


Recently, various applications of large models are still popular. Around the beginning of October, a series of rather gimmicky articles appeared, trying to apply large models to autonomous driving. I have been talking about a lot of related topics with many friends recently. When writing this article, on the one hand, I discovered that including myself, we have actually confused some very related but actually different concepts in the past. On the other hand, it is an extension of these concepts. There are some interesting thoughts that are worth sharing and discussing with everyone.
Large (Language) Model
This is undoubtedly the most popular direction at present, and it is also the focus of the most concentrated papers. How can large language models help autonomous driving? On the one hand, like GPT-4V, it provides extremely powerful semantic understanding capabilities through alignment with images, which will not be mentioned here for the time being; on the other hand, it uses LLM as an agent to directly implement driving behavior. The latter is actually the most sexy research direction at present, and is inextricably linked to the series of work on embedded AI.
Most of the latter type of work seen so far uses LLM: 1) directly used 2) fine-tuned through supervised learning 3) fine-tuned through reinforcement learning for driving tasks. In essence, there is no escape from the previous paradigm framework of driving based on learning methods. In fact, a very direct question is, why might it be better to use LLM to do this? Intuitively speaking, using words to drive is an inefficient and verbose thing. Then one day I suddenly figured out that LLM actually implements a pretrain for the agent through language! One of the important reasons why RL was difficult to generalize before was that it was difficult to unify various tasks and use various common data to pretrain. Each task could only be trained from scratch, but LLM is very good. Solved this problem. But in fact, there are several problems that are not well solved: 1) After completing pretrain, must the language be retained as the output interface? This actually brings a lot of inconvenience to many tasks, and also causes redundant calculations to a certain extent. 2) The approach of LLM as agent still does not overcome the essential problems of the existing RL model free method, and all the problems of model free methods still exist. Recently, we have also seen some attempts at model based LLM as agent, which may be an interesting direction.
The last thing I want to complain about in each paper is: It’s not just connecting to LLM and letting LLM output a reason to make your model interpretable. This reason may still be nonsense. . . Things that were not guaranteed before will not become guaranteed just because a sentence is output.
