


Lazy prediction library is a Python library for machine learning
Machine learning has helped usher in a transformative era of data analysis, revolutionizing the way we discover complex patterns, make precise predictions, and extract meaningful insights from complex data sets. However, the process of implementing a machine learning model can often feel overwhelming due to complex coding, meticulous parameter tuning, and exhaustive evaluation. Fortunately, Python provides an invaluable library called “Lazy Predict” that aims to simplify the entire process. In this article, we’ll start exploring the Lazy Predict library, delve into its diverse capabilities, and reveal the remarkable ways it accelerates machine learning workflows. By harnessing the power of Lazy Predict, data scientists and machine learning practitioners can save valuable time and energy, allowing them to focus on the critical task of analyzing and interpreting model results. So, let’s embark on this enlightening journey to uncover the fascinating features and significant benefits that Lazy Predict brings to the world of Python-based machine learning.
Latency Prediction Overview
Lazy Predict is a Python package designed to speed up the process of model selection and evaluation in machine learning. It can automatically build and evaluate multiple models on a given dataset, providing comprehensive summary reports demonstrating the performance of each model. By streamlining workflows, Lazy Predict reduces the time and effort required of data scientists and machine learning practitioners. It provides support for a variety of supervised machine learning models, enabling users to efficiently compare and select the best model for their specific tasks. With Lazy Predict, users can streamline their machine learning projects, freeing up time to focus on other critical aspects of analysis.
Installation and Setup
Before looking into the features of Lazy Predict, let’s go through the installation process first. Installing Lazy Predict is very simple using the pip package manager.
pip install lazypredict
This command will download and install the Lazy Predict library and its dependencies on your system.
After installing via pip, seamlessly integrate Lazy Predict into your Python project by importing the necessary classes and functions. With its powerful features, it automates model selection and evaluation to streamline your workflow. Easily analyze model performance and make informed decisions about which models to use. By leveraging Lazy Predict, speed up the machine learning process and focus more on interpreting and leveraging the results generated.
Use delayed prediction
Step 1: Import the required libraries and load the dataset
First, import the basic libraries required for machine learning tasks. For example, if you are solving a classification problem, you might need pandas for data manipulation, sci−kit-learn for model training, and LazyClassifier for lazy prediction. Supervise to take advantage of Lazy Predict’s capabilities. Additionally, load the dataset into a pandas DataFrame. Let's consider an example:
import pandas as pd from sklearn.datasets import load_iris from sklearn.model_selection import train_test_split from lazypredict.Supervised import LazyClassifier # Load the Iris dataset iris = load_iris() X = pd.DataFrame(iris.data, columns=iris.feature_names) y = iris.target
Step 2: Split the data into training set and test set
Now, use the train_test_split function in sci-kit-learn to split the data set into a training set and a test set. This allows you to evaluate the model's performance on unseen data.
This is an example:
# Split the data into training and testing sets X_train, X_test, y_train, y_test = train_test_split(X, y, test_size=0.2, random_state=42)
Step 3: Create a LazyClassifier instance and fit the data
Now comes the exciting part - create a LazyClassifier instance and put it into your training data. This step activates Lazy Predict’s remarkable capabilities to easily automate the building and evaluation of multiple machine learning models. You'll witness the power of Lazy Predict as it handles the complexities of model building and evaluation with ease, giving you a comprehensive understanding of the performance of various models.
This is an example:
# Create an instance of LazyClassifier clf = LazyClassifier(verbose=0, ignore_warnings=True, custom_metric=None) # Fit the classifier to the training data models, predictions = clf.fit(X_train, X_test, y_train, y_test)
In the above code, the verbose parameter is set to 0 to suppress the output of the model summary during the fitting process. The ignore_warnings parameter is set to True to ignore any warning messages that may occur. The custom_metric parameter allows users to define their own evaluation metrics as needed.
Step 4: Get Model Summary Report
After the fitting process is completed, you can get the Lazy Predict model summary report. This report compares the results of various models on the provided datasets.
This is an example:
print(models)
The output of Lazy Predict will present a comprehensive table showing the performance metrics of each model. The table contains the model name and its corresponding accuracy, balanced accuracy, F1 score, training time, and prediction time. It allows users to easily compare and evaluate the pros and cons of different models. The accuracy metric represents the overall correctness of the model's predictions, while the balanced accuracy takes into account an imbalanced data set.
Restrictions and Notes
-
Oversimplification
