Creativity is a unique human ability that enables us to create novel, valuable, and meaningful works, such as art, literature, science, technology, etc. Creativity is also an important driving force in human society. It promotes development and progress in culture, economy, education and other fields. What exactly is creativity? How did it come about? How is it evaluated and improved? These questions have been perplexing researchers from multiple disciplines such as psychologists, cognitive scientists, and philosophers. With the rapid development of artificial intelligence (AI) technology, these issues have also attracted attention in the AI field. Can AI be as creative as humans? If so, how do you define, measure, and cultivate AI creativity? These issues not only have theoretical significance, but also have practical value, because the creativity of AI will affect the application and development of AI in various fields.
The science and technology academic forum arxiv.org published the latest paper "Can AI Be as Creative as Humans?" on January 3. The paper was published by researchers from the United States, the United Kingdom, Singapore and other countries. Co-written by many well-known AI researchers, this paper is an in-depth exploration of AI creativity. It proposes a novel creativity assessment paradigm, namely relative creativity (Relative Creativity), and establishes a feasible Quantitative creativity framework, namely statistical creativity (Statistical Creativity). The paper not only advances theoretical discussions but also provides practical tools and methods for evaluating and improving the creative potential of AI. The main contributions and innovations of this paper are as follows:
proposes the concept of relative creativity, which transforms the creativity evaluation of AI systems from an absolute standard to one related to a specific human creator. Comparison, thereby recognizing the inherent subjectivity in the creative process and drawing on the comparative method of assessing intelligence from the Turing Test. This innovative approach compares AI creativity to human creativity, allowing us to better understand the performance and capabilities of AI systems in the creative process. By incorporating subjectivity into the evaluation system, we are able to more fully assess the creativity of AI systems and compare them to human creators. , introduced the concept of statistical creativity, combining methods of theory building and empirical evaluation. This method focuses on whether AI can imitate the creative output of a specific group of people, thereby quantitatively assessing the creativity of AI and improving the practical applicability of the theoretical framework.
Applying statistical creativity to autoregressive models is a widely used language modeling technique. This technique is considered to be somewhat creative and has a practical metric for assessing the statistical creativity of a model. Particularly when it comes to next-mark prediction, this metric is adaptable to the needs of contemporary AI models and shows potential to keep pace with technological advancements.
The research introduces an objective function called Statistical Creativity Loss (Statistical Creativity Loss) for training creative AI models. The study also analyzes the upper bound of statistical creativity loss, and its related concepts to generalization in deep learning, such as Rademacher complexity, mutual information, and ε-coverage number. These theoretical guidance and practical measures provide important help in cultivating the creative ability of AI models.
