The rise of artificial intelligence (AI) and machine learning (Machine Learning) is profoundly changing all walks of life. As the amount of data continues to increase and computing power improves, using AI and machine learning to make intelligent decisions has become one of the key strategies for enterprises and organizations. In order to effectively apply AI and machine learning, it is crucial to establish a complete integrated architecture
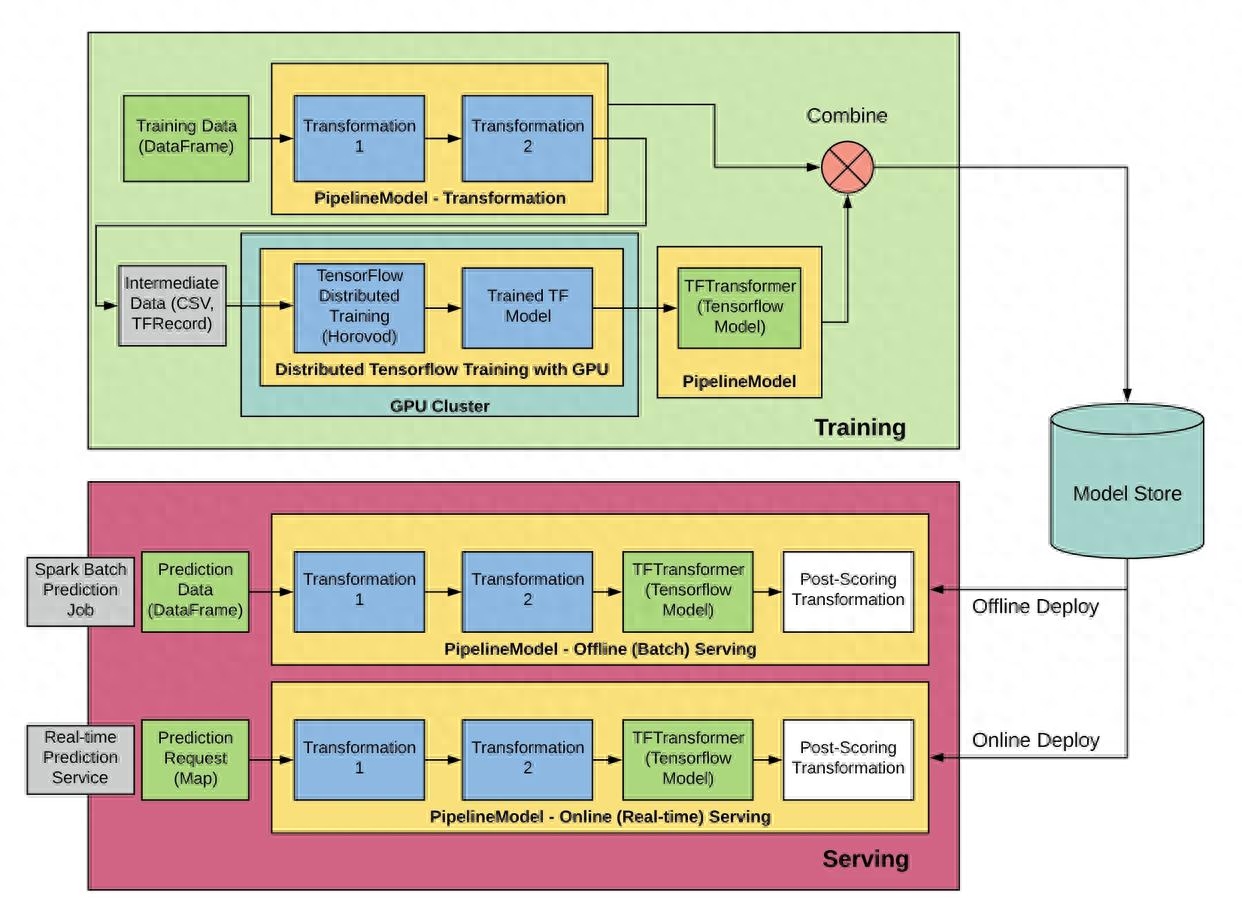
The significance of AI and machine learning integration
AI and machine learning can analyze huge data sets, discover patterns, predict trends, and make automated decisions. This is of great help in improving business efficiency, optimizing resource allocation, and discovering business opportunities. However, for AI and machine learning to truly work, a reasonable integration architecture is needed to ensure seamless connection between data flow, model training and deployment, and decision execution.
