Update nested dictionary in Python
In Python, a dictionary is a general-purpose data structure that allows you to store and retrieve key-value pairs efficiently. In particular, nested dictionaries provide a convenient way to organize and represent complex data. However, updating values in nested dictionaries can sometimes be a bit difficult.
Access nested dictionary elements
To update a value in a nested dictionary, we first need to access a specific key in the dictionary hierarchy. Python allows you to access nested elements by using keys consecutively. For example -
nested_dict = {'outer_key': {'inner_key': 'old_value'}} nested_dict['outer_key']['inner_key'] = 'new_value'
In the above code snippet, we access the nested element "inner_key" by continuously linking the key and update its value to "new_value".
Update single-level nested dictionary
Sometimes, you may encounter nested dictionaries where the keys at different levels have no fixed structure. In this case, a more general approach is needed. Python provides the update() method, which allows us to merge one dictionary into another dictionary, thus updating its values. This is an example −
nested_dict = {'outer_key': {'inner_key': 'old_value'}} update_dict = {'inner_key': 'new_value'} nested_dict['outer_key'].update(update_dict)
In the above code snippet, we created a separate dictionary update_dict that contains the key-value pairs we want to update. By using the update() method, we merge the update_dict into a nested dictionary at the specified key level, effectively updating its value.
Update multi-level nested dictionary
If you need to update values in multiple levels of a nested dictionary, you can use a recursive approach. This method involves recursively traversing the dictionary until the required key is found. This is an example −
def update_nested_dict(nested_dict, keys, new_value): if len(keys) == 1: nested_dict[keys[0]] = new_value else: key = keys[0] if key in nested_dict: update_nested_dict(nested_dict[key], keys[1:], new_value) nested_dict = {'outer_key': {'inner_key': {'deep_key': 'old_value'}}} keys = ['outer_key', 'inner_key', 'deep_key'] new_value = 'new_value' update_nested_dict(nested_dict, keys, new_value)
In the above code snippet, we define a recursive function update_nested_dict that takes as arguments a nested dictionary, a list of keys, and a new value. This function checks if there is only one key left in the list; if so, it updates the value in the nested dictionary. Otherwise, it traverses the nested dictionary deeper until it finds the required key.
Handling lost keys and creating new keys
When updating nested dictionaries, it is important to consider the case where the specified key may not exist. Python provides several techniques to handle such situations and create new keys when needed.
Use Setdefault() method
The setdefault() method is a convenience method for updating values in a nested dictionary when dealing with missing keys. It allows you to specify a default value if the key does not exist. If the key is found, the existing value is returned. Otherwise, the default value will be inserted into the dictionary. This is an example −
nested_dict = {'outer_key': {}} nested_dict['outer_key'].setdefault('inner_key', 'new_value')
In the above code snippet, we use the setdefault() method to update the value of "inner_key" within the "outer_key" of the nested dictionary. If "inner_key" does not exist, create it with value "new_value".
Use Defaultdict class
The defaultdict class in the collections module provides a powerful way to handle missing keys in nested dictionaries. When a non-existing key is accessed, it is automatically assigned a default value. This is an example −