Large (Visual) Model
In fact, the purely large visual model still has not seen that magical "emergence" moment. When talking about large visual models, there are generally two possible references: one is a super visual information feature extractor based on massive web data pre-training such as CLIP or DINO or SAM, which greatly improves the semantic understanding ability of the model; The other refers to the joint model of pairs (image, action, etc...) implemented by the world model represented by GAIA.
In fact, I think the former is just the result of continuing linear scale up along the traditional thinking. It is difficult to see the possibility of changing the amount of autonomous driving at present. In fact, the latter has continuously entered the field of vision of researchers due to the continuous publicity of Wayve and Tesla this year. When people talk about world models, they often include the fact that the model is end-to-end (directly outputs actions) and is related to LLM. In fact, this assumption is one-sided. My understanding of the world model is also very limited. I would like to recommend Lecun’s interview and @Yu Yang’s model based RL survey, which I will not expand on:
Yu Yang: About the environment model (world model) learning
https://www.php.cn/link/a2cdd86a458242d42a17c2bf4feff069
Pure visual autonomous driving
This is actually It is easy to understand that it refers to an autonomous driving system that relies only on visual sensors. This is actually the best and ultimate wish of autonomous driving: to drive with a pair of eyes like a human being. Such concepts are generally associated with the above two large models, because the complex semantics of images require strong abstraction capabilities to extract useful information. Under Tesla's recent continuous publicity offensive, this concept also overlaps with the end-to-end mentioned below. But in fact, there are many ways to achieve pure visual driving, and end-to-end is naturally one of them, but it is not the only one. The most difficult problem in realizing purely visual autonomous driving is that vision is inherently insensitive to 3D information, and large models have not essentially changed this. Specifically reflected in: 1) The way of passively receiving electromagnetic waves makes vision unlike other sensors that can measure geometric information in 3D space; 2) Perspective makes distant objects extremely sensitive to errors. This is very unfriendly for downstream planning and control, which are implemented in an equal-error 3D space by default. However, is driving by vision the same as being able to accurately estimate 3D distance and speed? I think this is a representation issue worthy of in-depth study in pure visual autonomous driving in addition to semantic understanding.
End-to-end automatic driving
