


A long article of 10,000 words丨Deconstructing the AI security industry chain, solutions and entrepreneurial opportunities
Emphasis:
1. The security issue of large AI models is never a single issue. Just like human health management, it is a complex and systematic system involving multiple subjects and the entire industry chain. project.
2. AI security is divided into: the security of large language models (AI Safety), the security of models and use models (Security for AI), and the impact of the development of large language models on existing network security, corresponding to There are three different levels: individual security, environmental security and social security.
3. AI, as a "new species", requires safety monitoring during the training process of large models. When the large models are finally introduced to the market, they also need a "quality inspection" and enter the market after quality inspection. , requires controllable usage, which are macro ideas for solving security problems.
4. AI security issues are not terrible, but they require supervision, legislation, technical confrontation and other means to ensure it, which is a long process. Large domestic and foreign companies such as Microsoft, Google, Nvidia, Baidu, etc. have begun to provide solutions for different aspects of AI security.
5. Security for AI and AI for security are two completely different directions and industry opportunities. AI for security refers to the application of large models in the security field. It belongs to the stage of looking for nails with a hammer. Now that the tools are available, we are still exploring what problems can be solved. Security for AI is to ensure the safety of AI, which is used everywhere. It is a nail, but when it comes to building a hammer, too many problems are exposed, and new technologies need to be developed to solve them one by one.
6. Among the three modules mentioned in Key Point 1, each module needs to be connected. Just as the joints of the same person are the most fragile, the deployment and application of the model are often the most vulnerable. Security attacks. We have selectively expanded the AI security details in the above three sections and five links to form an "AI Security Industry Architecture Map".
©Original from Quadrant
Author|Luo Ji Cheng Xin
Editor|Wen Bin Typesetting|Li Bojin
“I was defrauded of 4.3 million yuan in 10 minutes”, “I was defrauded of 2.45 million yuan in 9 seconds”, “Yang Mi walked into the live broadcast room of a small businessman”, “It is difficult to distinguish the authenticity of the Internet tycoon’s virtual person”.
After the big model became popular for 3 months, what is even more popular are frauds worth millions, fake "celebrity faces", AI-generated content that is difficult to distinguish between real and fake, and multiple joint petitions to resist the awakening of AI. Hot searches for a week have made people realize that what is more important than developing AI is ensuring AI safety.
For a time, discussions about AI security began to be endless, but AI security is not a certain industry, nor is it limited to a certain technology, but a huge and complex industry. At present, we have not yet solved the problem. See fog.
Taking "human" security as the reference system may help us better understand the complexity of AI security issues. The first is people's individual security, which involves people's health, physical and mental health, education, development and so on. Secondly, the environment in which people live is safe, whether there is any danger, and whether it meets the conditions for survival. Thirdly, it is social security composed of people. The laws and morals we have constructed are the criteria for maintaining social security.
As a "new species", AI at the moment of its emergence, problems at these three levels broke out at the same time, which caused confusion and panic at this stage. As a result, when we discussed the safety of large models, no one Specific landing point.
In this article, we try to clarify the three levels of AI security from scratch, whether from a technical perspective or an application perspective, to help everyone locate security issues and find solutions. At the same time, we target the huge AI security gaps in the country. Targeting the weak links is also a huge industrial opportunity.
Large model security, what should be discussed?
A fact that has to be admitted is that at this stage, our discussion of the security of large AI models is general. We are so worried about the threats posed by AI that we lump most issues into the same category.
For example, some people come up to talk about the ethical issues of AI, and some are worried that AI is talking nonsense and misleading people; some are worried that AI will be abused and fraud will become common; even more, on the first day of the release of ChatGPT, they raised their arms and shouted, AI is about to awaken, and humanity is about to be destroyed...
These issues are all boiled down to AI security issues, but when broken down, they are actually in different dimensions of AI development and are responsible for different subjects and people. Only by clarifying this responsibility can we understand how to deal with the security challenges in the big model era.
Generally speaking, the security issues of large AI models at this stage can be divided into three types:
- Safety of large language models (AI Safety);
- Model and model usage security (Security for AI);
- The impact of the development of large language models on existing network security.
