Sentiment level recognition problem in sentiment analysis
Sentiment level recognition problem in sentiment analysis requires specific code examples
Sentiment analysis is an important task in natural language processing, which aims to analyze text by computer Perform emotion classification and emotion level recognition. Emotion level recognition is an important part of sentiment analysis, which can help us understand the emotional information in text more accurately. This article will introduce the problem of emotion level recognition and provide some concrete code examples.
Emotion level recognition can divide the emotion of text into multiple levels, such as negative, neutral and positive. By identifying emotion levels in text, we can better understand people's emotional attitudes toward a topic or event.
When performing emotional level recognition, we can use machine learning methods. The following is a python-based example of code example for emotion level recognition using Naive Bayes classifier:
# 导入必要的库 import pandas as pd from sklearn.feature_extraction.text import CountVectorizer from sklearn.naive_bayes import MultinomialNB from sklearn.model_selection import train_test_split # 加载数据集 data = pd.read_csv('data.csv') # 划分特征和目标变量 X = data['text'] y = data['label'] # 文本向量化 vectorizer = CountVectorizer() X = vectorizer.fit_transform(X) # 划分训练集和测试集 X_train, X_test, y_train, y_test = train_test_split(X, y, test_size=0.2) # 创建并训练朴素贝叶斯分类器 classifier = MultinomialNB() classifier.fit(X_train, y_train) # 评估分类器性能 accuracy = classifier.score(X_test, y_test) print("Accuracy:", accuracy)
In this example, we first imported the necessary libraries and then loaded the files containing text and labels of data sets. Next, we use CountVectorizer
to convert the text into a document-term frequency matrix for use as input to the machine learning model. Then, we divide the data set into training set and test set. Finally, we created a Naive Bayes classifier, trained and evaluated it using the training data.
Of course, this is just a simple example, and actual emotion-level recognition problems may require more complex algorithms and feature engineering. In addition, other methods such as support vector machines, deep learning, etc. can also be used to solve the emotion level recognition problem.
To summarize, emotion level recognition is an important task in sentiment analysis, which can help us more accurately identify emotional information in text. Through machine learning algorithms, we are able to build models for emotion-level recognition and obtain valuable information from them. I hope the sample code provided in this article will be helpful to readers.
The above is the detailed content of Sentiment level recognition problem in sentiment analysis. For more information, please follow other related articles on the PHP Chinese website!

Introduction Suppose there is a farmer who daily observes the progress of crops in several weeks. He looks at the growth rates and begins to ponder about how much more taller his plants could grow in another few weeks. From th
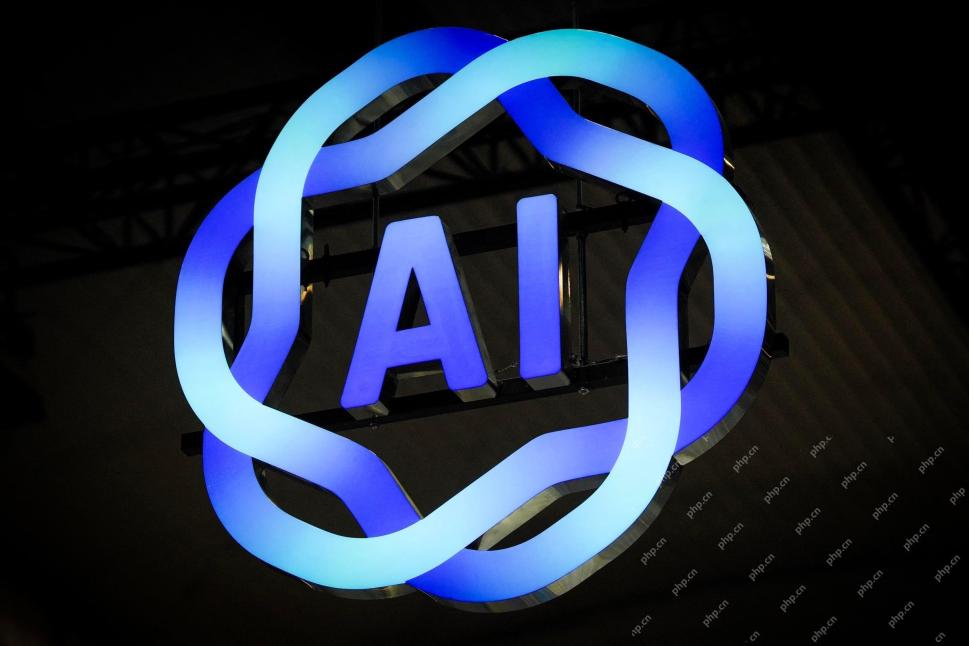
Soft AI — defined as AI systems designed to perform specific, narrow tasks using approximate reasoning, pattern recognition, and flexible decision-making — seeks to mimic human-like thinking by embracing ambiguity. But what does this mean for busine
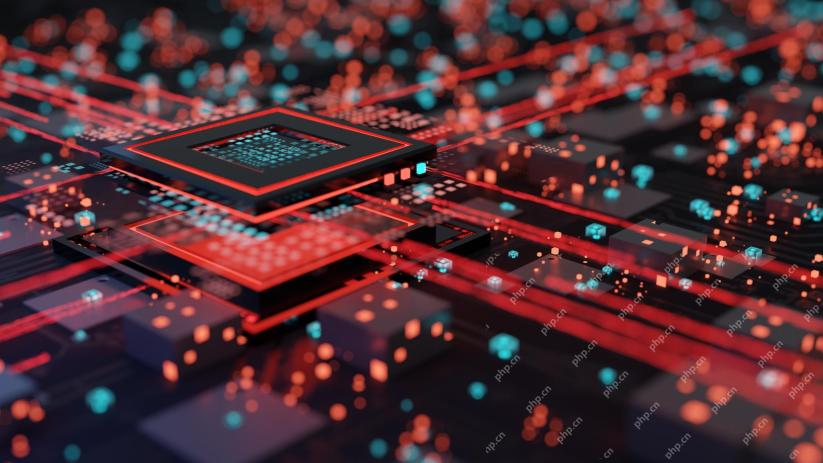
The answer is clear—just as cloud computing required a shift toward cloud-native security tools, AI demands a new breed of security solutions designed specifically for AI's unique needs. The Rise of Cloud Computing and Security Lessons Learned In th

