


Apache IoTDB: an innovative database that solves storage, query and usage problems in industrial IoT scenarios
With the advent of the Industry 4.0 era, the production environment has become more efficient with the introduction of digitization and automation. At the same time, people are beginning to pay attention to the potential value of the massive data brought by smart devices, but how to efficiently store the data generated by smart devices and how to better analyze the massive data has become a problem. Traditional database models and storage methods can no longer meet these needs. Therefore, the time series database emerged as the times require, aiming to achieve efficient data storage and query, and help better explore the potential value of data
Faced with such a situation, Tsinghua University in 2015 Started the development of IoTDB. On September 23, 2020, Apache IoTDB graduated and became an Apache Top-Level Project. It is currently the only Apache Foundation top-level project initiated by Chinese universities and is also the only open source project in the field of IoT data management under the Apache Foundation. In October 2021, the Apache IoTDB core team founded Tianmou Technology and continues to operate IoTDB to help industrial users solve the problems of data "storage, search, and use".
Regarding the core technology developed by Apache IoTDB, several participants collaborated to publish a review paper, which elaborated on the design of IoTDB in detail and completely. The article takes an industrial company that needs to manage tens of thousands of excavators as an example and describes the requirements: "The data is first packaged into the device, and then sent to the server through the 5G mobile network. In the server, the data is written into the time series database, For OLTP queries. Finally, data scientists can load data from the database into the big data platform for complex analysis and prediction, that is, OLAP tasks."
- Paper address: https://dl.acm.org/doi/abs/10.1145/3589775
- ##Project address: https://github.com/ apache/iotdb
The key points of the paper include the following parts:
1. Design of data model: Organization of time series at the logical level and storage in physical schema;
2. TsFile file Format: Self-developed columnar storage file format, which also meets the efficiency of writing, querying, etc.;
3. IoTDB engine : Mainly includes storage engines, query engines, etc.;
