Expression recognition issues in face recognition technology
The expression recognition problem in face recognition technology requires specific code examples
In recent years, face recognition technology has made important breakthroughs in various fields and has become an artificial One of the important branches in intelligent technology. Facial recognition technology has been widely used in security monitoring, facial payment, smart access control and other fields. However, although face recognition technology is quite mature, the expression recognition problem is still challenging.
Expression recognition refers to determining a person’s emotional state by analyzing the expression features on a person’s face. In daily life, people's expressions can convey a wealth of information, such as joy, anger, sorrow, joy, surprise, etc. Therefore, accurate recognition of expressions is of great significance to the application of face recognition technology.
In traditional face recognition technology, facial feature extraction is usually based on geometric features of the face, such as face outline, eye position, mouth position, etc. However, the extraction of these geometric features cannot directly reflect the human expression state, because expressions are generated by muscle movements. Therefore, traditional face recognition technology faces the challenge of expression recognition.
Fortunately, with the development of deep learning technology, expression recognition technology has made significant progress. Deep learning models can better capture the features of expressions by learning a large number of facial expression samples. Commonly used deep learning models include Convolutional Neural Network (CNN), Recurrent Neural Network (RNN), etc.
The following takes the use of convolutional neural networks to achieve expression recognition as an example to introduce a common method. First, we need to collect a batch of face image data with labeled expressions. These data can include facial expression images of different people, including different emotional states such as joy, anger, sadness, joy, and surprise. Then, we divide this batch of image data into a training set and a test set according to a certain proportion.
In terms of model construction, we can use multiple convolutional layers and pooling layers to extract features in the image. The convolutional layer extracts features from the image through sliding windows and a series of filters, while the pooling layer is used to reduce the image size and improve the efficiency of the model. Finally, we can use the fully connected layer to associate the features extracted by the convolutional layer with the actual expression, and perform training and optimization.
The following is a simple example code for expression recognition based on convolutional neural network:
import tensorflow as tf from tensorflow.keras import layers # 定义卷积神经网络模型 model = tf.keras.Sequential([ layers.Conv2D(32, (3, 3), activation='relu', input_shape=(48, 48, 1)), layers.MaxPooling2D((2, 2)), layers.Conv2D(64, (3, 3), activation='relu'), layers.MaxPooling2D((2, 2)), layers.Conv2D(64, (3, 3), activation='relu'), layers.Flatten(), layers.Dense(64, activation='relu'), layers.Dense(7, activation='softmax') ]) # 编译模型 model.compile(optimizer='adam', loss=tf.keras.losses.SparseCategoricalCrossentropy(from_logits=True), metrics=['accuracy']) # 加载数据集 # 这里假设你已经有了一个已经标注好的表情识别数据集 # 划分训练集和测试集 # 这里假设你已经将数据集分为了训练集和测试集 # 进行模型训练 model.fit(train_images, train_labels, epochs=10, validation_data=(test_images, test_labels)) # 进行预测 predictions = model.predict(test_images) # 输出预测结果 # 这里可以根据实际需要进行处理和输出
In the above code example, we use a simple convolutional neural network model for expression recognition . First, we define the structure of the model, including convolutional layers, pooling layers, and fully connected layers. We then compile the model and use the dataset for training and testing. Finally, we use the trained model to predict expression recognition.
It should be noted that the above code example is only a simple implementation of expression recognition. In actual applications, further processing and optimization of data may be required. In addition, there are other more complex and advanced models and algorithms in the field of expression recognition, such as using recurrent neural networks (RNN) for sequence modeling.
In short, the expression recognition problem in face recognition technology is a challenging task. Through the application of deep learning technology, especially the convolutional neural network model, we can better capture the characteristics of human facial expressions and achieve accurate expression recognition. Through the above code examples, we can further learn and apply technologies related to expression recognition.
The above is the detailed content of Expression recognition issues in face recognition technology. For more information, please follow other related articles on the PHP Chinese website!

