The answer is clear—just as cloud computing required a shift toward cloud-native security tools, AI demands a new breed of security solutions designed specifically for AI's unique needs.
The Rise of Cloud Computing and Security Lessons Learned
In the early days of cloud computing, businesses attempted to secure cloud environments using traditional on-premises security tools. This approach didn’t take into account the cloud’s distinct characteristics—shared responsibility models, massive scale, and the difficulty of securing data distributed across various locations. This led to a realization: effective cloud security couldn’t be achieved by simply applying old methods; it required new, cloud-native tools.
The shift to cloud-native security solutions was not just about scaling existing systems—it was about rethinking security to match the cloud’s architecture. These tools needed to be elastic, cloud-aware, and able to monitor and protect dynamic, distributed environments in real time. The cloud became the catalyst for a more sophisticated approach to security, and this same evolution is now required for AI.
The AI Security Challenge
AI systems differ fundamentally from traditional software applications. They can learn, adapt, and evolve in real time, creating a new set of risks. From generative AI tools like ChatGPT to more advanced agentic AI systems, the attack surface grows with each new model that’s introduced. Security tools designed for static systems simply cannot keep pace with the rapid changes in AI systems.
This challenge isn’t theoretical—it’s already here. As Moinul Khan, co-founder and CEO of Aurascape, pointed out during a recent conversation, “Organizations are focused on keeping bad actors out and protecting intellectual property—AI adds a layer of complexity to that.”
The core challenge is maintaining control over what AI systems are doing and ensuring that sensitive data doesn’t leave the organization in the process.
Why Traditional Security Tools Won’t Suffice
AI technologies constantly evolve, which is part of their value—but also part of their risk. Traditional security tools, which are designed for static environments, simply don’t have the capability to monitor AI systems’ behaviors effectively. These tools are unable to track how AI models learn, interact with data, or adapt to new inputs.
As Khan explained, traditional network security tools like firewalls and proxies are inadequate when it comes to AI applications. “When I post a file to Microsoft Copilot and ask for a summary, that’s an HTTP POST. But if I interact with Copilot through a series of back-and-forth queries, your existing firewalls and proxies are blind. They can’t see or understand the interactions.” This is where AI-native security tools come into play.
AI-native security solutions must be able to monitor AI-specific activities, providing visibility into data flows and AI outputs while offering granular control. These tools need to be designed with AI’s behavior in mind—understanding how AI models work, what data they process, and how their outputs are used.
The Rise of AI-Native Security Tools
In response to these unique challenges, AI-native security tools are emerging. These tools offer several key capabilities:
- Real-Time Threat Detection: AI-native security solutions must be able to detect anomalous behaviors in real time as AI systems process data, learn from new inputs, and generate outputs.
- Granular Control Over AI Systems: Just as cloud-native security tools provide granular control over cloud environments, AI-native security tools give businesses control over AI applications. This includes monitoring and controlling the data that AI systems access and ensuring their outputs align with security policies.
- Data Protection Across AI Systems: With AI processing vast amounts of data, security tools must ensure that sensitive information is not exposed or misused. AI-native solutions need to protect data as it moves through AI systems, ensuring compliance with regulations like GDPR and HIPAA.
Evolution of AI Security
The evolution of security for emerging technologies typically follows a pattern and offers a roadmap for securing AI.
Khan drew a direct parallel between the early days of cloud security and the current state of AI security: “We are not creating a new market; we are solving the same problem that organizations faced 20 years ago with the internet. The only difference is that now we are dealing with AI applications that need to be understood in an entirely different way. It’s not just about blocking bad actors, it’s about understanding the specific use cases, interactions, and data flows of AI systems.”
This shift to AI-native security is already happening. Aurascape recently emerged from stealth after a year of operations to position itself as a player in this movement. The company launched with $50M in funding from prominent investors like Mayfield Fund and Menlo Ventures, alongside strategic backers such as former Palo Alto Networks CEO Mark McLaughlin and former Zscaler Chief Strategy Officer Manoj Apte.
“We capture the entire query and response, giving you insight into what your users are doing with AI in real time,” Khan said. This capability allows businesses to monitor and secure data flows across a wide range of AI applications, providing both visibility and protection in a way that traditional security models cannot.
As the demand for AI solutions grows, the need for AI-native security tools has never been more urgent. Aurascape’s market entry, backed by its strong investor network and its AI Activity Control platform, is a step toward helping organizations meet this challenge.
Building a Secure Foundation for AI Adoption
As AI technologies become more integrated into business operations, securing these systems is paramount. The lessons learned from cloud security demonstrate that when new technologies emerge, security frameworks must evolve to meet those challenges. The shift to AI-native security tools is not just inevitable—it is essential for businesses that want to fully harness the power of AI without exposing themselves to unnecessary risks.
Aurascape’s approach, which utilizes AI to fight AI, exemplifies this evolution. By providing organizations with the ability to monitor and control AI applications in real time, businesses can confidently adopt AI technologies while protecting their most valuable assets—intellectual property and sensitive data.
To take advantage of the AI revolution while avoiding unnecessary risk, organizations must adopt AI-native security solutions that are built specifically to handle the unique demands of AI systems. Just as cloud-native security tools were necessary for securing the cloud, AI-native security tools will be critical in ensuring that AI can be adopted safely and securely across industries.
The future of AI is filled with potential—but only if we can secure it properly.
The above is the detailed content of Evolving Security Frameworks For The AI Frontier. For more information, please follow other related articles on the PHP Chinese website!
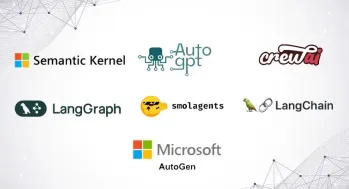
This article explores seven leading frameworks for building AI agents – autonomous software entities that perceive, decide, and act to achieve goals. These agents, surpassing traditional reinforcement learning, leverage advanced planning and reasoni
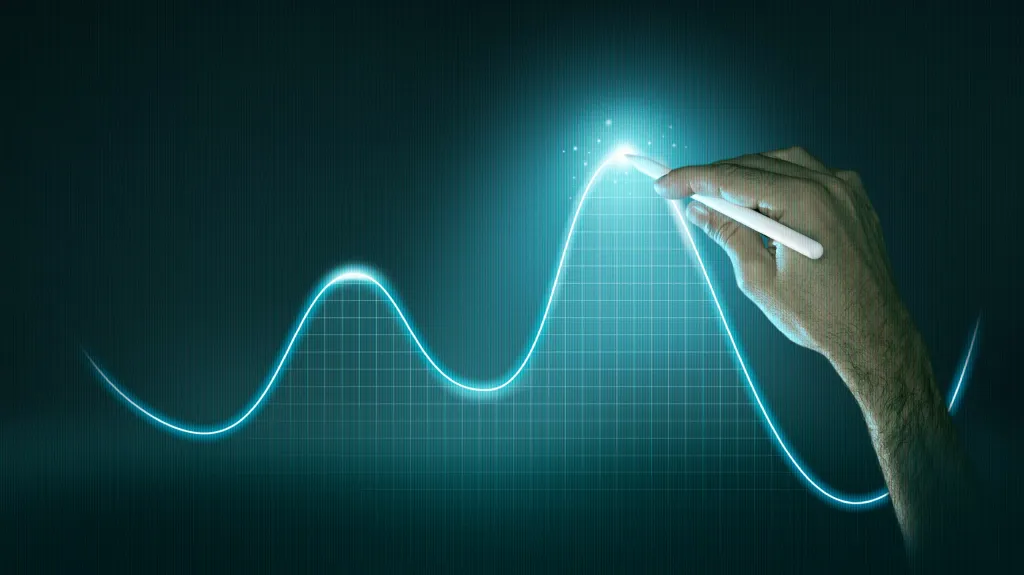
Understanding Type I and Type II Errors in Statistical Hypothesis Testing Imagine a clinical trial testing a new blood pressure medication. The trial concludes the drug significantly lowers blood pressure, but in reality, it doesn't. This is a Type

