


The perfect combination of ChatGPT and Python: creating an intelligent customer service chatbot
The perfect combination of ChatGPT and Python: creating an intelligent customer service chatbot
Introduction:
In today’s information age, intelligent customer service systems have become the link between enterprises and customers Important communication tool. In order to provide a better customer service experience, many companies have begun to turn to chatbots to complete tasks such as customer consultation and question answering. In this article, we will introduce how to use OpenAI’s powerful model ChatGPT and Python language to create an intelligent customer service chatbot to improve customer satisfaction and work efficiency.
- Preparation
First, we need to install the following Python libraries and tools: - Python 3
- OpenAI Gym
- TensorFlow
- OpenAI’s GPT model library
- PyTorch
- Data collection and preprocessing
In order to train our chatbot, we need to prepare a large amount of conversation data. This can be obtained from the company's historical customer service chat records, or by leveraging existing public data sets. Either way, you need to make sure the data is of good quality and formatted correctly.
Next, we use Python for data preprocessing. First, convert the conversation data into a suitable format, such as saving the questions and answers for each conversation as one line, separated by symbols such as tabs or commas. Then, perform text cleaning as needed, such as removing invalid characters, punctuation, etc. Finally, the data set is divided into a training set and a test set, usually using a ratio of 80% training set and 20% test set.
- Building ChatGPT model
In Python, we can use the GPT model library provided by OpenAI to build the ChatGPT model. First, import the necessary libraries and modules, such as tensorflow, transformers, etc. Then, load the pre-trained GPT model, which can be a pre-trained model provided by OpenAI, or a model obtained by training on a large-scale data set. For detailed procedures on how to train a GPT model, please refer to OpenAI’s documentation.
Next, we need to define an optimizer and loss function. ChatGPT models are usually trained using the Adam optimizer and cross-entropy loss function. Then, write a training loop that continuously adjusts the model weights through multiple iterations until the loss function converges or reaches a preset stopping condition.
- Deploying Chatbot
After training is completed, we can deploy the ChatGPT model to a server or cloud environment to respond to customer questions in real time. This can be achieved through Python’s Flask framework. First, install the Flask library and create a Flask application. Then, write a routing function to receive and process the client's HTTP request. In this routing function, we load the trained ChatGPT model and generate answers based on the input text. Finally, the answer is returned to the client in JSON format. - Run and Test
After deploying the chatbot, we can interact with the robot by sending HTTP requests to the server. You can use tools such as Postman to simulate the client's request and observe the bot's answers. At the same time, we can also write test functions in the code for automated testing of chatbots.
Conclusion:
By combining ChatGPT and Python language, we can easily build an intelligent customer service chatbot. This chatbot has a high level of intelligence and can interact with users in real time and provide accurate and useful answers. This will greatly improve customer satisfaction and work efficiency, bringing greater business value to the enterprise.
It should be noted that chatbots only provide automated answers based on rules and models and cannot completely replace human customer service. In practical applications, manual intervention and review may also be required to ensure the accuracy and reliability of answers. At the same time, chatbot training data and models also need to be continuously optimized and improved to adapt to changing user needs and industry environments.
Code example (based on Flask framework):
from flask import Flask, request, jsonify from transformers import BertTokenizer, TFBertForSequenceClassification app = Flask(__name__) # 加载训练好的ChatGPT模型 tokenizer = BertTokenizer.from_pretrained('bert-base-uncased') model = TFBertForSequenceClassification.from_pretrained('bert-base-uncased') @app.route('/chatbot', methods=['POST']) def chatbot(): text = request.json.get('text', '') # 文本预处理 inputs = tokenizer.encode_plus( text, None, add_special_tokens=True, max_length=512, pad_to_max_length=True, return_attention_mask=True, return_token_type_ids=True, truncation=True ) input_ids = inputs['input_ids'] attention_mask = inputs['attention_mask'] token_type_ids = inputs['token_type_ids'] # 调用ChatGPT模型生成回答 outputs = model({'input_ids': input_ids, 'attention_mask': attention_mask, 'token_type_ids': token_type_ids}) predicted_label = torch.argmax(outputs.logits).item() return jsonify({'answer': predicted_label}) if __name__ == '__main__': app.run(host='0.0.0.0', port=5000)
