


I2V-Adapter from the SD community: no configuration required, plug and play, perfectly compatible with Tusheng video plug-in
The image-to-video generation (I2V) task is a challenge in the field of computer vision that aims to convert static images into dynamic videos. The difficulty of this task is to extract and generate dynamic information in the temporal dimension from a single image while maintaining the authenticity and visual coherence of the image content. Existing I2V methods often require complex model architectures and large amounts of training data to achieve this goal.
Recently, a new research result "I2V-Adapter: A General Image-to-Video Adapter for Video Diffusion Models" led by Kuaishou was released. This research introduces an innovative image-to-video conversion method and proposes a lightweight adapter module, the I2V-Adapter. This adapter module is capable of converting static images into dynamic videos without changing the original structure and pre-trained parameters of existing text-to-video generation (T2V) models. This method has broad application prospects in the field of image to video conversion, and can bring more possibilities to video creation, media communication and other fields. The release of the research results is of great significance for promoting the development of image and video technology, and provides an effective tool and method for researchers in related fields.
- Paper address: https://arxiv.org/pdf/2312.16693 .pdf
- Project homepage: https://i2v-adapter.github.io/index.html
- Code address: https://github.com/I2V-Adapter/I2V-Adapter-repo
Relative to existing methods In other words, I2V-Adapter has made huge improvements in terms of trainable parameters, with the minimum number of parameters reaching 22M, which is only 1% of the mainstream solution Stable Video Diffusion. At the same time, the adapter is also compatible with customized T2I models (such as DreamBooth, Lora) and control tools (such as ControlNet) developed by the Stable Diffusion community. Through experiments, the researchers proved the effectiveness of I2V-Adapter in generating high-quality video content, opening up new possibilities for creative applications in the I2V field.
Method introduction
Temporal modeling with Stable Diffusion
Compared to image generation, video generation faces a unique challenge of modeling temporal coherence between video frames. Most current methods are based on pre-trained T2I models, such as Stable Diffusion and SDXL, by introducing timing modules to model the timing information in videos. Inspired by AnimateDiff, a model originally designed for customized T2V tasks, it models timing information by introducing a timing module decoupled from the T2I model, and retains the ability of the original T2I model to generate smooth videos . Therefore, the researchers believe that the pre-trained temporal module can be regarded as a universal temporal representation and can be applied to other video generation scenarios, such as I2V generation, without any fine-tuning. Therefore, the researchers directly used the pre-trained AnimateDiff timing module and kept its parameters fixed.
