Since the popularity of ChatGPT, the research and development of large AI models have emerged one after another, and different types of large AI models have also been launched one after another. There has been a fanatical war of hundreds of models in China.
As a revolutionary data model, ChatGPT brings surprises not only changes in content production methods, but also allows the industry to see the hope of general artificial intelligence, promoting the continuous emergence of large AI models and new applications. According to experts, the focus of future artificial intelligence research will largely shift to large models, and we are ushering in the era of large models.
The widespread application of traditional artificial intelligence is composed of three elements: computing power, data and algorithms. However, with the advent of the era of general artificial intelligence (AGI), massive data requires a lot of training and optimization to achieve higher prediction accuracy and generalization capabilities, and the demand for computing power is no longer the same.
As large models are in full swing, the huge "gap" between supply and demand for computing power is still widening. How to solve the "anxiety" of computing power has become a new goal for the industry.
The computing power gap is huge
To continuously optimize large models based on the Transformer structure, an increasingly large number of parameters are required to be "fed". Due to the increase in the magnitude of training parameters, the demand for computing power is also increasing, and the computing power cluster is changing. got bigger and bigger.
Taking ChatGPT as an example, in terms of computing power alone, OpenAI built a huge computing power cluster composed of nearly 30,000 NVIDIA V100 graphics cards in order to train ChatGPT. Calculated with half-precision FP16, this is a computing power cluster of nearly 4000P.
According to reports, the parameter scale of GPT-4 has reached the 100 billion level, and the corresponding computing power requirements have also increased significantly. The data shows that the number of large model parameters increases in proportion to the square of the computing power.
With the mushrooming of companies devoted to large AI models, coupled with the artificial intelligence ecosystem surrounding large models and the resulting demand for computing power for inference, the computing power gap in the future will be even more alarming.
Obviously, whether the computing power is sufficient will determine the success or failure of each company's large model products. Zou Yi, president of Tianshu Zhixin Product Line, believes that for leading companies, early GPT large models require about 10,000 NVIDIA GPUs, but iterative large models may require at least thousands of state-of-the-art GPUs to complete. With the emergence of many followers in this field, following companies must not lose to leading companies in terms of computing power, and even need to invest more in computing power infrastructure to catch up.
Ding Yunfan, Vice President of System Architecture of Biren Technology, delivered a speech on "Creating a Domestic Large Model Training System Based on High-Performance General-Purpose GPU" at the Beijing Zhiyuan Conference, a grand gathering of the artificial intelligence industry. He pointed out that the success factor of ChatGPT lies in the engineering and algorithm. For collaborative innovation, data is both fuel and foundation, especially high-quality data; algorithms play the role of engines; computing power is an accelerator, involving not only large GPU clusters, but also storage and network clusters.
Due to the ban, the main demand in the domestic market is the A800 and H800 launched by NVIDIA for the Chinese market. As demand continues to expand, the price of NVIDIA A800 has increased alarmingly, and the delivery cycle has also been lengthened, and even some new orders "may It won’t be delivered until December.”
Fortunately, many domestic companies have taken the lead in the field of general-purpose GPUs, and are also making progress in the fields of chip mass production, ecological construction, and application expansion. With the rise of the epoch-making AIGC industry, new trends are also ushering in. market space.
Requires computing power, software and hardware integration
Although we are facing unprecedented new business opportunities, we must seize the opportunity of the rise of large AI models and understand the computing power requirements that truly support large models from the bottom up.
In this regard, Zou Yi said that starting from the model, computing framework layer and operator acceleration, the computing power must meet three major elements. First, it is universal and can support rapid deformation of the model, rapid support of new operators, and rapid support of new operators. communication; second, it is easy to use, it can be implemented using existing algorithm modules, and tuning experience can be used for reference; third, it is easy to use, it can reconstruct parallel computing, full memory access exchange, full computing interconnection, etc.
To realize these three major elements, there is actually a more essential logic behind it. As Kunlun Core R&D Director Luo Hang said bluntly, domestic computing power industrialization must pass through three narrow gates: First, mass production, which can greatly dilute the huge investment in early tape-out, and diluting costs through mass production is the only way to achieve profitability. , is also one of the indicators to measure the maturity of chips; the second is ecology, in order to allow customers to better develop applications, we must strive to build a reasonable and suitable software ecosystem; the third is the integration of software and hardware into products, which must be combined with vertical industries to achieve Product value delivery.
In addition, in order to further support the massive expansion of training parameters, not only GPU manufacturers must have the ability to build thousands to tens of thousands of GPU card training clusters, but also must ensure that they can work continuously without failure during the training process. Stability and reliability create extremely stringent requirements. At the same time, it must also support scalable elastic capabilities to achieve elastic scalability of computing power.