01 The concept and definition of relative creativity
Creativity is an ability that is difficult to define and measure because it involves subjective , situational, multidimensional and dynamic factors. There may be different views and standards on what creativity is and what creative work is. Therefore, it is unrealistic and unfair to give an absolute definition of creativity or to give an absolute creativity assessment to AI systems. The author believes that the evaluation of creativity should be relative, not absolute, that is, the creativity of AI systems should be compared based on the levels and characteristics of different human creators. In this way, the assessment of creativity is no longer a static, fixed, and single standard, but a dynamic, flexible, and diverse process that can better reflect the nature and diversity of creativity.
The author proposed the concept of relative creativity (Relative Creativity), defining it as: an AI system is considered to be relatively creative if it can generate information based on the given biographical information of the human creator. A work that is indistinguishable from that creator's work, then the AI system can be considered as creative as that creator. The "relativity" of relative creativity is reflected in the fact that it depends on the differences between the individuals being compared. For example, an AI system might appear very creative when compared to a non-expert human creator, but less creative when compared to an expert designer or artist. Relative creativity also acknowledges the subjectivity of creativity, such as originality, divergent thinking, and problem-solving skills, which are incorporated into the selection process that anchors human creators. Since the assessment of creativity is based on a human perspective, the concept of relative creativity is also inspired by the Turing Test, a comparative method of assessing AI intelligence by judging whether an AI system can compete with humans. Have indistinguishable conversations.
Picture
Figure 1: Illustration of relative creativity and statistical creativity. In Figure 1, we explain relative creativity (a) and statistical creativity (b). Relative creativity refers to an assessment of an AI's ability to create art that is virtually indistinguishable from a hypothetical human creator, taking into account the same biographical influences. Statistical creativity, on the other hand, is assessed by measuring the ability of AI-generated creations to be indistinguishable from existing human creators, as determined by a distribution distance metric.
Relative creativity has some similarities with the Turing test, but there are also some differences. The similarity is that they are all based on a human perspective and evaluate the capabilities of the AI system by comparing the performance of the AI system with that of humans. The difference is that the goal of the Turing test is to evaluate the intelligence of an AI system, while the goal of relative creativity is to evaluate the creativity of an AI system. Intelligence and creativity are two different dimensions that may have some overlap but also some differences. For example, an AI system may be smart but not necessarily creative, and vice versa. Another difference is that the assessment of the Turing Test is conversation-based, while the assessment of relative creativity is based on work. Dialogue is an interactive, dynamic, real-time process, while work is a static, fixed, delayed result. Therefore, the assessment of relative creativity may be more difficult because it requires taking into account more factors, such as the style, content, quality, originality, etc. of the work. The author believes that relative creativity is a more comprehensive and in-depth method of assessing creativity, which takes into account not only the performance of the AI system, but also the background and characteristics of the human creator.