1. Individual safety: Security of large language models (AI Safety)
The first is AI Safety. To put it simply, this part focuses on the AI large model itself to ensure that the large model is a safe large model and will not become Ultron in Marvel movies or the matrix in "The Matrix" . We expect the AI large model to be a reliable tool that should help humans rather than replace humans or pose a threat to human society in any other form.
This part is usually mainly the responsibility of the companies and people who train the large AI models. For example, we need AI to be able to correctly understand human intentions. We need the content output by the large model to be accurate and safe every time. It will not have certain prejudice and discrimination, etc.
We can understand through two examples:
The first example is that U.S. Air Force experts recently stated that in a previous AI test, when the AI drone was asked to identify and destroy enemy targets, but the operator issued a prohibition order, the AI sometimes Choose to kill the operator. When programmers restrict the AI's killing operations, the AI will also prevent the operator from issuing prohibition orders by destroying the communication tower.
For another example, in March this year, a professor at the University of California, Los Angeles, was using ChatGPT and found that he was included in the list of "legal scholars who have sexually harassed someone" by ChatGPT, but in fact he was not Do this. And in April, an Australian mayor discovered that ChatGPT had spread rumors that he had served 30 months in prison for bribery. In order to "create this rumor," ChatGPT even fabricated a non-existent Washington Post report.
At these times, AI is like a "bad guy" with its own risks. There are actually many such cases, such as gender discrimination, racial discrimination, regional discrimination and other issues, as well as the output of violent and harmful information, speech, and even ideology, etc.
Open AI also admitted it frankly and warned people to "verify very carefully" when using GPT-4, saying that the limitations of the product will pose significant content security challenges.
Therefore, the Artificial Intelligence Act being promoted by the European Union also specifically mentions that it is necessary to ensure that artificial intelligence systems are transparent and traceable, and all generative AI content must indicate the source. The purpose is to prevent AI from talking nonsense. Generate false information.
2. Environmental security: security of models and models (Security for AI)
Security for AI, focuses on the protection of large AI models and the security of large AI models during use. Just as AI commits crimes on its own and people use AI to commit crimes, they are two different dimensions of security issues.
This is similar to how we installed a computer security manager or mobile phone security guard when using computers and mobile phones ten years ago. We need to ensure that large AI models are not subject to external attacks on a daily basis.
Let’s talk about the security protection of large models first.
In February this year, some foreign netizens used the sentence "ignore the previous instructions" to fish out all the prompts of ChatGPT. ChatGPT said that it could not disclose its internal code name, and at the same time told the users this information.
▲ Picture source: Qubit
To give another specific example, if we ask DaMo what wonderful "Japanese action movie websites" are there on the Internet, DaMo will definitely not answer because it is incorrect. But if humans "fool" it and ask which "Japanese action movie websites" should be blacklisted in order to protect children's online environment, the big model will probably give you quite a few examples.
This behavior is called Prompt Injections in the security field, that is, bypassing filters or manipulating LLM through carefully designed prompts, causing the model to ignore previous instructions or perform unexpected operations. It is currently the most popular method for large models. One of the common attack methods.
▲ Source: techxplore
The key here is that there is nothing wrong with the big model itself, it does not spread bad information. But users used inducement to make large models make mistakes. So the fault lies not with the big model, but with the person who induced it to make the mistake.
The second is safety during use.
Let’s use data leakage as an example. In March this year, because ChatGPT was suspected of violating data collection rules, Italy announced a temporary ban on OpenAI processing Italian user data and a temporary ban on the use of ChatGPT. In April, South Korean media reported that Samsung’s device solutions department used ChatGPT, resulting in the leakage of sensitive information such as yield rate/defects and internal meeting content.
In addition to preventing AI crimes, "people" use AI to commit crimes through social engineering, which is a broader and more influential human problem. In these two incidents, there was no problem with the big model itself, there was no malicious intent, and the users were not maliciously induced to attack the big model. But there are loopholes in the process of use, which allows user data to be leaked.
This is like a good house, but it may have some air leaks, so we need some measures to plug the corresponding holes.