from collections import defaultdict nested_dict = defaultdict(dict) nested_dict['outer_key']['inner_key'] = 'new_value'
In the above code snippet, we create a defaultdict and use the dict type as the default factory. This ensures that any key that does not exist will automatically create a new nested dictionary. We then proceed to update the "inner_key" inside the "outer_key" with the value "new_value".
Update values in deeply nested dictionaries
The recursive approach becomes more useful if you have a nested dictionary with multiple nesting levels and you need to update the values in the innermost level. You can extend the recursive function to handle deeply nested dictionaries by modifying the traversal logic accordingly.
def update_deep_nested_dict(nested_dict, keys, new_value): if len(keys) == 1: nested_dict[keys[0]] = new_value else: key = keys[0] if key in nested_dict: update_deep_nested_dict(nested_dict[key], keys[1:], new_value) else: nested_dict[key] = {} update_deep_nested_dict(nested_dict[key], keys[1:], new_value) nested_dict = {'outer_key': {'inner_key': {'deep_key': 'old_value'}}} keys = ['outer_key', 'inner_key', 'deep_key'] new_value = 'new_value' update_deep_nested_dict(nested_dict, keys, new_value)
In the above code snippet, we enhanced the previous recursive function to handle deeply nested dictionaries. If a key is missing from any level, a new empty dictionary is created and the function continues traversing until the innermost key is reached.
Immutable Nested Dictionary
It should be noted that dictionaries in Python are mutable objects. So when you update a nested dictionary, the changes will be reflected in all references to the dictionary. If you need to maintain the original state of a nested dictionary, you can create a deep copy of it before making any updates.
import copy nested_dict = {'outer_key': {'inner_key': 'old_value'}} updated_dict = copy.deepcopy(nested_dict) updated_dict['outer_key']['inner_key'] = 'new_value'
Example
import copy nested_dict = {'outer_key': {'inner_key': 'old_value'}} updated_dict = copy.deepcopy(nested_dict) updated_dict['outer_key']['inner_key'] = 'new_value' print("Original nested_dict:") print(nested_dict) print("\nUpdated_dict:") print(updated_dict)
Output
The following is the output of the code snippet mentioned in section −
Original nested_dict: {'outer_key': {'inner_key': 'old_value'}} Updated_dict: {'outer_key': {'inner_key': 'new_value'}}
In the above code snippet, the copy.deepcopy() function creates a complete copy of the nested dictionary, including all levels of nesting. This allows you to update the copied dictionary without affecting the original dictionary.
Update values using dictionary comprehension
For simple updates within a nested dictionary, you can use dictionary comprehensions. This approach is suitable when you have a known set of keys to update.
Example
nested_dict = {'outer_key': {'inner_key': 'old_value'}} keys_to_update = ['outer_key'] updated_dict = {key: 'new_value' if key in keys_to_update else value for key, value in nested_dict.items()}
Output
This is the output of the above code snippet−
Original nested_dict: {'outer_key': {'inner_key': 'old_value'}} Updated_dict: {'outer_key': {'inner_key': 'new_value'}}
在代码片段中,我们从包含嵌套结构的nested_dict字典开始。我们使用copy.deepcopy()创建nested_dict的深层副本并将其分配给updated_dict。然后,我们将updated_dict中'outer_key'内的'inner_key'的值更新为'new_value'。
最后,我们打印原始的nested_dict和更新后的updated_dict。从输出中可以看到,原始的nested_dict保持不变,而updated_dict反映了更新后的值。
结论
在 Python 中更新嵌套字典可能涉及访问特定键、合并字典或使用递归技术。通过了解这些不同的方法,您可以根据您的特定要求有效地更新嵌套字典中的值。在选择最合适的方法时,请记住考虑嵌套字典的结构和复杂性。