Adapter for attention layers
Another challenge in the I2V task is to maintain the ID information of the input image. There are two main current solutions: one is to use a pre-trained image encoder to encode the input image, and inject the encoded features into the model through a cross-attention mechanism to guide the denoising process; the other is to The image is concatenated with the noisy input in the channel dimension and then fed together into the subsequent network. However, the former method may cause the generated video ID to change because it is difficult for the image encoder to capture the underlying information; while the latter method often requires changing the structure and parameters of the T2I model, resulting in high training costs and poor compatibility.
In order to solve the above problems, researchers proposed I2V-Adapter. Specifically, the researcher inputs the input image and noised input to the network in parallel. In the spatial block of the model, all frames will additionally query the first frame information, that is, the key and value features come from the first frame without noise, and the output The result is added to the self attention of the original model. The output mapping matrix in this module is initialized with zeros and only the output mapping matrix and query mapping matrix are trained. In order to further enhance the model's understanding of the semantic information of the input image, the researchers introduced a pre-trained content adapter (this article uses IP-Adapter [8]) to inject the semantic features of the image.
Frame Similarity Prior
In order to further enhance the stability of the generated results, the researcher proposed the frame Inter-similarity prior is used to strike a balance between the stability and motion intensity of the generated video. The key assumption is that at a relatively low Gaussian noise level, the noisy first frame and the noisy subsequent frames are close enough, as shown in the following figure:
Therefore, the researcher assumes that all frames have similar structures and become indistinguishable after adding a certain amount of Gaussian noise. Therefore, the noisy input image can be used as a priori input for subsequent frames. In order to eliminate the misleading of high-frequency information, the researchers also used Gaussian blur operator and random mask mixing. Specifically, the operation is given by:
Experimental results
Quantitative Results
