How does Scrapy automate deployment to the server?
Scrapy is one of the most popular crawler frameworks in Python. It can help users quickly build crawlers and improve the efficiency of crawlers. The automatic deployment of Scrapy to the server can make it more convenient for users to deploy and manage crawler programs. This article will introduce how to automatically deploy Scrapy to the server.
1. Preparation tools
To automatically deploy Scrapy to the server, we need some tools, these tools include:
- Git: version control tool, used for Manage code between local and server.
- SSH: Secure Shell, securely transfer data and execute commands between local and remote servers.
- Virtualenv: Python virtual environment management tool, which can make our Scrapy deployment environment clearer and independent.
- Supervisor: Process control system, used to manage and monitor the Scrapy crawler process on the server.
These tools are very common. If they are not installed yet, you can install them according to the official documentation.
2. Create a project
Before automated Scrapy deployment, we first need to create a Scrapy project locally. You can use the Scrapy command line tool to create:
scrapy startproject myproject
This will create a Scrapy project named myproject
, which includes some default code and directory structure. Next, we can write Spiders, Pipelines and other components.
In order to make our Scrapy project more convenient to deploy and run on the server, we can create a requirements.txt
file in the project root directory to manage the project dependencies Python libraries and versions. This file can be created through pip, for example:
pip freeze > requirements.txt
This will automatically generate a requirements.txt
file, which contains all Python libraries installed in the current system and their version information . We need to manually remove the unnecessary libraries in it and keep the necessary libraries like Scrapy and other related libraries and tools. After determining the dependencies, we can use pip to install these dependencies in the virtual environment:
pip install -r requirements.txt
3. Configure the server
Before starting the deployment, we need to install the required components on the server. Taking Ubuntu as an example, we need to install Git, SSH, Virtualenv and Supervisor. It can be installed through the following command:
sudo apt-get update sudo apt-get install git ssh virtualenv supervisor
After the installation is completed, we need to create a new user on the server. This user will play an important role in subsequent deployment and operation and will have access to the Scrapy crawler. You can use the following command to create a new user named myuser
:
sudo adduser myuser
Next, we need to create a new directory to save Scrapy deployment files and related configurations. On the server, you can use the following command to create a directory /srv/myproject
:
sudo mkdir /srv/myproject sudo chown myuser:myuser /srv/myproject
4. Set up the Git warehouse and SSH
Next, we need to Scrapy projects are uploaded to a Git repository and deployed using SSH. Locally, we can use the following command to upload all the code in the Scrapy project to the Git repository:
git init git add . git commit -m "Initial commit" git remote add origin ssh://myuser@myserver.com/srv/myproject.git git push -u origin master
This code uploads the Scrapy project to the remote server and saves it in /srv/myproject.git
Under contents.
Next, we need to configure SSH on the server so that we can use SSH to connect to the Git repository and perform related operations. We can create an SSH key for this and add the public key to the authorized_keys
file on the server side.
First, we can create a new SSH key locally using the following command:
ssh-keygen
This will create a pair of public and private keys. Next, we need to add the public key to the authorized_keys
file on the server side:
ssh myuser@myserver.com "mkdir -p ~/.ssh && chmod 0700 ~/.ssh && echo 'PUBLIC_KEY' >> ~/.ssh/authorized_keys && chmod 0600 ~/.ssh/authorized_keys"
Please replace PUBLIC_KEY
with the public key on your local computer.
Now we can use SSH to connect to the server and perform operations.
5. Deploy the Scrapy project
Now, we are ready to automatically deploy the Scrapy project on the server. To do this, we need to create a new virtual environment on the server and install Scrapy and other required dependent libraries:
mkdir /srv/myproject/env virtualenv /srv/myproject/env source /srv/myproject/env/bin/activate pip install scrapy supervisor
Create a Scrapy project working directory on the server, clone the Scrapy project from the Git repository, and Create a supervisord.conf
file for configuring process management:
mkdir /srv/myproject/src cd /srv/myproject/src git clone ssh://myuser@myserver.com/srv/myproject.git . cp /srv/myproject/env/bin/supervisord /srv/myproject/env/bin/supervisord.conf /etc sudo supervisorctl reread sudo supervisorctl update
This will clone the Scrapy project to the server and place it in the /srv/myproject
directory Create the supervisord.conf
file. We can edit the supervisord.conf
file to start the Scrapy crawler:
[program:myproject] command=/srv/myproject/env/bin/scrapy crawl myspider directory=/srv/myproject/src autostart=true autorestart=true stopasgroup=true killasgroup=true
where the command
parameter is used to start the Scrapy crawler, and the directory
parameter is used to Specify the working directory. The autostart
and autorestart
parameters are used to automatically restart the Scrapy crawler after it stops. The stopasgroup
and killasgroup
parameters are used to stop the process. Stop all related processes at the same time.
Finally, we can use the following command to start the Scrapy crawler:
sudo supervisorctl start myproject
In this way, the Scrapy crawler can be deployed to the server and run automatically.
Summarize
Automated deployment of Scrapy to the server is a very convenient and efficient method. We can upload the code to the remote Git repository and connect to the server through SSH for deployment and management. By using Virtualenv and Supervisor, we can better control the project's environment and process, and let Scrapy crawlers run automatically on the server.
The above is the detailed content of How does Scrapy automate deployment to the server?. For more information, please follow other related articles on the PHP Chinese website!
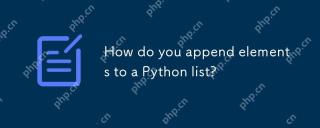
ToappendelementstoaPythonlist,usetheappend()methodforsingleelements,extend()formultipleelements,andinsert()forspecificpositions.1)Useappend()foraddingoneelementattheend.2)Useextend()toaddmultipleelementsefficiently.3)Useinsert()toaddanelementataspeci
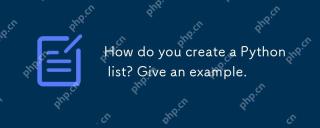
TocreateaPythonlist,usesquarebrackets[]andseparateitemswithcommas.1)Listsaredynamicandcanholdmixeddatatypes.2)Useappend(),remove(),andslicingformanipulation.3)Listcomprehensionsareefficientforcreatinglists.4)Becautiouswithlistreferences;usecopy()orsl
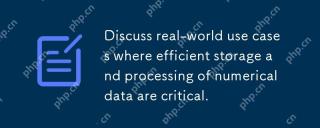
In the fields of finance, scientific research, medical care and AI, it is crucial to efficiently store and process numerical data. 1) In finance, using memory mapped files and NumPy libraries can significantly improve data processing speed. 2) In the field of scientific research, HDF5 files are optimized for data storage and retrieval. 3) In medical care, database optimization technologies such as indexing and partitioning improve data query performance. 4) In AI, data sharding and distributed training accelerate model training. System performance and scalability can be significantly improved by choosing the right tools and technologies and weighing trade-offs between storage and processing speeds.
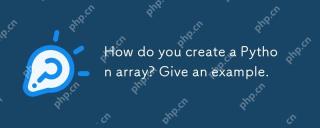
Pythonarraysarecreatedusingthearraymodule,notbuilt-inlikelists.1)Importthearraymodule.2)Specifythetypecode,e.g.,'i'forintegers.3)Initializewithvalues.Arraysofferbettermemoryefficiencyforhomogeneousdatabutlessflexibilitythanlists.
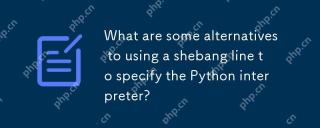
In addition to the shebang line, there are many ways to specify a Python interpreter: 1. Use python commands directly from the command line; 2. Use batch files or shell scripts; 3. Use build tools such as Make or CMake; 4. Use task runners such as Invoke. Each method has its advantages and disadvantages, and it is important to choose the method that suits the needs of the project.
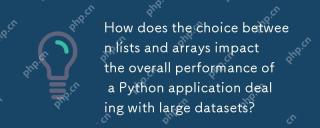
ForhandlinglargedatasetsinPython,useNumPyarraysforbetterperformance.1)NumPyarraysarememory-efficientandfasterfornumericaloperations.2)Avoidunnecessarytypeconversions.3)Leveragevectorizationforreducedtimecomplexity.4)Managememoryusagewithefficientdata
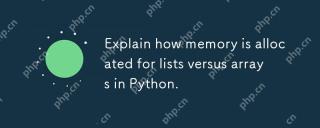
InPython,listsusedynamicmemoryallocationwithover-allocation,whileNumPyarraysallocatefixedmemory.1)Listsallocatemorememorythanneededinitially,resizingwhennecessary.2)NumPyarraysallocateexactmemoryforelements,offeringpredictableusagebutlessflexibility.
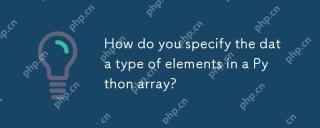
InPython, YouCansSpectHedatatYPeyFeLeMeReModelerErnSpAnT.1) UsenPyNeRnRump.1) UsenPyNeRp.DLOATP.PLOATM64, Formor PrecisconTrolatatypes.