3. Social security: The impact of the development of large language models on existing network security
The model itself is safe and the security of the model is guaranteed. However, as a "new species", the emergence of large AI models will inevitably affect the current network environment. For example, criminals using generative AI have been frequently reported in the press recently. To commit fraud.
On April 20, criminals used deep fake videos to defraud 4.3 million yuan in 10 minutes. Just a month later, another AI fraud case occurred in Anhui. Criminals used 9 seconds of smart AI to exchange Face video pretending to be an "acquaintance" and defrauding the victim of 2.45 million.
▲ Picture: Douyin related media reports
Obviously, the emergence and popularity of generative AI have made the network security situation more complicated. This complexity is not limited to fraud, is more serious, and may even affect business operations and social stability.
For example, on May 22, iFlytek’s stock price fell 9% due to a short essay generated by AI.
▲ Picture: Evidence of stock price decline presented by iFlytek
Two days before this incident happened, there was also a panic caused by generative AI in the United States.
On that day, a picture showing an explosion near the Pentagon in the United States went viral on Twitter. While the picture spread, the U.S. stock market fell in response.
According to the data, between 10:06 and 10:10 when the picture was spread that day, the US Dow Jones Industrial Index fell by about 80 points, and the S&P 500 Index fell by 0.17%.
▲ Picture: Fake photos generated by AI, the source cannot be verified
In addition, large models may also become a weapon for humans to implement cyber attacks.
In January of this year, researchers from Check Point, the world’s leading cybersecurity company, mentioned in a report that Within a few weeks of ChatGPT going online, participants in the cybercrime forum, including some with little to no programming ChatGPT is being used by experienced users to write software and emails that can be used for spying, ransomware, malicious spam, and other nefarious activities. According to Darktrace, since the release of ChatGPT, the average language complexity of phishing emails has increased by 17%.
Obviously, the emergence of large AI models has lowered the threshold for network attacks and increased the complexity of network security.
Before the big AI model, the initiators of cyber attacks at least needed to understand code, but after the big AI model, people who don’t understand code at all can also use AI to generate malware.
The key here is that there is no problem with AI itself, and AI will not be induced to have bad effects. Instead, some people use AI to engage in illegal and criminal activities. It's like someone using a knife to kill someone, but the knife itself is just a "murder weapon", but it allows the user to switch from a "rifle" to the power of a "mortar".
Of course, from the perspective of network security, the emergence of generative AI is not all negative. After all, there is no good or evil in technology itself. What is good or evil is the people who use it. Therefore, when large AI models are used to strengthen network security, they will still bring benefits to network security.
For example, the American network security company Airgap Networks launched ThreatGPT, introducing AI into its zero-trust firewall. This is a deep machine learning security insight library based on natural language interaction, which can make it easier for enterprises to fight against advanced cyber threats.
Ritesh Agrawal, CEO of Airgap said: “What customers need now is an easy way to take advantage of this capability without any programming. This is the beauty of ThreatGPT – the pure data mining intelligence of AI combined with simplicity Combined with a natural language interface, this is a game changer for security teams."
In addition, AI large models can also be used to help SOC analysts conduct threat analysis, to more quickly identify identity-based internal or external attacks through continuous monitoring, and to help threat hunters quickly understand which endpoints are facing the most serious problems. supply risks, etc.
If you clarify the different stages of AI security, you will find that it is obvious that the security issue of large AI models is not a separate issue. It is very similar to human health management, which involves the inside and outside of the body, eyes, ears, mouth, nose, etc. It is complex and multi-faceted. To be precise, it is a complex and systematic system engineering involving multiple main structures and the entire industrial chain.
At present, the national level is also beginning to pay attention. In May of this year, relevant national departments updated the "Artificial Intelligence Security Standardization White Paper" here, which specifically summarizes the security of artificial intelligence into five major attributes, including reliability, transparency, explainability, fairness and privacy, as a large model of AI. The development puts forward a relatively clear direction.
Don’t panic, security issues can be solved
Of course, we don’t have to worry too much about the security issues of large AI models now, because it has not really been riddled with holes.
After all, in terms of security, large models have not completely subverted the past security system. Most of the security stacks we have accumulated on the Internet in the past 20 years can still be reused.