According to Ding Yunfan’s summary, when training large models containing hundreds of billions of parameters, customers are most concerned about storability and scalability. In addition to this, customers also require models that are easy to use, fast and cost-effective.
It is worth mentioning that in order to fully support the development of large models, domestic manufacturers including Cambrian, Kunlun Core, Suiyuan, Biren, Tianshu Zhixin, Muxi, Moore Thread and other manufacturers are also focusing on underlying technical support. A lot of homework has been done to improve chip performance by maximizing data reuse, scalable large matrix calculations and asynchronous storage and calculation, and mixed-precision Transformer acceleration. At the same time, we continue to improve our capabilities in basic software.
"In addition to focusing on GPU computing power, cost and other aspects, Biren also provides strong support through multi-dimensional access: first, the cluster scale can be expanded on demand, and multiple data planes can communicate in parallel to reduce conflicts; second, it can access multiple A machine learning framework that performs flexible scheduling with data parallelism, supports automatic fault tolerance and expansion and contraction, greatly speeds up training, improves efficiency, and supports task migration across switches, reduces resource fragmentation under a single switch, improves resource utilization, and guarantees tasks. Stability." Ding Yunfan said.
Ecology is the key to the future
Looking at a deeper level, the training of domestic large models is inseparable from the support of GPUs with large computing power, and it also requires the construction of a mature industrial ecosystem that integrates software and hardware with full stack coverage.
Training large AI models can be regarded as a systematic project that tests comprehensive upgrades. Ding Yunfan said that this project involves high-performance computing clusters, machine learning platforms, acceleration libraries, training frameworks, and inference engines. It requires distributed hardware including CPU, storage, and communication to support efficient interconnection and integrate with the training framework. The process achieves comprehensive collaboration while achieving parallel expansion, stability and reliability.
It is precisely because of this demand that domestic GPUs must adapt to large model training systems from a perspective that is not limited to computing power. How to provide one-stop large model computing power and enabling services for industry partners is the ultimate test. , so ecological construction is also crucial.
Around this demand, some domestic GPU manufacturers have already taken the lead and are committed to creating full-stack large-model solutions integrating software and hardware, including high-performance infrastructure based on large computing power chips, intelligent scheduling and management of thousands of The GPU card’s machine learning platform, high-performance operator library and communication library, as well as the training framework that is compatible and adaptable to mainstream large models, continue to make efforts.
In order to jointly promote the collaborative innovation of computing power and large model applications, the industrial ecology of GPU manufacturers and domestic large models is also accelerating the pace of cooperation.
It is reported that Biren Technology has successively joined Zhiyuan Research Institute’s FlagOpen (Feizhi) large model technology open source system and Zhiyuan Research Institute’s “AI Open Ecological Laboratory” project, and has carried out in the field of AI large model software and hardware ecological construction a series of collaborations. The Ministry of Science and Technology's Science and Technology Innovation 2030 "New Generation Artificial Intelligence" major project - "Artificial Intelligence Basic Model Support Platform and Evaluation Technology" in which both parties participated has made important progress.
In addition, Biren Technology also participated in the construction and joint release of the "Fei Paddle AI Studio Hardware Ecological Zone", hoping to work closely with many ecological partners including Baidu Fei Paddle, combining China's AI framework and AI computing power The advantages provide a strong driving force for the development of China's AI industry.
According to reports, Tianshu Zhixin’s general-purpose GPU products widely support various large model frameworks such as DeepSpeed, Colossal, and BM Train. The computing power cluster solution based on them also effectively supports mainstream AIGC models such as LLaMa, GPT-2, and CPM. The model's Pretrain and Finetune are also adapted to open source projects of multiple domestic research institutions, including Tsinghua University, Zhiyuan University, and Fudan University.
Looking to the future, the demand for large AI models will continue to rise. How domestic GPU manufacturers continue to iterate products, upgrade computing power solutions, and adapt to large models that support more efficient and complex algorithms will remain a lasting test. .
[Source: Jiweiwang]
The above is the detailed content of The rise of large AI models tests the computing power of domestic GPUs. For more information, please follow other related articles on the PHP Chinese website!
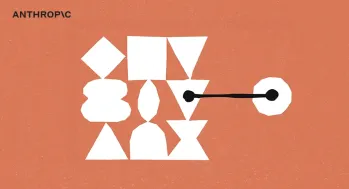
The Model Context Protocol (MCP): A Universal Connector for AI and Data We're all familiar with AI's role in daily coding. Replit, GitHub Copilot, Black Box AI, and Cursor IDE are just a few examples of how AI streamlines our workflows. But imagine
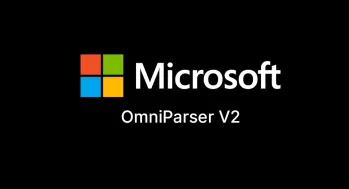
Microsoft's OmniParser V2 and OmniTool: Revolutionizing GUI Automation with AI Imagine AI that not only understands but also interacts with your Windows 11 interface like a seasoned professional. Microsoft's OmniParser V2 and OmniTool make this a re
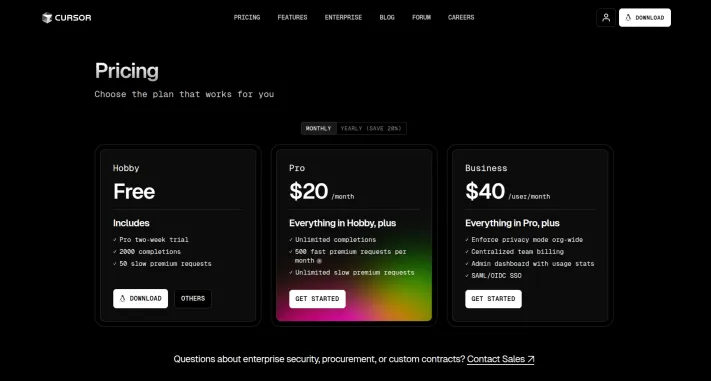
Vibe coding is reshaping the world of software development by letting us create applications using natural language instead of endless lines of code. Inspired by visionaries like Andrej Karpathy, this innovative approach lets dev