Distributed solutions refer to decomposing a task or problem into multiple subtasks , and allocate these subtasks to multiple computers or nodes for processing. This solution improves system reliability, scalability and performance. By distributing tasks to multiple computers, the load on a single computer can be reduced and the concurrent processing capabilities of the system can be improved. At the same time, distributed solutions can also enhance the fault tolerance of the system through redundant backup and failover. Even if a node fails, the system can still continue to run. In today's big data and cloud computing environment, distributed solutions have become a common architectural pattern and are widely used in various fields, such as distributed databases, distributed storage systems, distributed computing platforms, etc.
For the following content, we will explain these key parts in more detail
Detailed interpretation
Requires data model design
As shown in the figure below, we use The tree structure is used to meet high-intensity write operations, and can effectively handle the common delayed data arrival problem in IoT scenarios
In the tree structure, each leaf node represents A sensor, each sensor has a corresponding device. As shown in the bottom two levels in the figure, the same applies to the upward levels
(2) The logical structure has been explained in the previous article, Now we will look at the implementation of the physical structure, which mainly includes two parts: time series (Time series) and sequence family (Series family). The figure below shows that each time series consists of two attributes: time and value. The time series is located through the complete path from the root node to the leaf node. The above figure shows the concept of sequence cluster. A sequence cluster may contain multiple devices, and their data will be stored together in TsFile (a file structure, which will be explained later)
What needs to be rewritten is: 2. TsFile file format design
TsFile is Apache IoTDB's self-developed columnar storage file format. Its structure is shown in the figure below:
When designing TsFile, the research team mainly focused on solving the following problems:
- Save space and compress data as much as possible
- Reduce the number of files
- Will query the time series together in the physical location Close to
- Reduce disk fragmentation
- Efficient access
Mainly provided The solution is:
