MongoDB's practical summary of large-scale data storage and index optimization
MongoDB is a popular NoSQL database suitable for large-scale data storage and processing. This article will summarize the practical experience of MongoDB in large-scale data storage and index optimization.
First of all, in terms of large-scale data storage, MongoDB provides scalability and high-performance solutions. In terms of storage, we can use sharding to distribute data across multiple hosts to achieve horizontal expansion of data. Each shard is an independent cluster and can carry large amounts of data. In addition, we can achieve redundant storage of data through replica sets to improve data availability and fault tolerance. By setting up a replica set, if one node fails, the system will automatically transfer read and write requests to other nodes, thus ensuring the stability and reliability of the entire system.
Secondly, index optimization is the key to improving query performance. MongoDB supports multiple types of indexes, including single-field indexes, compound indexes, text indexes, etc. When designing indexes, we need to make trade-offs based on specific query needs and data characteristics. For frequently used query fields, you can consider creating a single-field index, which can greatly improve query performance. For queries on multiple fields, you can create a composite index to achieve more efficient queries by combining multiple fields. Additionally, for fields that contain text, we can use full-text indexing for efficient text searches.
In addition, reasonable data model design is also an important factor in improving performance. When designing a data model, we need to consider the relationship between data and the frequency of queries, as well as the complexity of queries. Generally speaking, putting related data in the same document can improve query efficiency and avoid multiple queries and connection operations. At the same time, we can use nested documents and arrays to represent complex data structures, thereby reducing the complexity of data storage and querying.
In addition, performance can be improved by adding hardware resources. MongoDB supports adding more nodes in the cluster to expand the computing and storage capabilities of the system. We can increase the throughput of the system through horizontal expansion, and the number and size of nodes can be adjusted according to specific needs.
Finally, monitoring and optimization are important tasks that cannot be ignored. We can discover potential problems and bottlenecks by monitoring the running status of the system and query performance indicators, and then take corresponding optimization measures. For example, by using MongoDB's own monitoring tools or third-party monitoring software, we can monitor various indicators of the system in real time, including the usage of resources such as CPU, memory, and disk, as well as performance indicators such as query response time and throughput. By analyzing these indicators, we can discover problems in time and perform performance tuning to improve system stability and availability.
To sum up, MongoDB has a lot of practical experience in large-scale data storage and index optimization. Through reasonable data model design, index optimization, hardware resource expansion and monitoring optimization, we can improve the performance and stability of the MongoDB system and meet the needs of large-scale data storage and processing. At the same time, as technology continues to develop, we also need to continue to learn and practice to adapt to changing data storage and processing needs.
The above is the detailed content of MongoDB's practical summary of large-scale data storage and index optimization. For more information, please follow other related articles on the PHP Chinese website!
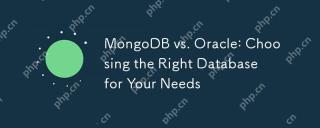
MongoDB is suitable for unstructured data and high scalability requirements, while Oracle is suitable for scenarios that require strict data consistency. 1.MongoDB flexibly stores data in different structures, suitable for social media and the Internet of Things. 2. Oracle structured data model ensures data integrity and is suitable for financial transactions. 3.MongoDB scales horizontally through shards, and Oracle scales vertically through RAC. 4.MongoDB has low maintenance costs, while Oracle has high maintenance costs but is fully supported.
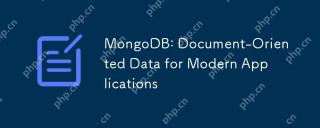
MongoDB has changed the way of development with its flexible documentation model and high-performance storage engine. Its advantages include: 1. Patternless design, allowing fast iteration; 2. The document model supports nesting and arrays, enhancing data structure flexibility; 3. The automatic sharding function supports horizontal expansion, suitable for large-scale data processing.
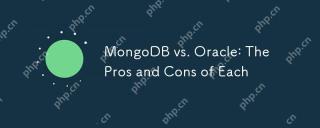
MongoDB is suitable for projects that iterate and process large-scale unstructured data quickly, while Oracle is suitable for enterprise-level applications that require high reliability and complex transaction processing. MongoDB is known for its flexible document storage and efficient read and write operations, suitable for modern web applications and big data analysis; Oracle is known for its strong data management capabilities and SQL support, and is widely used in industries such as finance and telecommunications.
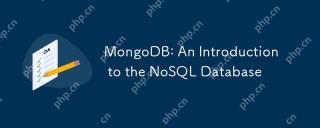
MongoDB is a document-based NoSQL database that uses BSON format to store data, suitable for processing complex and unstructured data. 1) Its document model is flexible and suitable for frequently changing data structures. 2) MongoDB uses WiredTiger storage engine and query optimizer to support efficient data operations and queries. 3) Basic operations include inserting, querying, updating and deleting documents. 4) Advanced usage includes using an aggregation framework for complex data analysis. 5) Common errors include connection problems, query performance problems, and data consistency problems. 6) Performance optimization and best practices include index optimization, data modeling, sharding, caching, monitoring and tuning.
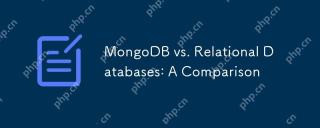
MongoDB is suitable for scenarios that require flexible data models and high scalability, while relational databases are more suitable for applications that complex queries and transaction processing. 1) MongoDB's document model adapts to the rapid iterative modern application development. 2) Relational databases support complex queries and financial systems through table structure and SQL. 3) MongoDB achieves horizontal scaling through sharding, which is suitable for large-scale data processing. 4) Relational databases rely on vertical expansion and are suitable for scenarios where queries and indexes need to be optimized.
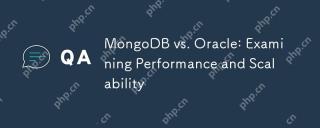
MongoDB performs excellent in performance and scalability, suitable for high scalability and flexibility requirements; Oracle performs excellent in requiring strict transaction control and complex queries. 1.MongoDB achieves high scalability through sharding technology, suitable for large-scale data and high concurrency scenarios. 2. Oracle relies on optimizers and parallel processing to improve performance, suitable for structured data and transaction control needs.
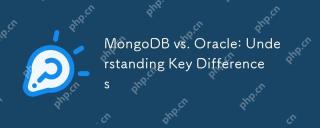
MongoDB is suitable for handling large-scale unstructured data, and Oracle is suitable for enterprise-level applications that require transaction consistency. 1.MongoDB provides flexibility and high performance, suitable for processing user behavior data. 2. Oracle is known for its stability and powerful functions and is suitable for financial systems. 3.MongoDB uses document models, and Oracle uses relational models. 4.MongoDB is suitable for social media applications, while Oracle is suitable for enterprise-level applications.
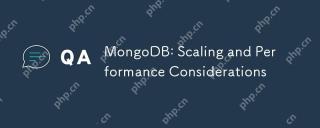
MongoDB's scalability and performance considerations include horizontal scaling, vertical scaling, and performance optimization. 1. Horizontal expansion is achieved through sharding technology to improve system capacity. 2. Vertical expansion improves performance by increasing hardware resources. 3. Performance optimization is achieved through rational design of indexes and optimized query strategies.


