


A new result recently discovered by Google DeepMind has caused widespread controversy in the Transformer field:
Its generalization ability cannot be extended to content beyond the training data.
This conclusion has not yet been further verified, but it has alarmed many big names. For example, Francois Chollet, the father of Keras, said that if the news is true, it will become a big news. A big deal in the modeling world.
Google Transformer is the infrastructure behind today's large models, and the "T" in GPT that we are familiar with refers to it.
A series of large models show strong contextual learning capabilities and can quickly learn examples and complete new tasks.
But now, researchers also from Google seem to have pointed out its fatal flaw - it is powerless beyond the training data, that is, human beings' existing knowledge.
For a time, many practitioners believed that AGI had become out of reach again.
Some netizens pointed out that there are some key details that have been overlooked in the paper. For example, the experiment only involves the scale of GPT-2, and the training data is not rich enough
As time goes by, more netizens who have carefully studied this paper pointed out that there is nothing wrong with the research conclusion itself, but people have made excessive interpretations based on it.
After the paper triggered heated discussions among netizens, one of the authors also publicly made two clarifications:
First of all, the experiment used a simple Transformer is neither a "big" model nor a language model;
Secondly, the model can learn new tasks, but it cannot be generalized tonew types Task
After that, another netizen repeated this experiment in Colab, but got completely different results.
So, let’s first take a look at this paper and what Samuel, who proposed different results, said.
The new function is almost unpredictable
In this experiment, the author used a Jax-based machine learning framework to train a Transformer model close to the size of GPT-2, which only contains the decoder part
This model contains 12 layers, 8 attention heads, the embedding space dimension is 256, and the number of parameters is about 9.5 million
In order to test its generalization ability, the author chose functions as test objects . They input linear functions and sine functions into the model as training data.
These two functions are known to the model at this time, and the prediction results are naturally very good. However, when the researchers put the linear function and Problems arise when convex combinations of sinusoidal functions are performed.
Convexity combination is not that mysterious. The author constructed a function of the form f(x)=a·kx (1-a)sin(x). In our opinion, it is just two functions according to Proportions simply add up.
The reason why we think this is because our brains have this generalization ability, but large-scale models are different
For models that have only learned linear and sine functions, simple The addition looks novel
For this new function, Transformer’s predictions have almost no accuracy (see Figure 4c), so the author believes that the model lacks generalization ability on the function
In order to further verify his conclusion, the author adjusted the weight of the linear or sinusoidal function, but even so the prediction performance of Transformer did not change significantly.
There is only one exception - when the weight of one of the items is close to 1, the model's prediction results are more consistent with the actual situation.
If the weight is 1, it means that the unfamiliar new function directly becomes the function that has been seen during training. This kind of data is obviously not helpful to the generalization ability of the model
Further experiments also showed that Transformer is not only very sensitive to the type of function, but even the same type of function may become unfamiliar conditions.
The researchers found that when changing the frequency of the sine function, even a simple function model, the prediction results will appear to change
Only when the frequency is close to the function in the training data, the model Only when the frequency is too high or too low can a more accurate prediction be given. When the frequency is too high or too low, the prediction results will have serious deviations...
Accordingly, the author believes that as long as the conditions are slightly It's a little different. I don't know how to do it with a large model. Doesn't this mean that the generalization ability is poor?
The author also describes some limitations in the research and how to apply observations on functional data to tokenized natural language problems.
The team also tried similar experiments on language models but encountered some obstacles. How to properly define task families (equivalent to the types of functions here), convex combinations, etc. have yet to be solved.
However, Samuel’s model is small in scale, with only 4 layers. It can be applied to the combination of linear and sine functions after 5 minutes of training on Colab
So what if it cannot be generalized
Based on the comprehensive content of the entire article, Quora CEO’s conclusion in this article is very narrow and can only be established if many assumptions are true
Sloan Prize winner and UCLA professor Gu Quanquan said that the conclusion of the paper itself is not controversial, but it should not be over-interpreted.
According to previous research, the Transformer model cannot generalize only when faced with content that is significantly different from the pre-training data. In fact, the generalization ability of large models is usually evaluated by the diversity and complexity of tasks
If you carefully investigate the generalization ability of Transformer, I am afraid it will take a bullet. Fly for a while longer.
But, even if we really lack generalization ability, what can we do?
NVIDIA AI scientist Jim Fan said that this phenomenon is actually not surprising, because Transformer is not a panacea. The large model performs well because the training data is just right. It's the content we care about.
Jim further added, This is like saying, use 100 billion photos of cats and dogs to train a visual model, and then let the model identify aircraft, and then find, Wow, I really don’t know him.
#When humans face some unknown tasks, not only large-scale models may not be able to find solutions. Does this also imply that humans lack generalization ability?
Therefore, in a goal-oriented process, whether it is a large model or a human, the ultimate goal is to solve the problem, and generalization is only a means
Change this expression into Chinese. Since the generalization ability is insufficient, then train it until there is no data other than the training sample
So, what do you think of this research?
Paper address: https://arxiv.org/abs/2311.00871
The above is the detailed content of Google's large model research has triggered fierce controversy: the generalization ability beyond the training data has been questioned, and netizens said that the AGI singularity may be delayed.. For more information, please follow other related articles on the PHP Chinese website!

