


Meta open source text generates a large music model. We tried it with the lyrics of 'Qilixiang'
Before entering the text, let’s listen to two pieces of music generated by MusicGen. We enter the text description "a man walks in the rain, come accross a beautiful girl, and they dance happily"
and then try to enter the first two sentences from the lyrics of Jay Chou's "Qili Xiang" "Out the window" The sparrows are talkative on the telephone poles. What you said makes it feel like summer." (Chinese supported)
Trial address: https://huggingface.co/spaces /facebook/MusicGen
Text-to-music refers to the task of generating musical works given a text description, such as "90s guitar riff rock song." Generating music involves modeling long sequences as a challenging task. Unlike speech, music requires the use of the full spectrum, which means the signal is sampled at a higher rate, i.e. the standard sampling rate for music recordings is 44.1 kHz or 48 kHz, while speech is sampled at 16 kHz.
In addition, music contains the harmony and melody of different instruments, which gives the music a complex structure. But because human listeners are so sensitive to dissonance, they don’t have much tolerance for melodies in generated music. Of course, the ability to control the generation process in multiple ways is essential for music creators, such as keys, instruments, melodies, genres, etc.
Recent advances in self-supervised audio representation learning, sequence modeling, and audio synthesis provide the conditions for developing such models. To make audio modeling easier, recent research proposes to represent audio signals as a stream of discrete tokens that "represent the same signal." This enables high-quality audio generation and efficient audio modeling. However this requires joint modeling of several parallel dependency flows.
Kharitonov et al. [2022], Kreuk et al. [2022] proposed to use a delay method to model multiple streams of speech tokens in parallel, that is, introducing offsets between different streams. Agostinelli et al. [2023] proposed using multiple discrete token sequences of different granularities to represent musical fragments and modeling them using a hierarchy of autoregressive models. Meanwhile, Donahue et al. [2023] adopted a similar approach but targeted the task of singing to accompaniment generation. Recently, Wang et al. [2023] proposed to solve this problem in two stages: restrict modeling to the first token stream. A post-network is then applied to jointly model the remaining flows in a non-autoregressive manner.
In this article, Meta AI researchers propose MUSICGEN, a simple and controllable music generation model that can generate high-quality music given a text description. music.
##Paper address: https: //arxiv.org/pdf/2306.05284.pdf
The researchers proposed a general framework for modeling multiple parallel acoustic token streams as a generalization of previous research ( See Figure 1) below. In order to improve the controllability of generated samples, this paper also introduces unsupervised melody conditions, allowing the model to generate structurally matching music based on given harmony and melody. This paper performs an extensive evaluation of MUSICGEN, and the proposed method outperforms the evaluation baselines by a large margin: MUSICGEN receives a subjective score of 84.8 out of 100, compared to 80.5 for the best baseline. Additionally, this article provides an ablation study that illustrates the importance of each component to overall model performance.
Finally, human evaluation shows that MUSICGEN produces high-quality samples that both conform to the textual description and are also better melodically aligned with the given harmonic structure.
The main contributions of this article are as follows:
- Proposed a simple and efficient model: can produce high-quality music at 32khz. MUSICGEN can generate consistent music with a single-stage language model through an effective codebook interleaving strategy;
- proposes a single model for text and melody conditional generation, and the generated audio is consistent with The melodies provided are consistent and consistent with the textual condition information;