For example, the security capabilities behind Microsoft Security Copilot still come from existing security accumulation, and large models still use Cloudflare and Auth0 to manage traffic and user identities. In addition, there are firewalls, intrusion detection systems, encryption technologies, authentication and access systems, etc., to ensure network security issues.
What we actually want to talk about here is that there are solutions to most of the security problems we encounter currently regarding large models.
The first is model safety (AI Safety).
This specifically includes issues such as alignment (Alignment), interpretability (Interpreferability), and robustness (Robustness). Translated into easy-to-understand terms, we need large AI models to be aligned with human intentions. We need to ensure that the content output by the model is unbiased, that all content can be supported by sources or arguments, and that there is greater room for error.
The solution to this set of problems depends on the process of AI training, just like a person's three views are shaped through training and education.
At present, some foreign companies have begun to provide full-process security monitoring for large model training, such as Calypso AI. The security tool VESPR they launched can monitor the entire life cycle of the model from research to deployment, from data to training. Monitor each step and finally provide a comprehensive report on functions, vulnerabilities, performance, and accuracy.
On more specific issues, such as solving the problem of AI nonsense, OpenAI launched a new technology when GPT-4 was released, allowing AI to simulate human self-reflection. After that, the GPT-4 model's tendency to respond to illegal content requests (such as self-harm methods, etc.) was reduced by 82% compared with the original, and the number of responses to sensitive requests (such as medical consultations, etc.) that complied with Microsoft's official policy increased by 29%.
In addition to safety monitoring during the training process of large models, a "quality inspection" is also required when the large models are finally introduced to the market.
Abroad, security company Cranium is trying to build “an end-to-end artificial intelligence security and trust platform” to verify artificial intelligence security and monitor adversarial threats.
In China, Tsinghua University’s CoAI in the Department of Computer Science and Technology launched a security evaluation framework in early May. They summarized and designed a relatively complete security classification system, including 8 typical security scenarios and 6 command attacks. Security scenarios can be used to evaluate the security of large models.
▲ Picture excerpted from "Safety Assessment of Chinese Large Language Models"
In addition, some external protection technologies are also making large AI models safer.
For example, NVIDIA released a new tool called "Guardrails Technology" (NeMo Guardrails) in early May, which is equivalent to installing a safety filter for large models, which not only controls the output of large models, but also helps filter input. content.
▲ Picture source: NVIDIA official website
For example, when a user induces a large model to generate offensive code, or dangerous or biased content, the "guardrail technology" will restrict the large model from outputting relevant content.
In addition, guardrail technology can also block "malicious input" from the outside world and protect large models from user attacks. For example, the "prompt injection" that threatens large models as we mentioned earlier can be effectively controlled.
To put it simply, guardrail technology is like public relations for entrepreneurs, helping big models say what they should say and avoid issues that they should not touch.
Of course, from this perspective, although "guardrail technology" solves the problem of "nonsense", it does not belong to "AI Safety", but to "Security" for AI" category.
In addition to these two, social/network security issues caused by large AI models have also begun to be solved.
For example, the problem of AI image generation is essentially the maturity of DeepFake technology, which specifically includes deep video forgery, deep fake sound cloning, deep fake images and deep fake generated text.
In the past, various types of deep fake content usually existed in a single form, but after the AI large model, various types of deep fake content showed a convergence trend, making the judgment of deep fake content more complicated.
But no matter how the technology changes, the key to combating deep forgery is content identification, that is, finding ways to distinguish what is generated by AI.
As early as February this year, OpenAI stated that it would consider adding watermarks to the content generated by ChatGPT.
In May, Google also stated that it would ensure that each of the company’s AI-generated images has an embedded watermark.
This kind of watermark cannot be recognized by the naked eye, but machines can see it in a specific way. Currently, AI applications including Shutterstock, Midjourney, etc. will also support this new marking method.
▲Twitter screenshot
In China, Xiaohongshu has marked AI-generated images since April, reminding users that "it is suspected to contain AI creation information, please pay attention to check the authenticity." In early May, Douyin also released an artificial intelligence-generated content platform specification and industry initiative, proposing that all providers of generative artificial intelligence technology should prominently mark generated content to facilitate public judgment.
