


Build multi-tier distributed applications using Python and Scala
With the rapid development of science and technology, human beings' demand for efficient, fast, and accurate calculation and data processing has become increasingly urgent. The emergence of distributed applications provides us with a brand new solution. As one of the two most popular programming languages at present, can Python and Scala be combined to build more powerful and efficient distributed applications? Next, let’s take a look at whether Python and Scala can work together to create multi-level distributed applications.
What is a multi-tier distributed application?
In large and complex systems, layered architecture is a common organizational method, and multi-level distributed applications are also developed based on this idea. Generally speaking, multi-level distributed applications can be divided into three layers: runtime layer, application layer and resource layer.
- Runtime layer
The runtime layer is the most basic and lowest part of a multi-level distributed application. It is responsible for managing services, load balancing, fault detection and fault tolerance in distributed systems, providing a solid foundation for the normal operation of the above two layers.
- Application layer
The application layer is the core part of a multi-level distributed application and can implement specific application tasks, such as analysis, search, recommendation, etc. The application layer is also the highest-level part, which usually accepts user requests and gives response results.
- Resource layer
The resource layer is a bridge between the running layer and the application layer of multi-level distributed applications. It can manage all resources such as databases, storage devices, computing resources, etc. so that they can be utilized efficiently. The resource layer can also help the application layer achieve scalability and flexibility.
The advantages of combining Python and Scala
Python and Scala can be regarded as two completely different programming languages. Python focuses on the simplicity and ease of use of the language, while Scala focuses more on oriented Objects can be considered as an upgraded version of Java. While there are indeed many differences between the two, they can be used to build efficient multi-tier distributed applications.
In addition, both Python and Scala have the following things in common:
- Can be used in conjunction with a large number of different technical frameworks
- Both can process and manage big data, Support big data analysis and processing
- Both support distributed computing and multi-threaded processing
Therefore, the combination of Python and Scala can bring the following advantages:
- High program development efficiency: Libraries in Python and frameworks in Scala can effectively reduce developers’ programming workload and greatly improve the readability and maintainability of code.
- High performance: Scala can be integrated with Java, which means that the performance of the Java virtual machine can be efficiently utilized. Python can also be integrated with C, so that C can be used when high-performance computing is required. performance.
- Modularization: Both Python and Scala support modular development, which is very beneficial for multi-person collaborative development.
How to build multi-level distributed applications?
When using Python and Scala to build multi-level distributed applications, you need to do the following work first:
- Determine program requirements: clarify the functions and services that need to be developed, and determine the system required hierarchical structure.
- Choose a development framework: Choose the Python framework and Scala framework that suits you, and use them to build the system during the development process.
- Install and configure the development environment: Set up a development environment on Python and Scala, and use relevant IDEs or editors for development. Developers also need to be proficient in the use of related technologies, such as Git, etc.
When carrying out specific development, the runtime layer needs to be developed first. In Python, you can use frameworks such as Flask for development. In Scala, you can use frameworks such as Akka or Play for development. These frameworks can use distributed code to directly process business logic and manage data.
Next, you need to develop the application layer. In Python, you can use libraries such as Pandas for development, and in Scala, you can use Spark and Scikit-learn. These libraries and frameworks can help developers complete tasks such as data analysis and machine learning more efficiently.
Finally, the resource layer needs to be developed to manage dynamic resources through various cluster management technologies, such as Apache, to provide efficient management and support for upper-layer applications.
Summary
The benefits of using Python and Scala to build multi-level distributed applications not only greatly improve development efficiency and program operation efficiency, but also enable developers to better manage and utilize large-scale applications. data. After learning Python and Scala in depth, we can combine them to build efficient multi-level distributed applications, which will help improve team collaboration and project development efficiency and are suitable for various large-scale and important data analysis and computing tasks.