02 The concept and definition of statistical creativity
Although the concept of relative creativity is enlightening, it still lacks an operational assessment method. In order to make up for this shortcoming, the author introduces the concept of statistical creativity (Statistical Creativity), which is defined as: an AI system is considered to be statistically creative if it can generate, given a group of human creators, Output that is indistinguishable from the creative output of the group, then the AI system can be considered as creative as the group. The “statistical” nature of statistical creativity is reflected in the fact that it evaluates the creativity of AI systems based on observable data rather than abstract standards.
Picture
The author proposed a measure of statistical creativity, namely E0(q), which is an empirical measure , used to estimate the indistinguishability between an AI model and the creative capabilities of a group of human creators. The lower the value of E0(q), the better the AI model is able to imitate the creative ability of the group. The author also gives a theorem of statistical creativity, clarifying the conditions under which an AI model can be classified as having δ-creativity, that is, E0(q)
03 Measurement and Application of Statistical Creativity of Autoregressive Model
Autoregressive model is a common large-scale language model (LLMs) technology, which is based on the previous article probability distribution to predict the next token, thereby generating a coherent text sequence. Autoregressive models are considered to have a certain degree of creativity due to their ability to generate novel texts such as poems, stories, codes, etc. However, how to evaluate and improve the creativity of autoregressive models is still an open question. The authors apply the concept of statistical creativity to autoregressive models and propose a measure of statistical creativity for next token prediction, namely E1(q), which is an empirical measure used to estimate an autoregressive model and an Indistinguishability between the creative abilities of groups of human creators. The lower the value of E1(q), the better the autoregressive model is able to imitate the creative ability of the group.
Picture
The author also gave a theorem of the statistical creativity of the autoregressive model and clarified an autoregressive model A condition that can be classified as having δ-creativity is that E1(q)
Next token prediction is the core technology of autoregressive models, which determines the quality and diversity of text sequences generated by the model. The difficulty of next token prediction depends on the given context, and the likelihood of the target's token. If the context is clear and specific, then predicting the next token is easier because some tokens are more reasonable and common. For example, if the context is "I like to eat", then the next tag might be "apple", "bread", "dumplings", etc. However, if the context is vague and abstract, then the prediction of the next token is more difficult because many tokens are possible with no clear advantage. For example, if the context is "I want", then the next tag might be "travel", "study", "sleep", etc. In this case, the autoregressive model requires greater creativity to generate meaningful and interesting text sequences.