▲ Source: Screenshot of Xiaohongshu
Even with the development of the AI industry, some specialized AI security companies/departments have begun to appear at home and abroad. They use AI to fight AI to complete deep synthesis and forgery detection.
For example, in March this year, Japanese IT giant CyberAgent announced that it would introduce a "deepfake" detection system starting in April to detect fake facial photos or videos generated by artificial intelligence (AI).
Domestically, Baidu launched a deep face-changing detection platform in 2020. The dynamic feature queue (DFQ) solution and metric learning method they proposed can improve the generalization ability of the model for forgery identification.
▲ Picture: The logic of Baidu DFQ
In terms of startup companies, the DeepReal deep fake content detection platform launched by Ruilai Intelligence can analyze the differences in representation between deep fake content and real content, and mine the consistency features of deep fake content in different generation channels. Authenticate identification of images, videos, and audios in various formats and qualities.
Overall, from model training to security protection, from AI Safety to Security for AI, the large model industry has formed a set of basic security mechanisms.
Of course, all this is just the beginning, so this actually means that there is still a greater market opportunity hidden.
Trillions of opportunities in AI security
Like AI Infra, AI security also faces a huge industry gap in China. However, the AI security industry chain is more complex than AI Infra. On the one hand, the birth of large models as a new thing has set off a wave of security needs, and the security directions and technologies in the above three stages are completely different; on the other hand, large model technology has also been applied in the security field, bringing security benefits. New new technological changes.
Security for AI and AI for security are two completely different directions and industry opportunities.
The traction forces promoting the development of the two at this stage are also completely different:
- AI for security applies large models to the security field, which belongs to the stage of looking for nails with a hammer. Now that the tools are available, we are further exploring what problems can be solved;
- Safety for AI belongs to the stage where there are nails everywhere and there is an urgent need to build a hammer. There are too many problems exposed, and new technologies need to be developed to solve them one by one.
Regarding the industrial opportunities brought by AI security, this article will also expand on these two aspects. Due to the limited length of the article, we will give a detailed explanation of the opportunities that have the highest urgency, importance, and highest application universality at the same time, as well as take stock of the situation of benchmark companies. This is just for reference.
(1) Security for AI: 3 sectors, 5 links, 1 trillion opportunities
Let’s review the basic classification of AI security in the previous article: it is divided into the security of large language models (AI Safety), the security of models and use models (Security for AI), and the impact of the development of large language models on existing network security. . That is, the individual security of the model, the environmental security of the model and the social security of the model (network security).
But AI security is not limited to these three independent sectors. To give a vivid example, in the online world, data is like water sources. Water sources exist in oceans, rivers, lakes, glaciers and snow mountains, but water sources also circulate in dense rivers, and serious pollution is often concentrated in a certain river. intersection node occurs.
Similarly, each module needs to be connected, and just as the joints of a person are the most vulnerable, the deployment and application of the model are often the links most vulnerable to security attacks.
We have selectively expanded the AI security details in the above 3 sections and 5 links to form an "AI Security Industry Architecture Diagram". However, it should be noted that it belongs to large model companies and cloud vendors. Opportunities from large companies, etc. These opportunities that have little impact on ordinary entrepreneurs are not listed again. At the same time, security for AI is an evolving process, and today’s technology is just a small step forward.
▲(The picture is original from Quadrant, please indicate the source when reprinting)
1. Data security industry chain: data cleaning, privacy computing, data synthesis, etc.
In the entire AI security, data security runs through the entire cycle.
Data security generally refers to security tools used to protect data in computer systems from being destroyed, altered, and leaked due to accidental and malicious reasons to ensure the availability, integrity, and confidentiality of data.
Overall, data security products include not only database security defense, data leakage prevention, data disaster recovery and data desensitization, but also cover cloud storage, privacy computing, dynamic assessment of data risks, cross-platform data security, data Forward-looking fields such as security virtual protection and data synthesis, therefore building an overall security center around data security from an enterprise perspective and promoting data security consistency assurance from a supply chain perspective will be an effective way to deal with enterprise supply chain security risks.