- Column storage: eliminates null values, saves disk usage; data access locality
- time Sequence encoding: Taking advantage of the unique characteristics of time series in IoT scenarios
- Frequency domain encoding: Frequency domain analysis of time series is widely performed in signal processing
- Specific structure analysis: Page (Page) is the basic storage unit. Chunk contains multiple Pages. The pages in a chunk belong to the same time series and are variable in size; Chunk Group contains multiple Chunks, multiple pages in a group. Chunk belongs to one or more series of devices written within the same period of time. They are placed in continuous disk space because they are often queried together; Block is in memory, and the written block group is first in memory. Buffering is performed in TsFile, and when the memory reaches the threshold, all block groups are flushed to TsFile; the index (FileIndex) records information at the end of the file for data access.
The content that needs to be rewritten is: 3. IoTDB engine
In this part, researchers mainly focus on delayed arrival, efficient query processing and the design of SQL-like queries in IoT scenarios. The structure of the IoTDB engine is shown in the following figure:
In the figure, we can see that the storage engine part is mainly used to process the writing of TsFile, Read and manage. In this part, automatic delay separation technology is adopted (as shown in the figure below)
In most cases, when the time range in TsFile Lazy data separation is recommended when there is no overlap. However, for situations where most data is unordered, lazy data separation is not recommended
After rewriting: Another important component is the query engine, which is responsible for converting SQL Queries are converted into operators that can be executed in the database. At the same time, in order to adapt to industrial IoT scenarios, Apache IoTDB has also designed a rich time series data query function
The content that needs to be rewritten is: 4. Decentralized solution
TsFile can be distributed on HDFS and operated by Spark. In addition, it also provides native solutions for better handling of data distribution and query processing, including partition replication, NB-Raft replication and dynamic read consistency
Comparison results
In the paper, we compare TsFile and IoTDB, which are state-of-the-art file formats and timing databases widely used in industry. Through the following figure, we demonstrate the advantages of Apache IoTDB in many aspects
