


I am used to Stable Diffusion, and now I finally have a Matryoshka-style Diffusion model, made by Apple.
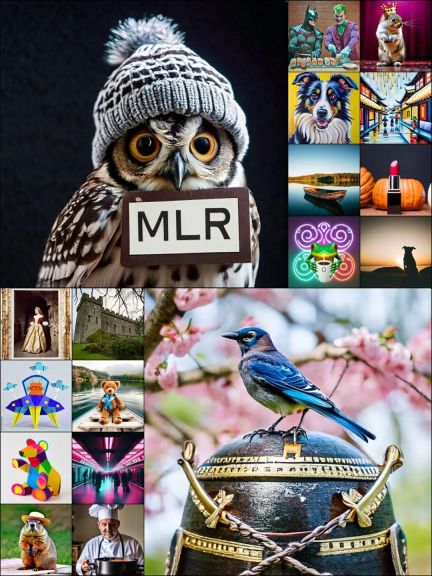
The above is the detailed content of Apple's large Vincent picture model unveiled: Russian matryoshka-like spread, supporting 1024x1024 resolution. For more information, please follow other related articles on the PHP Chinese website!
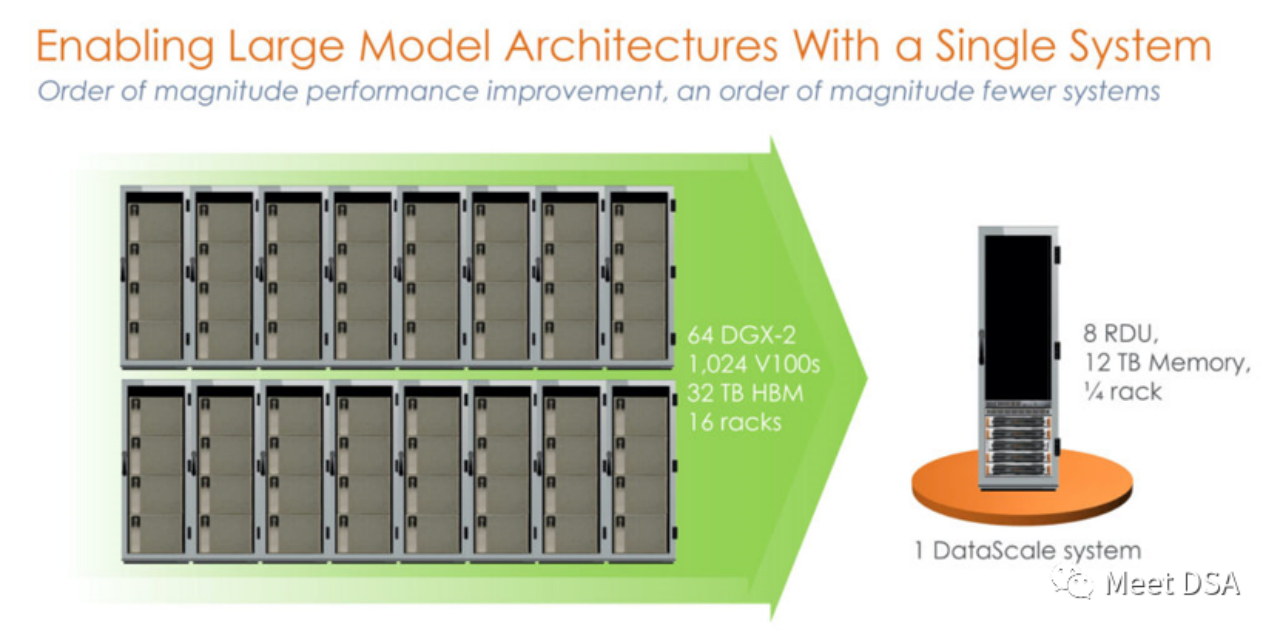
你可能听过以下犀利的观点:1.跟着NVIDIA的技术路线,可能永远也追不上NVIDIA的脚步。2.DSA或许有机会追赶上NVIDIA,但目前的状况是DSA濒临消亡,看不到任何希望另一方面,我们都知道现在大模型正处于风口位置,业界很多人想做大模型芯片,也有很多人想投大模型芯片。但是,大模型芯片的设计关键在哪,大带宽大内存的重要性好像大家都知道,但做出来的芯片跟NVIDIA相比,又有何不同?带着问题,本文尝试给大家一点启发。纯粹以观点为主的文章往往显得形式主义,我们可以通过一个架构的例子来说明Sam