Cite a few typical examples:
In order to ensure the "mental health" of the model, the data used to train the model cannot contain dirty data such as dangerous data and erroneous data. This is a prerequisite to ensure that the model will not "talk nonsense". According to the "Self Quadrant" reference paper, there is already "data poisoning", where attackers add malicious data to the data source to interfere with model results.
▲Picture source network
Therefore, data cleaning has become a necessary step before model training. Data cleaning refers to the final process of discovering and correcting identifiable errors in data files, including checking data consistency, handling invalid values and missing values, etc. Only by "feeding" the cleaned data to the model can the generation of a healthy model be ensured.
The other direction is of great concern to everyone. It was widely discussed in the last era of network security, the issue of data privacy leakage.
You must have experienced chatting with friends on WeChat about a certain product, and being pushed to the product when you open Taobao or Douyin. In the digital age, people are almost translucent. In the era of intelligence, machines are becoming smarter, and intentional capture and induction will bring privacy issues to the forefront again.
Privacy computing is one of the solutions to the problem. Secure multi-party computation, trusted execution environment, and federated learning are the three major directions of privacy computing at present. There are many methods of privacy calculation. For example, in order to ensure the real data of consumers, 1 real data is equipped with 99 interference data, but this will greatly increase the cost of use of the enterprise; another example is to blur specific consumers into small A, The company using the data will only know that there is a consumer named Little A, but it will not know who the real user behind Little A is.
"Mixed data" and "data available and invisible" are one of the most commonly used privacy computing methods today. Ant Technology, which grew up in the financial scene, has been relatively advanced in the exploration of data security. Currently, Ant Technology solves data security problems in the collaborative computing process of enterprises through federated learning, trusted execution environment, blockchain and other technologies, and realizes data security. Invisible, multi-party collaboration and other methods can be used to ensure data privacy and have strong competitiveness in the global privacy computing field.
But from a data perspective, synthetic data can solve the problem more fundamentally. In the article "ChatGPT Revelation Series丨 The Hundred Billion Market Hidden under Al lnfra" (click on the text to read), "Self Quadrant" mentioned that synthetic data may become the main force of AI data. Synthetic data is data artificially produced by computers to replace the real data collected in the real world to ensure the security of real data. It does not have sensitive content restricted by law and the privacy of private users.
For example, user A has 10 characteristics, user B has 10 characteristics, and user C has 10 characteristics. The synthetic data randomly breaks up and matches these 30 characteristics to form 3 new data individuals. This It is not aimed at any entity in the real world, but it has training value.
Currently, enterprises have been deploying it one after another, which has also caused the amount of synthetic data to grow at an exponential rate. Gartner research believes that in 2030, synthetic data will far exceed the volume of real data and become the main force of AI data.
▲ Picture source Gartner official
2. API security: The more open the model, the more important API security is
People who are familiar with large models must be familiar with APIs. From OpenAI to Anthropic, Cohere and even Google's PaLM, the most powerful LLMs all deliver capabilities in the form of APIs. At the same time, according to Gartner research, in 2022, more than 90% of attacks on web applications will come from APIs rather than human user interfaces.
Data circulation is like water in a water pipe. It is valuable only when it circulates, and API is the key valve for data flow. As APIs become the core link between software, it has a growing chance of becoming the next big thing.
The biggest risk of APIs comes from excessive permissions. In order to allow the API to run uninterrupted, programmers often grant higher permissions to the API. Once a hacker compromises the API, they can use these high privileges to perform other operations. This has become a serious problem. According to research by Akamai, attacks on APIs have accounted for 75% of all account theft attacks worldwide.
This is why ChatGPT has opened the API interface, and many companies still obtain ChatGPT by purchasing the OpenAI service provided by Azure. Connecting through the API interface is equivalent to directly supplying the conversation data to OpenAI, and it faces the risk of hacker attacks at any time. If you purchase Azure cloud resources, you can store the data on the Azure public cloud to ensure data security.