Introduction Suppose there is a farmer who daily observes the progress of crops in several weeks. He looks at the growth rates and begins to ponder about how much more taller his plants could grow in another few weeks. From th
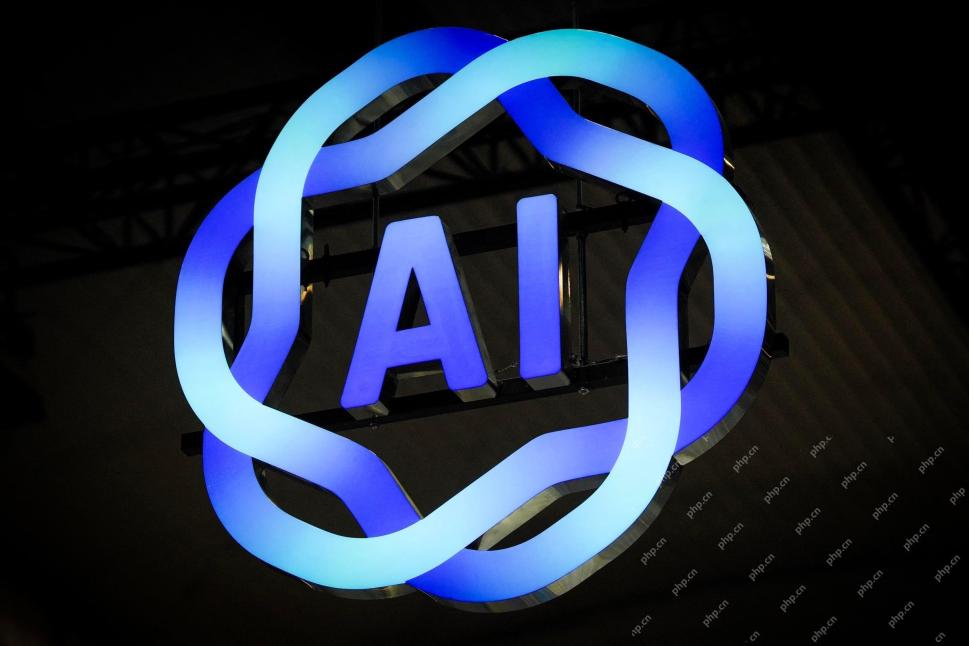
Soft AI — defined as AI systems designed to perform specific, narrow tasks using approximate reasoning, pattern recognition, and flexible decision-making — seeks to mimic human-like thinking by embracing ambiguity. But what does this mean for busine
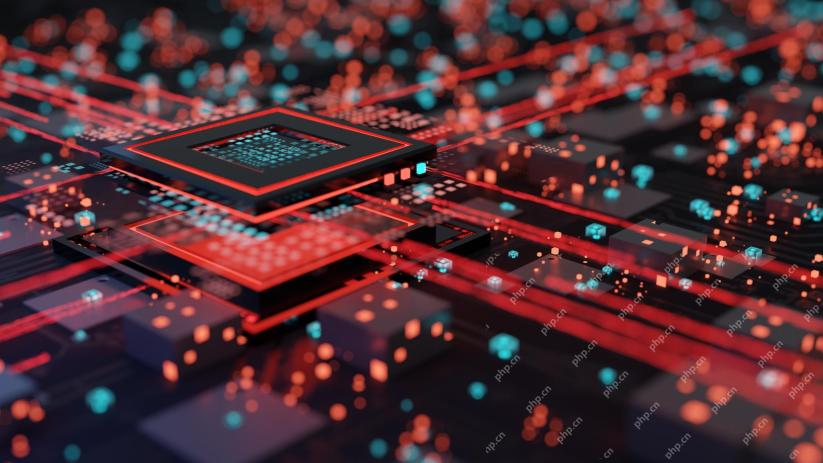
The answer is clear—just as cloud computing required a shift toward cloud-native security tools, AI demands a new breed of security solutions designed specifically for AI's unique needs. The Rise of Cloud Computing and Security Lessons Learned In th