This concept refers to the control signal from the sensor to the final output (in fact, I think it can also be broadly included to the waypoints of the upstream layer planning information) using a jointly optimized model. This can either be a direct end-to-end method that inputs sensor data like ALVINN as early as the 1980s and outputs control signals directly through a neural network, or it can be a staged end-to-end method like this year's CVPR best paper UniAD. However, a common point of these methods is that the downstream supervision signal can be directly passed to the upstream, instead of each module having its own self-defined optimization goals. Overall, this is a correct idea. After all, deep learning relies on such joint optimization to make its fortune. However, for systems such as autonomous driving or general-purpose robots, which are often extremely complex and deal with the physical world, there are many problems that need to be overcome in terms of engineering implementation and data organization and utilization efficiency.
Feed-Forward End-to-End Autonomous Driving
This concept seems to be rarely mentioned, but in fact I find that the existence of end-to-end itself is valuable. But the problem lies in the way of using Feed-Forward to observe. Including me, in fact, I have always defaulted that end-to-end driving must be in the form of Feed-Forward, because 99% of current deep learning-based methods assume such a structure, which means that the final output of concern (such as control signals )u = f(x), x is the various observations of the sensor. Here f can be a very complex function. But in fact, in some problems, we hope to make the final output satisfy or be close to certain properties, so it is difficult for the Feed-Forward form to give such a guarantee. So there is another way we can write u* = argmin g(u, x) s.t. h(u, x)
With the development of large models, this direct Feed-Forward end-to-end autonomous driving solution has ushered in a wave of revival. Of course, large models are very powerful, but I raise a question and hope everyone will think about it: If the large model is omnipotent end-to-end, does that mean that the large model should be able to play Go/Gobang end-to-end? Woolen cloth? Paradigms like AlphaGo should be meaningless? I believe everyone knows that the answer is no. Of course, this Feed-Forward method can be used as a fast approximate solver and achieve good results in most scenarios.