Lazy Predict provides a quick evaluation of a model, but may oversimplify the model selection process. It does not take into account model-specific hyperparameter tuning or advanced feature engineering techniques, which can significantly affect model performance.
-
Dataset Size
The performance of Lazy Predict is affected by the size of the data set, and it is important to consider the computational impact when working with large data sets. As data set sizes increase, running and evaluating multiple models can become more computationally demanding and time-consuming.
-
Model Diversity
While Lazy Predict supports a wide range of models, it may not include some specialized or state-of-the-art models. In this case, users may need to explore other libraries or implement specific models manually.
-
Interpretability
Lazy Predict focuses on performance evaluation rather than providing detailed model explanations. If interpretability is critical for a specific task, users may need to employ alternative techniques to analyze and understand the inner workings of the model.
in conclusion
Lazy Predict is a valuable asset in the Python ecosystem, streamlining machine learning workflows by automating model selection and evaluation. It saves time and effort for users of all levels, allowing them to explore multiple models, compare performance, and gain insights quickly. Ideal for rapid prototyping, education, and initial model exploration, Lazy Predict increases productivity and efficiency. However, it is important to consider its limitations and complement it with additional steps, such as hyperparameter tuning and feature engineering for complex tasks. Overall, Lazy Predict is a powerful tool that can significantly enhance machine learning toolkits and benefit Python-based projects.
The above is the detailed content of Lazy prediction library is a Python library for machine learning. For more information, please follow other related articles on the PHP Chinese website!
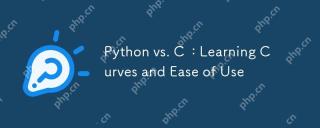
Python is easier to learn and use, while C is more powerful but complex. 1. Python syntax is concise and suitable for beginners. Dynamic typing and automatic memory management make it easy to use, but may cause runtime errors. 2.C provides low-level control and advanced features, suitable for high-performance applications, but has a high learning threshold and requires manual memory and type safety management.
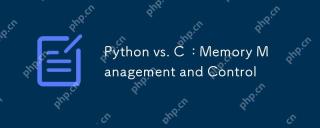
Python and C have significant differences in memory management and control. 1. Python uses automatic memory management, based on reference counting and garbage collection, simplifying the work of programmers. 2.C requires manual management of memory, providing more control but increasing complexity and error risk. Which language to choose should be based on project requirements and team technology stack.
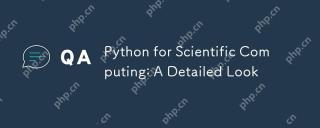
Python's applications in scientific computing include data analysis, machine learning, numerical simulation and visualization. 1.Numpy provides efficient multi-dimensional arrays and mathematical functions. 2. SciPy extends Numpy functionality and provides optimization and linear algebra tools. 3. Pandas is used for data processing and analysis. 4.Matplotlib is used to generate various graphs and visual results.
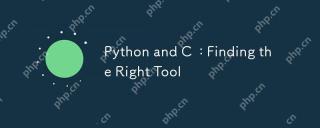
Whether to choose Python or C depends on project requirements: 1) Python is suitable for rapid development, data science, and scripting because of its concise syntax and rich libraries; 2) C is suitable for scenarios that require high performance and underlying control, such as system programming and game development, because of its compilation and manual memory management.
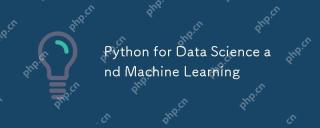
Python is widely used in data science and machine learning, mainly relying on its simplicity and a powerful library ecosystem. 1) Pandas is used for data processing and analysis, 2) Numpy provides efficient numerical calculations, and 3) Scikit-learn is used for machine learning model construction and optimization, these libraries make Python an ideal tool for data science and machine learning.
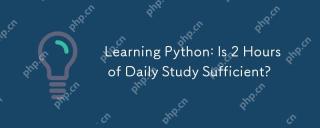
Is it enough to learn Python for two hours a day? It depends on your goals and learning methods. 1) Develop a clear learning plan, 2) Select appropriate learning resources and methods, 3) Practice and review and consolidate hands-on practice and review and consolidate, and you can gradually master the basic knowledge and advanced functions of Python during this period.
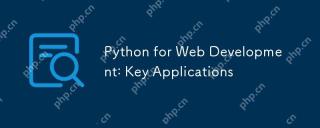
Key applications of Python in web development include the use of Django and Flask frameworks, API development, data analysis and visualization, machine learning and AI, and performance optimization. 1. Django and Flask framework: Django is suitable for rapid development of complex applications, and Flask is suitable for small or highly customized projects. 2. API development: Use Flask or DjangoRESTFramework to build RESTfulAPI. 3. Data analysis and visualization: Use Python to process data and display it through the web interface. 4. Machine Learning and AI: Python is used to build intelligent web applications. 5. Performance optimization: optimized through asynchronous programming, caching and code
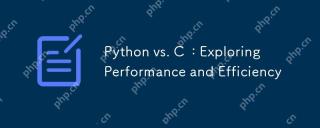
Python is better than C in development efficiency, but C is higher in execution performance. 1. Python's concise syntax and rich libraries improve development efficiency. 2.C's compilation-type characteristics and hardware control improve execution performance. When making a choice, you need to weigh the development speed and execution efficiency based on project needs.