- An extensive objective and manual evaluation of key design choices of the proposed approach was performed.
Method Overview
MUSICGEN contains a decoder based on an autoregressive transformer and is conditioned on a text or melody representation. The (language) model is based on the quantization unit of the EnCodec audio tokenizer, which provides high-fidelity reconstruction from low-frame discrete representations. In addition, compression models deploying residual vector quantization (RVQ) will generate multiple parallel streams. In this setting, each stream consists of discrete tokens from different learned codebooks.
Previous work proposed some modeling strategies to solve this problem. The researchers proposed a novel modeling framework that can be generalized to various codebook interleaving modes. There are also several variations of this framework. Based on patterns, they can take advantage of the internal structure of quantized audio tokens. Finally MUSICGEN supports conditional generation based on text or melody.
Audio tokenization
The researchers used EnCodec, which is a convolutional autoencoder that uses RVQ quantified latent space and adversarial reconstruction losses. Given a reference audio random variable X ∈ R^d·f_s, where d represents the audio duration and f_s represents the sampling rate. EnCodec encodes this variable into a continuous tensor with frame rate f_r ≪ f_s, and then the representation is quantized as Q ∈ {1, . . . , N}^K×d・f_r, where K represents the codebook used in RVQ Quantity, N represents the codebook size.
Codebook interleaved mode
Exact flattened autoregressive decomposition. The autoregressive model requires a discrete random sequence U ∈ {1, . . . , N}^S and sequence length S. By convention, researchers will use U_0 = 0, which is a deterministic special token that represents the beginning of the sequence. They can then model the distribution.
Inexact autoregressive decomposition. Another possibility is to consider autoregressive decomposition, where some codebooks require parallel predictions. For example, define another sequence, V_0 = 0, and t∈ {1, . . . , N}, k ∈ {1, . . . , K}, V_t,k = Q_t,k. When codebook index k is removed (e.g. V_t), this represents the concatenation of all codebooks at time t.
Arbitrary codebook interleaving mode. To experiment with such decompositions and accurately measure the impact of using imprecise decompositions, the researchers introduced a codebook interleaving mode. First consider Ω = {(t, k) : {1, . . . , d・f_r}, k ∈ {1, . . . , K}}, which is the set of all time step and codebook index pairs. The codebook pattern is the sequence P=(P_0, P_1, P_2, . . . , P_S), where P_0 = ∅, and 0
Model conditionalization
Text conditionalization. Given a textual description that matches an input audio
Melody conditioning. While text is the dominant approach to conditional generative models today, a more natural approach to music is to condition on a melodic structure from another audio track or even whistling or humming. This approach also allows for iterative optimization of model outputs. To support this, we attempted to control melodic structure by jointly modulating the input chromatogram and text description. In initial experiments, they observed that conditioning on the original chromatogram often reconstructed the original sample, leading to overfitting. To this end, researchers select major time-frequency bins in each time step to introduce information bottlenecks.
Model architecture
Codebook projection and position embedding. Given a codebook pattern, only some codebooks exist in each pattern step P_s. The researcher retrieves the value from Q corresponding to the index in P_s. Each codebook appears in P_s at most once or not at all.
Transformer decoder. The input is fed into a transformer with L layers and D dimensions, each layer consisting of a causal self-attention block. A cross-attention block is then used, which is provided by the conditioning signal C. When using melodic conditioning, the researcher prefixes the conditional tensor C to the transformer input.