Hot AI Tools

Undresser.AI Undress
AI-powered app for creating realistic nude photos

AI Clothes Remover
Online AI tool for removing clothes from photos.

Undress AI Tool
Undress images for free

Clothoff.io
AI clothes remover

Video Face Swap
Swap faces in any video effortlessly with our completely free AI face swap tool!

Hot Article

Hot Tools

mPDF
mPDF is a PHP library that can generate PDF files from UTF-8 encoded HTML. The original author, Ian Back, wrote mPDF to output PDF files "on the fly" from his website and handle different languages. It is slower than original scripts like HTML2FPDF and produces larger files when using Unicode fonts, but supports CSS styles etc. and has a lot of enhancements. Supports almost all languages, including RTL (Arabic and Hebrew) and CJK (Chinese, Japanese and Korean). Supports nested block-level elements (such as P, DIV),

SublimeText3 Linux new version
SublimeText3 Linux latest version

SublimeText3 Chinese version
Chinese version, very easy to use
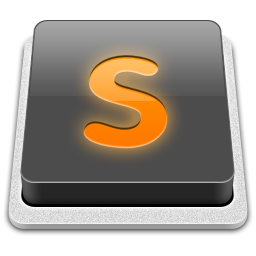
SublimeText3 Mac version
God-level code editing software (SublimeText3)
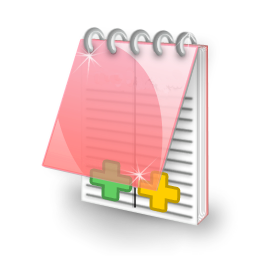
EditPlus Chinese cracked version
Small size, syntax highlighting, does not support code prompt function