The above two figures show TsFile’s advantages in write throughput, read time cost and synchronization performance. This is mainly due to TsFile’s IoT-aware structure design, which avoids storing redundant information such as deviceId. Although there is no obvious advantage in disk usage of TsFile, this is because a more granular index is built, resulting in more space taken up. However, this sacrifice can lead to extraordinary query time improvements, as we can see a clear advantage in read time cost
in the above chart It can be clearly seen that IoTDB exhibits better performance in almost all tests, including higher write throughput and lower write latency
In the above experiments, we observed that IoTDB showed better performance when the query data size was larger. Especially in large-scale data aggregation, the advantages of IoTDB are particularly obvious
Summary
This paper introduces a new method called Apache IoTDB A new time series data management system that adopts an open architecture and is specifically designed to support real-time query and big data analysis for IoT applications. The system includes a new time series file format called TsFile, which uses column storage to store times and values to avoid null values and achieve effective compression. Based on TsFile, the IoTDB engine uses an LSM tree-like strategy to handle high-intensity writes and can handle the common delayed data arrival problem in IoT scenarios. Rich scalable query functions and pre-computed statistical information in TsFile enable IoTDB to efficiently handle OLTP and OLAP tasks
IoTDB has become better able to cope with industrial IoT scenarios A new type of database, which is the result of the above technology
The above is the detailed content of Apache IoTDB: an innovative database that solves storage, query and usage problems in industrial IoT scenarios. For more information, please follow other related articles on the PHP Chinese website!
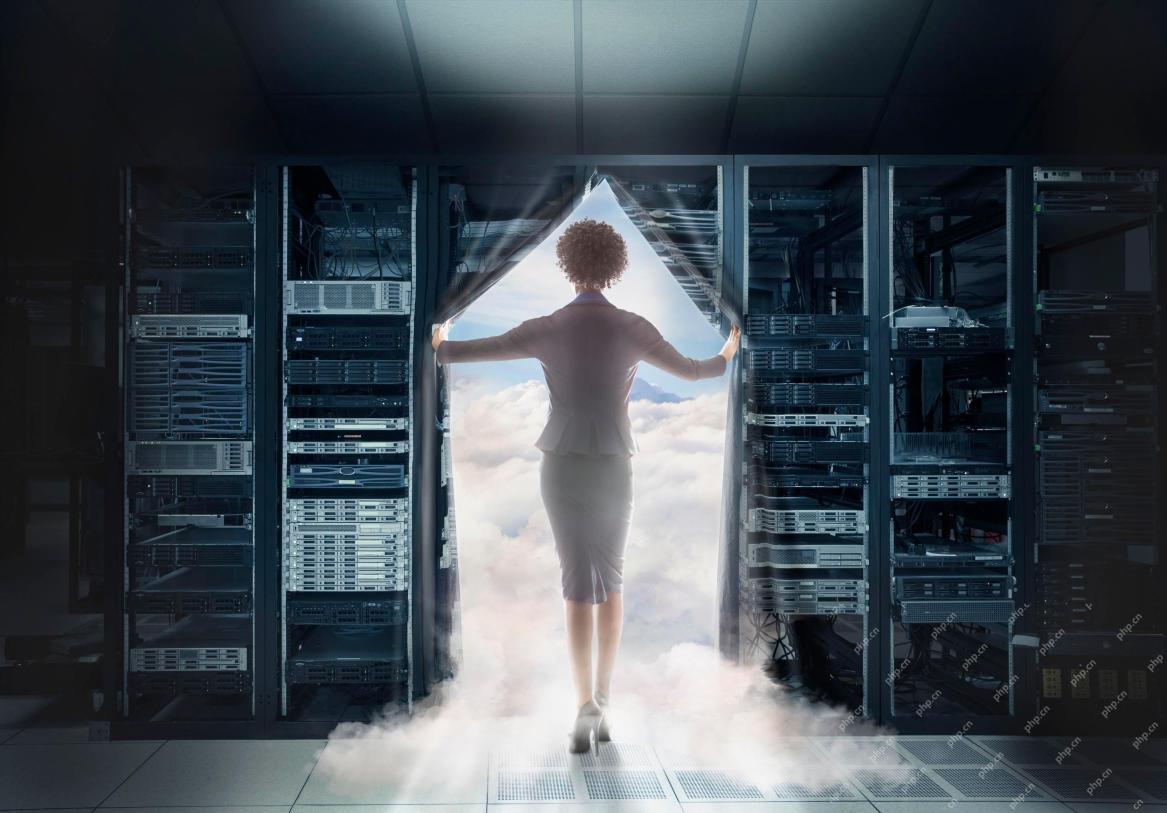