Logits prediction. At pattern step P_s, the output of the transformer decoder is converted into a logits prediction of Q values. Each codebook appears at most once in P_s 1. If the codebook exists, a codebook-specific linear layer is applied from the D channel to N to obtain the logits prediction.
Experimental results
Audio tokenization model. The study uses a non-causal five-layer EnCodec model for 32 kHz mono audio with a stride of 640, a frame rate of 50 Hz, and an initial hidden size of 64 that is doubled for each of the five layers of the model.
Transformer model, studied and trained autoregressive Transformer models of different sizes: 300M, 1.5B, 3.3B parameters.
Training data set. Study using 20,000 hours of licensed music to train MUSICGEN. In detail, the study used an in-house dataset containing 10K high-quality tracks, as well as the ShutterStock and Pond5 music datasets containing 25K and 365K instrumental-only tracks respectively.
Evaluation dataset. The study evaluates the proposed method on the MusicCaps benchmark and compares it with previous work. MusicCaps are composed of 5.5K samples (10 seconds long) prepared by expert musicians and 1K subsets balanced across genres.
Table 1 below gives the comparison of the proposed method with Mousai, Riffusion, MusicLM and Noise2Music. Results show that MUSICGEN outperforms baselines evaluated by human listeners in terms of audio quality and consistency with the provided text description. Noise2Music performs best on FAD on MusicCaps, followed by MUSICGEN trained with text conditions. Interestingly, adding the melody condition degraded the objective metrics, but did not significantly affect the human ratings and was still better than the evaluated baseline.
The researcher uses objective and subjective measures on the given evaluation set, in the text MUSICGEN was evaluated under the same conditions as melody representation. The results are shown in Table 2 below. The results show that MUSICGEN trained with chromatogram conditionalization successfully generates music that follows a given melody, allowing for better control over the generated output. MUSICGEN is robust to dropping chroma at inference time using OVL and REL.
The impact of codebook interleaving mode. We evaluated various codebook patterns using the framework in Section 2.2, K = 4, given by the audio tokenization model. This article reports objective and subjective evaluations in Table 3 below. Although flattening improves generation, it is computationally expensive. Similar performance can be achieved at a fraction of the cost using simple deferral methods.
The effect of model size. Table 4 below reports the results for different model sizes, namely 300M, 1.5B and 3.3B parametric models. As expected, scaling up the model size results in better scores, but only at the expense of longer training and inference times. In terms of subjective evaluation, the overall quality is optimal at 1.5B, but larger models can better understand text prompts.
The above is the detailed content of Meta open source text generates a large music model. We tried it with the lyrics of 'Qilixiang'. For more information, please follow other related articles on the PHP Chinese website!
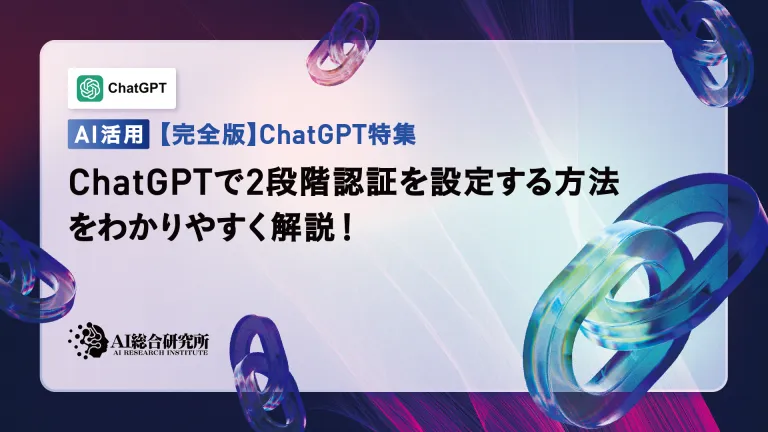
ChatGPT Security Enhanced: Two-Stage Authentication (2FA) Configuration Guide Two-factor authentication (2FA) is required as a security measure for online platforms. This article will explain in an easy-to-understand manner the 2FA setup procedure and its importance in ChatGPT. This is a guide for those who want to use ChatGPT safely. Click here for OpenAI's latest AI agent, OpenAI Deep Research ⬇️ [ChatGPT] What is OpenAI Deep Research? A thorough explanation of how to use it and the fee structure! table of contents ChatG
![[For businesses] ChatGPT training | A thorough introduction to 8 free training options, subsidies, and examples!](https://img.php.cn/upload/article/001/242/473/174704251871181.jpg?x-oss-process=image/resize,p_40)