Python 的灵活性和内置方法(例如 update()、setdefault() 和 defaultdict 类)提供了强大的工具来处理各种情况,包括丢失键和创建新键。
The above is the detailed content of Update nested dictionary in Python. For more information, please follow other related articles on the PHP Chinese website!
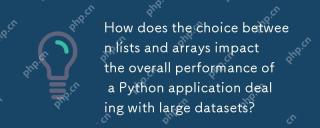
ForhandlinglargedatasetsinPython,useNumPyarraysforbetterperformance.1)NumPyarraysarememory-efficientandfasterfornumericaloperations.2)Avoidunnecessarytypeconversions.3)Leveragevectorizationforreducedtimecomplexity.4)Managememoryusagewithefficientdata
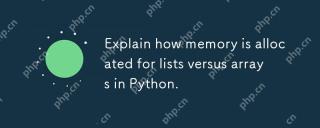
InPython,listsusedynamicmemoryallocationwithover-allocation,whileNumPyarraysallocatefixedmemory.1)Listsallocatemorememorythanneededinitially,resizingwhennecessary.2)NumPyarraysallocateexactmemoryforelements,offeringpredictableusagebutlessflexibility.
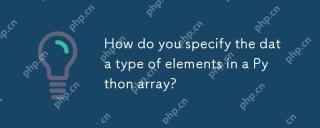
InPython, YouCansSpectHedatatYPeyFeLeMeReModelerErnSpAnT.1) UsenPyNeRnRump.1) UsenPyNeRp.DLOATP.PLOATM64, Formor PrecisconTrolatatypes.
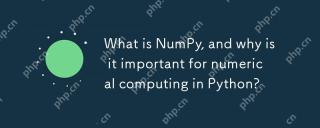
NumPyisessentialfornumericalcomputinginPythonduetoitsspeed,memoryefficiency,andcomprehensivemathematicalfunctions.1)It'sfastbecauseitperformsoperationsinC.2)NumPyarraysaremorememory-efficientthanPythonlists.3)Itoffersawiderangeofmathematicaloperation
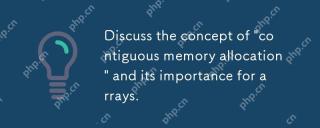
Contiguousmemoryallocationiscrucialforarraysbecauseitallowsforefficientandfastelementaccess.1)Itenablesconstanttimeaccess,O(1),duetodirectaddresscalculation.2)Itimprovescacheefficiencybyallowingmultipleelementfetchespercacheline.3)Itsimplifiesmemorym
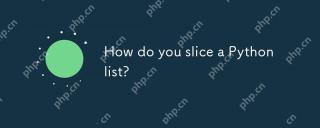
SlicingaPythonlistisdoneusingthesyntaxlist[start:stop:step].Here'showitworks:1)Startistheindexofthefirstelementtoinclude.2)Stopistheindexofthefirstelementtoexclude.3)Stepistheincrementbetweenelements.It'susefulforextractingportionsoflistsandcanuseneg
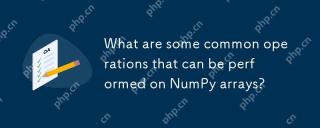
NumPyallowsforvariousoperationsonarrays:1)Basicarithmeticlikeaddition,subtraction,multiplication,anddivision;2)Advancedoperationssuchasmatrixmultiplication;3)Element-wiseoperationswithoutexplicitloops;4)Arrayindexingandslicingfordatamanipulation;5)Ag
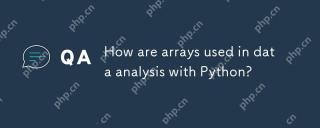
ArraysinPython,particularlythroughNumPyandPandas,areessentialfordataanalysis,offeringspeedandefficiency.1)NumPyarraysenableefficienthandlingoflargedatasetsandcomplexoperationslikemovingaverages.2)PandasextendsNumPy'scapabilitieswithDataFramesforstruc


Hot AI Tools

Undresser.AI Undress
AI-powered app for creating realistic nude photos

AI Clothes Remover
Online AI tool for removing clothes from photos.

Undress AI Tool
Undress images for free

Clothoff.io
AI clothes remover

Video Face Swap
Swap faces in any video effortlessly with our completely free AI face swap tool!

Hot Article

Hot Tools

Notepad++7.3.1
Easy-to-use and free code editor

SublimeText3 Linux new version
SublimeText3 Linux latest version
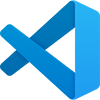
VSCode Windows 64-bit Download
A free and powerful IDE editor launched by Microsoft

SAP NetWeaver Server Adapter for Eclipse
Integrate Eclipse with SAP NetWeaver application server.

mPDF
mPDF is a PHP library that can generate PDF files from UTF-8 encoded HTML. The original author, Ian Back, wrote mPDF to output PDF files "on the fly" from his website and handle different languages. It is slower than original scripts like HTML2FPDF and produces larger files when using Unicode fonts, but supports CSS styles etc. and has a lot of enhancements. Supports almost all languages, including RTL (Arabic and Hebrew) and CJK (Chinese, Japanese and Korean). Supports nested block-level elements (such as P, DIV),