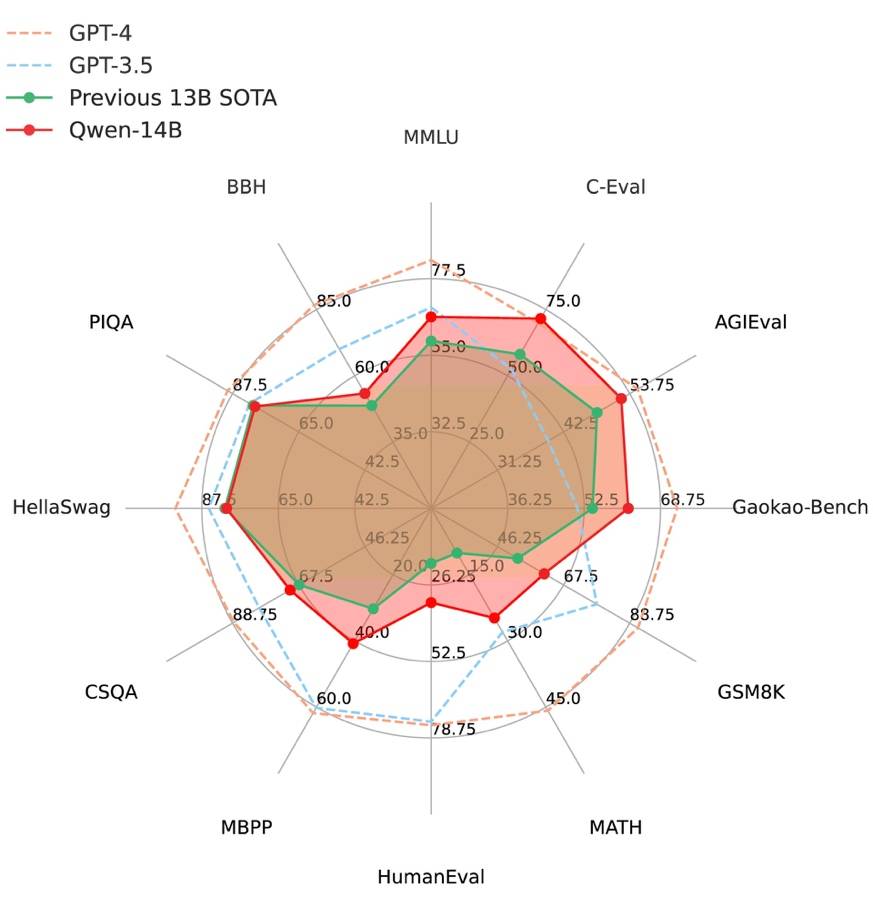
2021年9月25日,阿里云发布了开源项目通义千问140亿参数模型Qwen-14B以及其对话模型Qwen-14B-Chat,并且可以免费商用。Qwen-14B在多个权威评测中表现出色,超过了同等规模的模型,甚至有些指标接近Llama2-70B。此前,阿里云还开源了70亿参数模型Qwen-7B,仅一个多月的时间下载量就突破了100万,成为开源社区的热门项目Qwen-14B是一款支持多种语言的高性能开源模型,相比同类模型使用了更多的高质量数据,整体训练数据超过3万亿Token,使得模型具备更强大的推
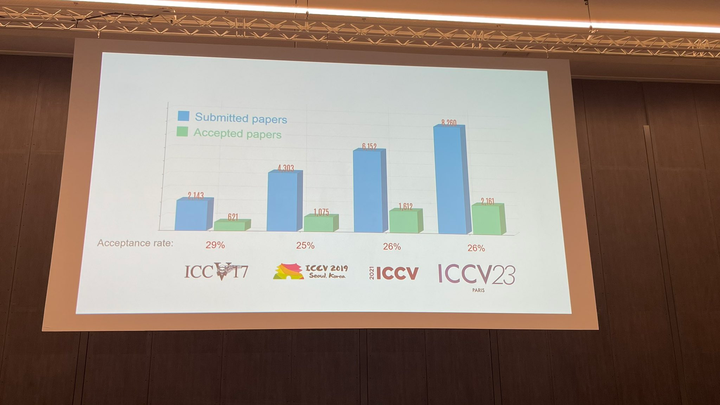
在法国巴黎举行了国际计算机视觉大会ICCV(InternationalConferenceonComputerVision)本周开幕作为全球计算机视觉领域顶级的学术会议,ICCV每两年召开一次。ICCV的热度一直以来都与CVPR不相上下,屡创新高在今天的开幕式上,ICCV官方公布了今年的论文数据:本届ICCV共有8068篇投稿,其中有2160篇被接收,录用率为26.8%,略高于上一届ICCV2021的录用率25.9%在论文主题方面,官方也公布了相关数据:多视角和传感器的3D技术热度最高在今天的开