Judging from the current plans of various companies that have disclosed their use of Neural Planner, the neural part only provides a number of initialization proposals for subsequent optimization plans to alleviate the problem of highly non-convexity in subsequent optimization. This is essentially the same thing as fast rollout in AlphaGo. But AlphaGo will not call the subsequent MCTS search a "cover-up" solution. . . Finally, I hope this can help everyone clarify the differences and connections between these concepts, and that everyone can clearly understand what they are talking about when discussing issues. . .The above is the detailed content of Let's talk about several large models and autonomous driving concepts that have become popular recently.. For more information, please follow other related articles on the PHP Chinese website!
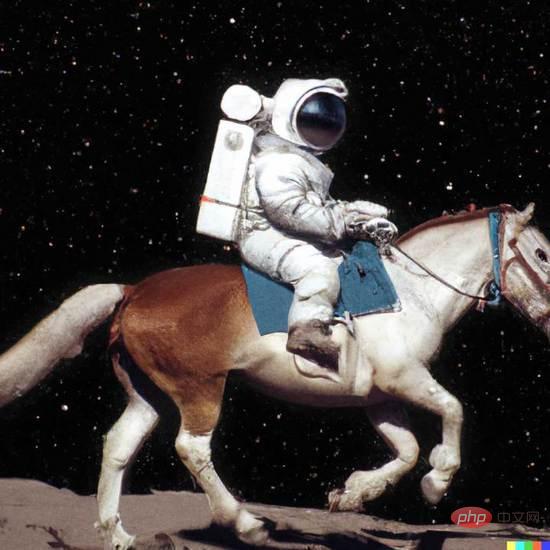
1 前言在发布DALL·E的15个月后,OpenAI在今年春天带了续作DALL·E 2,以其更加惊艳的效果和丰富的可玩性迅速占领了各大AI社区的头条。近年来,随着生成对抗网络(GAN)、变分自编码器(VAE)、扩散模型(Diffusion models)的出现,深度学习已向世人展现其强大的图像生成能力;加上GPT-3、BERT等NLP模型的成功,人类正逐步打破文本和图像的信息界限。在DALL·E 2中,只需输入简单的文本(prompt),它就可以生成多张1024*1024的高清图像。这些图像甚至
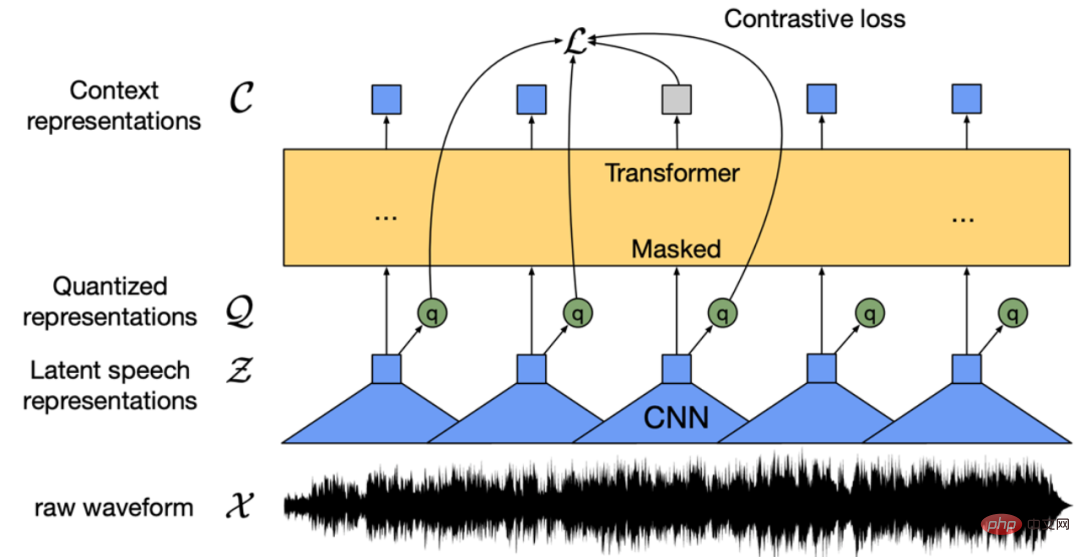
Wav2vec 2.0 [1],HuBERT [2] 和 WavLM [3] 等语音预训练模型,通过在多达上万小时的无标注语音数据(如 Libri-light )上的自监督学习,显著提升了自动语音识别(Automatic Speech Recognition, ASR),语音合成(Text-to-speech, TTS)和语音转换(Voice Conversation,VC)等语音下游任务的性能。然而这些模型都没有公开的中文版本,不便于应用在中文语音研究场景。 WenetSpeech [4] 是
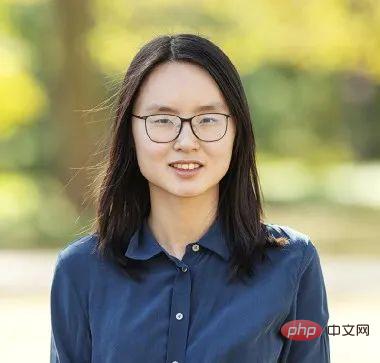
“Making large models smaller”这是很多语言模型研究人员的学术追求,针对大模型昂贵的环境和训练成本,陈丹琦在智源大会青源学术年会上做了题为“Making large models smaller”的特邀报告。报告中重点提及了基于记忆增强的TRIME算法和基于粗细粒度联合剪枝和逐层蒸馏的CofiPruning算法。前者能够在不改变模型结构的基础上兼顾语言模型困惑度和检索速度方面的优势;而后者可以在保证下游任务准确度的同时实现更快的处理速度,具有更小的模型结构。陈丹琦 普
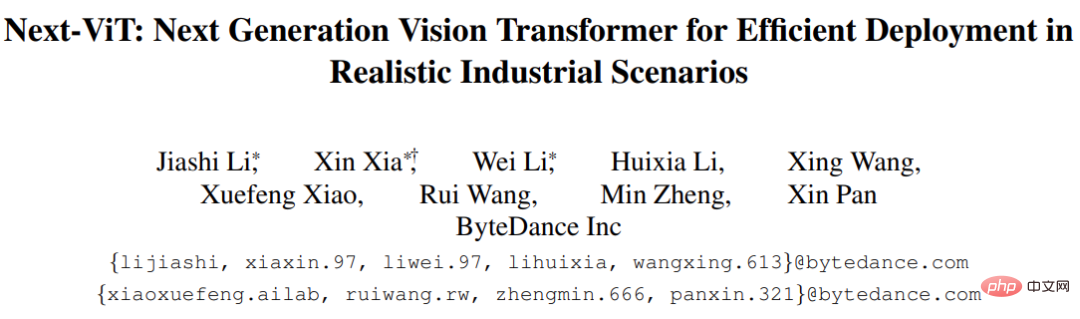
由于复杂的注意力机制和模型设计,大多数现有的视觉 Transformer(ViT)在现实的工业部署场景中不能像卷积神经网络(CNN)那样高效地执行。这就带来了一个问题:视觉神经网络能否像 CNN 一样快速推断并像 ViT 一样强大?近期一些工作试图设计 CNN-Transformer 混合架构来解决这个问题,但这些工作的整体性能远不能令人满意。基于此,来自字节跳动的研究者提出了一种能在现实工业场景中有效部署的下一代视觉 Transformer——Next-ViT。从延迟 / 准确性权衡的角度看,
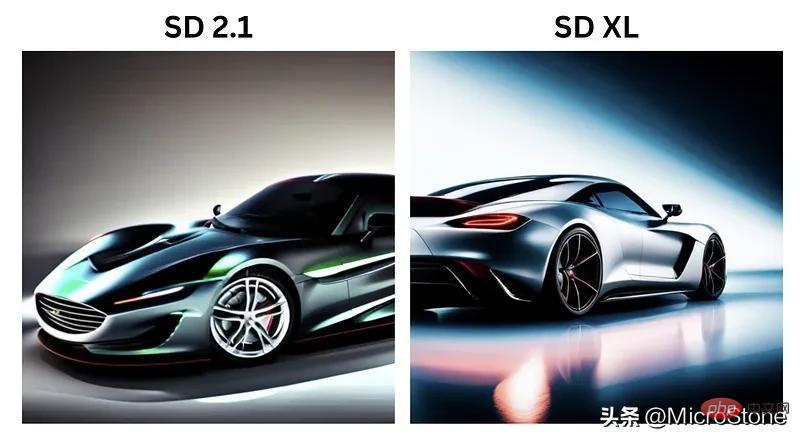
3月27号,Stability AI的创始人兼首席执行官Emad Mostaque在一条推文中宣布,Stable Diffusion XL 现已可用于公开测试。以下是一些事项:“XL”不是这个新的AI模型的官方名称。一旦发布稳定性AI公司的官方公告,名称将会更改。与先前版本相比,图像质量有所提高与先前版本相比,图像生成速度大大加快。示例图像让我们看看新旧AI模型在结果上的差异。Prompt: Luxury sports car with aerodynamic curves, shot in a
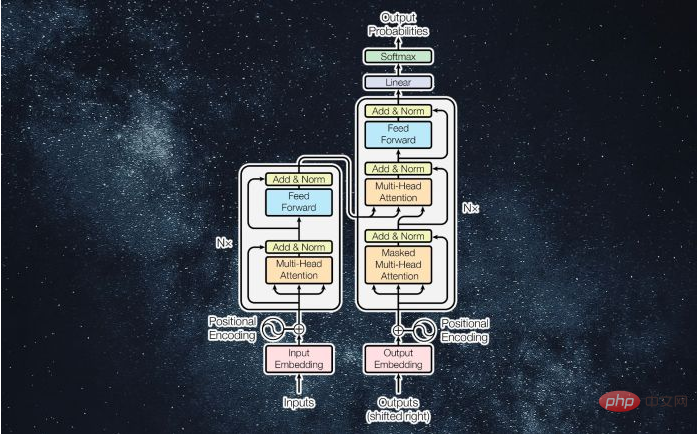
译者 | 李睿审校 | 孙淑娟近年来, Transformer 机器学习模型已经成为深度学习和深度神经网络技术进步的主要亮点之一。它主要用于自然语言处理中的高级应用。谷歌正在使用它来增强其搜索引擎结果。OpenAI 使用 Transformer 创建了著名的 GPT-2和 GPT-3模型。自从2017年首次亮相以来,Transformer 架构不断发展并扩展到多种不同的变体,从语言任务扩展到其他领域。它们已被用于时间序列预测。它们是 DeepMind 的蛋白质结构预测模型 AlphaFold
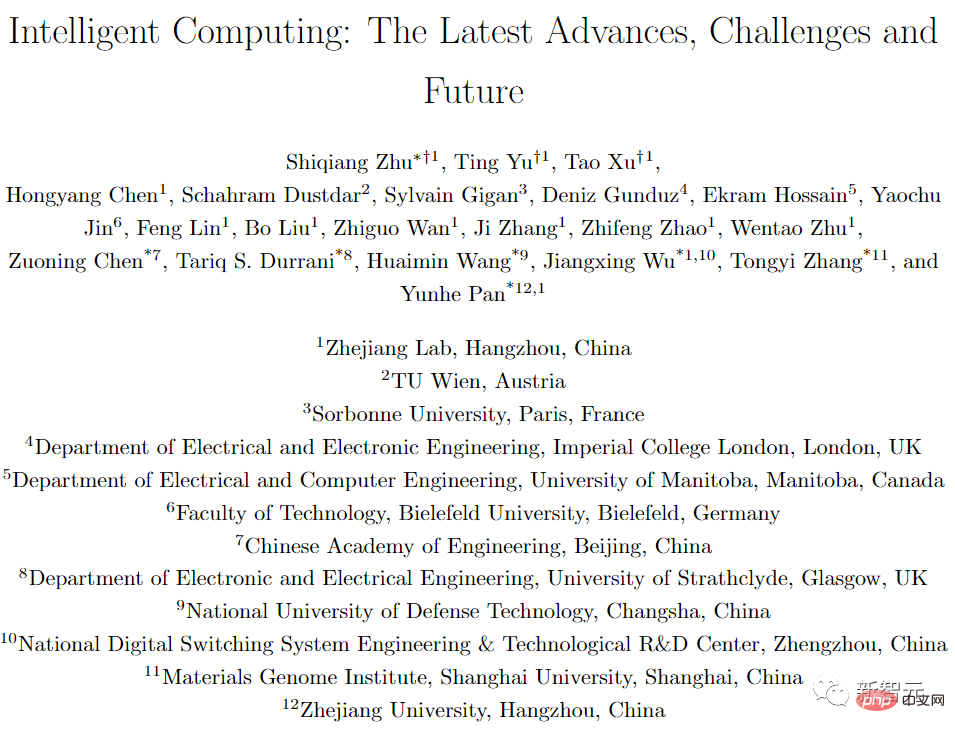
人工智能就是一个「拼财力」的行业,如果没有高性能计算设备,别说开发基础模型,就连微调模型都做不到。但如果只靠拼硬件,单靠当前计算性能的发展速度,迟早有一天无法满足日益膨胀的需求,所以还需要配套的软件来协调统筹计算能力,这时候就需要用到「智能计算」技术。最近,来自之江实验室、中国工程院、国防科技大学、浙江大学等多达十二个国内外研究机构共同发表了一篇论文,首次对智能计算领域进行了全面的调研,涵盖了理论基础、智能与计算的技术融合、重要应用、挑战和未来前景。论文链接:https://spj.scien
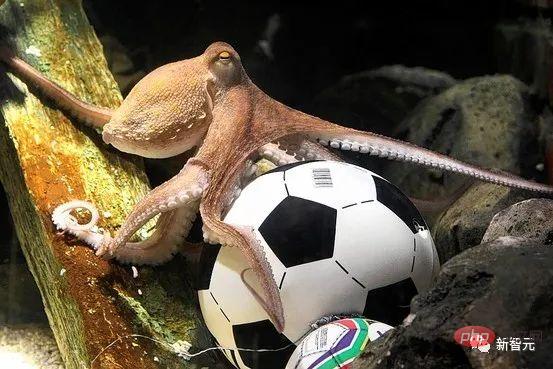
说起2010年南非世界杯的最大网红,一定非「章鱼保罗」莫属!这只位于德国海洋生物中心的神奇章鱼,不仅成功预测了德国队全部七场比赛的结果,还顺利地选出了最终的总冠军西班牙队。不幸的是,保罗已经永远地离开了我们,但它的「遗产」却在人们预测足球比赛结果的尝试中持续存在。在艾伦图灵研究所(The Alan Turing Institute),随着2022年卡塔尔世界杯的持续进行,三位研究员Nick Barlow、Jack Roberts和Ryan Chan决定用一种AI算法预测今年的冠军归属。预测模型图