The importance of the next mark prediction is that it reflects the level of creativity of the autoregressive model and its similarity to the creativity of human creators. An autoregressive model exhibits the same creativity as a human creator if it can generate the next token indistinguishable from the work of a human creator in a different context. This assessment of creativity can be carried out through measures of statistical creativity, such as E1(q). The author believes that this evaluation method is a more objective and scientific method that does not rely on human subjective judgment but is based on the calculation of data and probability. The author's research provides a new perspective and method for the creativity of autoregressive models, helping to improve the generation ability and quality of the model.
04 Measurement and Application of Statistical Creativity of Prompt-Based Large Language Models
Prompt-conditioned large language models (LLMs) are A cutting-edge modeling paradigm that uses prompts to unlock the potential capabilities of models. Hints are a method of providing input and output formats to the model, which can be used to guide the model to complete different tasks, such as text classification, text generation, text summarization, etc. Prompts function similarly to human heuristics, stimulating creativity and flexibility in models. However, how to evaluate and enhance the creativity of prompt-based LLMs remains an open question. The authors apply the concept of statistical creativity to cue-based LLMs and propose a measure of statistical creativity for different contextual cues, namely E2(q), which is an empirical measure used to estimate a cue-based LLM. and the indistinguishability of the creative capabilities of a community of human creators. The lower the value of E2(q), the better the prompt-based LLM is able to imitate the creative ability of the group. The author also gives an inference on the statistical creativity of a prompt-based LLM, clarifying the conditions under which a prompt-based LLM can be classified as having δ-creativity, that is, E2(q)
05 Definition and optimization method of statistical creativity loss
Statistical Creativity Loss (Statistical Creativity Loss) is an optimizable objective function , used to train creative AI models. It is defined based on measures of statistical creativity, such as E0(q), E1(q) or E2(q). The lower the value of statistical creativity loss, the better the AI model is able to imitate the creative ability of a group of human creators. The authors propose an upper bound on statistical creativity loss, formula (2), and its concepts related to generalization in deep learning, such as Rademacher complexity, mutual information, and ε -Covering number (ε-covering number), etc. The authors analyze the upper bound on the loss of statistical creativity as a function of the log-likelihood of the next token prediction and the amount of creator-production data required to achieve statistical creativity. The authors' findings underscore the importance of diversity in creator-work pairs, rather than simply having large amounts of creation data. This insight makes the concept of statistical creativity particularly applicable in current AI frameworks based on next-mark prediction. The author's research not only provides a theoretical perspective, but also guides the discussion of AI creativity, advocates the use of relative evaluation to promote empirical research, and establishes a framework for evaluating and improving the creative ability of AI models.