随着智慧司法的兴起,智能化方法驱动的智能法律系统有望惠及不同群体。例如,为法律专业人员减轻文书工作,为普通民众提供法律咨询服务,为法学学生提供学习和考试辅导。由于法律知识的独特性和司法任务的多样性,此前的智慧司法研究方面主要着眼于为特定任务设计自动化算法,难以满足对司法领域提供支撑性服务的需求,离应用落地有不小的距离。而大型语言模型(LLMs)在不同的传统任务上展示出强大的能力,为智能法律系统的进一步发展带来希望。近日,复旦大学数据智能与社会计算实验室(FudanDISC)发布大语言模型驱动的中
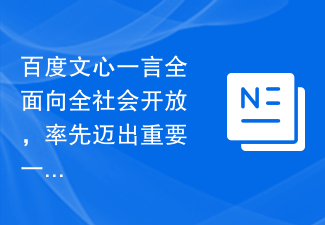
8月31日,文心一言首次向全社会全面开放。用户可以在应用商店下载“文心一言APP”或登录“文心一言官网”(https://yiyan.baidu.com)进行体验据报道,百度计划推出一系列经过全新重构的AI原生应用,以便让用户充分体验生成式AI的理解、生成、逻辑和记忆等四大核心能力今年3月16日,文心一言开启邀测。作为全球大厂中首个发布的生成式AI产品,文心一言的基础模型文心大模型早在2019年就在国内率先发布,近期升级的文心大模型3.5也持续在十余个国内外权威测评中位居第一。李彦宏表示,当文心
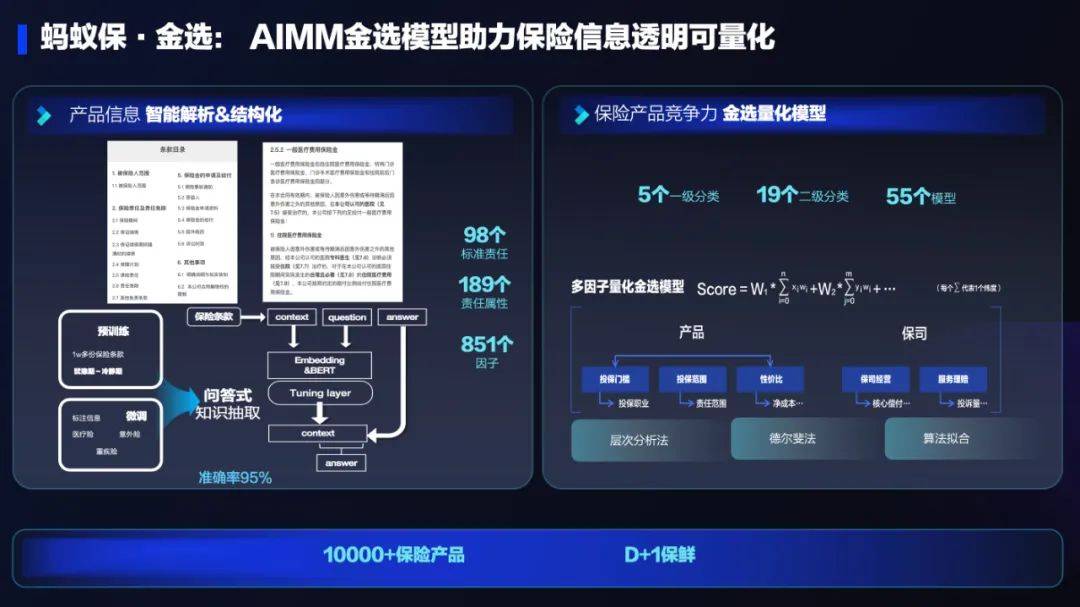
保险行业对于社会民生和国民经济的重要性不言而喻。作为风险管理工具,保险为人民群众提供保障和福利,推动经济的稳定和可持续发展。在新的时代背景下,保险行业面临着新的机遇和挑战,需要不断创新和转型,以适应社会需求的变化和经济结构的调整近年来,中国的保险科技蓬勃发展。