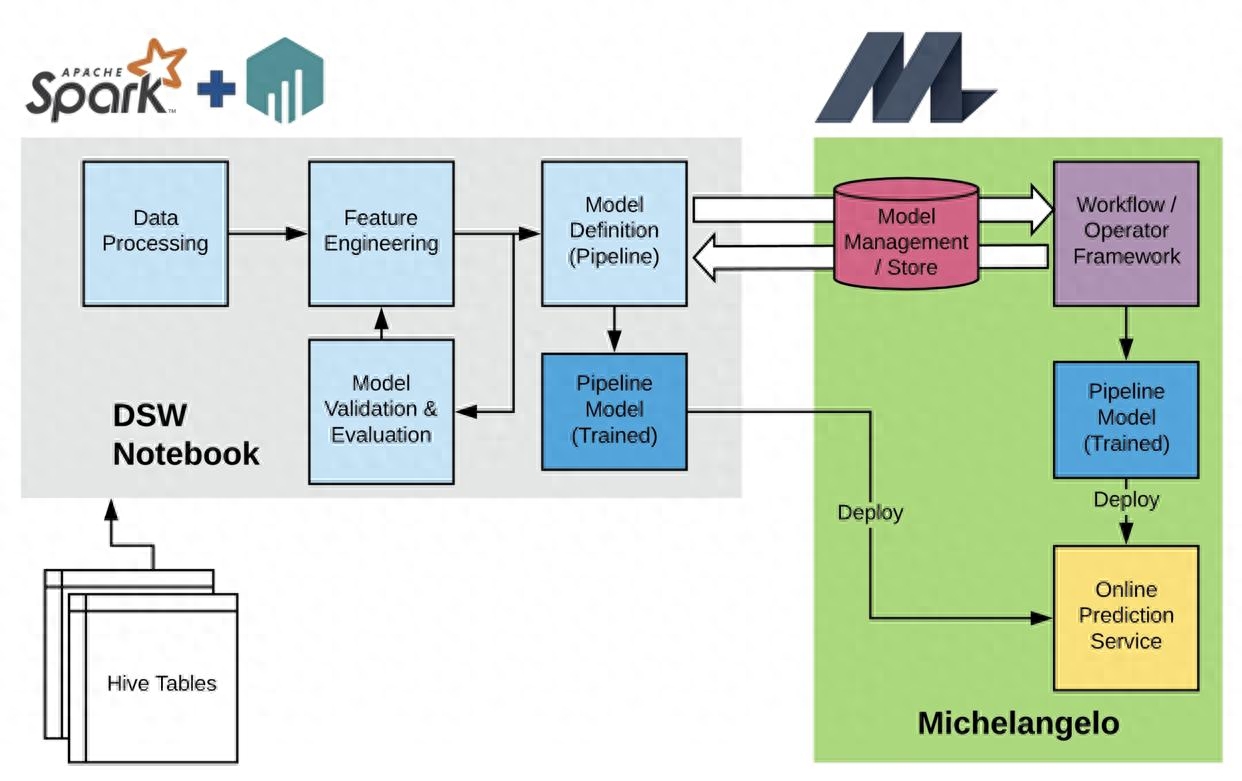
Design of AI and machine learning integrated architecture
- Data collection and processing: First , it is necessary to establish a stable and reliable data collection and processing process. Obtain data from different data sources and perform cleaning, preprocessing, feature extraction, etc. to provide high-quality data for subsequent model training.
- Model training: In the model training stage, a unified model development and training platform needs to be established. This platform should be able to support the selection of different algorithms, the tuning of model parameters, and the ability to automate training and verification.