Entrepreneurs and using AI and Generative AI to make their businesses better. At the same time, it is important to remember generative AI, like all technologies, is an amplifier – making the good great and the mediocre, worse. A rigorous 2024 study o
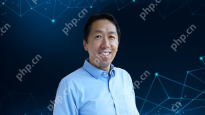
Unlock the Power of Embedding Models: A Deep Dive into Andrew Ng's New Course Imagine a future where machines understand and respond to your questions with perfect accuracy. This isn't science fiction; thanks to advancements in AI, it's becoming a r
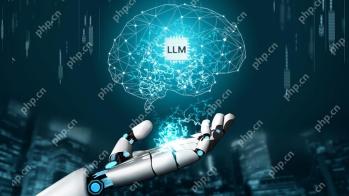
Large Language Models (LLMs) and the Inevitable Problem of Hallucinations You've likely used AI models like ChatGPT, Claude, and Gemini. These are all examples of Large Language Models (LLMs), powerful AI systems trained on massive text datasets to
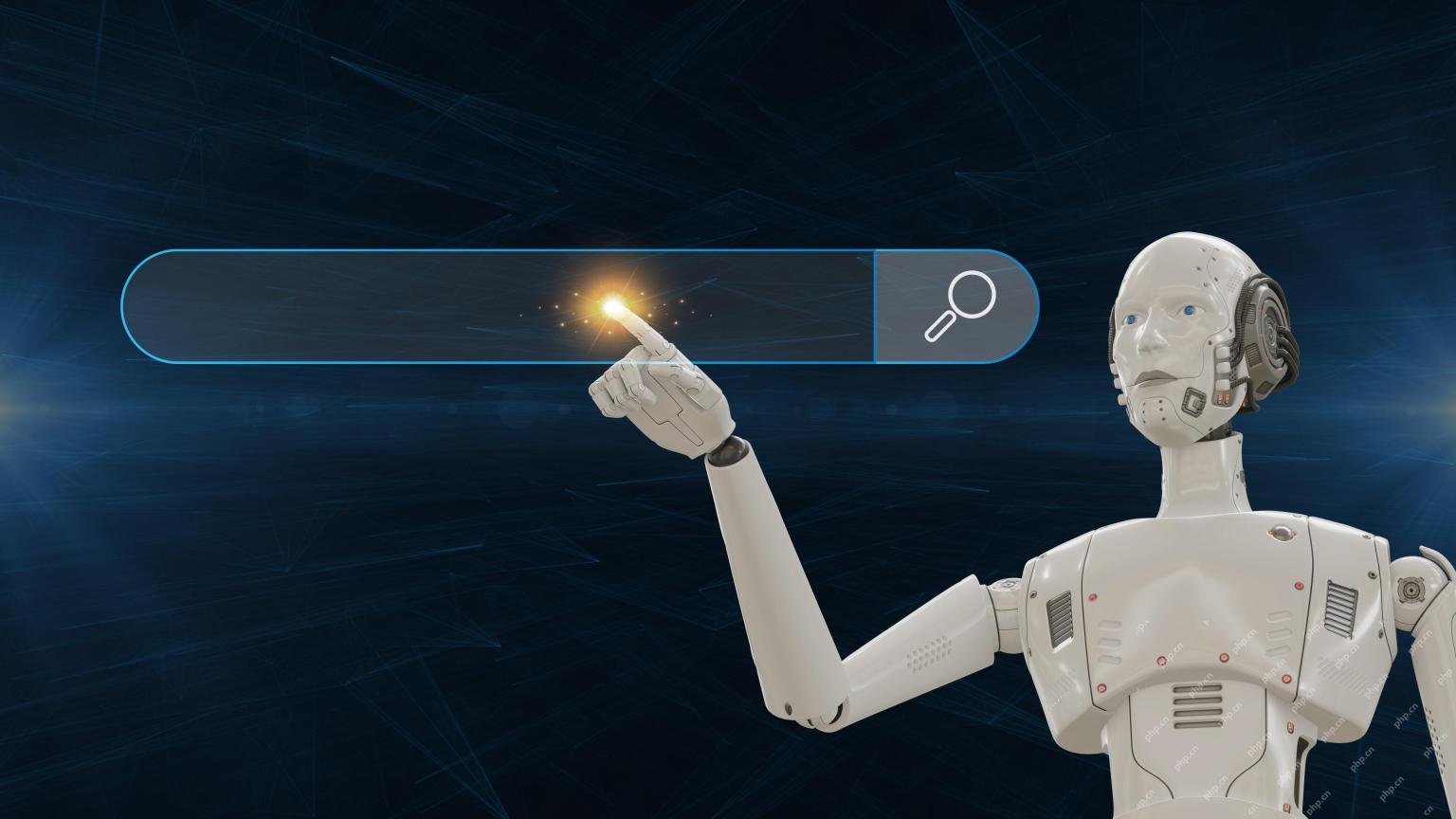
Recent research has shown that AI Overviews can cause a whopping 15-64% decline in organic traffic, based on industry and search type. This radical change is causing marketers to reconsider their whole strategy regarding digital visibility. The New
