How to implement genetic algorithm in C
#Introduction:
Genetic algorithm is an optimization algorithm that simulates natural selection and genetic inheritance mechanisms. Its main idea is Search for optimal solutions by simulating the process of biological evolution. In the field of computer science, genetic algorithms are widely used to solve optimization problems, such as machine learning, parameter optimization, combinatorial optimization, etc. This article will introduce how to implement a genetic algorithm in C# and provide specific code examples.
1. Basic Principles of Genetic Algorithm
Genetic algorithm uses coding to represent candidate solutions in the solution space, and uses operations such as selection, crossover and mutation to optimize the current solution. The basic process of the genetic algorithm is as follows:
- Initialize the population: Generate a certain number of candidate solutions, called a population.
- Fitness calculation: Calculate the fitness of each individual according to the requirements of the problem.
- Selection operation: Select some better individuals as parents based on fitness.
- Crossover operation: Produce some offspring individuals through crossover operation.
- Mutation operation: perform mutation operation on some offspring individuals.
- Update population: merge parent and offspring individuals to update the population.
- Judge the stop conditions: According to actual needs, judge whether the stop conditions are met, otherwise return to step 3.
2. Steps to implement genetic algorithm in C
- # Define the encoding method of the solution: According to the characteristics of the problem, define the encoding method of the solution, which can be binary, real number, Integer etc.
For example, suppose you want to solve an optimal value problem of integer encoding. The encoding method of the solution can be represented by an integer array.
class Solution { public int[] Genes { get; set; } // 解的编码方式,用整数数组表示 public double Fitness { get; set; } // 适应度 }
- Initialize the population: Generate a certain number of random solutions as the initial population.
List<Solution> population = new List<Solution>(); Random random = new Random(); for (int i = 0; i < populationSize; i++) { Solution solution = new Solution(); solution.Genes = new int[chromosomeLength]; for (int j = 0; j < chromosomeLength; j++) { solution.Genes[j] = random.Next(minGeneValue, maxGeneValue + 1); } population.Add(solution); }
- Fitness calculation: Calculate the fitness of each individual according to the requirements of the problem.
void CalculateFitness(List<Solution> population) { // 根据问题的要求,计算每个个体的适应度,并更新Fitness属性 // ... }
- Selection operation: select some better individuals as parents based on fitness.
Common selection operations include roulette selection, elimination method selection, competition method selection, etc.
List<Solution> Select(List<Solution> population, int selectedPopulationSize) { List<Solution> selectedPopulation = new List<Solution>(); // 根据适应度选择一部分较好的个体,并将其加入selectedPopulation中 // ... return selectedPopulation; }
- Crossover operation: Produce a portion of offspring individuals through crossover operation.
Common crossover operations include single-point crossover, multi-point crossover, uniform crossover, etc.
List<Solution> Crossover(List<Solution> selectedPopulation, int offspringPopulationSize) { List<Solution> offspringPopulation = new List<Solution>(); // 通过交叉操作产生一部分后代个体,并将其加入offspringPopulation中 // ... return offspringPopulation; }
- Mutation operation: perform mutation operation on some offspring individuals.
Common mutation operations include bitwise mutation, non-uniform mutation, polynomial mutation, etc.
void Mutation(List<Solution> offspringPopulation) { // 对一部分后代个体进行变异操作 // ... }
- Update population: merge parent and offspring individuals to update the population.
List<Solution> UpdatePopulation(List<Solution> population, List<Solution> offspringPopulation) { List<Solution> newPopulation = new List<Solution>(); // 将父代和后代个体合并更新种群,并选择适应度较好的个体加入newPopulation中 // ... return newPopulation; }
- Judgment of stop conditions: Based on actual needs, determine whether the stop conditions are met.
For example, you can set the algorithm to stop when the number of iterations reaches the upper limit or the fitness reaches a certain threshold.
3. Summary
This article introduces the basic steps of implementing genetic algorithms in C# and provides corresponding code examples. As an optimization algorithm, genetic algorithm is widely used in the field of computer science to search for optimal solutions by simulating the process of biological evolution. I hope this article will be helpful to readers in understanding and applying genetic algorithms.
The above is the detailed content of How to implement genetic algorithm in C#. For more information, please follow other related articles on the PHP Chinese website!
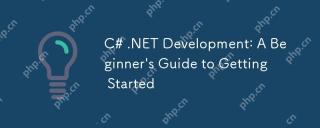
To start C#.NET development, you need to: 1. Understand the basic knowledge of C# and the core concepts of the .NET framework; 2. Master the basic concepts of variables, data types, control structures, functions and classes; 3. Learn advanced features of C#, such as LINQ and asynchronous programming; 4. Be familiar with debugging techniques and performance optimization methods for common errors. With these steps, you can gradually penetrate the world of C#.NET and write efficient applications.
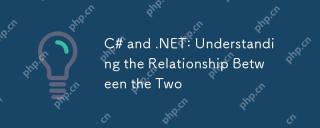
The relationship between C# and .NET is inseparable, but they are not the same thing. C# is a programming language, while .NET is a development platform. C# is used to write code, compile into .NET's intermediate language (IL), and executed by the .NET runtime (CLR).
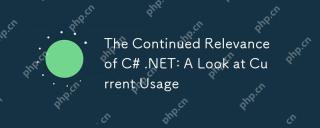
C#.NET is still important because it provides powerful tools and libraries that support multiple application development. 1) C# combines .NET framework to make development efficient and convenient. 2) C#'s type safety and garbage collection mechanism enhance its advantages. 3) .NET provides a cross-platform running environment and rich APIs, improving development flexibility.
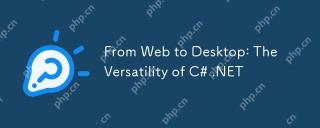
C#.NETisversatileforbothwebanddesktopdevelopment.1)Forweb,useASP.NETfordynamicapplications.2)Fordesktop,employWindowsFormsorWPFforrichinterfaces.3)UseXamarinforcross-platformdevelopment,enablingcodesharingacrossWindows,macOS,Linux,andmobiledevices.
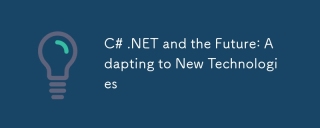
C# and .NET adapt to the needs of emerging technologies through continuous updates and optimizations. 1) C# 9.0 and .NET5 introduce record type and performance optimization. 2) .NETCore enhances cloud native and containerized support. 3) ASP.NETCore integrates with modern web technologies. 4) ML.NET supports machine learning and artificial intelligence. 5) Asynchronous programming and best practices improve performance.
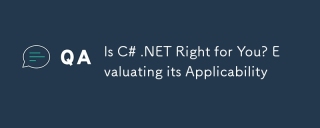
C#.NETissuitableforenterprise-levelapplicationswithintheMicrosoftecosystemduetoitsstrongtyping,richlibraries,androbustperformance.However,itmaynotbeidealforcross-platformdevelopmentorwhenrawspeediscritical,wherelanguageslikeRustorGomightbepreferable.
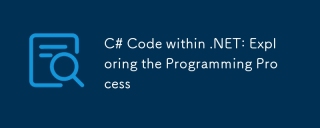
The programming process of C# in .NET includes the following steps: 1) writing C# code, 2) compiling into an intermediate language (IL), and 3) executing by the .NET runtime (CLR). The advantages of C# in .NET are its modern syntax, powerful type system and tight integration with the .NET framework, suitable for various development scenarios from desktop applications to web services.
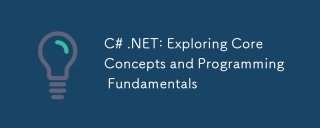
C# is a modern, object-oriented programming language developed by Microsoft and as part of the .NET framework. 1.C# supports object-oriented programming (OOP), including encapsulation, inheritance and polymorphism. 2. Asynchronous programming in C# is implemented through async and await keywords to improve application responsiveness. 3. Use LINQ to process data collections concisely. 4. Common errors include null reference exceptions and index out-of-range exceptions. Debugging skills include using a debugger and exception handling. 5. Performance optimization includes using StringBuilder and avoiding unnecessary packing and unboxing.