Introduction Suppose there is a farmer who daily observes the progress of crops in several weeks. He looks at the growth rates and begins to ponder about how much more taller his plants could grow in another few weeks. From th
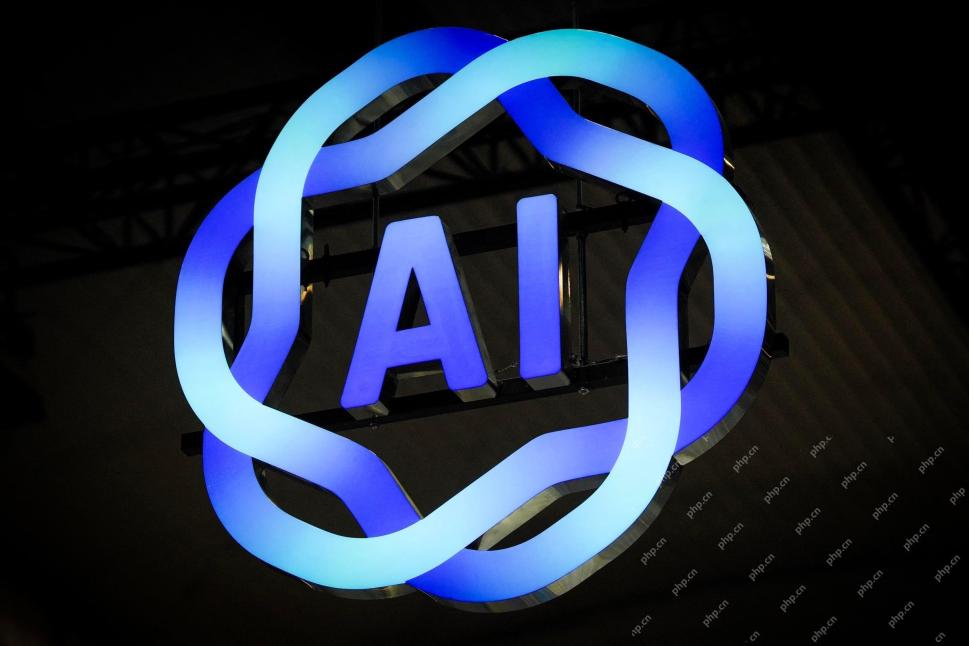
Soft AI — defined as AI systems designed to perform specific, narrow tasks using approximate reasoning, pattern recognition, and flexible decision-making — seeks to mimic human-like thinking by embracing ambiguity. But what does this mean for busine
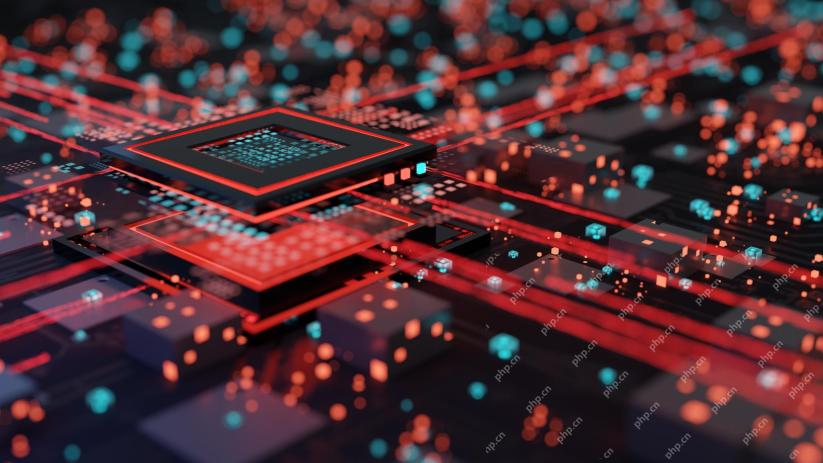
The answer is clear—just as cloud computing required a shift toward cloud-native security tools, AI demands a new breed of security solutions designed specifically for AI's unique needs. The Rise of Cloud Computing and Security Lessons Learned In th