The use of generated AI is attracting attention as the key to improving business efficiency and creating new businesses. In particular, OpenAI's ChatGPT has been adopted by many companies due to its versatility and accuracy. However, the shortage of personnel who can effectively utilize ChatGPT is a major challenge in implementing it. In this article, we will explain the necessity and effectiveness of "ChatGPT training" to ensure successful use of ChatGPT in companies. We will introduce a wide range of topics, from the basics of ChatGPT to business use, specific training programs, and how to choose them. ChatGPT training improves employee skills
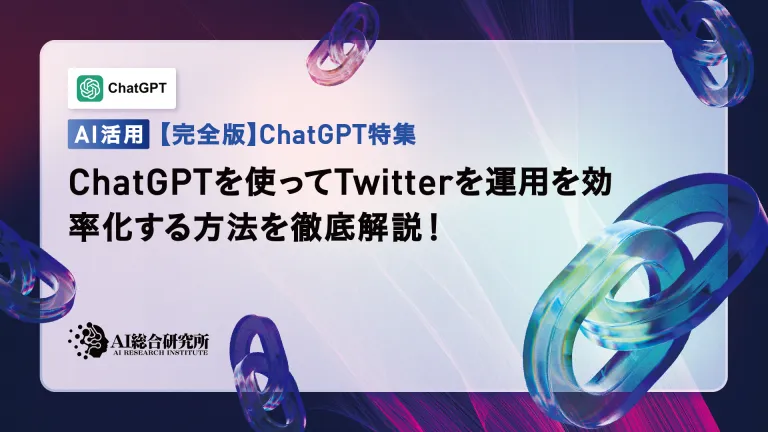
Improved efficiency and quality in social media operations are essential. Particularly on platforms where real-time is important, such as Twitter, requires continuous delivery of timely and engaging content. In this article, we will explain how to operate Twitter using ChatGPT from OpenAI, an AI with advanced natural language processing capabilities. By using ChatGPT, you can not only improve your real-time response capabilities and improve the efficiency of content creation, but you can also develop marketing strategies that are in line with trends. Furthermore, precautions for use
![[For Mac] Explaining how to get started and how to use the ChatGPT desktop app!](https://img.php.cn/upload/article/001/242/473/174704239752855.jpg?x-oss-process=image/resize,p_40)
ChatGPT Mac desktop app thorough guide: from installation to audio functions Finally, ChatGPT's desktop app for Mac is now available! In this article, we will thoroughly explain everything from installation methods to useful features and future update information. Use the functions unique to desktop apps, such as shortcut keys, image recognition, and voice modes, to dramatically improve your business efficiency! Installing the ChatGPT Mac version of the desktop app Access from a browser: First, access ChatGPT in your browser.
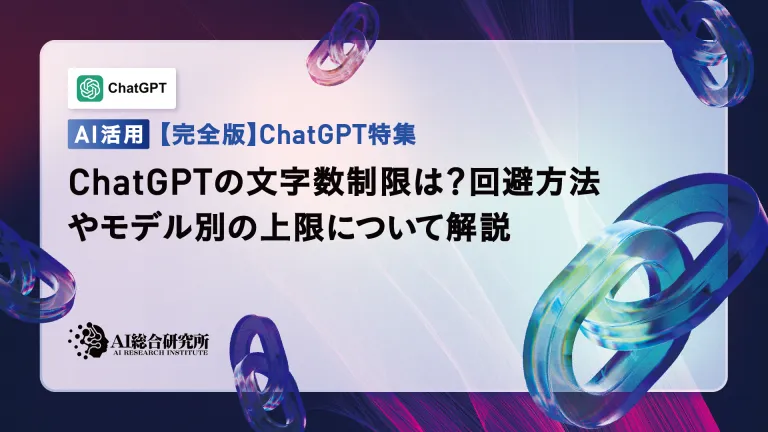
When using ChatGPT, have you ever had experiences such as, "The output stopped halfway through" or "Even though I specified the number of characters, it didn't output properly"? This model is very groundbreaking and not only allows for natural conversations, but also allows for email creation, summary papers, and even generate creative sentences such as novels. However, one of the weaknesses of ChatGPT is that if the text is too long, input and output will not work properly. OpenAI's latest AI agent, "OpenAI Deep Research"
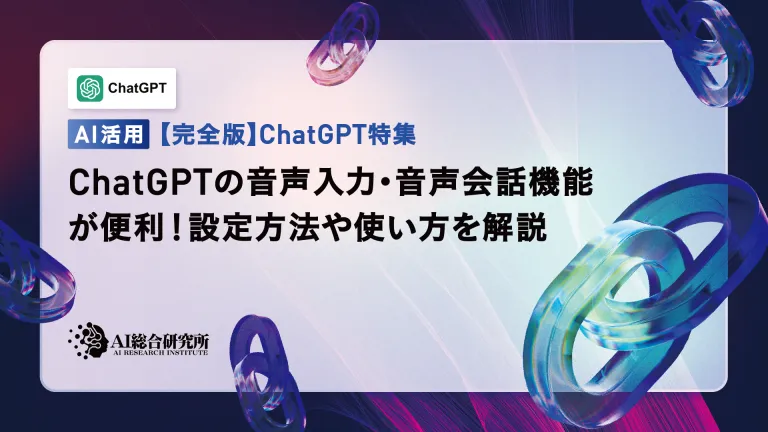
ChatGPT is an innovative AI chatbot developed by OpenAI. It not only has text input, but also features voice input and voice conversation functions, allowing for more natural communication. In this article, we will explain how to set up and use the voice input and voice conversation functions of ChatGPT. Even when you can't take your hands off, ChatGPT responds and responds with audio just by talking to you, which brings great benefits in a variety of situations, such as busy business situations and English conversation practice. A detailed explanation of how to set up the smartphone app and PC, as well as how to use each.
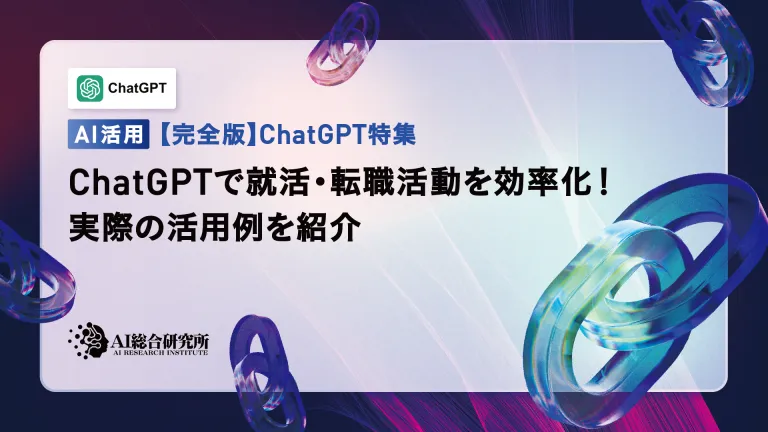
The shortcut to success! Effective job change strategies using ChatGPT In today's intensifying job change market, effective information gathering and thorough preparation are key to success. Advanced language models like ChatGPT are powerful weapons for job seekers. In this article, we will explain how to effectively utilize ChatGPT to improve your job hunting efficiency, from self-analysis to application documents and interview preparation. Save time and learn techniques to showcase your strengths to the fullest, and help you make your job search a success. table of contents Examples of job hunting using ChatGPT Efficiency in self-analysis: Chat
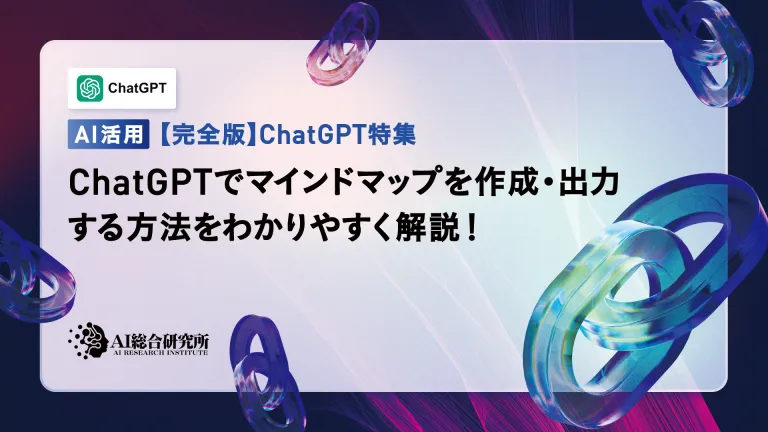
Mind maps are useful tools for organizing information and coming up with ideas, but creating them can take time. Using ChatGPT can greatly streamline this process. This article will explain in detail how to easily create mind maps using ChatGPT. Furthermore, through actual examples of creation, we will introduce how to use mind maps on various themes. Learn how to effectively organize and visualize your ideas and information using ChatGPT. OpenAI's latest AI agent, OpenA