This article calculated four quantitative indicators: DoverVQA (aesthetic score), CLIPTemp (first frame consistency), FlowScore (motion range) and WarppingError (motion error) Used to evaluate the quality of generated videos. Table 1 shows that I2V-Adapter received the highest aesthetic score and also exceeded all comparison schemes in terms of first frame consistency. In addition, the video generated by I2V-Adapter has the largest motion amplitude and relatively low motion error, indicating that this model is able to generate more dynamic videos while maintaining the accuracy of temporal motion.
Qualitative results
Image Animation (left is input, right is Output):
# #w/ Personalized T2Is (left is input, right is output):
Summary
This paper proposes I2V-Adapter, a plug-and-play lightweight module for image-to-video generation tasks. This method keeps the spatial block and motion block structures and parameters of the original T2V model fixed, inputs the first frame without noise and the subsequent frames with noise in parallel, and allows all frames to interact with the first frame without noise through the attention mechanism, thus Produce a video that is temporally coherent and consistent with the first frame. Researchers have demonstrated the effectiveness of this method on I2V tasks through quantitative and qualitative experiments. In addition, its decoupled design allows the solution to be directly combined with modules such as DreamBooth, Lora and ControlNet, proving the compatibility of the solution and promoting research on customized and controllable image-to-video generation.
The above is the detailed content of I2V-Adapter from the SD community: no configuration required, plug and play, perfectly compatible with Tusheng video plug-in. For more information, please follow other related articles on the PHP Chinese website!
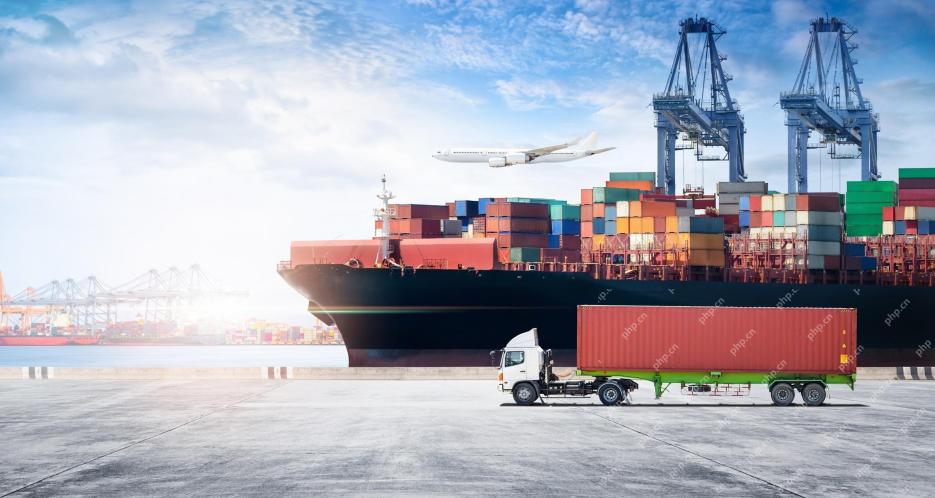
The term "AI-ready workforce" is frequently used, but what does it truly mean in the supply chain industry? According to Abe Eshkenazi, CEO of the Association for Supply Chain Management (ASCM), it signifies professionals capable of critic
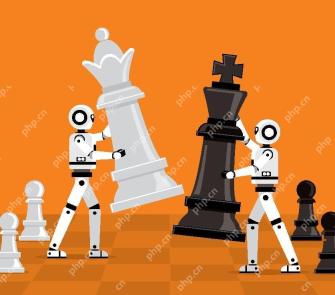
The decentralized AI revolution is quietly gaining momentum. This Friday in Austin, Texas, the Bittensor Endgame Summit marks a pivotal moment, transitioning decentralized AI (DeAI) from theory to practical application. Unlike the glitzy commercial
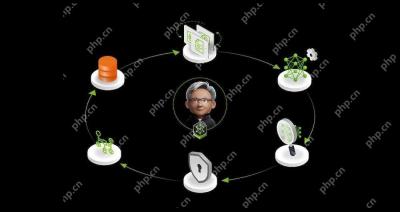
Enterprise AI faces data integration challenges The application of enterprise AI faces a major challenge: building systems that can maintain accuracy and practicality by continuously learning business data. NeMo microservices solve this problem by creating what Nvidia describes as "data flywheel", allowing AI systems to remain relevant through continuous exposure to enterprise information and user interaction. This newly launched toolkit contains five key microservices: NeMo Customizer handles fine-tuning of large language models with higher training throughput. NeMo Evaluator provides simplified evaluation of AI models for custom benchmarks. NeMo Guardrails implements security controls to maintain compliance and appropriateness
