


The latest research results of the Peking University team show that:
random token can induce hallucination in large models!
For example, if the large model (Vicuna-7B) is given a "garbled code", it will inexplicably misunderstand historical common sense
Even with some simple modification tips, large models may fall into traps
These popular large models, such as Baichuan2-7B, InternLM-7B, ChatGLM, Ziya-LLaMA -7B, LLaMA-7B-chat and Vicuna-7B will all encounter similar situations
This means that random strings can control large models to output arbitrary content, "endorsing illusions" ".
The above findings come from the latest research by the research group of Professor Yuan Li of Peking University.
This study proposes:
The hallucination phenomenon of large models is very likely to be another perspective of adversarial examples.
The paper not only shows two methods that can easily induce large model hallucinations, but also proposes simple and effective defense methods. The code has been open source.
Two extreme mode attack large models
The study proposed two hallucination attack methods:
- Random noise attack (OoD attack) is a common machine Learn model attack methods. In this attack, the attacker feeds the model some random noise that is not common in the training data. This noise can interfere with the model’s ability to make judgments, causing it to make erroneous predictions when processing data from the real world. Random noise attack is a covert attack method because it uses similar characteristics to normal data and is difficult to be detected by the model. In order to resist this attack, some effective anomaly detection methods need to be used to identify and filter out these random noises, that is, to allow meaningless random strings to induce large models to produce predefined phantom outputs.