Hot AI Tools

Undresser.AI Undress
AI-powered app for creating realistic nude photos

AI Clothes Remover
Online AI tool for removing clothes from photos.

Undress AI Tool
Undress images for free

Clothoff.io
AI clothes remover

Video Face Swap
Swap faces in any video effortlessly with our completely free AI face swap tool!

Hot Article

Hot Tools

SecLists
SecLists is the ultimate security tester's companion. It is a collection of various types of lists that are frequently used during security assessments, all in one place. SecLists helps make security testing more efficient and productive by conveniently providing all the lists a security tester might need. List types include usernames, passwords, URLs, fuzzing payloads, sensitive data patterns, web shells, and more. The tester can simply pull this repository onto a new test machine and he will have access to every type of list he needs.
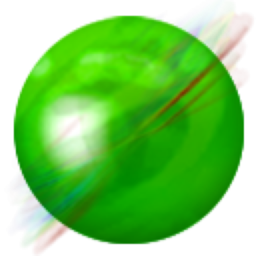
ZendStudio 13.5.1 Mac
Powerful PHP integrated development environment

MantisBT
Mantis is an easy-to-deploy web-based defect tracking tool designed to aid in product defect tracking. It requires PHP, MySQL and a web server. Check out our demo and hosting services.

MinGW - Minimalist GNU for Windows
This project is in the process of being migrated to osdn.net/projects/mingw, you can continue to follow us there. MinGW: A native Windows port of the GNU Compiler Collection (GCC), freely distributable import libraries and header files for building native Windows applications; includes extensions to the MSVC runtime to support C99 functionality. All MinGW software can run on 64-bit Windows platforms.

SublimeText3 Linux new version
SublimeText3 Linux latest version