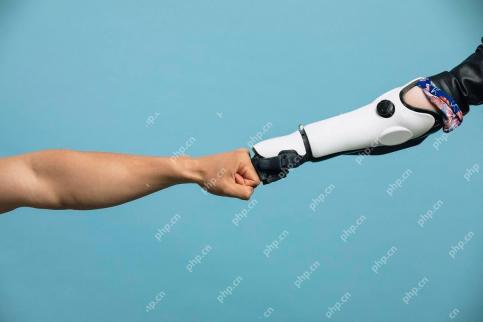
A recent report from Elon University’s Imagining The Digital Future Center surveyed nearly 300 global technology experts. The resulting report, ‘Being Human in 2035’, concluded that most are concerned that the deepening adoption of AI systems over t


Hot AI Tools

Undresser.AI Undress
AI-powered app for creating realistic nude photos

AI Clothes Remover
Online AI tool for removing clothes from photos.

Undress AI Tool
Undress images for free

Clothoff.io
AI clothes remover

AI Hentai Generator
Generate AI Hentai for free.

Hot Article

Hot Tools

MantisBT
Mantis is an easy-to-deploy web-based defect tracking tool designed to aid in product defect tracking. It requires PHP, MySQL and a web server. Check out our demo and hosting services.
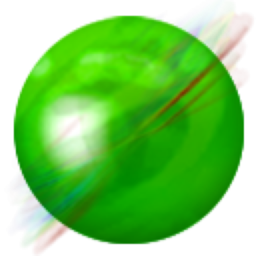
ZendStudio 13.5.1 Mac
Powerful PHP integrated development environment
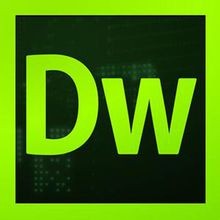
Dreamweaver CS6
Visual web development tools

SublimeText3 English version
Recommended: Win version, supports code prompts!

SublimeText3 Linux new version
SublimeText3 Linux latest version