06 Related work
The author finally reviews previous related work on the definition and application of creativity, mainly involving vision and language. field. The authors note that while there are many studies that attempt to apply elements of creativity in generative models, none directly define creativity or directly optimize it. Rather, the authors' research aims to build a theoretical foundation for creativity, a framework that naturally incorporates previous insights into the diversity and quality of generation. The authors expect their contributions to lay the foundation for future research and guide the improvement of model creativity.
In the field of vision, creative image generation models have made significant advances, raising questions about whether machines can produce creative art. Hertzmann (2018) explores this issue in depth, highlighting the intersection between computer graphics and artistic innovation. Xu et al. (2012a) proposed a creative three-dimensional modeling method that can generate diverse models according to user preferences. Generative adversarial networks (GANs) (Goodfellow et al., 2014) were used by Elgammal et al. (2017) to drive the creation of unique artistic styles by maximizing deviations from known styles. Sbai et al. (2018) further increase this bias, encouraging the model to be of a different style than the training set. Creative generation can also be viewed as a combinatorial process, with Ge et al. (2021) and Ranaweera (2016) emphasizing the importance of the integration of detailed elements. On this basis, Vinker et al. (2023) decomposed the concept of personalization into visual elements for innovative reorganization, enriching creative output.
In the field of language, the development of language models has also prompted researchers to explore how to optimize the use of data to improve the adaptability of models in different fields, tasks and languages (Gururangan et al., 2020; Devlin et al. al., 2019; Conneau et al., 2020). Researchers have also shown a tendency to use language models to interpret nuances in human communication (Schwartz et al., 2013; Wu et al., 2022). This insight has also been used to improve classification models (Hovy, 2015; Flek, 2020). With the increasing popularity of generative models, people are also interested in controllable text generation, that is, the output of the model must satisfy some constraints, such as politeness (Saha et al., 2022; Sennrich et al., 2016), emotion (Liu et al., 2016) al., 2021; Dathathri et al., 2019; He et al., 2020) or other style constraints. Finally, text style transfer (TST), which converts the style of input text into a set target style, has also become a popular task. Style can refer to a range of different text- and author-specific characteristics, such as politeness (Madaan et al., 2020), formality (Rao and Tetreault, 2018; Briakou et al., 2021), simplicity (Zhu et al., 2021) 2010; van den Bercken et al., 2019; Weng et al., 2019; Cao et al., 2020), author (Xu et al., 2012b; Carlson et al., 2018), author gender (Prabhumoye et al. , 2018) and so on (Jin et al., 2022). While all of these applications attempt to apply elements of creativity in generative models, none directly define creativity or directly optimize it. Instead, they focus on improving the model's performance on predefined tasks as a proxy for creativity. In contrast, the authors' research aims at establishing a theoretical foundation for creativity. This framework naturally incorporates previous insights into the diversity and quality of generation. The authors expect their contributions to lay the foundation for future research and guide the improvement of model creativity.