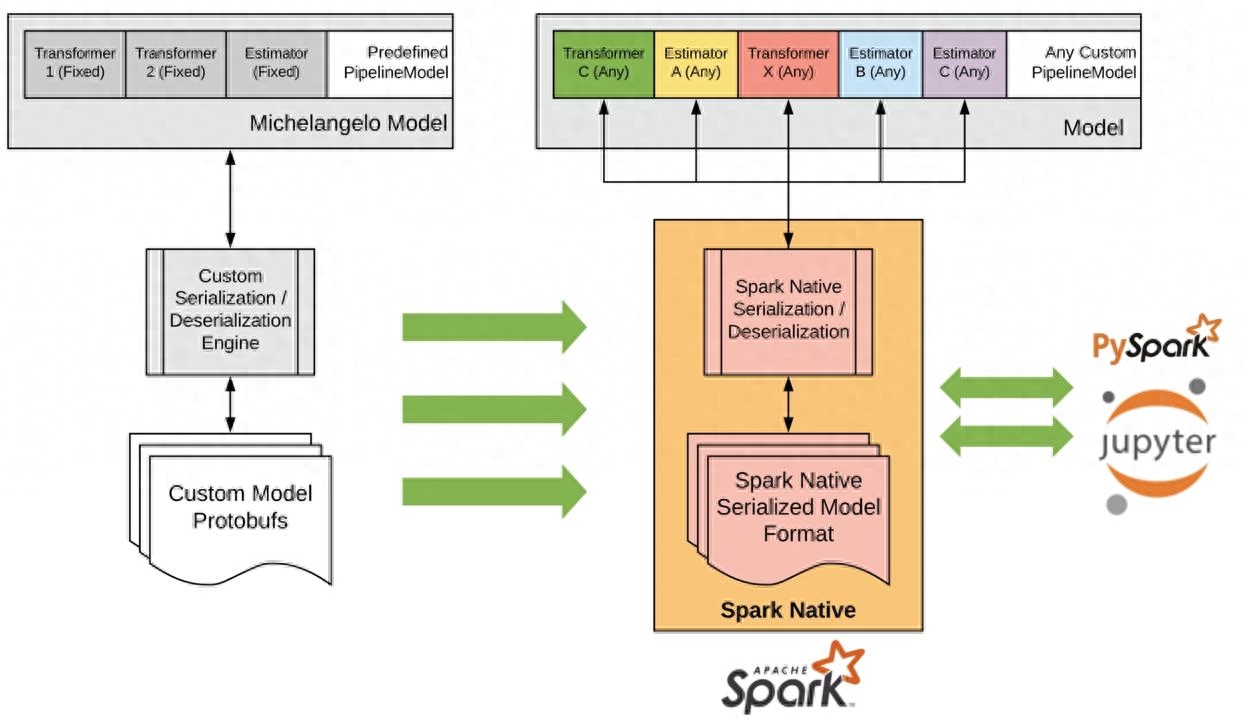
- Model deployment: The trained model needs to be deployed into actual applications. This requires a flexible model deployment architecture that can support different types of models, real-time and offline application scenarios.
- Intelligent decision-making: The ultimate goal of integration is to achieve intelligent decision-making. This includes applying model predictions to actual business, automatically triggering actions, or providing strong support for human decision-making.