Entrepreneurs and using AI and Generative AI to make their businesses better. At the same time, it is important to remember generative AI, like all technologies, is an amplifier – making the good great and the mediocre, worse. A rigorous 2024 study o
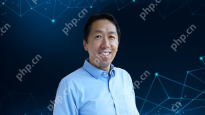
Unlock the Power of Embedding Models: A Deep Dive into Andrew Ng's New Course Imagine a future where machines understand and respond to your questions with perfect accuracy. This isn't science fiction; thanks to advancements in AI, it's becoming a r
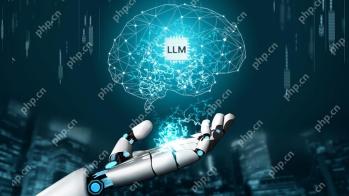
Large Language Models (LLMs) and the Inevitable Problem of Hallucinations You've likely used AI models like ChatGPT, Claude, and Gemini. These are all examples of Large Language Models (LLMs), powerful AI systems trained on massive text datasets to
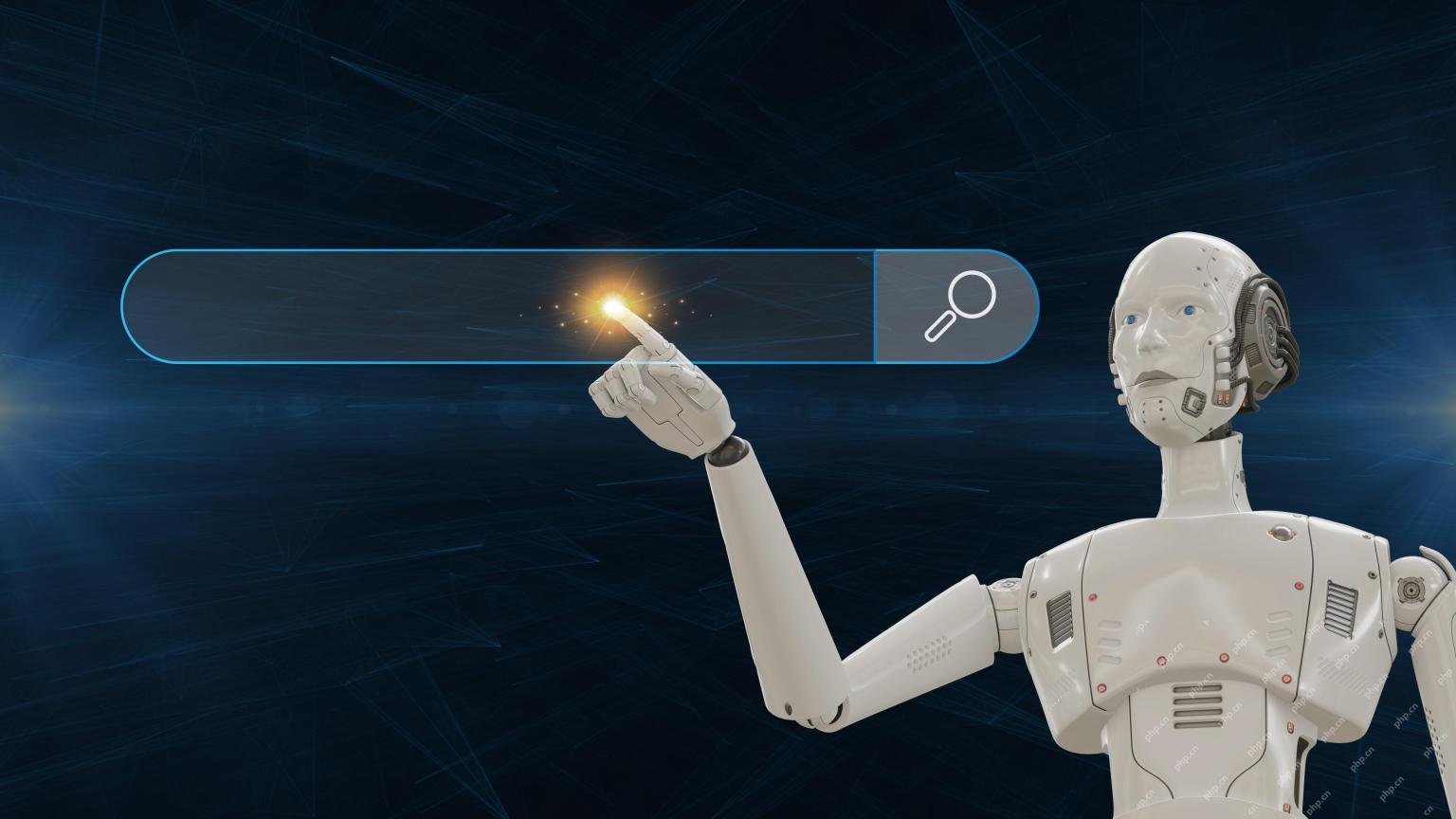
Recent research has shown that AI Overviews can cause a whopping 15-64% decline in organic traffic, based on industry and search type. This radical change is causing marketers to reconsider their whole strategy regarding digital visibility. The New
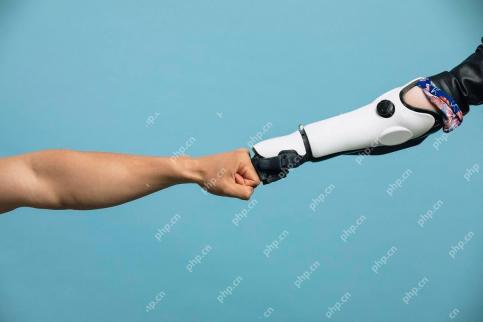
A recent report from Elon University’s Imagining The Digital Future Center surveyed nearly 300 global technology experts. The resulting report, ‘Being Human in 2035’, concluded that most are concerned that the deepening adoption of AI systems over t