Reference: https://arxiv.org/abs/2401.01623
The above is the detailed content of Can artificial intelligence display creativity like humans?. For more information, please follow other related articles on the PHP Chinese website!
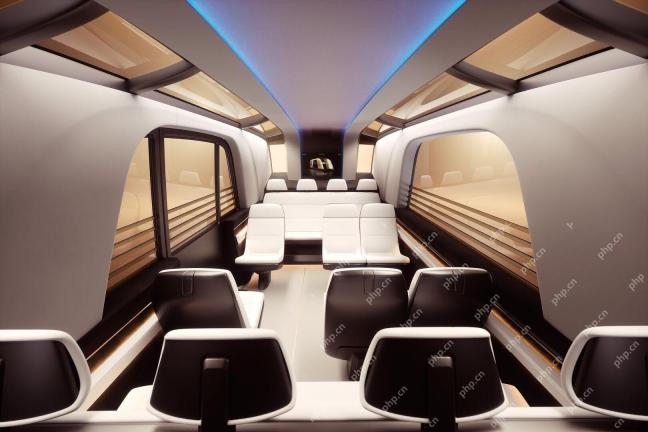
Since 2008, I've championed the shared-ride van—initially dubbed the "robotjitney," later the "vansit"—as the future of urban transportation. I foresee these vehicles as the 21st century's next-generation transit solution, surpas
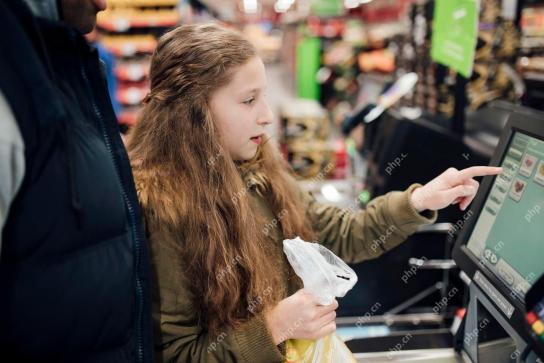
Revolutionizing the Checkout Experience Sam's Club's innovative "Just Go" system builds on its existing AI-powered "Scan & Go" technology, allowing members to scan purchases via the Sam's Club app during their shopping trip.
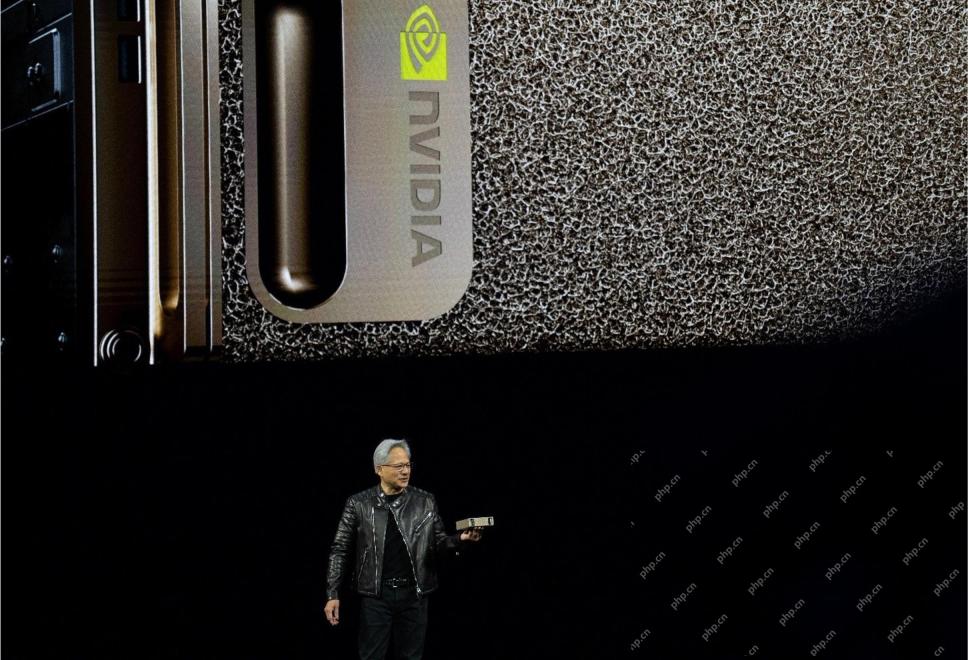
Nvidia's Enhanced Predictability and New Product Lineup at GTC 2025 Nvidia, a key player in AI infrastructure, is focusing on increased predictability for its clients. This involves consistent product delivery, meeting performance expectations, and
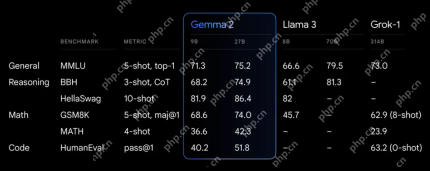
Google's Gemma 2: A Powerful, Efficient Language Model Google's Gemma family of language models, celebrated for efficiency and performance, has expanded with the arrival of Gemma 2. This latest release comprises two models: a 27-billion parameter ver
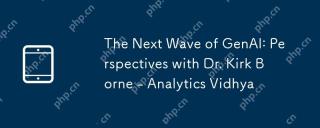
This Leading with Data episode features Dr. Kirk Borne, a leading data scientist, astrophysicist, and TEDx speaker. A renowned expert in big data, AI, and machine learning, Dr. Borne offers invaluable insights into the current state and future traje
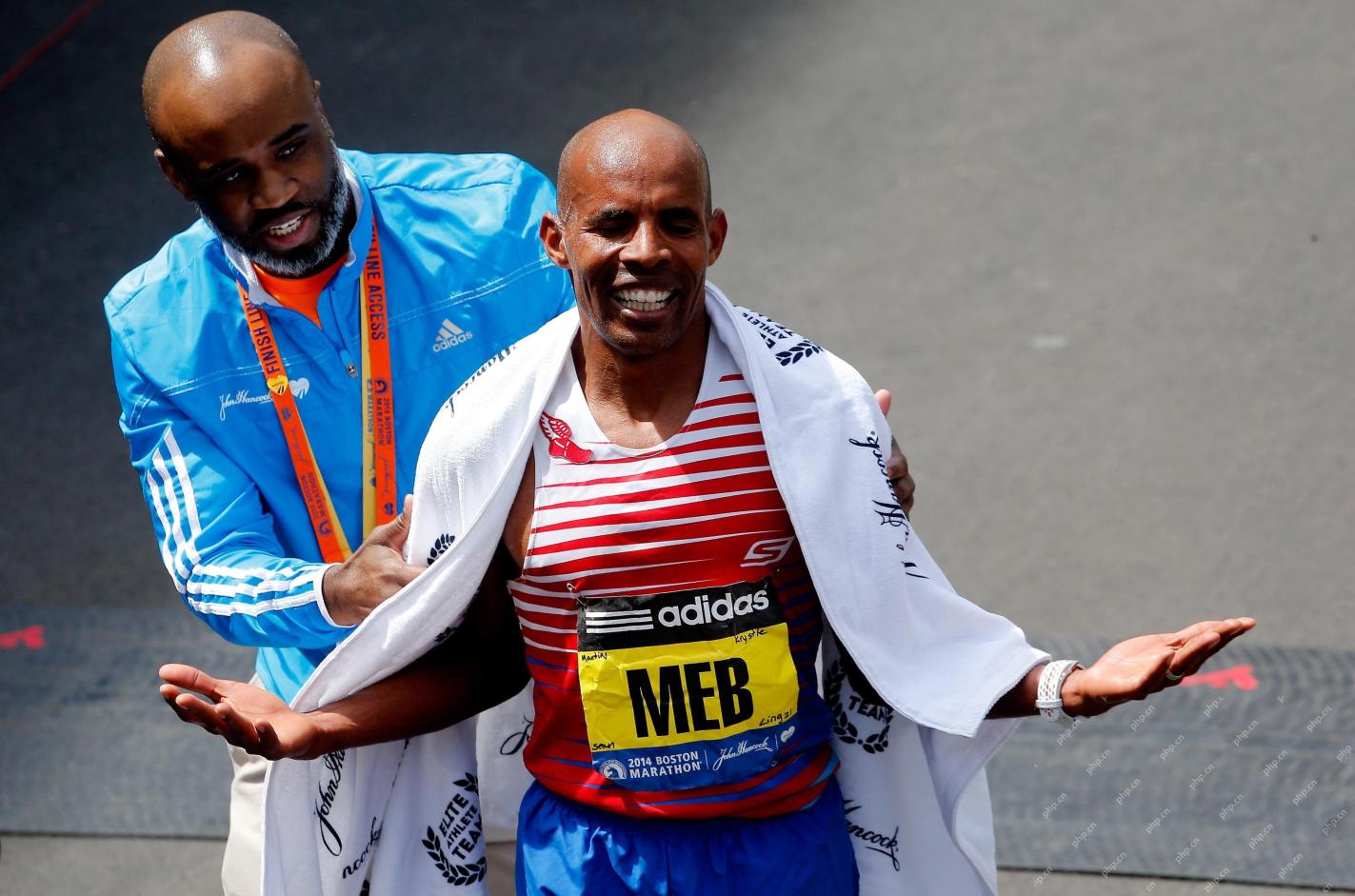
There were some very insightful perspectives in this speech—background information about engineering that showed us why artificial intelligence is so good at supporting people’s physical exercise. I will outline a core idea from each contributor’s perspective to demonstrate three design aspects that are an important part of our exploration of the application of artificial intelligence in sports. Edge devices and raw personal data This idea about artificial intelligence actually contains two components—one related to where we place large language models and the other is related to the differences between our human language and the language that our vital signs “express” when measured in real time. Alexander Amini knows a lot about running and tennis, but he still
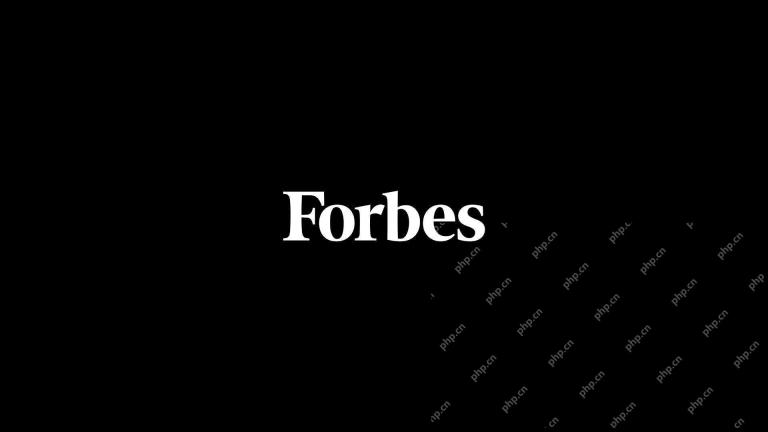
Caterpillar's Chief Information Officer and Senior Vice President of IT, Jamie Engstrom, leads a global team of over 2,200 IT professionals across 28 countries. With 26 years at Caterpillar, including four and a half years in her current role, Engst
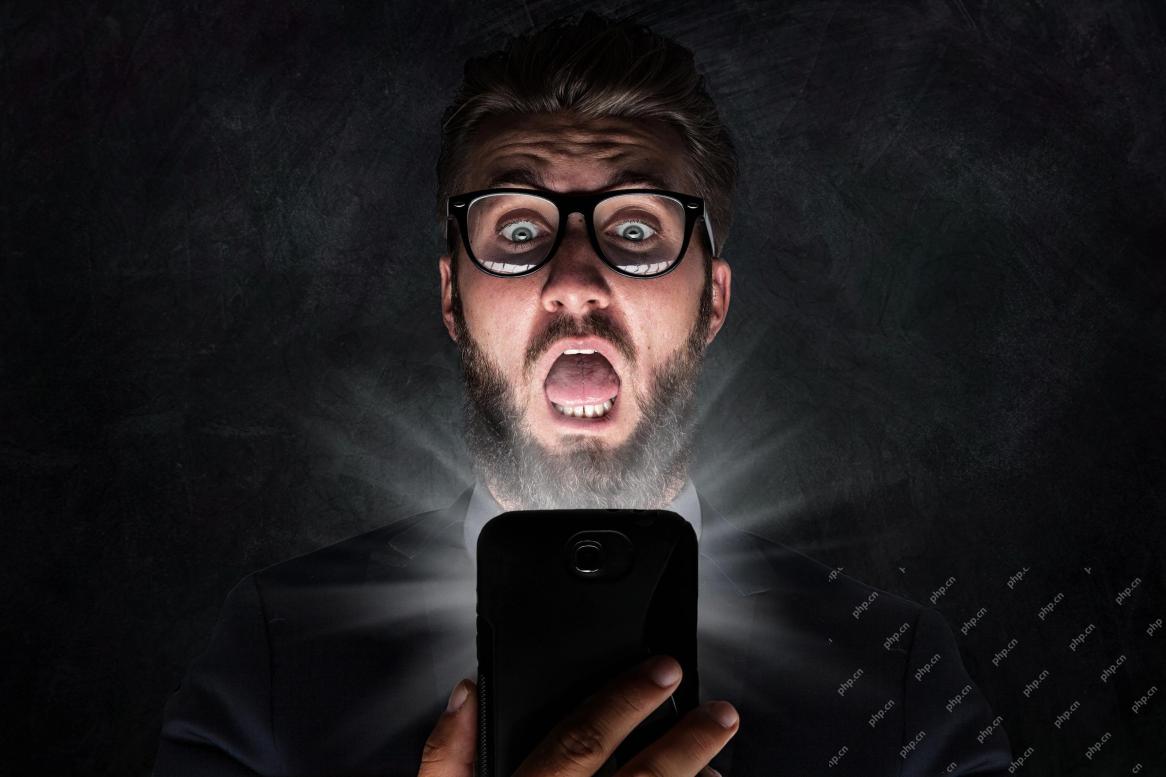
Google Photos' New Ultra HDR Tool: A Quick Guide Enhance your photos with Google Photos' new Ultra HDR tool, transforming standard images into vibrant, high-dynamic-range masterpieces. Ideal for social media, this tool boosts the impact of any photo,