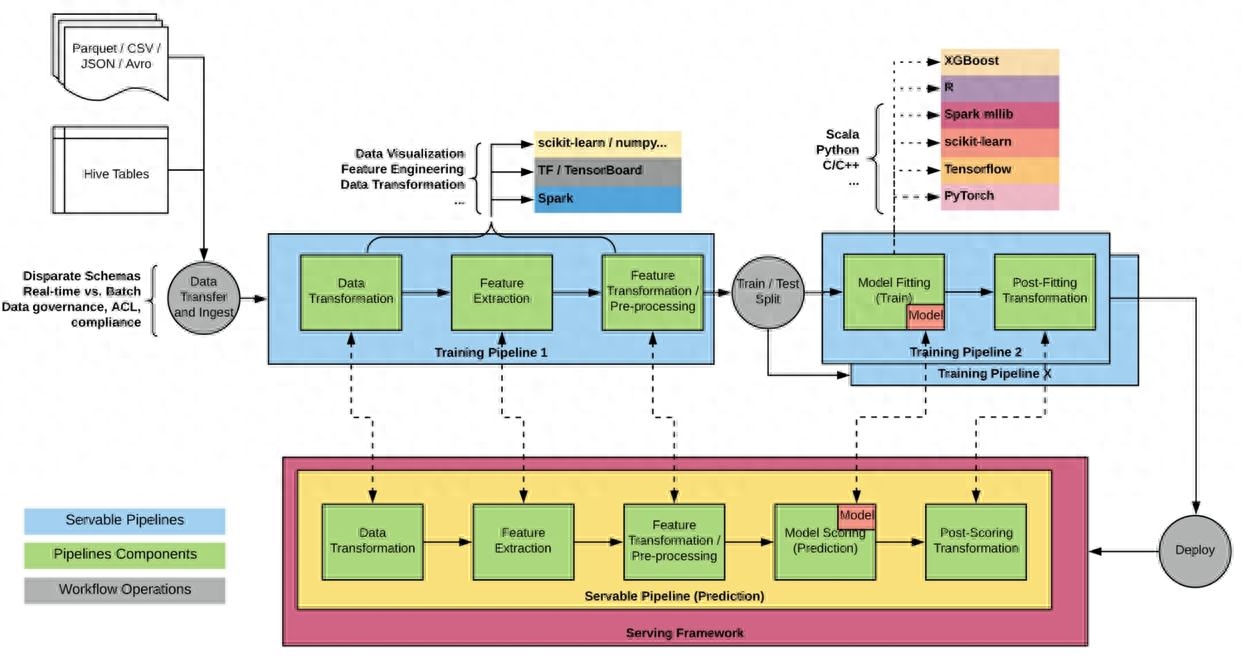
- Feedback and iteration: A good AI integration architecture should be able to continuously collect feedback data, monitor the performance of the model, and Make iterative improvements. This continuous optimization cycle is key to ensuring continued progress in AI applications.
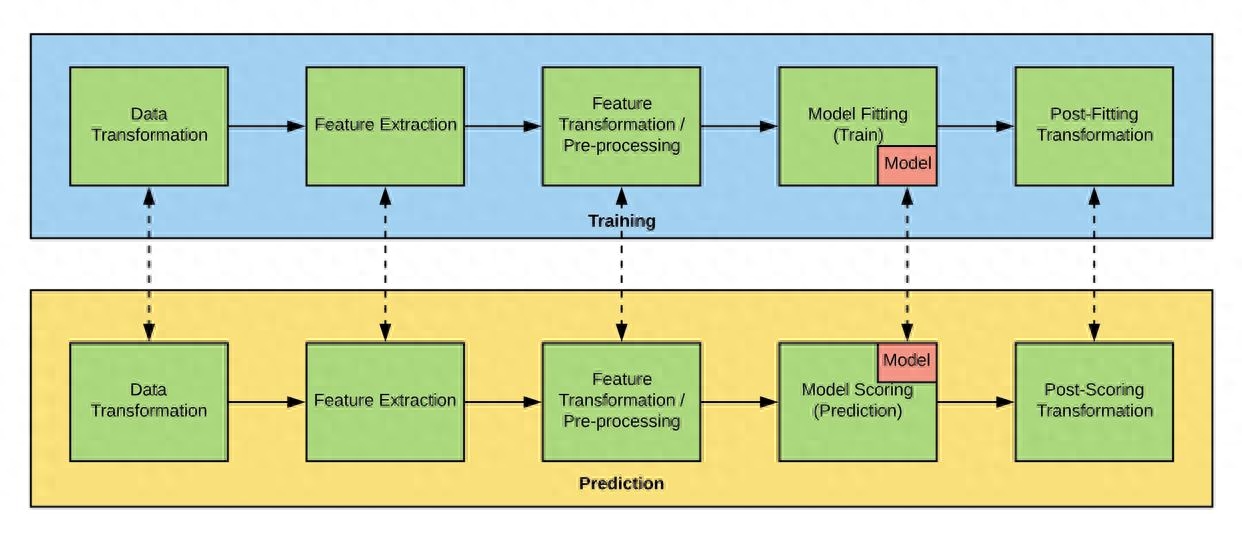
Application of AI and machine learning integrated architecture
This integrated architecture can be applied In multiple fields, including:
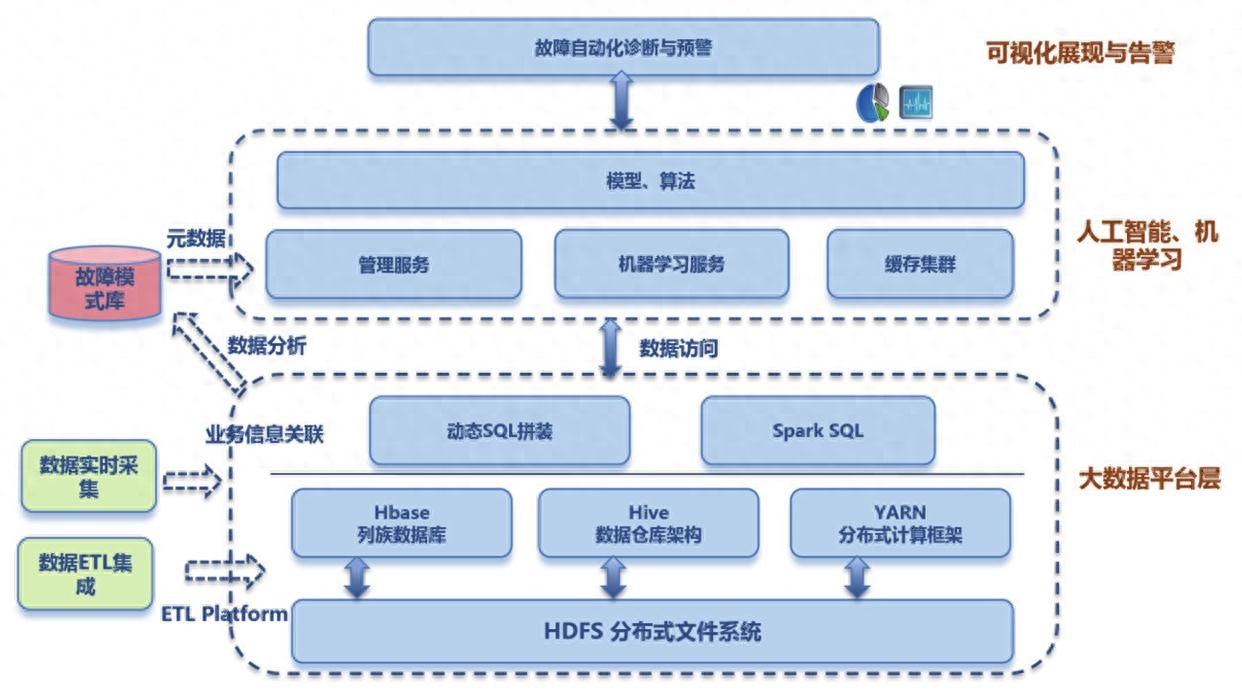
- Financial field: Using AI and machine learning for risk assessment, transaction prediction, and portfolio optimization etc. to achieve smarter investment decisions.
- Manufacturing: Apply AI and machine learning in the manufacturing process for quality control, equipment maintenance, production optimization, and improve production efficiency and product quality.
- Medical and health: Use AI to analyze medical data, carry out disease prediction, diagnostic assistance, drug research and development, and realize personalized medical decision-making.
- Marketing: Based on user behavior data, apply AI and machine learning to carry out precise marketing, personalized recommendations, and improve market response rate.