- Weak Semantic Attack refers to a common attack method on the Internet. This attack method is typically carried out by persuading users to unknowingly provide personal information or perform malicious actions. Compared with other more direct attack methods, weak semantic attacks are more subtle and often use social engineering and deception to mislead users. Internet users should be vigilant to avoid being affected by weak semantic attacks, which cause large models to produce completely different illusory output while keeping the original prompt semantics basically unchanged.
Random Noise Attack (OoD Attack):
The following are some experimental results conducted on open source large models. More results can be found in the paper or Found in open source GitHub
Weak Semantic Attack(Weak Semantic Attack):
paper The hallucination attack method is introduced:
According to the diagram, the hallucination attack consists of the following three parts: the construction of the hallucination data set, weak semantic attack and OoD attack
The first is hallucination data set construction.
The author collected some common questions x and input them into a large model, and got the correct answer y
Then he replaced the subject, predicate and object of the sentence to construct a non-existent fact, where T is the set containing all consistent facts.
Finally, the result of constructing the hallucination data set can be obtained:
Then the weak semantic attack part.
First sample a QA pair that does not conform to the facts, and start the illusion of stability in the future
. The author hopes to find an adversarial prompt
to maximize the log likelihood.
where is the parameter of the large model and
is the input space.
is composed of l tokens.
However, since the language is discontinuous, there is no way to directly optimize x like adversarial attacks in the image field.
Inspired by a 2019 study (Universal Adversarial Triggers for Attacking and Analyzing NLP), the research team used a gradient-based token replacement strategy to indirectly maximize the log likelihood.
Among them, is the embedding against token
, and
is a semantic extractor.
Let’s look at this formula simply. Under semantic constraints, find those tokens that make the likelihood gradient change the most and replace them. Finally, we can ensure that the obtained adversarial prompt is semantically consistent with the original prompt x. In too many cases, the model is induced to output predefined hallucinations
.
In this article, in order to simplify the optimization process, the constraint item is changed to instead.
The last part is the OoD attack
In the OoD attack, we start from a completely random string, without any semantic constraints, to maximize the above log likelihood, that is Can.
The paper also elaborates on the attack success rate of hallucination attacks on different models and different modes.
The length of the prompt is increased to improve the attack success rate. An in-depth discussion (doubled)
