


Python problems encountered in concurrent programming and their solutions
Title: Python problems and solutions encountered in concurrent programming
Introduction:
In modern computer systems, the use of concurrent programming can give full play to multi-core processing improve the performance of the processor and improve the running efficiency of the program. As a widely used programming language, Python also has powerful concurrent programming capabilities. However, some problems are often encountered in concurrent programming. This article will introduce some common Python problems in concurrent programming and provide corresponding solutions, with specific code examples.
1. Global Interpreter Lock (GIL)
- Problem Overview:
In Python, the Global Interpreter Lock (Global Interpreter Lock, GIL for short) is a kind of Limitations of multi-threaded Python programs. GIL prevents concurrent programs from truly being executed in parallel on multi-core processors, thus affecting the performance of Python concurrent programs. - Solution:
(1) Use multi-process instead of multi-thread to achieve true parallel execution between multiple processes.
(2) Use tools such as Cython to bypass GIL restrictions by writing C extension modules.
Sample code:
import multiprocessing def compute(num): result = num * 2 return result if __name__ == '__main__': pool = multiprocessing.Pool() numbers = [1, 2, 3, 4, 5] results = pool.map(compute, numbers) print(results)
2. Thread safety
- Problem overview:
In a multi-threaded environment, multiple threads access the share at the same time Resources may cause thread safety issues such as data races, leading to program errors. - Solution:
(1) Use a mutex (Mutex) to ensure that only one thread can access shared resources at the same time.
(2) Use thread-safe data structures, such as the Queue queue in the threading module.
Sample code:
import threading import time class Counter: def __init__(self): self.value = 0 self.lock = threading.Lock() def increment(self): with self.lock: old_value = self.value time.sleep(1) # 模拟耗时操作 self.value = old_value + 1 if __name__ == '__main__': counter = Counter() threads = [] for _ in range(5): t = threading.Thread(target=counter.increment) threads.append(t) t.start() for t in threads: t.join() print(counter.value)
3. Concurrent data sharing
- Problem overview:
In a multi-threaded or multi-process program, the data Sharing is a very common requirement, but it also brings problems such as data consistency and race conditions. - Solution:
(1) Use thread-safe data structures, such as the Queue queue in the threading module to coordinate data sharing between different threads/processes.
(2) Use inter-process communication (IPC) mechanisms, such as queues, pipes, etc.
Sample code:
import multiprocessing def consumer(queue): while True: item = queue.get() if item == 'end': break print(f'consume {item}') def producer(queue): for i in range(5): print(f'produce {i}') queue.put(i) queue.put('end') if __name__ == '__main__': queue = multiprocessing.Queue() p1 = multiprocessing.Process(target=consumer, args=(queue,)) p2 = multiprocessing.Process(target=producer, args=(queue,)) p1.start() p2.start() p1.join() p2.join()
Conclusion:
This article provides corresponding solutions by analyzing common Python problems in concurrent programming, with specific code Example. Concurrent programming is an important means to improve the efficiency of program operation. Properly solving problems in concurrent programming will greatly improve the concurrency capabilities and performance of the program.
The above is the detailed content of Python problems encountered in concurrent programming and their solutions. For more information, please follow other related articles on the PHP Chinese website!
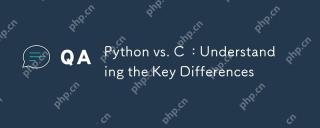
Python and C each have their own advantages, and the choice should be based on project requirements. 1) Python is suitable for rapid development and data processing due to its concise syntax and dynamic typing. 2)C is suitable for high performance and system programming due to its static typing and manual memory management.
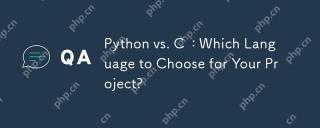
Choosing Python or C depends on project requirements: 1) If you need rapid development, data processing and prototype design, choose Python; 2) If you need high performance, low latency and close hardware control, choose C.
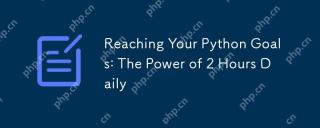
By investing 2 hours of Python learning every day, you can effectively improve your programming skills. 1. Learn new knowledge: read documents or watch tutorials. 2. Practice: Write code and complete exercises. 3. Review: Consolidate the content you have learned. 4. Project practice: Apply what you have learned in actual projects. Such a structured learning plan can help you systematically master Python and achieve career goals.
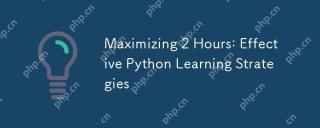
Methods to learn Python efficiently within two hours include: 1. Review the basic knowledge and ensure that you are familiar with Python installation and basic syntax; 2. Understand the core concepts of Python, such as variables, lists, functions, etc.; 3. Master basic and advanced usage by using examples; 4. Learn common errors and debugging techniques; 5. Apply performance optimization and best practices, such as using list comprehensions and following the PEP8 style guide.
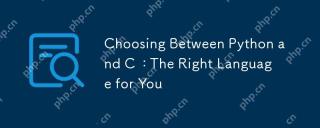
Python is suitable for beginners and data science, and C is suitable for system programming and game development. 1. Python is simple and easy to use, suitable for data science and web development. 2.C provides high performance and control, suitable for game development and system programming. The choice should be based on project needs and personal interests.
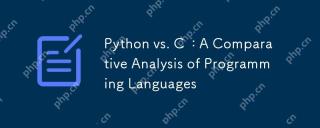
Python is more suitable for data science and rapid development, while C is more suitable for high performance and system programming. 1. Python syntax is concise and easy to learn, suitable for data processing and scientific computing. 2.C has complex syntax but excellent performance and is often used in game development and system programming.
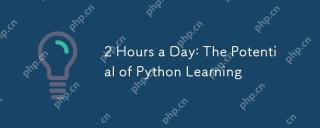
It is feasible to invest two hours a day to learn Python. 1. Learn new knowledge: Learn new concepts in one hour, such as lists and dictionaries. 2. Practice and exercises: Use one hour to perform programming exercises, such as writing small programs. Through reasonable planning and perseverance, you can master the core concepts of Python in a short time.
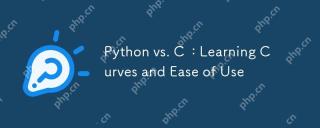
Python is easier to learn and use, while C is more powerful but complex. 1. Python syntax is concise and suitable for beginners. Dynamic typing and automatic memory management make it easy to use, but may cause runtime errors. 2.C provides low-level control and advanced features, suitable for high-performance applications, but has a high learning threshold and requires manual memory and type safety management.


Hot AI Tools

Undresser.AI Undress
AI-powered app for creating realistic nude photos

AI Clothes Remover
Online AI tool for removing clothes from photos.

Undress AI Tool
Undress images for free

Clothoff.io
AI clothes remover

Video Face Swap
Swap faces in any video effortlessly with our completely free AI face swap tool!

Hot Article

Hot Tools
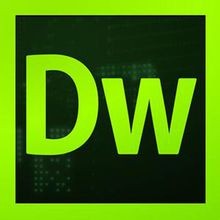
Dreamweaver CS6
Visual web development tools

SAP NetWeaver Server Adapter for Eclipse
Integrate Eclipse with SAP NetWeaver application server.

MantisBT
Mantis is an easy-to-deploy web-based defect tracking tool designed to aid in product defect tracking. It requires PHP, MySQL and a web server. Check out our demo and hosting services.

Zend Studio 13.0.1
Powerful PHP integrated development environment

PhpStorm Mac version
The latest (2018.2.1) professional PHP integrated development tool