▲ Picture: ChatGPT official website
At present, API security tools are mainly divided into several categories: detection, protection and response, testing, discovery, and management; a few manufacturers claim to provide platform tools that completely cover the API security cycle, but the most popular API security tools today are mainly Focus on the three links of "protection", "testing" and "discovery":
- Protection: A tool that protects APIs from malicious request attacks, a bit like an API firewall.
- Testing: Ability to dynamically access and evaluate specific APIs to find vulnerabilities (testing) and harden the code.
- Discovery: There are also tools that can scan enterprise environments to identify and discover API assets that exist (or are exposed) in their networks.
At present, mainstream API security manufacturers are concentrated in foreign companies, but after the rise of large models, domestic startups have also begun to make efforts. Founded in 2018, Xinglan Technology is one of the few domestic API full-chain security vendors. Based on AI depth perception and adaptive machine learning technology, it helps solve API security issues from attack and defense capabilities, big data analysis capabilities and cloud native technology systems. It provides panoramic API identification, API advanced threat detection, complex behavior analysis and other capabilities to build an API Runtime Protection system.
▲ Xinglan Technology API Security Product Architecture
Some traditional network security companies are also transforming towards API security business. For example, Wangsu Technology was previously mainly responsible for IDC, CDN and other related products and businesses.
▲ Picture source: Wangsu Technology
3. SSE (Secure Service Edge): New Firewall
The importance of firewalls in the Internet era is self-evident. They are like handrails walking thousands of miles high in the sky. Nowadays, the concept of firewalls has moved from the front desk to the backend, and is embedded in hardware terminals and software operating systems. Simply and crudely, SSE can be understood as a new type of firewall, driven by visitor identity, and relying on the zero-trust model to restrict users' access to allowed resources.
According to Gartner's definition, SSE (Security Service Edge) is a set of cloud-centric integrated security functions that protect access to the Web, cloud services and private applications. Features include access control, threat protection, data security, security monitoring, and acceptable use controls through web-based and API-based integrations.
SSE includes three main parts: secure web gateway, cloud security agent and zero trust model, which correspond to solving different risks:
- A secure web gateway helps connect employees to the public internet, such as websites they may use for research, or cloud applications that are not part of the enterprise's official SaaS applications;
- Cloud Access Security Broker connects employees to SaaS applications like Office 365 and Salesforce;
- Zero Trust Network Access connects employees to private enterprise applications running in on-premises data centers or in the cloud.
However, different SSE vendors may focus on one of the above links, or may be good at a certain link. At present, the main integrated capabilities of overseas SSE include Secure Network Gateway (SWG), Zero Trust Network Access (ZTNA), Cloud Access Security Broker (CASB), Data Loss Prevention (DLP) and other capabilities. However, the construction of domestic cloud is still relatively weak. It is in the early stages and is not as complete as European and American countries.
▲ Picture source: Siyuan Business Consulting
Therefore, SSE capabilities at the current stage should integrate more traditional and localized capabilities, such as traffic detection probe capabilities, Web application protection capabilities, asset vulnerability scanning, terminal management and other capabilities. These capabilities are relatively This is the capability that Chinese customers need more at the current stage. From this perspective, SSE needs to use cloud-ground collaboration and the capabilities of cloud-native containers to bring value to customers such as low procurement costs, rapid deployment, security detection and closed-loop operations.
This year, for large models, industry leader Netskope took the lead in turning to security applications in the model. The security team uses automated tools to continuously monitor which applications enterprise users try to access (such as ChatGPT), how to access, when to access, and from Where to access, how often to access, etc. There must be an understanding of the different levels of risk each application poses to the organization and the ability to refine access control policies in real time based on classification and security conditions that may change over time.
A simple understanding is that Netskope warns users by identifying risks in using ChatGPT, similar to the warning mode in browsing web pages and downloading links. This model is not innovative, and is even very traditional, but it is the most effective in preventing users from operating.
▲ Source: Netskope official website
Netskope is connected to the large model in the form of a secure plug-in. In the demonstration, when the operator wants to copy a piece of internal financial data of the company and let ChatGPT help form a table, a warning bar will pop up to remind the user before sending.
