


Explain how memory is allocated for lists versus arrays in Python.
In Python, lists use dynamic memory allocation with over-allocation, while NumPy arrays allocate fixed memory. 1) Lists allocate more memory than needed initially, resizing when necessary. 2) NumPy arrays allocate exact memory for elements, offering predictable usage but less flexibility.
When it comes to understanding how memory is allocated for lists and arrays in Python, we're diving into a fascinating aspect of the language that often goes unnoticed until you start dealing with performance optimization or memory management issues. Let's unpack this topic with a blend of technical insight and practical experience.
In Python, what we commonly refer to as "arrays" are actually lists, which are dynamic and flexible. However, for the sake of this discussion, let's differentiate between Python's native lists and the more traditional concept of arrays that you might find in languages like C or Java.
Memory Allocation for Python Lists:
Python lists are implemented as dynamic arrays, which means they can grow or shrink in size as elements are added or removed. This flexibility comes with a cost in terms of memory management. When you create a list, Python allocates a contiguous block of memory to store the list's elements. But here's where it gets interesting: Python doesn't allocate just enough memory for the initial elements. Instead, it allocates more memory than needed, anticipating that the list might grow. This over-allocation is a strategy to minimize the frequency of resizing the list.
Here's a simple example to illustrate:
my_list = [1, 2, 3]
When you create my_list
, Python might allocate memory for, say, 8 elements, even though you've only initialized it with 3. This extra memory is what allows the list to grow without immediately needing to reallocate.
However, when the list does need to grow beyond its pre-allocated capacity, Python will create a new, larger array, copy the old elements into it, and then deallocate the old array. This process can be expensive in terms of performance, especially for large lists.
Memory Allocation for Arrays (NumPy Arrays):
Now, let's talk about arrays in the context of NumPy, which provides a more traditional array-like structure in Python. NumPy arrays are fixed-size, homogeneous data structures stored in a contiguous block of memory. When you create a NumPy array, the memory allocation is straightforward: it allocates exactly the amount of memory needed for the array's elements.
Here's an example:
import numpy as np my_array = np.array([1, 2, 3])
In this case, my_array
will allocate memory for exactly 3 integers, no more, no less. If you try to change the size of a NumPy array after creation, you'll need to create a new array, which can be less flexible but more efficient in terms of memory usage and performance.
Deep Dive into Memory Management:
Lists: The dynamic nature of lists means that memory allocation can be more complex. Python uses a technique called "over-allocation" to manage list growth. This means that when a list grows, it doesn't always need to reallocate memory, which can save time but at the cost of using more memory than strictly necessary. However, this can lead to memory fragmentation over time, especially if lists are frequently resized.
Arrays (NumPy): NumPy arrays, on the other hand, offer more predictable memory usage. Since they are fixed-size, you know exactly how much memory they will use. This can be a significant advantage in scenarios where memory is a critical resource, such as in scientific computing or data analysis.
Practical Experience and Tips:
In my experience, choosing between lists and arrays often comes down to the specific requirements of your project. If you need flexibility and don't mind a bit of overhead, Python lists are great. They're easy to use and can handle a variety of data types. However, if you're dealing with large datasets or performance-critical applications, NumPy arrays can offer significant advantages in terms of memory efficiency and speed.
Pitfalls and Considerations:
Lists: Be cautious of the overhead of frequent resizing. If you know the approximate size of your list in advance, consider initializing it with a larger size to minimize reallocations.
Arrays (NumPy): While they're efficient, they're less flexible. If your data size changes frequently, you might end up creating and destroying arrays more often than you'd like, which can still impact performance.
In conclusion, understanding how memory is allocated for lists versus arrays in Python can help you make more informed decisions about which data structure to use in different scenarios. Whether you're optimizing for speed, memory, or flexibility, the choice between these two can have a significant impact on your application's performance and resource usage.
The above is the detailed content of Explain how memory is allocated for lists versus arrays in Python.. For more information, please follow other related articles on the PHP Chinese website!
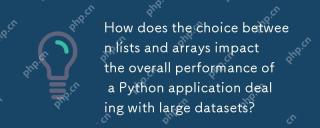
ForhandlinglargedatasetsinPython,useNumPyarraysforbetterperformance.1)NumPyarraysarememory-efficientandfasterfornumericaloperations.2)Avoidunnecessarytypeconversions.3)Leveragevectorizationforreducedtimecomplexity.4)Managememoryusagewithefficientdata
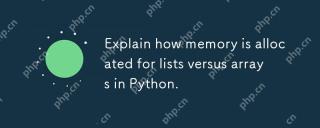
InPython,listsusedynamicmemoryallocationwithover-allocation,whileNumPyarraysallocatefixedmemory.1)Listsallocatemorememorythanneededinitially,resizingwhennecessary.2)NumPyarraysallocateexactmemoryforelements,offeringpredictableusagebutlessflexibility.
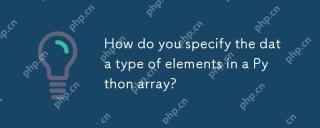
InPython, YouCansSpectHedatatYPeyFeLeMeReModelerErnSpAnT.1) UsenPyNeRnRump.1) UsenPyNeRp.DLOATP.PLOATM64, Formor PrecisconTrolatatypes.
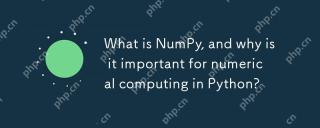
NumPyisessentialfornumericalcomputinginPythonduetoitsspeed,memoryefficiency,andcomprehensivemathematicalfunctions.1)It'sfastbecauseitperformsoperationsinC.2)NumPyarraysaremorememory-efficientthanPythonlists.3)Itoffersawiderangeofmathematicaloperation
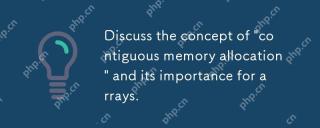
Contiguousmemoryallocationiscrucialforarraysbecauseitallowsforefficientandfastelementaccess.1)Itenablesconstanttimeaccess,O(1),duetodirectaddresscalculation.2)Itimprovescacheefficiencybyallowingmultipleelementfetchespercacheline.3)Itsimplifiesmemorym
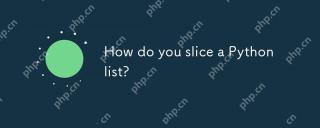
SlicingaPythonlistisdoneusingthesyntaxlist[start:stop:step].Here'showitworks:1)Startistheindexofthefirstelementtoinclude.2)Stopistheindexofthefirstelementtoexclude.3)Stepistheincrementbetweenelements.It'susefulforextractingportionsoflistsandcanuseneg
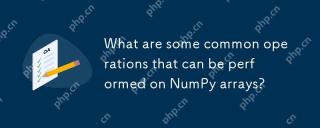
NumPyallowsforvariousoperationsonarrays:1)Basicarithmeticlikeaddition,subtraction,multiplication,anddivision;2)Advancedoperationssuchasmatrixmultiplication;3)Element-wiseoperationswithoutexplicitloops;4)Arrayindexingandslicingfordatamanipulation;5)Ag
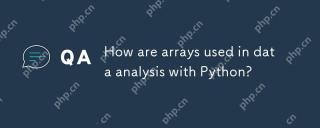
ArraysinPython,particularlythroughNumPyandPandas,areessentialfordataanalysis,offeringspeedandefficiency.1)NumPyarraysenableefficienthandlingoflargedatasetsandcomplexoperationslikemovingaverages.2)PandasextendsNumPy'scapabilitieswithDataFramesforstruc