Hot AI Tools

Undresser.AI Undress
AI-powered app for creating realistic nude photos

AI Clothes Remover
Online AI tool for removing clothes from photos.

Undress AI Tool
Undress images for free

Clothoff.io
AI clothes remover

Video Face Swap
Swap faces in any video effortlessly with our completely free AI face swap tool!

Hot Article

Hot Tools

Atom editor mac version download
The most popular open source editor

SublimeText3 English version
Recommended: Win version, supports code prompts!

mPDF
mPDF is a PHP library that can generate PDF files from UTF-8 encoded HTML. The original author, Ian Back, wrote mPDF to output PDF files "on the fly" from his website and handle different languages. It is slower than original scripts like HTML2FPDF and produces larger files when using Unicode fonts, but supports CSS styles etc. and has a lot of enhancements. Supports almost all languages, including RTL (Arabic and Hebrew) and CJK (Chinese, Japanese and Korean). Supports nested block-level elements (such as P, DIV),

DVWA
Damn Vulnerable Web App (DVWA) is a PHP/MySQL web application that is very vulnerable. Its main goals are to be an aid for security professionals to test their skills and tools in a legal environment, to help web developers better understand the process of securing web applications, and to help teachers/students teach/learn in a classroom environment Web application security. The goal of DVWA is to practice some of the most common web vulnerabilities through a simple and straightforward interface, with varying degrees of difficulty. Please note that this software

MinGW - Minimalist GNU for Windows
This project is in the process of being migrated to osdn.net/projects/mingw, you can continue to follow us there. MinGW: A native Windows port of the GNU Compiler Collection (GCC), freely distributable import libraries and header files for building native Windows applications; includes extensions to the MSVC runtime to support C99 functionality. All MinGW software can run on 64-bit Windows platforms.