Entrepreneurs and using AI and Generative AI to make their businesses better. At the same time, it is important to remember generative AI, like all technologies, is an amplifier – making the good great and the mediocre, worse. A rigorous 2024 study o
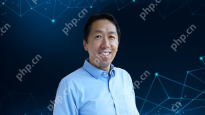
Unlock the Power of Embedding Models: A Deep Dive into Andrew Ng's New Course Imagine a future where machines understand and respond to your questions with perfect accuracy. This isn't science fiction; thanks to advancements in AI, it's becoming a r
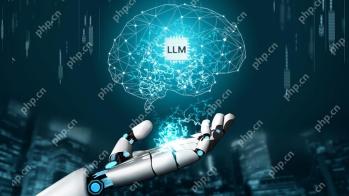
Large Language Models (LLMs) and the Inevitable Problem of Hallucinations You've likely used AI models like ChatGPT, Claude, and Gemini. These are all examples of Large Language Models (LLMs), powerful AI systems trained on massive text datasets to
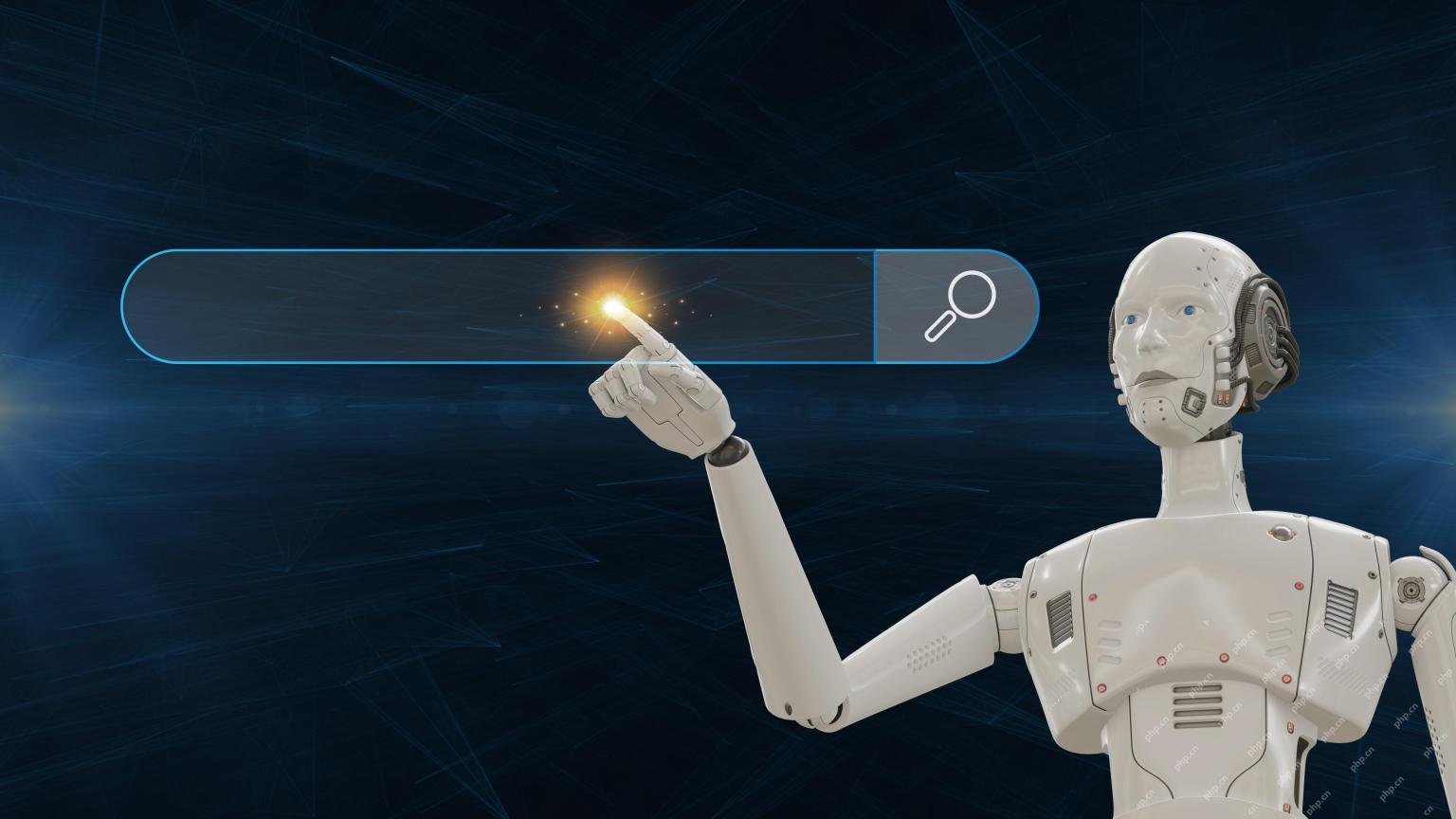
Recent research has shown that AI Overviews can cause a whopping 15-64% decline in organic traffic, based on industry and search type. This radical change is causing marketers to reconsider their whole strategy regarding digital visibility. The New
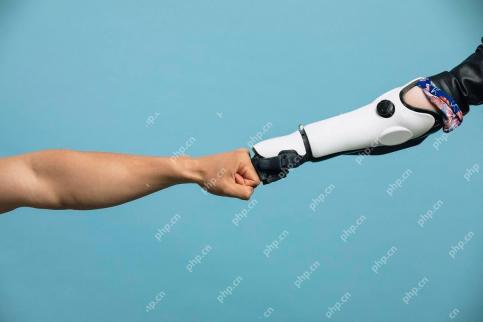
A recent report from Elon University’s Imagining The Digital Future Center surveyed nearly 300 global technology experts. The resulting report, ‘Being Human in 2035’, concluded that most are concerned that the deepening adoption of AI systems over t


