


How to use PHP microservices to implement distributed machine learning and intelligent recommendations
How to use PHP microservices to implement distributed machine learning and intelligent recommendations
Overview:
With the rapid development of the Internet, the explosive growth of data volume has made traditional The machine learning algorithm cannot meet the needs of big data analysis and intelligent recommendation. To address this challenge, distributed machine learning and intelligent recommendation technologies emerged. This article will introduce how to use PHP microservices to implement distributed machine learning and intelligent recommendations, and provide relevant code examples.
- System architecture design
When designing distributed machine learning and intelligent recommendation systems, the following aspects need to be considered: - Data storage: use a distributed storage system (such as Hadoop, Cassandra, etc.) to store massive data.
- Data preprocessing: Use a distributed computing framework (such as Spark) to preprocess data, such as data cleaning, feature extraction, etc.
- Model training: Use distributed machine learning algorithms (such as TensorFlow, XGBoost, etc.) to train the preprocessed data and generate models.
- Model inference: Use a distributed computing framework to deploy models to multiple servers to achieve intelligent recommendations.
- Use PHP microservices to implement distributed machine learning and intelligent recommendations
Since the PHP language is widely used in web development, using PHP microservices to implement distributed machine learning and intelligent recommendations has high flexibility and scalability.
2.1 Data Storage
In PHP microservices, NoSQL databases (such as MongoDB) can be used as distributed storage systems to store massive data. The following is a sample code for using MongoDB to store data:
<?php // 连接MongoDB $mongo = new MongoDBClient("mongodb://localhost:27017"); // 选择数据库 $db = $mongo->mydb; // 选择集合 $collection = $db->mycollection; // 插入数据 $data = array("name" => "John", "age" => 25); $collection->insertOne($data); // 查询数据 $result = $collection->findOne(array("name" => "John")); print_r($result); ?>
2.2 Data preprocessing
Data preprocessing is a very critical step in machine learning. You can use PHP microservices and distributed computing frameworks (such as Apache Spark ) are combined to achieve this. The following is a sample code for data preprocessing using Spark:
<?php // 创建SparkSession $spark = SparkSparkSession::builder() ->appName("Data Preprocessing") ->getOrCreate(); // 读取数据 $data = $spark->read()->format("csv") ->option("header", "true") ->load("data.csv"); // 数据清洗 $data = $data->filter($data["age"] > 18); // 特征提取 $vectorAssembler = new SparkFeatureVectorAssembler(); $vectorAssembler->setInputCols(["age"]) ->setOutputCol("features"); $data = $vectorAssembler->transform($data); // 打印数据 $data->show(); ?>
2.3 Model training
Model training is the core part of distributed machine learning, which can use PHP microservices and distributed machine learning frameworks (such as TensorFlow , XGBoost, etc.). The following is a sample code for model training using TensorFlow:
<?php // 加载TensorFlow库 require_once "tensorflow.php"; // 创建TensorFlow会话 $session = new TensorFlowSession(); // 定义模型 $input = new TensorFlowTensor(TensorFlowDataType::FLOAT, [2, 2]); $const = TensorFlowMath::add($input, TensorFlowMath::scalar(TensorFlowDataType::FLOAT, 2.0)); $output = $session->run([$const], [$input->initWithValue([[1.0, 2.0], [3.0, 4.0]])]); // 打印结果 print_r($output); ?>
2.4 Model Inference
Model inference is the core part of intelligent recommendation. You can use PHP microservices and distributed computing framework to deploy the model and make recommendations The results are returned to the client. The following is a sample code for model inference using PHP microservices:
<?php // 加载模型 $model = new MyModel(); // 接收客户端请求 $input = $_POST["input"]; // 调用模型推断 $output = $model->predict($input); // 返回推荐结果给客户端 echo $output; ?>
Summary:
This article introduces how to use PHP microservices to implement distributed machine learning and intelligent recommendations. By combining distributed storage systems, distributed computing frameworks, and distributed machine learning algorithms, big data can be effectively processed and intelligent recommendations can be achieved. Through the demonstration of sample code, readers can further understand and practice related technologies, and explore the application prospects of PHP in the field of big data.
The above is the detailed content of How to use PHP microservices to implement distributed machine learning and intelligent recommendations. For more information, please follow other related articles on the PHP Chinese website!
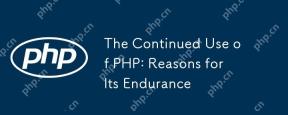
What’s still popular is the ease of use, flexibility and a strong ecosystem. 1) Ease of use and simple syntax make it the first choice for beginners. 2) Closely integrated with web development, excellent interaction with HTTP requests and database. 3) The huge ecosystem provides a wealth of tools and libraries. 4) Active community and open source nature adapts them to new needs and technology trends.
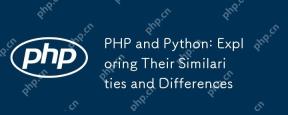
PHP and Python are both high-level programming languages that are widely used in web development, data processing and automation tasks. 1.PHP is often used to build dynamic websites and content management systems, while Python is often used to build web frameworks and data science. 2.PHP uses echo to output content, Python uses print. 3. Both support object-oriented programming, but the syntax and keywords are different. 4. PHP supports weak type conversion, while Python is more stringent. 5. PHP performance optimization includes using OPcache and asynchronous programming, while Python uses cProfile and asynchronous programming.
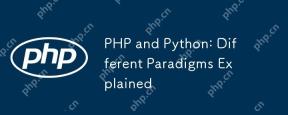
PHP is mainly procedural programming, but also supports object-oriented programming (OOP); Python supports a variety of paradigms, including OOP, functional and procedural programming. PHP is suitable for web development, and Python is suitable for a variety of applications such as data analysis and machine learning.
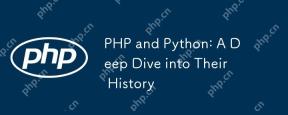
PHP originated in 1994 and was developed by RasmusLerdorf. It was originally used to track website visitors and gradually evolved into a server-side scripting language and was widely used in web development. Python was developed by Guidovan Rossum in the late 1980s and was first released in 1991. It emphasizes code readability and simplicity, and is suitable for scientific computing, data analysis and other fields.
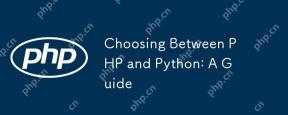
PHP is suitable for web development and rapid prototyping, and Python is suitable for data science and machine learning. 1.PHP is used for dynamic web development, with simple syntax and suitable for rapid development. 2. Python has concise syntax, is suitable for multiple fields, and has a strong library ecosystem.
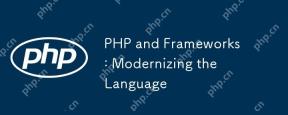
PHP remains important in the modernization process because it supports a large number of websites and applications and adapts to development needs through frameworks. 1.PHP7 improves performance and introduces new features. 2. Modern frameworks such as Laravel, Symfony and CodeIgniter simplify development and improve code quality. 3. Performance optimization and best practices further improve application efficiency.
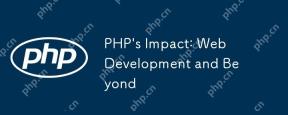
PHPhassignificantlyimpactedwebdevelopmentandextendsbeyondit.1)ItpowersmajorplatformslikeWordPressandexcelsindatabaseinteractions.2)PHP'sadaptabilityallowsittoscaleforlargeapplicationsusingframeworkslikeLaravel.3)Beyondweb,PHPisusedincommand-linescrip
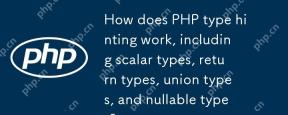
PHP type prompts to improve code quality and readability. 1) Scalar type tips: Since PHP7.0, basic data types are allowed to be specified in function parameters, such as int, float, etc. 2) Return type prompt: Ensure the consistency of the function return value type. 3) Union type prompt: Since PHP8.0, multiple types are allowed to be specified in function parameters or return values. 4) Nullable type prompt: Allows to include null values and handle functions that may return null values.