Sumy: Your AI-Powered Summarization Assistant Tired of sifting through endless documents? Sumy, a powerful Python library, offers a streamlined solution for automatic text summarization. This article explores Sumy's capabilities, guiding you throug
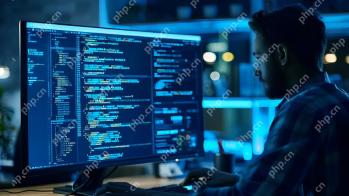
Data Challenges: Mastering SQL's CASE Statement for Accurate Insights Who needs lawyers when you've got data enthusiasts? Data analysts, scientists, and everyone in the vast data world face their own complex challenges, ensuring systems function fla
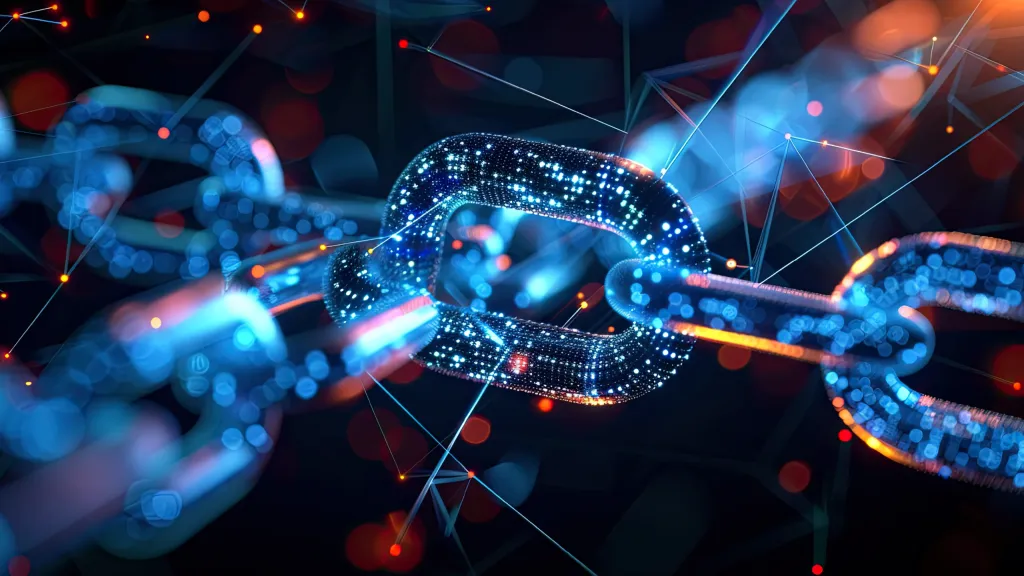
Harnessing the Power of Knowledge Chains in AI: A Deep Dive into Prompt Engineering Do you know that Artificial Intelligence (AI) can not only understand your questions but also weave together vast amounts of knowledge to deliver insightful answers?
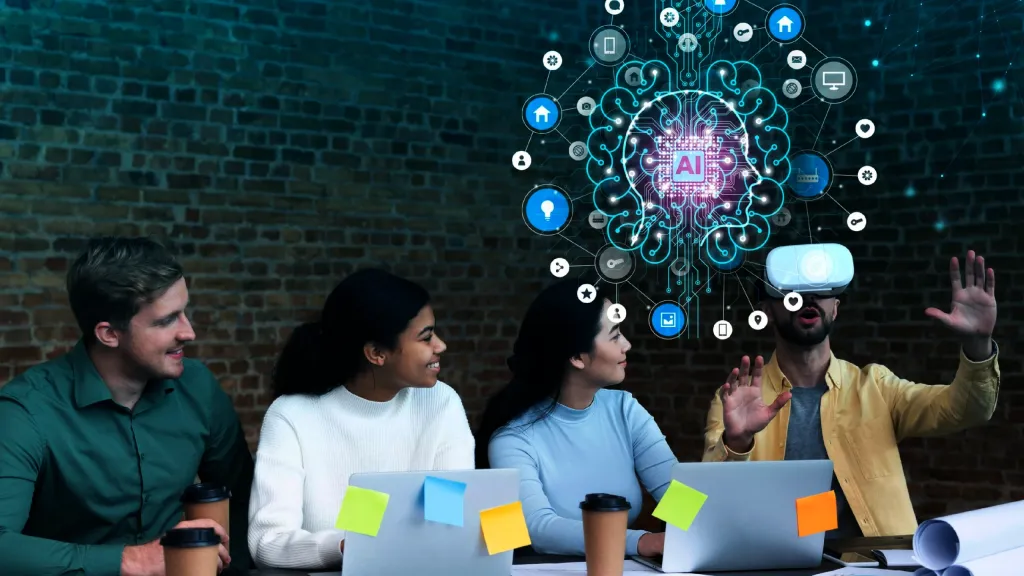