Revolutionizing App Development: A Deep Dive into Replit Agent Tired of wrestling with complex development environments and obscure configuration files? Replit Agent aims to simplify the process of transforming ideas into functional apps. This AI-p
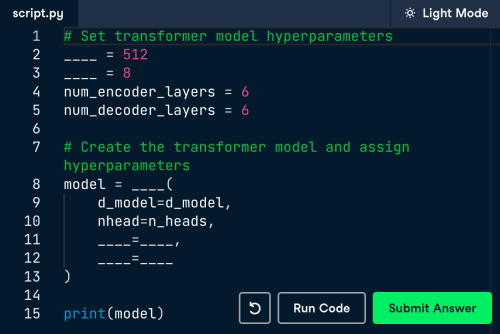
This blog post shares my experience testing Runway ML's new Act-One animation tool, covering both its web interface and Python API. While promising, my results were less impressive than expected. Want to explore Generative AI? Learn to use LLMs in P
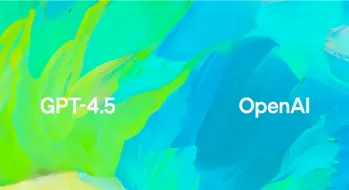
February 2025 has been yet another game-changing month for generative AI, bringing us some of the most anticipated model upgrades and groundbreaking new features. From xAI’s Grok 3 and Anthropic’s Claude 3.7 Sonnet, to OpenAI’s G
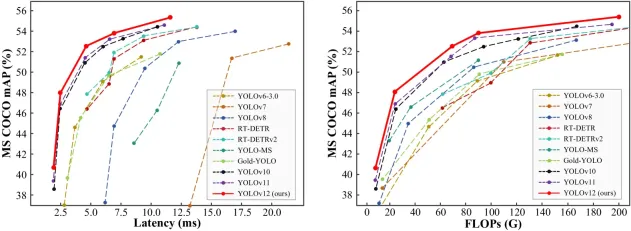
YOLO (You Only Look Once) has been a leading real-time object detection framework, with each iteration improving upon the previous versions. The latest version YOLO v12 introduces advancements that significantly enhance accuracy
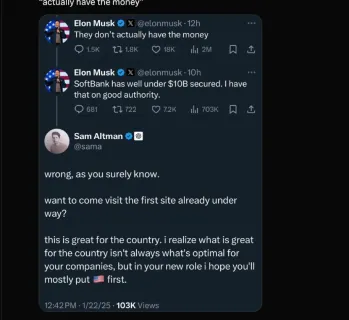
The $500 billion Stargate AI project, backed by tech giants like OpenAI, SoftBank, Oracle, and Nvidia, and supported by the U.S. government, aims to solidify American AI leadership. This ambitious undertaking promises a future shaped by AI advanceme


Hot AI Tools

Undresser.AI Undress
AI-powered app for creating realistic nude photos

AI Clothes Remover
Online AI tool for removing clothes from photos.

Undress AI Tool
Undress images for free

Clothoff.io
AI clothes remover

AI Hentai Generator
Generate AI Hentai for free.

Hot Article

Hot Tools

mPDF
mPDF is a PHP library that can generate PDF files from UTF-8 encoded HTML. The original author, Ian Back, wrote mPDF to output PDF files "on the fly" from his website and handle different languages. It is slower than original scripts like HTML2FPDF and produces larger files when using Unicode fonts, but supports CSS styles etc. and has a lot of enhancements. Supports almost all languages, including RTL (Arabic and Hebrew) and CJK (Chinese, Japanese and Korean). Supports nested block-level elements (such as P, DIV),

SublimeText3 Linux new version
SublimeText3 Linux latest version

Notepad++7.3.1
Easy-to-use and free code editor

PhpStorm Mac version
The latest (2018.2.1) professional PHP integrated development tool
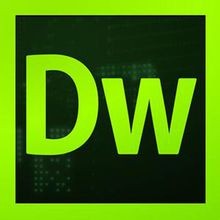
Dreamweaver CS6
Visual web development tools