Hot AI Tools

Undresser.AI Undress
AI-powered app for creating realistic nude photos

AI Clothes Remover
Online AI tool for removing clothes from photos.

Undress AI Tool
Undress images for free

Clothoff.io
AI clothes remover

Video Face Swap
Swap faces in any video effortlessly with our completely free AI face swap tool!

Hot Article

Hot Tools
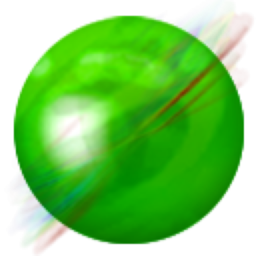
ZendStudio 13.5.1 Mac
Powerful PHP integrated development environment

mPDF
mPDF is a PHP library that can generate PDF files from UTF-8 encoded HTML. The original author, Ian Back, wrote mPDF to output PDF files "on the fly" from his website and handle different languages. It is slower than original scripts like HTML2FPDF and produces larger files when using Unicode fonts, but supports CSS styles etc. and has a lot of enhancements. Supports almost all languages, including RTL (Arabic and Hebrew) and CJK (Chinese, Japanese and Korean). Supports nested block-level elements (such as P, DIV),

MinGW - Minimalist GNU for Windows
This project is in the process of being migrated to osdn.net/projects/mingw, you can continue to follow us there. MinGW: A native Windows port of the GNU Compiler Collection (GCC), freely distributable import libraries and header files for building native Windows applications; includes extensions to the MSVC runtime to support C99 functionality. All MinGW software can run on 64-bit Windows platforms.
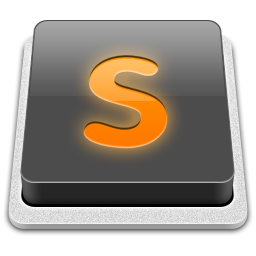
SublimeText3 Mac version
God-level code editing software (SublimeText3)
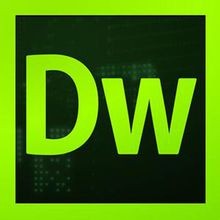
Dreamweaver CS6
Visual web development tools