Introduction Joanna Maciejewska recently shared a insightful observation on X: The biggest challenge with the AI push? It's misdirected. I want AI to handle laundry and dishes so I can focus on art and writing, not the other way around. — Joanna Ma
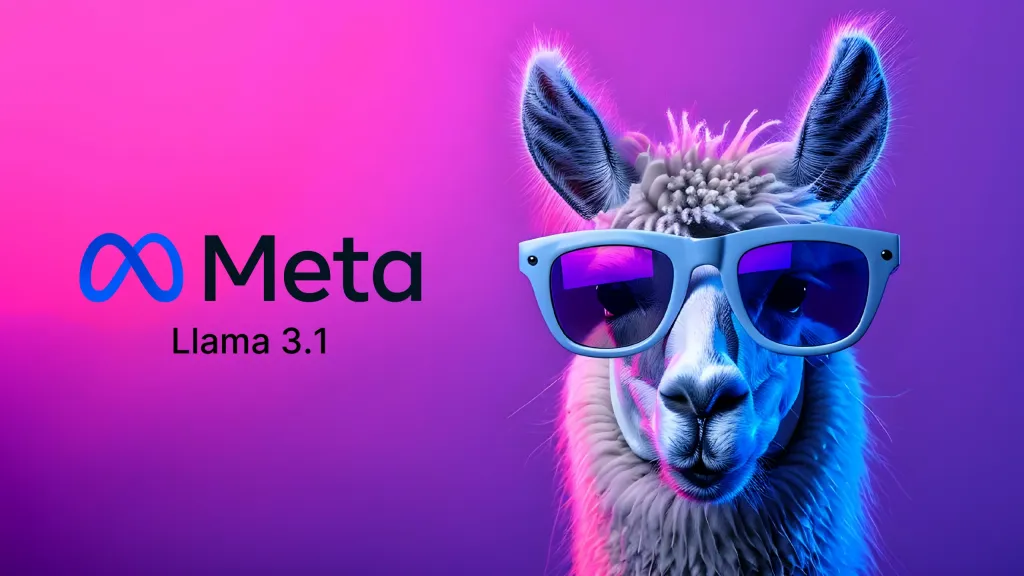
Meta's Llama 3.1: A Deep Dive into Open-Source LLM Capabilities Meta continues to lead the charge in open-source Large Language Models (LLMs). The Llama family, evolving from Llama to Llama 2, Llama 3, and now Llama 3.1, demonstrates a commitment to
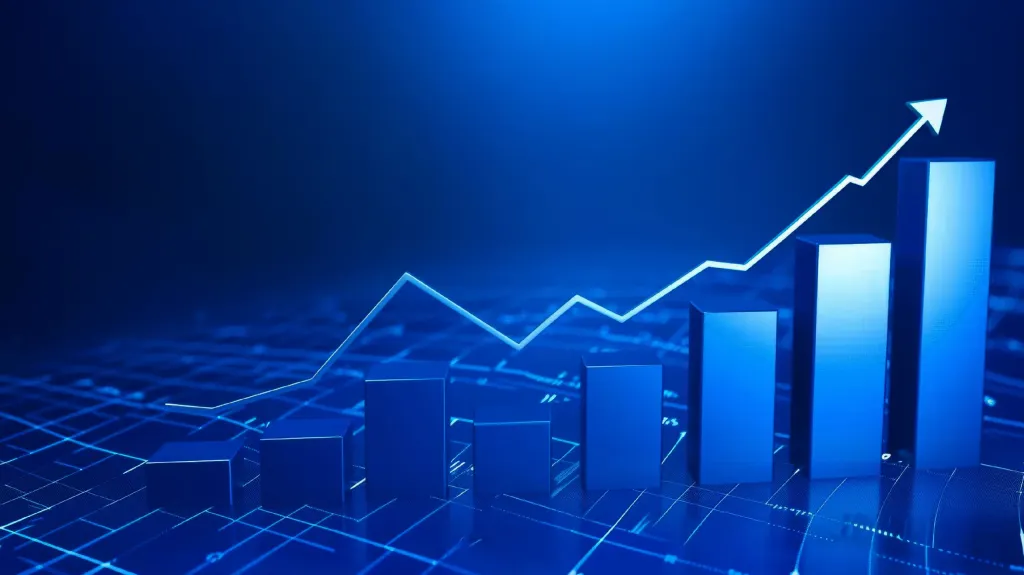
Introduction Statistical Process Control (SPC) charts are essential tools in quality management, enabling organizations to monitor, control, and improve their processes. By applying statistical methods, SPC charts visually represent data variations