In John Rawls' seminal 1971 book The Theory of Justice, he proposed a thought experiment that we should take as the core of today's AI design and use decision-making: the veil of ignorance. This philosophy provides a simple tool for understanding equity and also provides a blueprint for leaders to use this understanding to design and implement AI equitably. Imagine that you are making rules for a new society. But there is a premise: you don’t know in advance what role you will play in this society. You may end up being rich or poor, healthy or disabled, belonging to a majority or marginal minority. Operating under this "veil of ignorance" prevents rule makers from making decisions that benefit themselves. On the contrary, people will be more motivated to formulate public
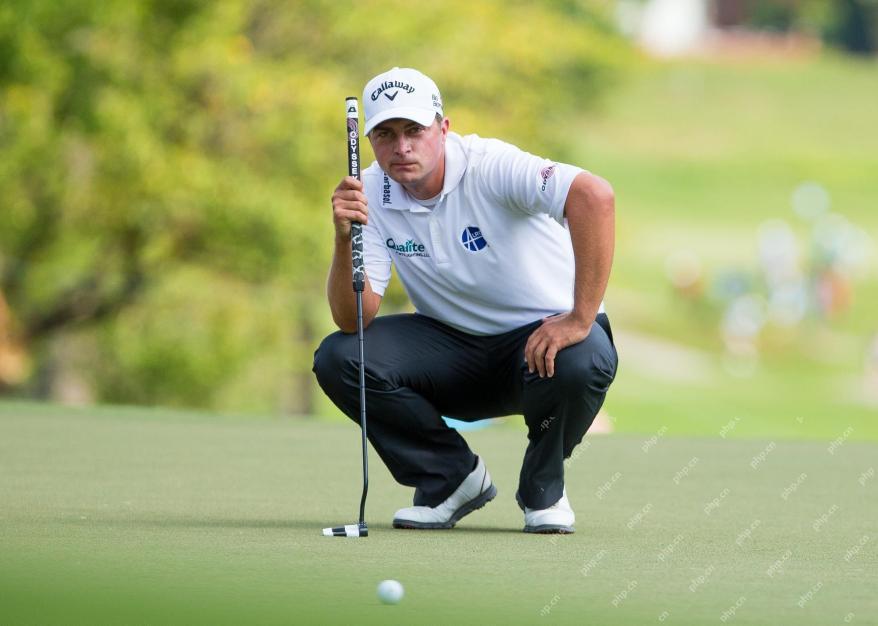
Numerous companies specialize in robotic process automation (RPA), offering bots to automate repetitive tasks—UiPath, Automation Anywhere, Blue Prism, and others. Meanwhile, process mining, orchestration, and intelligent document processing speciali
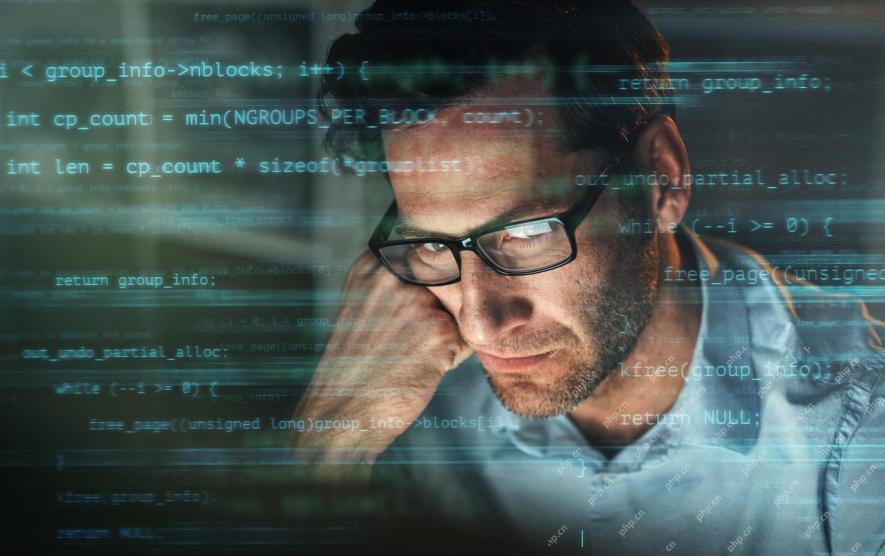
The future of AI is moving beyond simple word prediction and conversational simulation; AI agents are emerging, capable of independent action and task completion. This shift is already evident in tools like Anthropic's Claude. AI Agents: Research a
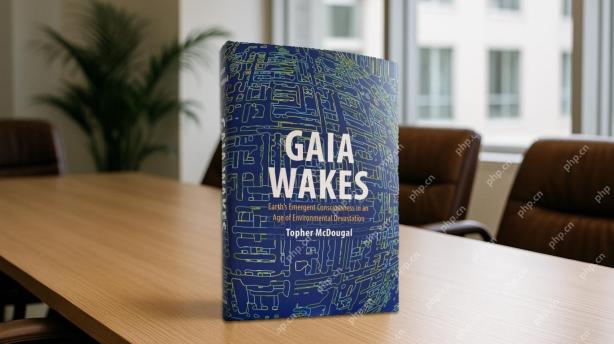
Rapid technological advancements necessitate a forward-looking perspective on the future of work. What happens when AI transcends mere productivity enhancement and begins shaping our societal structures? Topher McDougal's upcoming book, Gaia Wakes:
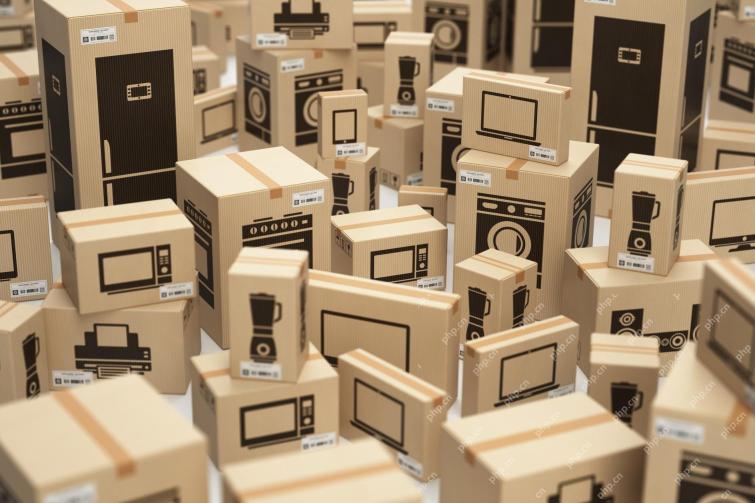
Product classification, often involving complex codes like "HS 8471.30" from systems such as the Harmonized System (HS), is crucial for international trade and domestic sales. These codes ensure correct tax application, impacting every inv
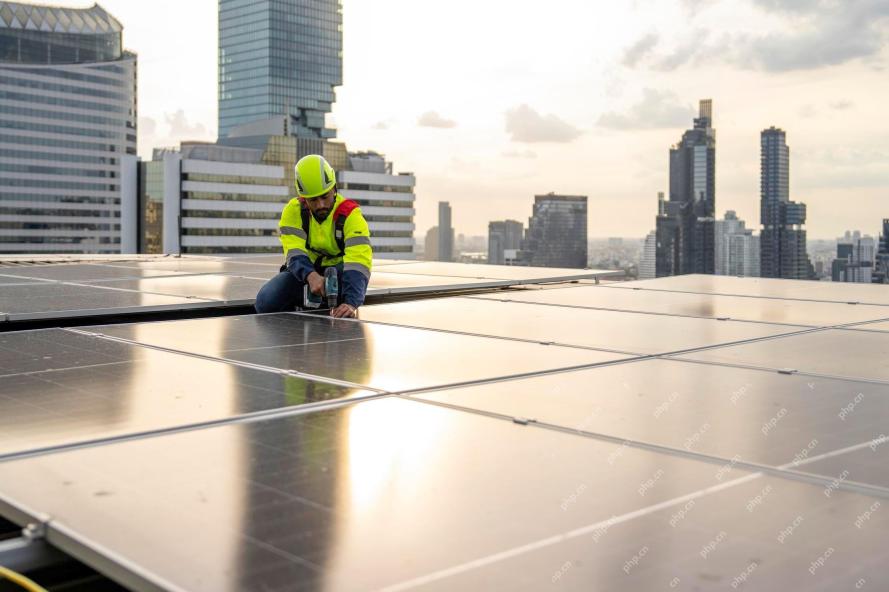
The future of energy consumption in data centers and climate technology investment This article explores the surge in energy consumption in AI-driven data centers and its impact on climate change, and analyzes innovative solutions and policy recommendations to address this challenge. Challenges of energy demand: Large and ultra-large-scale data centers consume huge power, comparable to the sum of hundreds of thousands of ordinary North American families, and emerging AI ultra-large-scale centers consume dozens of times more power than this. In the first eight months of 2024, Microsoft, Meta, Google and Amazon have invested approximately US$125 billion in the construction and operation of AI data centers (JP Morgan, 2024) (Table 1). Growing energy demand is both a challenge and an opportunity. According to Canary Media, the looming electricity