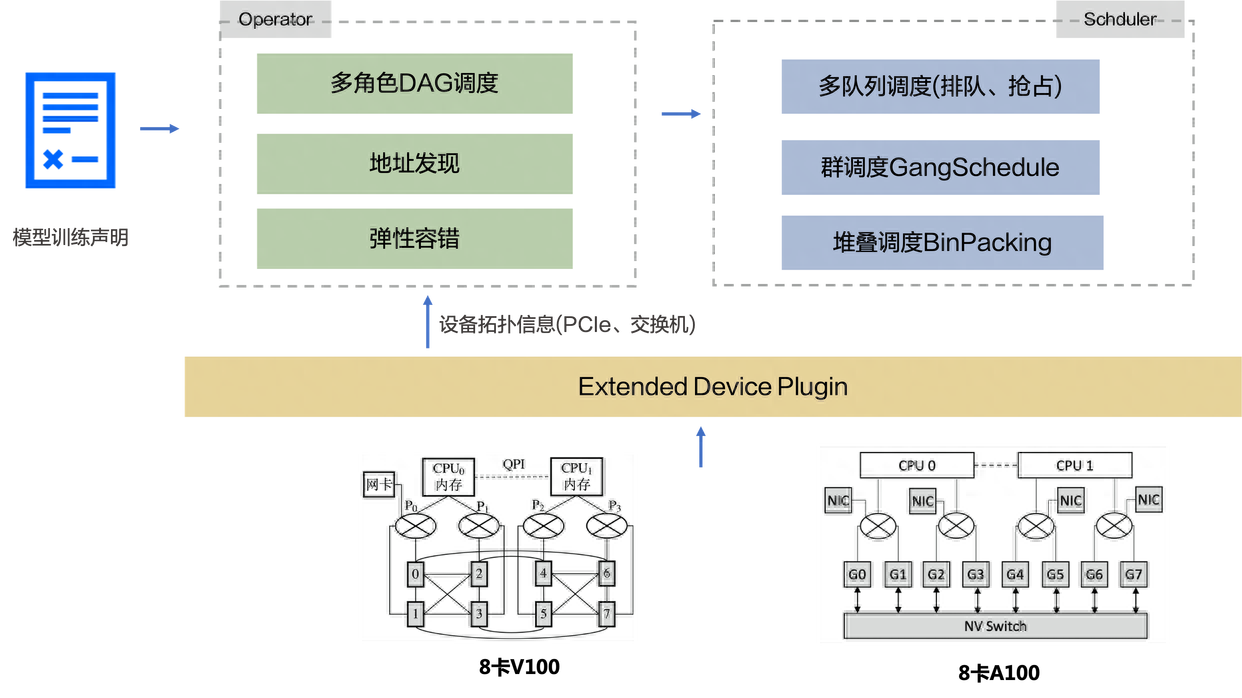
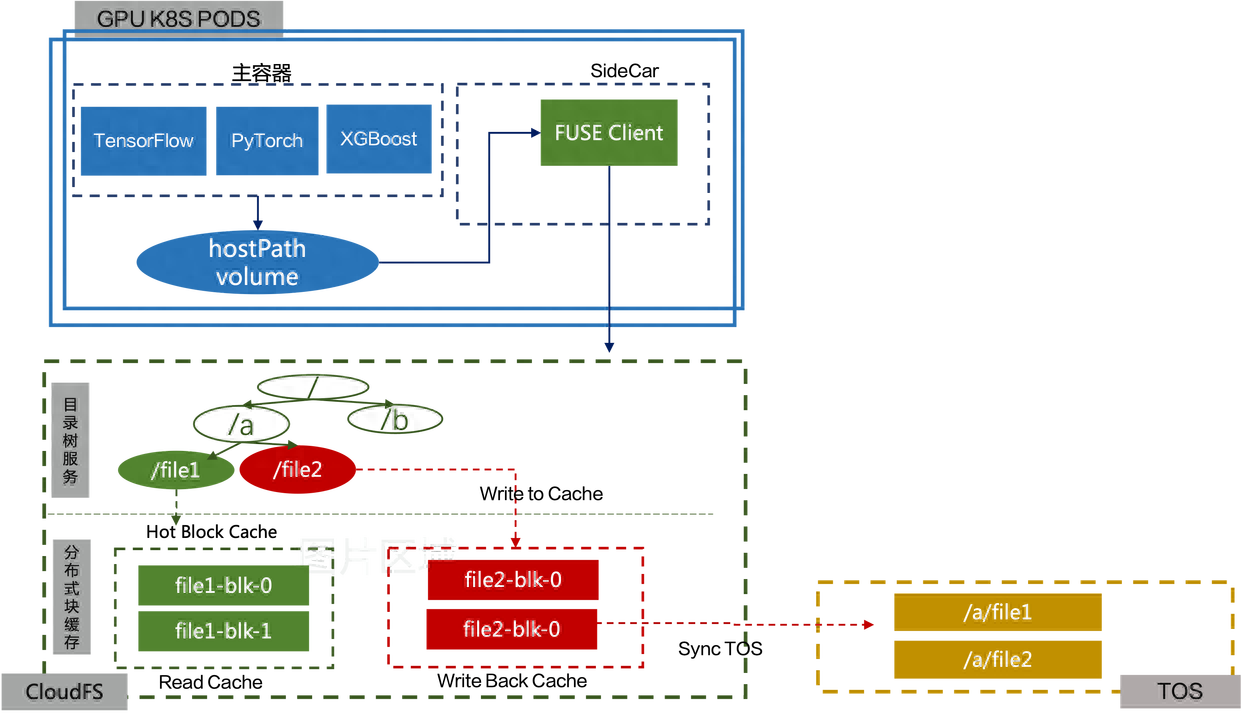
The design and application of AI and machine learning integrated architecture can help enterprises achieve more intelligent, efficient, and accurate decisions and promote business innovation and