powerful solution. This article explores hill climbing and simul
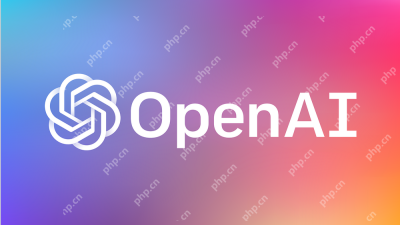
The release includes three distinct models, GPT-4.1, GPT-4.1 mini and GPT-4.1 nano, signaling a move toward task-specific optimizations within the large language model landscape. These models are not immediately replacing user-facing interfaces like
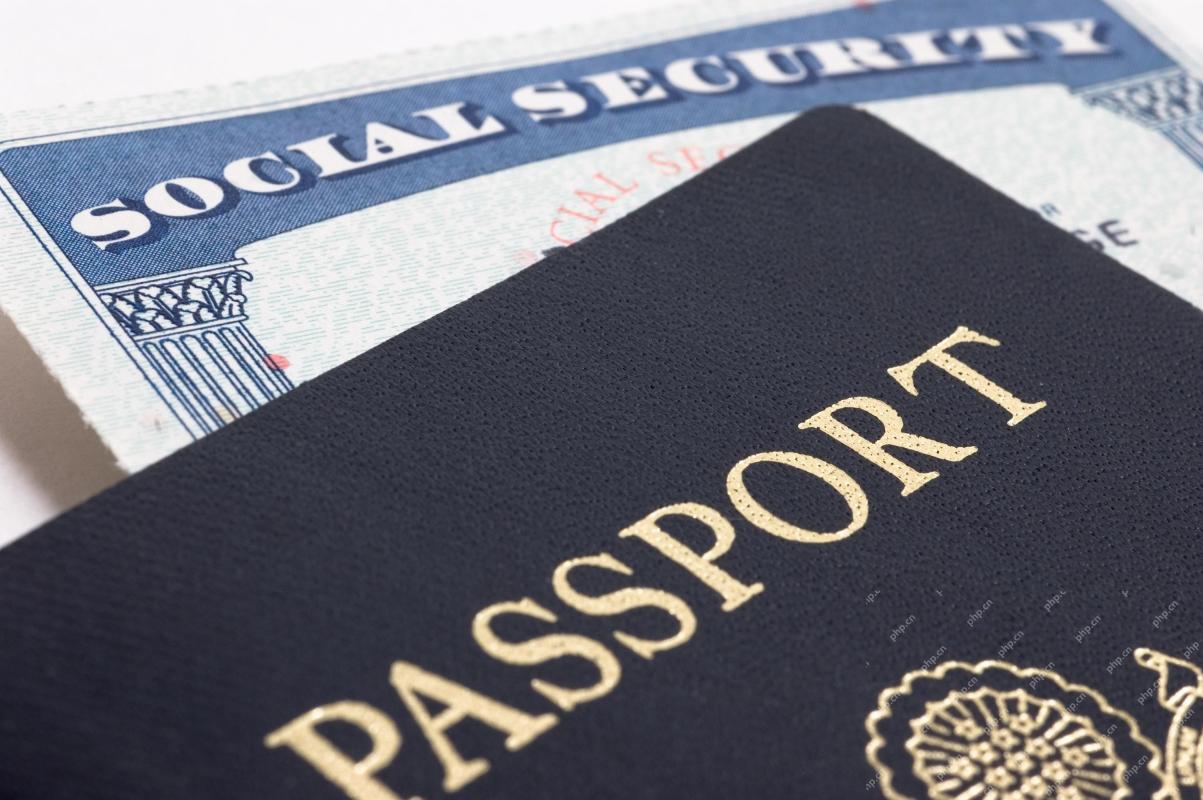
Chip giant Nvidia said on Monday it will start manufacturing AI supercomputers— machines that can process copious amounts of data and run complex algorithms— entirely within the U.S. for the first time. The announcement comes after President Trump si


Hot AI Tools

Undresser.AI Undress
AI-powered app for creating realistic nude photos

AI Clothes Remover
Online AI tool for removing clothes from photos.

Undress AI Tool
Undress images for free

Clothoff.io
AI clothes remover

AI Hentai Generator
Generate AI Hentai for free.

Hot Article

Hot Tools

Atom editor mac version download
The most popular open source editor

MinGW - Minimalist GNU for Windows
This project is in the process of being migrated to osdn.net/projects/mingw, you can continue to follow us there. MinGW: A native Windows port of the GNU Compiler Collection (GCC), freely distributable import libraries and header files for building native Windows applications; includes extensions to the MSVC runtime to support C99 functionality. All MinGW software can run on 64-bit Windows platforms.
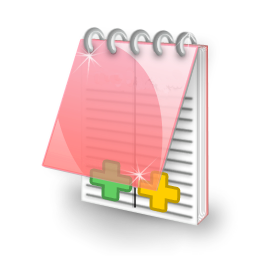
EditPlus Chinese cracked version
Small size, syntax highlighting, does not support code prompt function
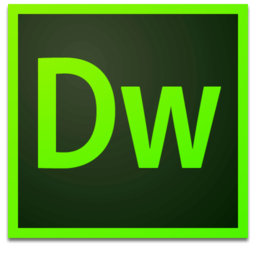
Dreamweaver Mac version
Visual web development tools

Notepad++7.3.1
Easy-to-use and free code editor