The research team finally proposed a simple defense strategy, which is to reject the response by exploiting the entropy predicted by the first token
This research comes from the team of Professor Yuan Li from Peking University Shenzhen Graduate School/School of Information Engineering.
Paper link: https://arxiv.org/pdf/2310.01469.pdf
##GitHub address: https:// github.com/PKU-YuanGroup/Hallucination-Attack
Zhihu original post
The content that needs to be rewritten is: https://zhuanlan.zhihu.com/p/661444210?
The above is the detailed content of Peking University team: All it takes to induce the 'hallucination' of a large model is a string of garbled characters! All big and small alpacas are recruited. For more information, please follow other related articles on the PHP Chinese website!
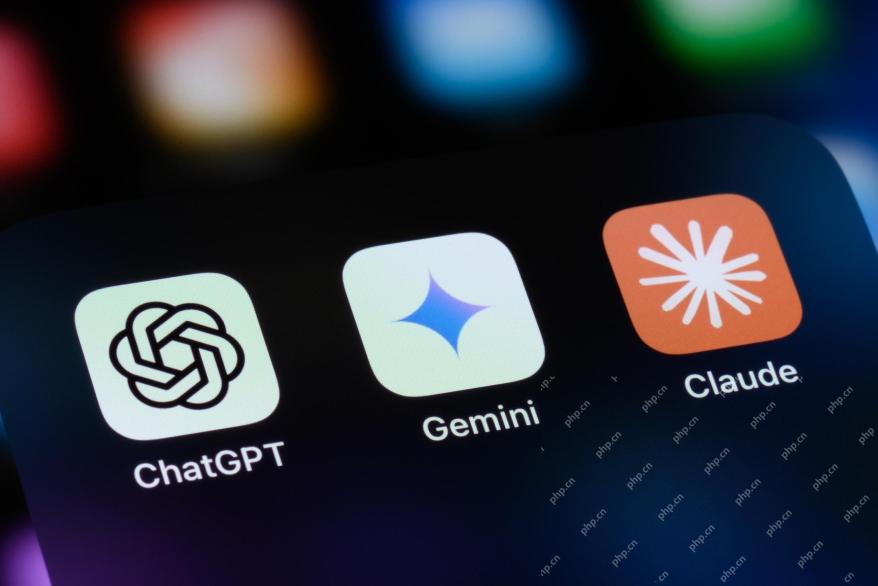
The unchecked internal deployment of advanced AI systems poses significant risks, according to a new report from Apollo Research. This lack of oversight, prevalent among major AI firms, allows for potential catastrophic outcomes, ranging from uncont
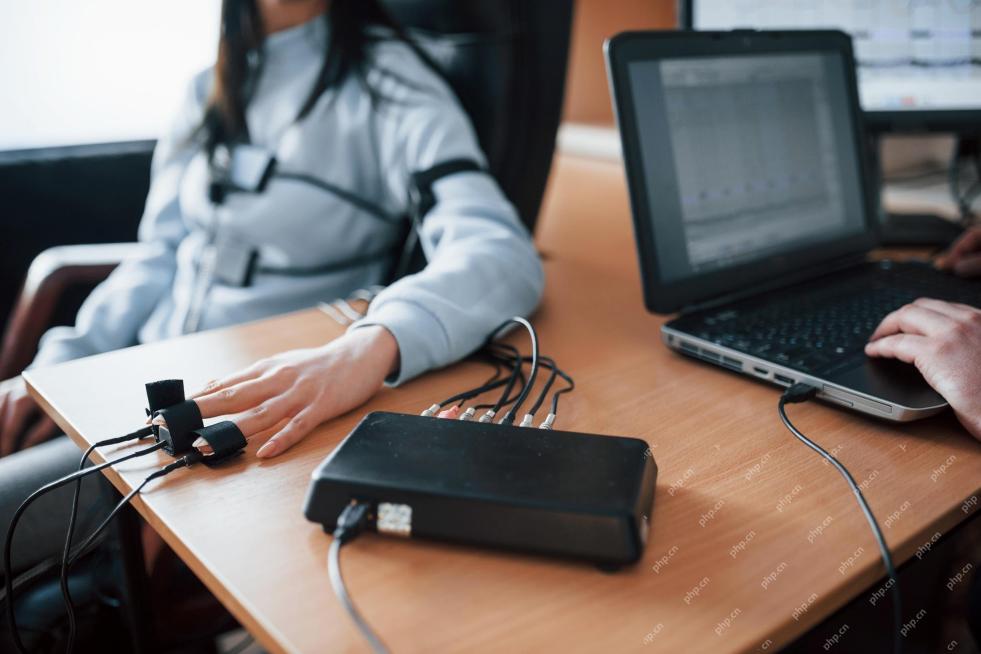
Traditional lie detectors are outdated. Relying on the pointer connected by the wristband, a lie detector that prints out the subject's vital signs and physical reactions is not accurate in identifying lies. This is why lie detection results are not usually adopted by the court, although it has led to many innocent people being jailed. In contrast, artificial intelligence is a powerful data engine, and its working principle is to observe all