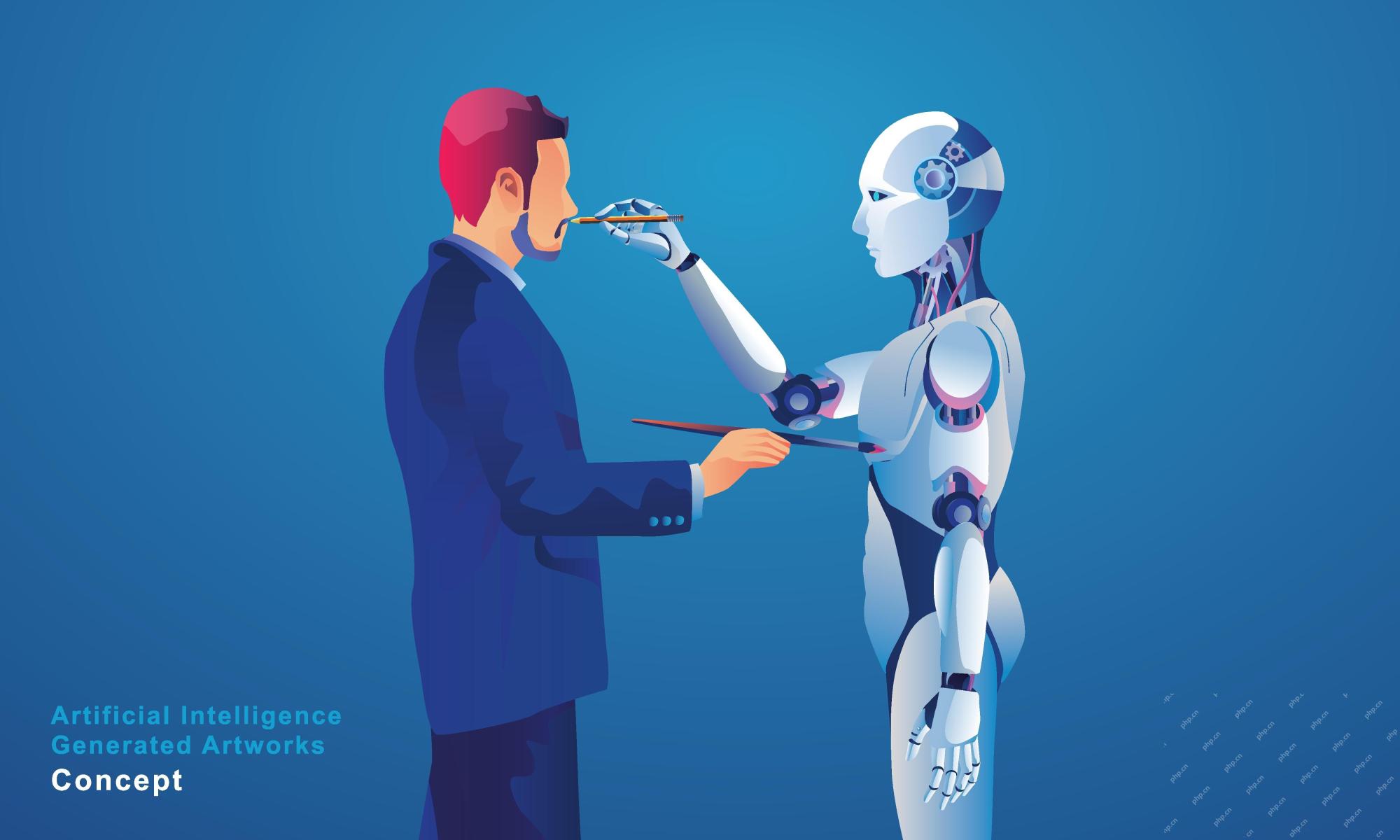
AI: The Future of Art and Design Artificial intelligence (AI) is changing the field of art and design in unprecedented ways, and its impact is no longer limited to amateurs, but more profoundly affecting professionals. Artwork and design schemes generated by AI are rapidly replacing traditional material images and designers in many transactional design activities such as advertising, social media image generation and web design. However, professional artists and designers also find the practical value of AI. They use AI as an auxiliary tool to explore new aesthetic possibilities, blend different styles, and create novel visual effects. AI helps artists and designers automate repetitive tasks, propose different design elements and provide creative input. AI supports style transfer, which is to apply a style of image
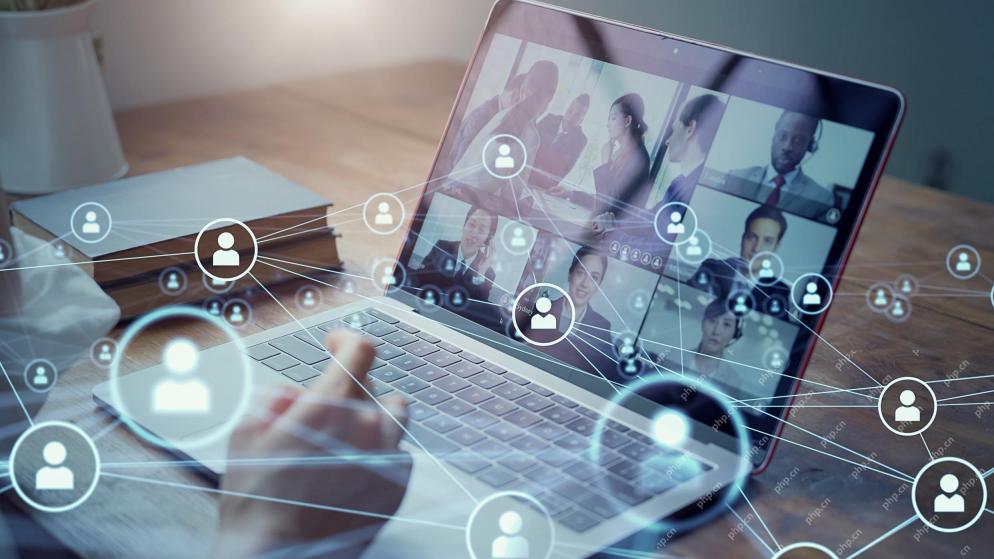
Zoom, initially known for its video conferencing platform, is leading a workplace revolution with its innovative use of agentic AI. A recent conversation with Zoom's CTO, XD Huang, revealed the company's ambitious vision. Defining Agentic AI Huang d
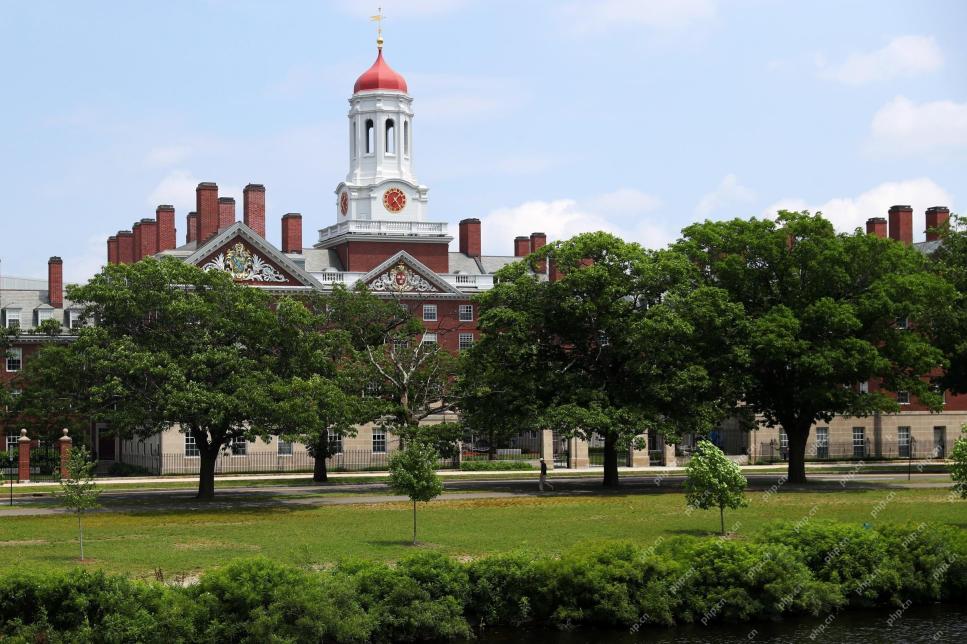
Will AI revolutionize education? This question is prompting serious reflection among educators and stakeholders. The integration of AI into education presents both opportunities and challenges. As Matthew Lynch of The Tech Edvocate notes, universit
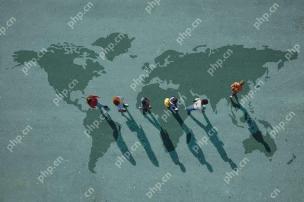
The development of scientific research and technology in the United States may face challenges, perhaps due to budget cuts. According to Nature, the number of American scientists applying for overseas jobs increased by 32% from January to March 2025 compared with the same period in 2024. A previous poll showed that 75% of the researchers surveyed were considering searching for jobs in Europe and Canada. Hundreds of NIH and NSF grants have been terminated in the past few months, with NIH’s new grants down by about $2.3 billion this year, a drop of nearly one-third. The leaked budget proposal shows that the Trump administration is considering sharply cutting budgets for scientific institutions, with a possible reduction of up to 50%. The turmoil in the field of basic research has also affected one of the major advantages of the United States: attracting overseas talents. 35
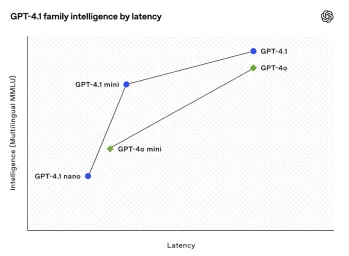
OpenAI unveils the powerful GPT-4.1 series: a family of three advanced language models designed for real-world applications. This significant leap forward offers faster response times, enhanced comprehension, and drastically reduced costs compared t