Hot AI Tools

Undresser.AI Undress
AI-powered app for creating realistic nude photos

AI Clothes Remover
Online AI tool for removing clothes from photos.

Undress AI Tool
Undress images for free

Clothoff.io
AI clothes remover

AI Hentai Generator
Generate AI Hentai for free.

Hot Article

Hot Tools

SublimeText3 Chinese version
Chinese version, very easy to use

MantisBT
Mantis is an easy-to-deploy web-based defect tracking tool designed to aid in product defect tracking. It requires PHP, MySQL and a web server. Check out our demo and hosting services.

PhpStorm Mac version
The latest (2018.2.1) professional PHP integrated development tool
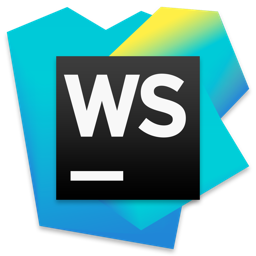
WebStorm Mac version
Useful JavaScript development tools
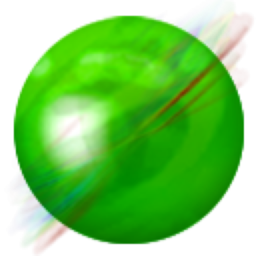
ZendStudio 13.5.1 Mac
Powerful PHP integrated development environment