Hot AI Tools

Undresser.AI Undress
AI-powered app for creating realistic nude photos

AI Clothes Remover
Online AI tool for removing clothes from photos.

Undress AI Tool
Undress images for free

Clothoff.io
AI clothes remover

Video Face Swap
Swap faces in any video effortlessly with our completely free AI face swap tool!

Hot Article

Hot Tools

Notepad++7.3.1
Easy-to-use and free code editor

MantisBT
Mantis is an easy-to-deploy web-based defect tracking tool designed to aid in product defect tracking. It requires PHP, MySQL and a web server. Check out our demo and hosting services.
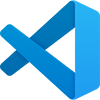
VSCode Windows 64-bit Download
A free and powerful IDE editor launched by Microsoft
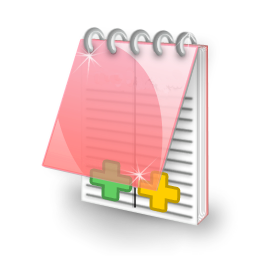
EditPlus Chinese cracked version
Small size, syntax highlighting, does not support code prompt function
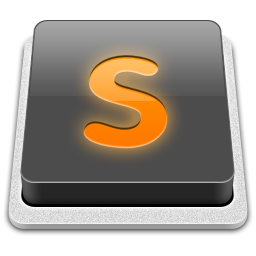
SublimeText3 Mac version
God-level code editing software (SublimeText3)