Hot AI Tools

Undresser.AI Undress
AI-powered app for creating realistic nude photos

AI Clothes Remover
Online AI tool for removing clothes from photos.

Undress AI Tool
Undress images for free

Clothoff.io
AI clothes remover

Video Face Swap
Swap faces in any video effortlessly with our completely free AI face swap tool!

Hot Article

Hot Tools

mPDF
mPDF is a PHP library that can generate PDF files from UTF-8 encoded HTML. The original author, Ian Back, wrote mPDF to output PDF files "on the fly" from his website and handle different languages. It is slower than original scripts like HTML2FPDF and produces larger files when using Unicode fonts, but supports CSS styles etc. and has a lot of enhancements. Supports almost all languages, including RTL (Arabic and Hebrew) and CJK (Chinese, Japanese and Korean). Supports nested block-level elements (such as P, DIV),

SublimeText3 Linux new version
SublimeText3 Linux latest version
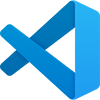
VSCode Windows 64-bit Download
A free and powerful IDE editor launched by Microsoft

SAP NetWeaver Server Adapter for Eclipse
Integrate Eclipse with SAP NetWeaver application server.

Safe Exam Browser
Safe Exam Browser is a secure browser environment for taking online exams securely. This software turns any computer into a secure workstation. It controls access to any utility and prevents students from using unauthorized resources.