aspects. This means that scientists can apply artificial intelligence to applications seeking truth through a variety of ways. One approach is to analyze the vital sign responses of the person being interrogated like a lie detector, but with a more detailed and precise comparative analysis. Another approach is to use linguistic markup to analyze what people actually say and use logic and reasoning. As the saying goes, one lie breeds another lie, and eventually
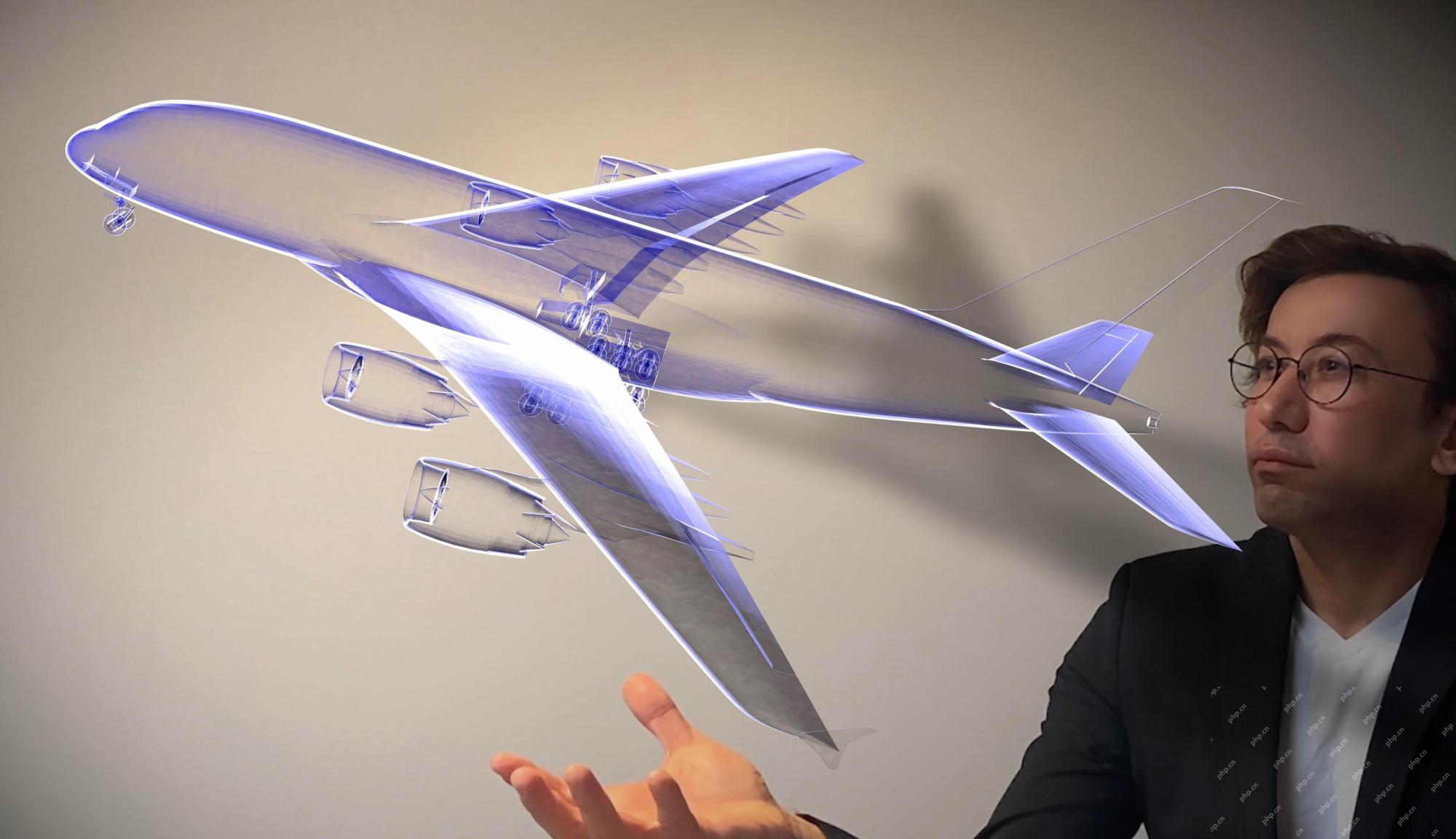
The aerospace industry, a pioneer of innovation, is leveraging AI to tackle its most intricate challenges. Modern aviation's increasing complexity necessitates AI's automation and real-time intelligence capabilities for enhanced safety, reduced oper
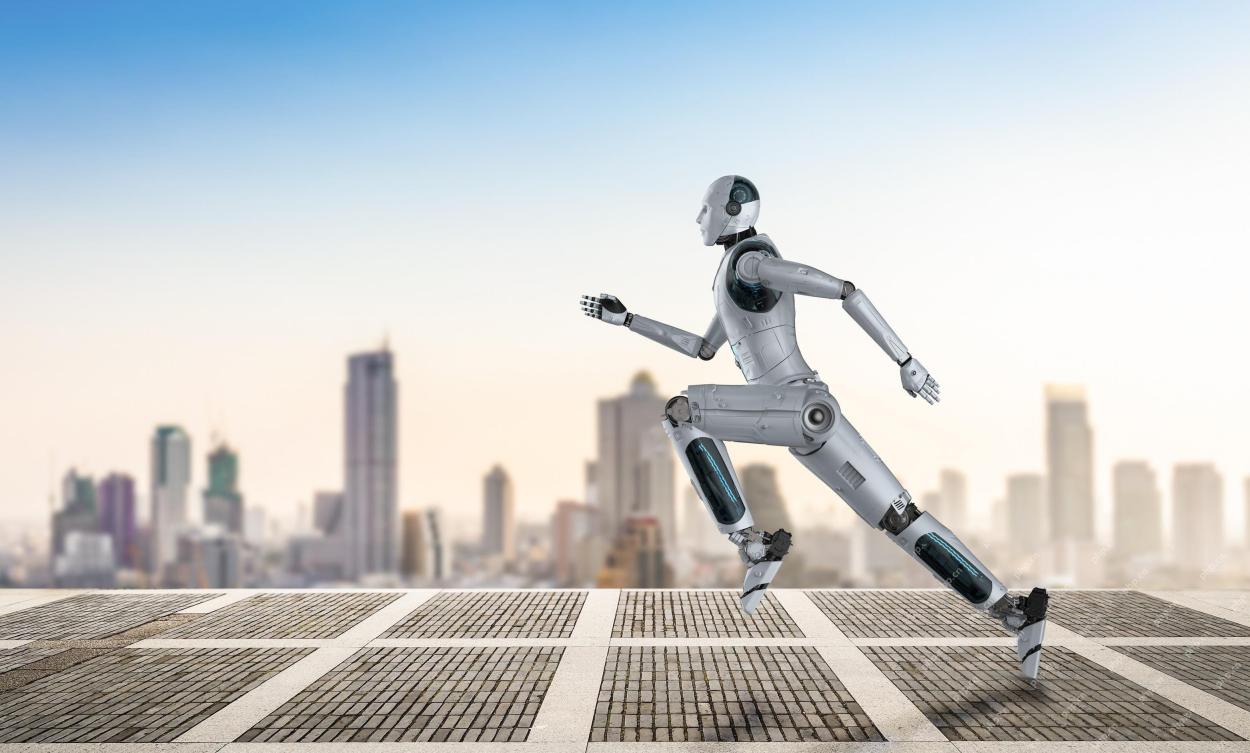
The rapid development of robotics has brought us a fascinating case study. The N2 robot from Noetix weighs over 40 pounds and is 3 feet tall and is said to be able to backflip. Unitree's G1 robot weighs about twice the size of the N2 and is about 4 feet tall. There are also many smaller humanoid robots participating in the competition, and there is even a robot that is driven forward by a fan. Data interpretation The half marathon attracted more than 12,000 spectators, but only 21 humanoid robots participated. Although the government pointed out that the participating robots conducted "intensive training" before the competition, not all robots completed the entire competition. Champion - Tiangong Ult developed by Beijing Humanoid Robot Innovation Center
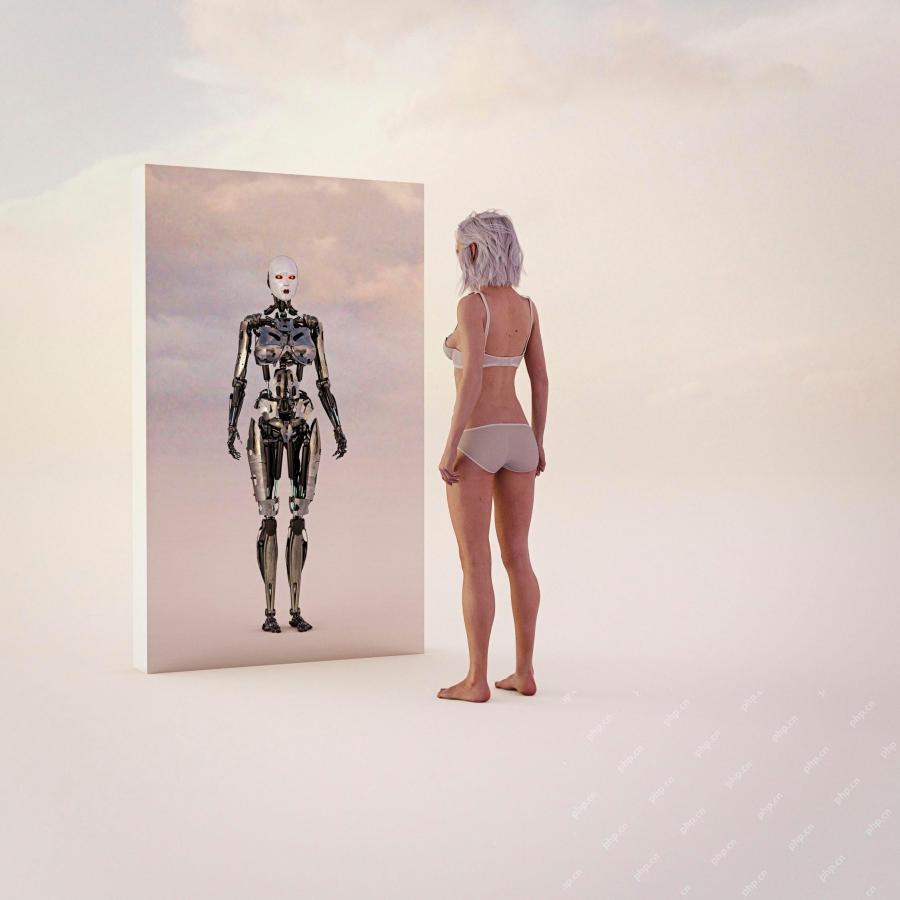