通过创新的商业模式和先进的技术手段,积极推动保险行业实现数字化和智能化转型。保险科技的目标是提升保险服务的便利性、个性化和智能化水平,以前所未有的速度改变传统保险业的面貌。这一发展趋势为保险行业注入了新的活力,使保险产品更贴近人民群众的实际
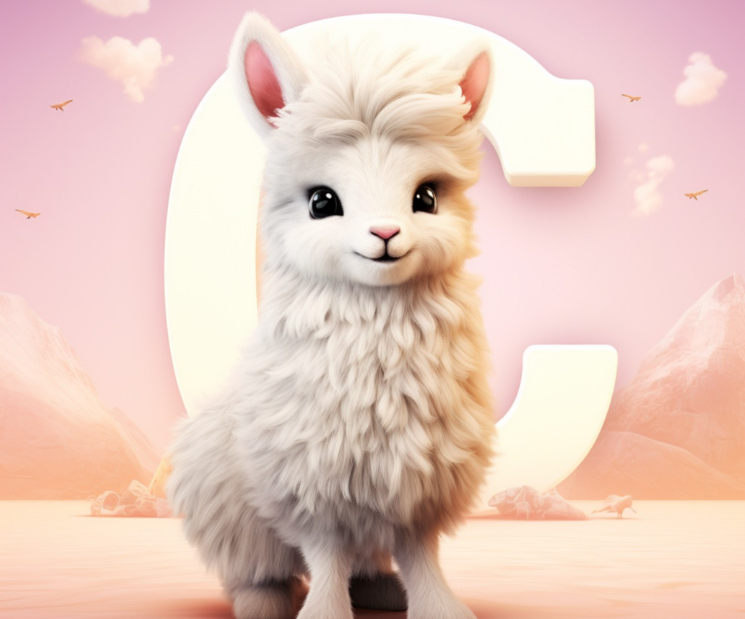
不得不说,Llama2的「二创」项目越来越硬核、有趣了。自Meta发布开源大模型Llama2以来,围绕着该模型的「二创」项目便多了起来。此前7月,特斯拉前AI总监、重回OpenAI的AndrejKarpathy利用周末时间,做了一个关于Llama2的有趣项目llama2.c,让用户在PyTorch中训练一个babyLlama2模型,然后使用近500行纯C、无任何依赖性的文件进行推理。今天,在Karpathyllama2.c项目的基础上,又有开发者创建了一个启动Llama2的演示操作系统,以及一个
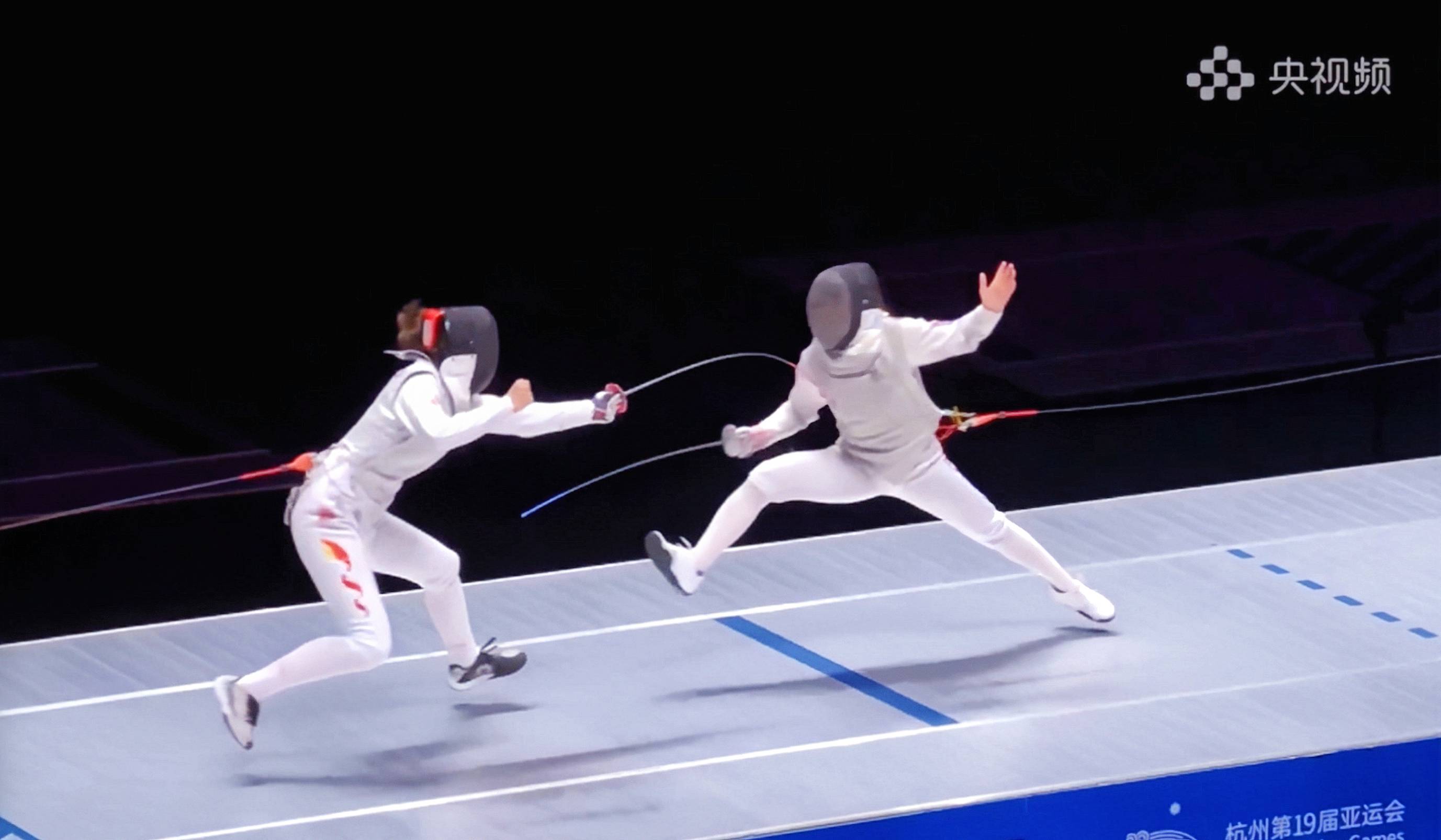
杭州第19届亚运会不仅是国际顶级体育盛会,更是一场精彩绝伦的中国科技盛宴。本届亚运会中,快手StreamLake与杭州电信深度合作,联合打造智慧观赛新体验,在击剑赛事的转播中,全面应用了快手StreamLake六自由度技术,其中“子弹时间”也是首次应用于击剑项目国际顶级赛事。中国电信杭州分公司智能亚运专班组长芮杰表示,依托快手StreamLake自研的4K3D虚拟运镜视频技术和中国电信5G/全光网,通过赛场内部署的4K专业摄像机阵列实时采集的高清竞赛视频,