Hot AI Tools

Undresser.AI Undress
AI-powered app for creating realistic nude photos

AI Clothes Remover
Online AI tool for removing clothes from photos.

Undress AI Tool
Undress images for free

Clothoff.io
AI clothes remover

AI Hentai Generator
Generate AI Hentai for free.

Hot Article

Hot Tools

Notepad++7.3.1
Easy-to-use and free code editor

Zend Studio 13.0.1
Powerful PHP integrated development environment

SecLists
SecLists is the ultimate security tester's companion. It is a collection of various types of lists that are frequently used during security assessments, all in one place. SecLists helps make security testing more efficient and productive by conveniently providing all the lists a security tester might need. List types include usernames, passwords, URLs, fuzzing payloads, sensitive data patterns, web shells, and more. The tester can simply pull this repository onto a new test machine and he will have access to every type of list he needs.
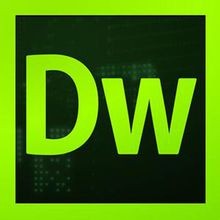
Dreamweaver CS6
Visual web development tools
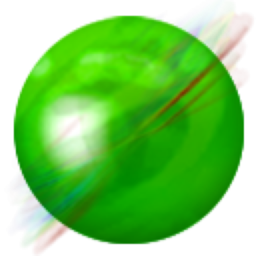
ZendStudio 13.5.1 Mac
Powerful PHP integrated development environment