Artificial intelligence, in its current form, isn't truly intelligent; it's adept at mimicking and refining existing data. We're not creating artificial intelligence, but rather artificial inference—machines that process information, while humans su
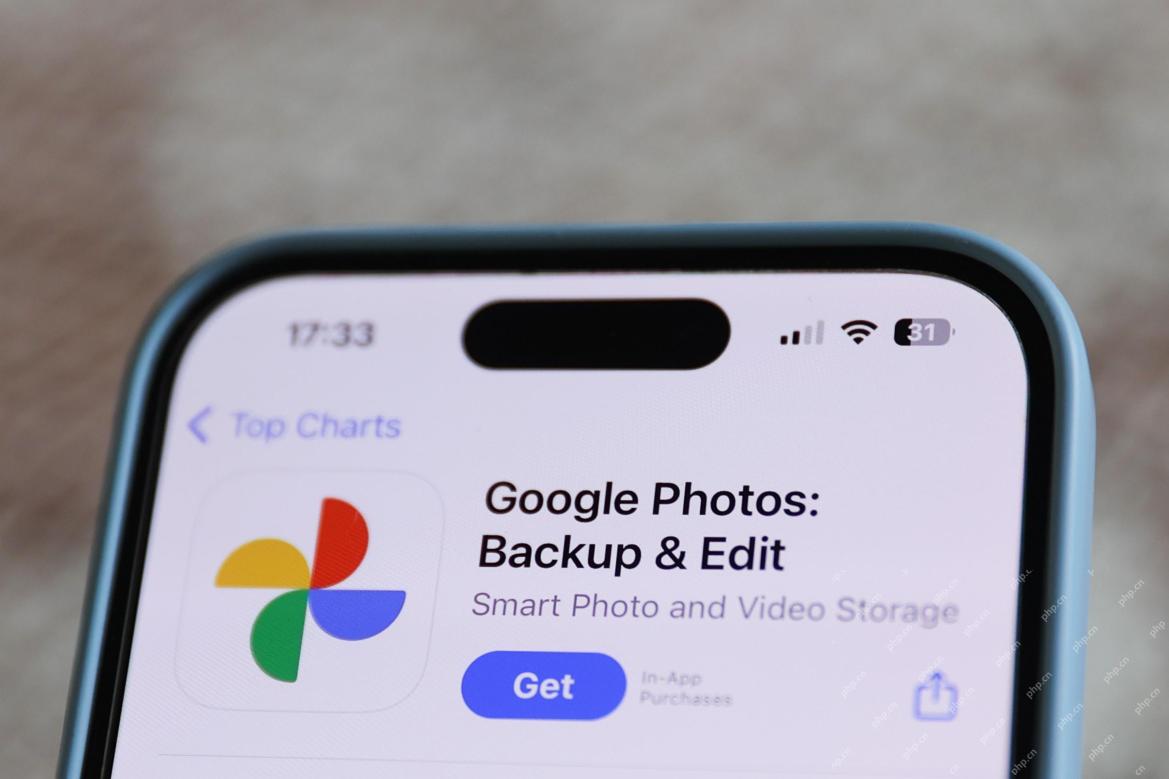
A report found that an updated interface was hidden in the code for Google Photos Android version 7.26, and each time you view a photo, a row of newly detected face thumbnails are displayed at the bottom of the screen. The new facial thumbnails are missing name tags, so I suspect you need to click on them individually to see more information about each detected person. For now, this feature provides no information other than those people that Google Photos has found in your images. This feature is not available yet, so we don't know how Google will use it accurately. Google can use thumbnails to speed up finding more photos of selected people, or may be used for other purposes, such as selecting the individual to edit. Let's wait and see. As for now
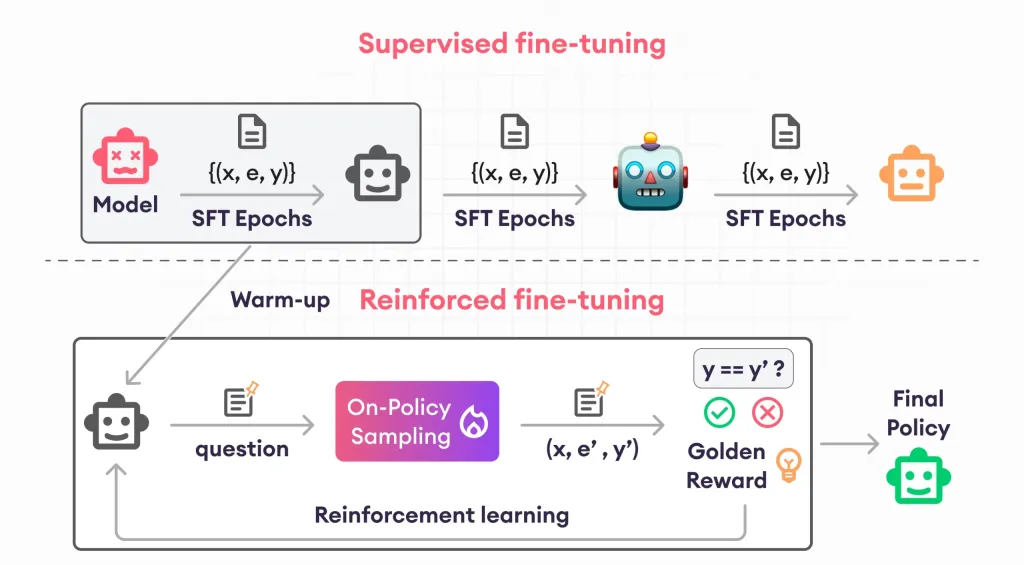
Reinforcement finetuning has shaken up AI development by teaching models to adjust based on human feedback. It blends supervised learning foundations with reward-based updates to make them safer, more accurate, and genuinely help
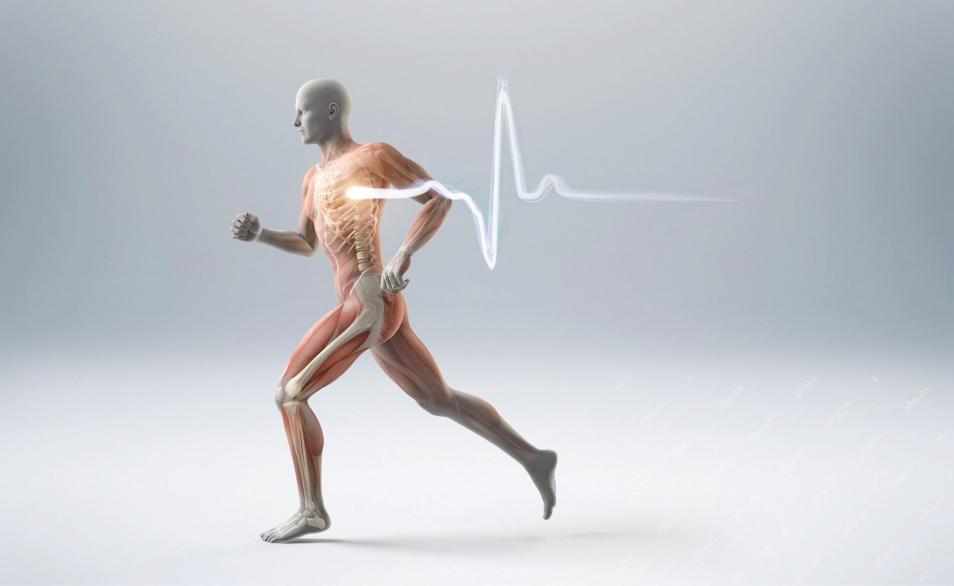
Scientists have extensively studied human and simpler neural networks (like those in C. elegans) to understand their functionality. However, a crucial question arises: how do we adapt our own neural networks to work effectively alongside novel AI s