Hot AI Tools

Undresser.AI Undress
AI-powered app for creating realistic nude photos

AI Clothes Remover
Online AI tool for removing clothes from photos.

Undress AI Tool
Undress images for free

Clothoff.io
AI clothes remover

AI Hentai Generator
Generate AI Hentai for free.

Hot Article

Hot Tools

SublimeText3 Chinese version
Chinese version, very easy to use
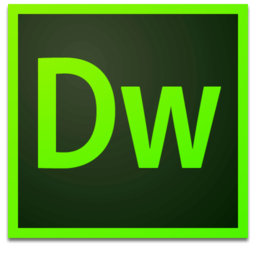
Dreamweaver Mac version
Visual web development tools
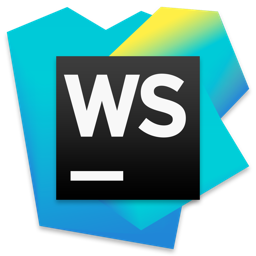
WebStorm Mac version
Useful JavaScript development tools

Notepad++7.3.1
Easy-to-use and free code editor

SecLists
SecLists is the ultimate security tester's companion. It is a collection of various types of lists that are frequently used during security assessments, all in one place. SecLists helps make security testing more efficient and productive by conveniently providing all the lists a security tester might need. List types include usernames, passwords, URLs, fuzzing payloads, sensitive data patterns, web shells, and more. The tester can simply pull this repository onto a new test machine and he will have access to every type of list he needs.