Hot AI Tools

Undresser.AI Undress
AI-powered app for creating realistic nude photos

AI Clothes Remover
Online AI tool for removing clothes from photos.

Undress AI Tool
Undress images for free

Clothoff.io
AI clothes remover

Video Face Swap
Swap faces in any video effortlessly with our completely free AI face swap tool!

Hot Article

Hot Tools

MinGW - Minimalist GNU for Windows
This project is in the process of being migrated to osdn.net/projects/mingw, you can continue to follow us there. MinGW: A native Windows port of the GNU Compiler Collection (GCC), freely distributable import libraries and header files for building native Windows applications; includes extensions to the MSVC runtime to support C99 functionality. All MinGW software can run on 64-bit Windows platforms.
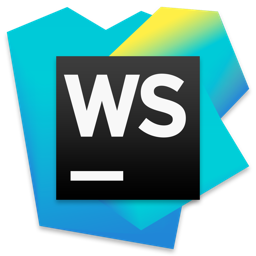
WebStorm Mac version
Useful JavaScript development tools

mPDF
mPDF is a PHP library that can generate PDF files from UTF-8 encoded HTML. The original author, Ian Back, wrote mPDF to output PDF files "on the fly" from his website and handle different languages. It is slower than original scripts like HTML2FPDF and produces larger files when using Unicode fonts, but supports CSS styles etc. and has a lot of enhancements. Supports almost all languages, including RTL (Arabic and Hebrew) and CJK (Chinese, Japanese and Korean). Supports nested block-level elements (such as P, DIV),

DVWA
Damn Vulnerable Web App (DVWA) is a PHP/MySQL web application that is very vulnerable. Its main goals are to be an aid for security professionals to test their skills and tools in a legal environment, to help web developers better understand the process of securing web applications, and to help teachers/students teach/learn in a classroom environment Web application security. The goal of DVWA is to practice some of the most common web vulnerabilities through a simple and straightforward interface, with varying degrees of difficulty. Please note that this software

SecLists
SecLists is the ultimate security tester's companion. It is a collection of various types of lists that are frequently used during security assessments, all in one place. SecLists helps make security testing more efficient and productive by conveniently providing all the lists a security tester might need. List types include usernames, passwords, URLs, fuzzing payloads, sensitive data patterns, web shells, and more. The tester can simply pull this repository onto a new test machine and he will have access to every type of list he needs.