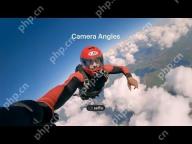
Generative AI is revolutionizing film and television production. Luma's Ray 2 model, as well as Runway's Gen-4, OpenAI's Sora, Google's Veo and other new models, are improving the quality of generated videos at an unprecedented speed. These models can easily create complex special effects and realistic scenes, even short video clips and camera-perceived motion effects have been achieved. While the manipulation and consistency of these tools still need to be improved, the speed of progress is amazing. Generative video is becoming an independent medium. Some models are good at animation production, while others are good at live-action images. It is worth noting that Adobe's Firefly and Moonvalley's Ma
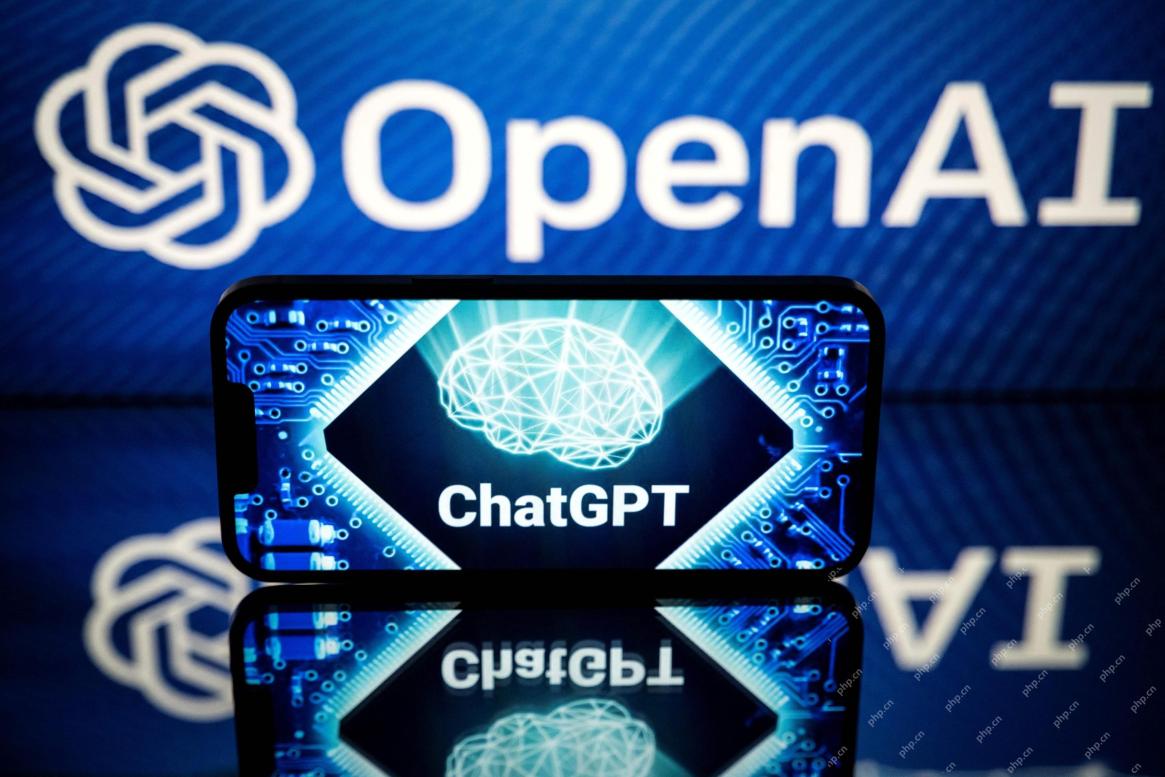
ChatGPT user experience declines: is it a model degradation or user expectations? Recently, a large number of ChatGPT paid users have complained about their performance degradation, which has attracted widespread attention. Users reported slower responses to models, shorter answers, lack of help, and even more hallucinations. Some users expressed dissatisfaction on social media, pointing out that ChatGPT has become “too flattering” and tends to verify user views rather than provide critical feedback. This not only affects the user experience, but also brings actual losses to corporate customers, such as reduced productivity and waste of computing resources. Evidence of performance degradation Many users have reported significant degradation in ChatGPT performance, especially in older models such as GPT-4 (which will soon be discontinued from service at the end of this month). this


Hot AI Tools

Undresser.AI Undress
AI-powered app for creating realistic nude photos

AI Clothes Remover
Online AI tool for removing clothes from photos.

Undress AI Tool
Undress images for free

Clothoff.io
AI clothes remover

Video Face Swap
Swap faces in any video effortlessly with our completely free AI face swap tool!

Hot Article

Hot Tools

Atom editor mac version download
The most popular open source editor
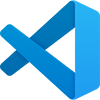
VSCode Windows 64-bit Download
A free and powerful IDE editor launched by Microsoft

Zend Studio 13.0.1
Powerful PHP integrated development environment

SublimeText3 English version
Recommended: Win version, supports code prompts!

Notepad++7.3.1
Easy-to-use and free code editor