The above is the detailed content of Build multi-tier distributed applications using Python and Scala. For more information, please follow other related articles on the PHP Chinese website!
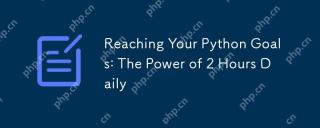
By investing 2 hours of Python learning every day, you can effectively improve your programming skills. 1. Learn new knowledge: read documents or watch tutorials. 2. Practice: Write code and complete exercises. 3. Review: Consolidate the content you have learned. 4. Project practice: Apply what you have learned in actual projects. Such a structured learning plan can help you systematically master Python and achieve career goals.
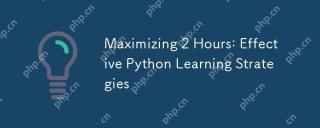
Methods to learn Python efficiently within two hours include: 1. Review the basic knowledge and ensure that you are familiar with Python installation and basic syntax; 2. Understand the core concepts of Python, such as variables, lists, functions, etc.; 3. Master basic and advanced usage by using examples; 4. Learn common errors and debugging techniques; 5. Apply performance optimization and best practices, such as using list comprehensions and following the PEP8 style guide.
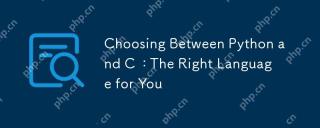
Python is suitable for beginners and data science, and C is suitable for system programming and game development. 1. Python is simple and easy to use, suitable for data science and web development. 2.C provides high performance and control, suitable for game development and system programming. The choice should be based on project needs and personal interests.
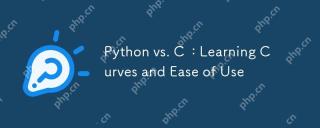
Python is easier to learn and use, while C is more powerful but complex. 1. Python syntax is concise and suitable for beginners. Dynamic typing and automatic memory management make it easy to use, but may cause runtime errors. 2.C provides low-level control and advanced features, suitable for high-performance applications, but has a high learning threshold and requires manual memory and type safety management.
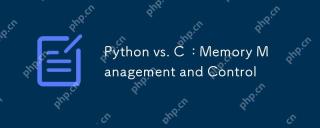
Python and C have significant differences in memory management and control. 1. Python uses automatic memory management, based on reference counting and garbage collection, simplifying the work of programmers. 2.C requires manual management of memory, providing more control but increasing complexity and error risk. Which language to choose should be based on project requirements and team technology stack.
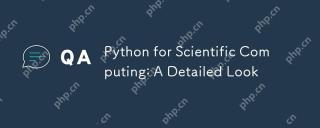
Python's applications in scientific computing include data analysis, machine learning, numerical simulation and visualization. 1.Numpy provides efficient multi-dimensional arrays and mathematical functions. 2. SciPy extends Numpy functionality and provides optimization and linear algebra tools. 3. Pandas is used for data processing and analysis. 4.Matplotlib is used to generate various graphs and visual results.
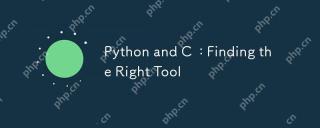
Whether to choose Python or C depends on project requirements: 1) Python is suitable for rapid development, data science, and scripting because of its concise syntax and rich libraries; 2) C is suitable for scenarios that require high performance and underlying control, such as system programming and game development, because of its compilation and manual memory management.
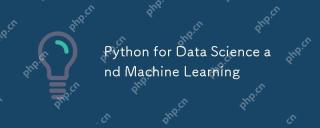
Python is widely used in data science and machine learning, mainly relying on its simplicity and a powerful library ecosystem. 1) Pandas is used for data processing and analysis, 2) Numpy provides efficient numerical calculations, and 3) Scikit-learn is used for machine learning model construction and optimization, these libraries make Python an ideal tool for data science and machine learning.


Hot AI Tools

Undresser.AI Undress
AI-powered app for creating realistic nude photos

AI Clothes Remover
Online AI tool for removing clothes from photos.

Undress AI Tool
Undress images for free

Clothoff.io
AI clothes remover

Video Face Swap
Swap faces in any video effortlessly with our completely free AI face swap tool!

Hot Article

Hot Tools

MantisBT
Mantis is an easy-to-deploy web-based defect tracking tool designed to aid in product defect tracking. It requires PHP, MySQL and a web server. Check out our demo and hosting services.

SAP NetWeaver Server Adapter for Eclipse
Integrate Eclipse with SAP NetWeaver application server.

MinGW - Minimalist GNU for Windows
This project is in the process of being migrated to osdn.net/projects/mingw, you can continue to follow us there. MinGW: A native Windows port of the GNU Compiler Collection (GCC), freely distributable import libraries and header files for building native Windows applications; includes extensions to the MSVC runtime to support C99 functionality. All MinGW software can run on 64-bit Windows platforms.

PhpStorm Mac version
The latest (2018.2.1) professional PHP integrated development tool
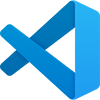
VSCode Windows 64-bit Download
A free and powerful IDE editor launched by Microsoft