development. With the continuous advancement of technology, the application of AI and machine learning will become more and more widespread, and the design of integrated architecture will continue to evolve, creating greater value for enterprises
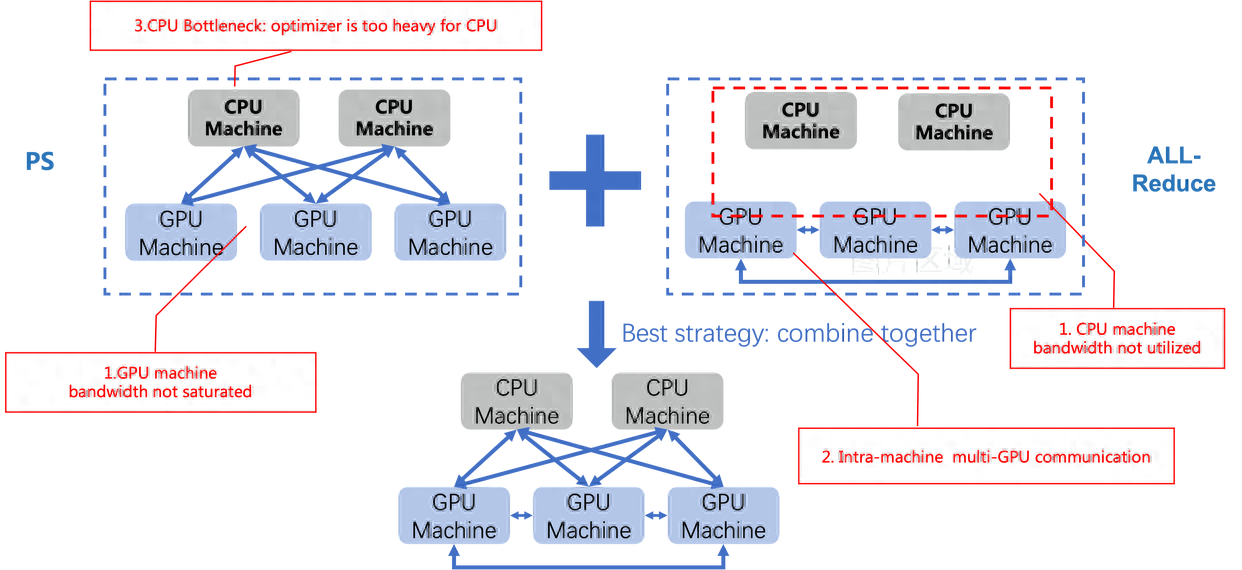