Hot AI Tools

Undresser.AI Undress
AI-powered app for creating realistic nude photos

AI Clothes Remover
Online AI tool for removing clothes from photos.

Undress AI Tool
Undress images for free

Clothoff.io
AI clothes remover

AI Hentai Generator
Generate AI Hentai for free.

Hot Article

Hot Tools

Safe Exam Browser
Safe Exam Browser is a secure browser environment for taking online exams securely. This software turns any computer into a secure workstation. It controls access to any utility and prevents students from using unauthorized resources.
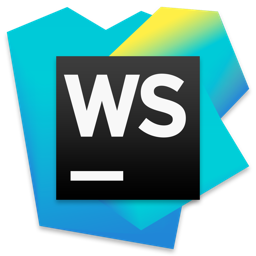
WebStorm Mac version
Useful JavaScript development tools

SAP NetWeaver Server Adapter for Eclipse
Integrate Eclipse with SAP NetWeaver application server.

MinGW - Minimalist GNU for Windows
This project is in the process of being migrated to osdn.net/projects/mingw, you can continue to follow us there. MinGW: A native Windows port of the GNU Compiler Collection (GCC), freely distributable import libraries and header files for building native Windows applications; includes extensions to the MSVC runtime to support C99 functionality. All MinGW software can run on 64-bit Windows platforms.

Atom editor mac version download
The most popular open source editor