The above is a simple example for reference only. It can be modified and expanded according to actual conditions to meet your needs.
References:
- OpenAI GPT model: https://openai.com/models/gpt
- Flask official documentation: https://flask.palletsprojects .com/
- Transformers library documentation: https://huggingface.co/transformers/
- TensorFlow official documentation: https://www.tensorflow.org/
The above is the detailed content of The perfect combination of ChatGPT and Python: creating an intelligent customer service chatbot. For more information, please follow other related articles on the PHP Chinese website!
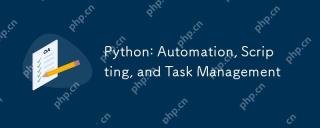
Python excels in automation, scripting, and task management. 1) Automation: File backup is realized through standard libraries such as os and shutil. 2) Script writing: Use the psutil library to monitor system resources. 3) Task management: Use the schedule library to schedule tasks. Python's ease of use and rich library support makes it the preferred tool in these areas.
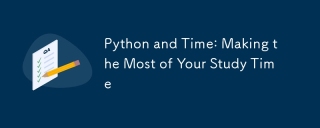
To maximize the efficiency of learning Python in a limited time, you can use Python's datetime, time, and schedule modules. 1. The datetime module is used to record and plan learning time. 2. The time module helps to set study and rest time. 3. The schedule module automatically arranges weekly learning tasks.
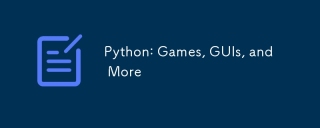
Python excels in gaming and GUI development. 1) Game development uses Pygame, providing drawing, audio and other functions, which are suitable for creating 2D games. 2) GUI development can choose Tkinter or PyQt. Tkinter is simple and easy to use, PyQt has rich functions and is suitable for professional development.
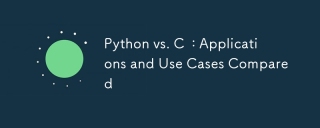
Python is suitable for data science, web development and automation tasks, while C is suitable for system programming, game development and embedded systems. Python is known for its simplicity and powerful ecosystem, while C is known for its high performance and underlying control capabilities.
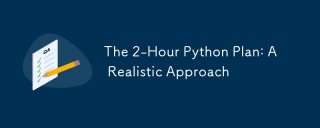
You can learn basic programming concepts and skills of Python within 2 hours. 1. Learn variables and data types, 2. Master control flow (conditional statements and loops), 3. Understand the definition and use of functions, 4. Quickly get started with Python programming through simple examples and code snippets.
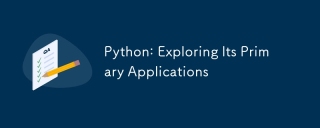
Python is widely used in the fields of web development, data science, machine learning, automation and scripting. 1) In web development, Django and Flask frameworks simplify the development process. 2) In the fields of data science and machine learning, NumPy, Pandas, Scikit-learn and TensorFlow libraries provide strong support. 3) In terms of automation and scripting, Python is suitable for tasks such as automated testing and system management.
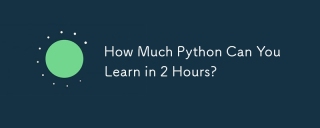
You can learn the basics of Python within two hours. 1. Learn variables and data types, 2. Master control structures such as if statements and loops, 3. Understand the definition and use of functions. These will help you start writing simple Python programs.
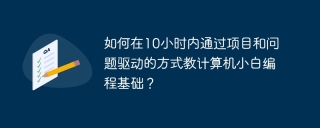
How to teach computer novice programming basics within 10 hours? If you only have 10 hours to teach computer novice some programming knowledge, what would you choose to teach...


Hot AI Tools

Undresser.AI Undress
AI-powered app for creating realistic nude photos

AI Clothes Remover
Online AI tool for removing clothes from photos.

Undress AI Tool
Undress images for free

Clothoff.io
AI clothes remover

AI Hentai Generator
Generate AI Hentai for free.

Hot Article

Hot Tools

DVWA
Damn Vulnerable Web App (DVWA) is a PHP/MySQL web application that is very vulnerable. Its main goals are to be an aid for security professionals to test their skills and tools in a legal environment, to help web developers better understand the process of securing web applications, and to help teachers/students teach/learn in a classroom environment Web application security. The goal of DVWA is to practice some of the most common web vulnerabilities through a simple and straightforward interface, with varying degrees of difficulty. Please note that this software
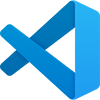
VSCode Windows 64-bit Download
A free and powerful IDE editor launched by Microsoft

SublimeText3 Linux new version
SublimeText3 Linux latest version

Atom editor mac version download
The most popular open source editor

SublimeText3 Chinese version
Chinese version, very easy to use