Hot AI Tools

Undresser.AI Undress
AI-powered app for creating realistic nude photos

AI Clothes Remover
Online AI tool for removing clothes from photos.

Undress AI Tool
Undress images for free

Clothoff.io
AI clothes remover

Video Face Swap
Swap faces in any video effortlessly with our completely free AI face swap tool!

Hot Article

Hot Tools
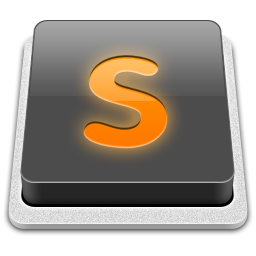
SublimeText3 Mac version
God-level code editing software (SublimeText3)

Zend Studio 13.0.1
Powerful PHP integrated development environment

PhpStorm Mac version
The latest (2018.2.1) professional PHP integrated development tool

SecLists
SecLists is the ultimate security tester's companion. It is a collection of various types of lists that are frequently used during security assessments, all in one place. SecLists helps make security testing more efficient and productive by conveniently providing all the lists a security tester might need. List types include usernames, passwords, URLs, fuzzing payloads, sensitive data patterns, web shells, and more. The tester can simply pull this repository onto a new test machine and he will have access to every type of list he needs.

SublimeText3 English version
Recommended: Win version, supports code prompts!