Hot AI Tools

Undresser.AI Undress
AI-powered app for creating realistic nude photos

AI Clothes Remover
Online AI tool for removing clothes from photos.

Undress AI Tool
Undress images for free

Clothoff.io
AI clothes remover

AI Hentai Generator
Generate AI Hentai for free.

Hot Article

Hot Tools

DVWA
Damn Vulnerable Web App (DVWA) is a PHP/MySQL web application that is very vulnerable. Its main goals are to be an aid for security professionals to test their skills and tools in a legal environment, to help web developers better understand the process of securing web applications, and to help teachers/students teach/learn in a classroom environment Web application security. The goal of DVWA is to practice some of the most common web vulnerabilities through a simple and straightforward interface, with varying degrees of difficulty. Please note that this software

Notepad++7.3.1
Easy-to-use and free code editor

Safe Exam Browser
Safe Exam Browser is a secure browser environment for taking online exams securely. This software turns any computer into a secure workstation. It controls access to any utility and prevents students from using unauthorized resources.

mPDF
mPDF is a PHP library that can generate PDF files from UTF-8 encoded HTML. The original author, Ian Back, wrote mPDF to output PDF files "on the fly" from his website and handle different languages. It is slower than original scripts like HTML2FPDF and produces larger files when using Unicode fonts, but supports CSS styles etc. and has a lot of enhancements. Supports almost all languages, including RTL (Arabic and Hebrew) and CJK (Chinese, Japanese and Korean). Supports nested block-level elements (such as P, DIV),

MantisBT
Mantis is an easy-to-deploy web-based defect tracking tool designed to aid in product defect tracking. It requires PHP, MySQL and a web server. Check out our demo and hosting services.