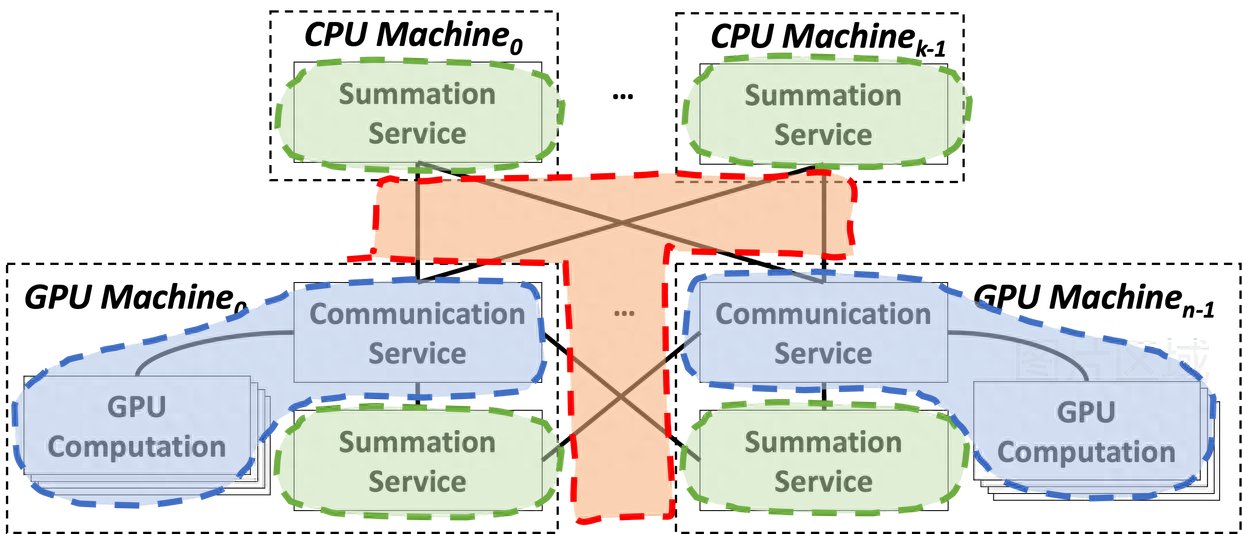
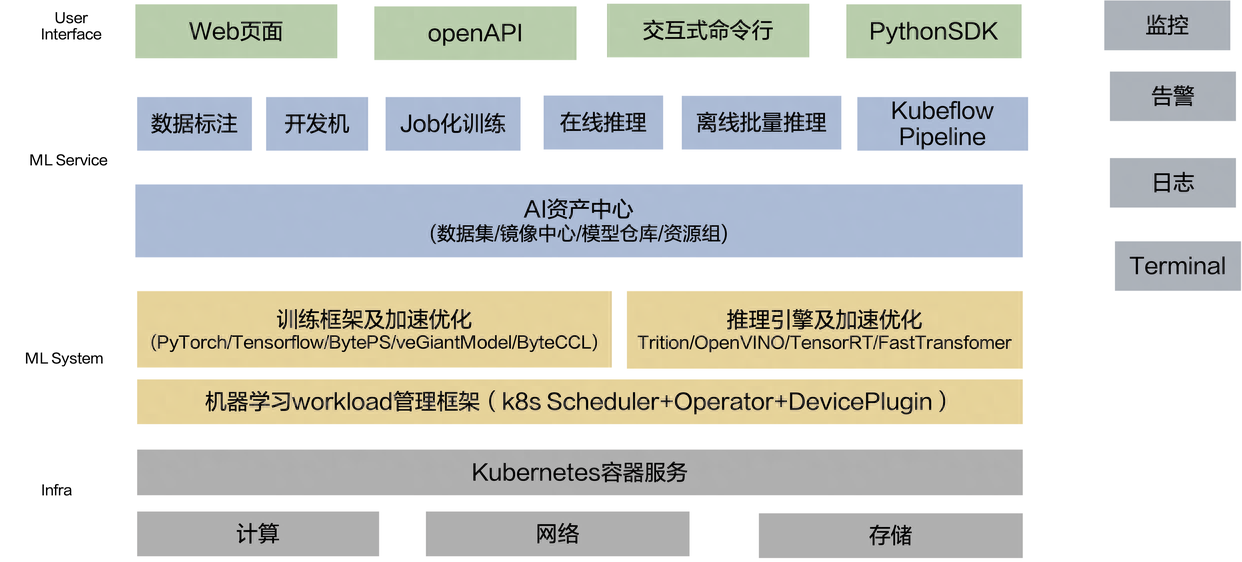
The above is the detailed content of AI and machine learning integrated architecture: realizing intelligent decision-making. For more information, please follow other related articles on the PHP Chinese website!