Hot AI Tools

Undresser.AI Undress
AI-powered app for creating realistic nude photos

AI Clothes Remover
Online AI tool for removing clothes from photos.

Undress AI Tool
Undress images for free

Clothoff.io
AI clothes remover

AI Hentai Generator
Generate AI Hentai for free.

Hot Article

Hot Tools
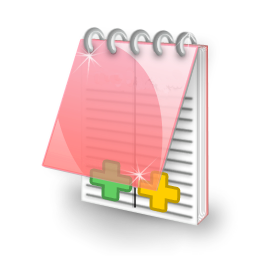
EditPlus Chinese cracked version
Small size, syntax highlighting, does not support code prompt function

SublimeText3 English version
Recommended: Win version, supports code prompts!

MinGW - Minimalist GNU for Windows
This project is in the process of being migrated to osdn.net/projects/mingw, you can continue to follow us there. MinGW: A native Windows port of the GNU Compiler Collection (GCC), freely distributable import libraries and header files for building native Windows applications; includes extensions to the MSVC runtime to support C99 functionality. All MinGW software can run on 64-bit Windows platforms.

SublimeText3 Linux new version
SublimeText3 Linux latest version

SAP NetWeaver Server Adapter for Eclipse
Integrate Eclipse with SAP NetWeaver application server.