Hot AI Tools

Undresser.AI Undress
AI-powered app for creating realistic nude photos

AI Clothes Remover
Online AI tool for removing clothes from photos.

Undress AI Tool
Undress images for free

Clothoff.io
AI clothes remover

Video Face Swap
Swap faces in any video effortlessly with our completely free AI face swap tool!

Hot Article

Hot Tools

MantisBT
Mantis is an easy-to-deploy web-based defect tracking tool designed to aid in product defect tracking. It requires PHP, MySQL and a web server. Check out our demo and hosting services.
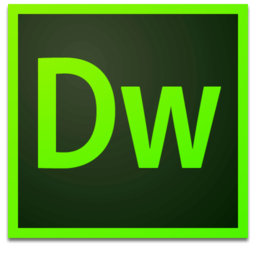
Dreamweaver Mac version
Visual web development tools
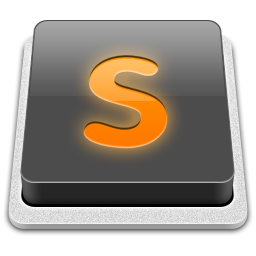
SublimeText3 Mac version
God-level code editing software (SublimeText3)

PhpStorm Mac version
The latest (2018.2.1) professional PHP integrated development tool
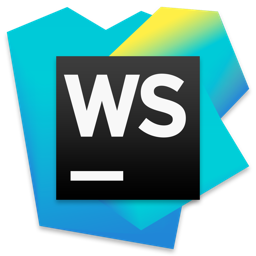
WebStorm Mac version
Useful JavaScript development tools