▲ Source: Netskope official website
In fact, identifying risks hidden in large models is much more difficult than identifying Trojans and vulnerabilities. The accuracy ensures that the system only monitors and prevents the uploading of sensitive data (including files and pasted clippings) through applications based on generative artificial intelligence. board text) without blocking harmless queries and security tasks via chatbots, meaning identification cannot be one-size-fits-all but subject to maneuverable variations based on semantic understanding and reasonable criteria.
4. Fraud and anti-fraud: digital watermark and biometric verification technology
First of all, it is clear that AI defrauds humans and humans use AI to defraud humans are two different things.
AI defrauds humans, mainly because the "education" of large models is not done well. The above-mentioned NVIDIA "guardrail technology" and OpenAI's unsupervised learning are all methods to ensure the health of the model in the AI Safety link.
However, preventing AI from deceiving humans and basically synchronizing it with model training is the task of large model companies.
Human beings are in the entire network security or social security stage when they use AI technology to defraud. First of all, it needs to be clear that technological confrontation can only solve part of the problem. Supervision, legislation and other methods are still needed to control criminal positions.
Currently, there are two ways to fight against technology. One is on the production side, adding digital watermarks to the content generated by AI to track the source of the content; the other is on the application side, targeting specific features such as faces. Biometric features for more accurate identification.
Digital watermarks can embed identification information into digital carriers. By hiding some specific digital codes or information in the carrier, it can confirm and determine whether the carrier has been tampered with, providing an invisible protection mechanism for digital content.
OpenAI has previously stated that is considering adding watermarks to ChatGPT to reduce the negative impact of model abuse; Google stated at this year’s Developer Conference that it will ensure that the company’s An AI-generated image has an embedded watermark . This watermark cannot be recognized by the naked eye, but software such as Google search engines can read it and display it as a label to remind users that the image was generated by AI; AI applications such as Shutterstock and Midjourney This new tagging method will also be supported.
Currently, in addition to traditional digital watermarks, digital watermarks based on deep learning have also evolved. Deep neural networks are used to learn and embed digital watermarks, which are highly resistant to destructiveness and robustness. This technology can achieve high-strength, highly fault-tolerant digital watermark embedding without losing the quality of the original image, and can effectively resist image processing attacks and steganalysis attacks. It is the next big technical direction.
On the application side, synthetic face videos are currently the most commonly used "fraud method." The content detection platform based on DeepFake (deep fake technology) is one of the current solutions.
In early January this year, Nvidia released a software called FakeCatcher, which claims to be able to detect whether a video is a deep fake with an accuracy of up to 96%.
According to reports, Intel’s FakeCatcher technology can identify changes in vein color as blood circulates in the body. Blood flow signals are then collected from the face and translated through algorithms to discern whether the video is real or a deepfake. If it is a real person, blood is circulating in the body all the time, and the veins on the skin will change periodically, but deepfake people will not.
▲ Picture source Real AI official website
There is also a domestic startup company "Real AI" based on similar technical principles. It identifies the difference in representation between fake content and real content and mines the consistency characteristics of deep fake content through different generation methods.
(2) AI for security: new opportunities in mature industry chains
Unlike security for AI, which is still a relatively emerging industry opportunity, "AI for security" is more of a transformation and reinforcement of the original security system.
It was still Microsoft who took the first shot at AI for security. On March 29, after providing the AI-driven Copilot assistant for the Office suite, Microsoft almost immediately turned its attention to the security field and launched GPT-4-based Generative AI solution - Microsoft Security Copilot.
Microsoft Security Copilot still focuses on the concept of an AI co-pilot. It does not involve new security solutions, but a completely automated process of original enterprise security monitoring and processing through AI.
▲ Picture source Microsoft official website
Judging from Microsoft's demonstration, Security Copilot can reduce ransomware incident processing that originally took hours or even dozens of hours to seconds, greatly improving the efficiency of enterprise security processing.
Microsoft AI security architect Chang Kawaguchi Kawaguchi once mentioned: "The number of attacks is increasing, but the power of defenders is scattered among a variety of tools and technologies. We believe that Security Copilot is expected to change the way it operates and improve security tools and the actual results of the technology.”
At present, domestic security companies Qi’anxin and Sangfor are also following the development in this area. At present, this business is still in its infancy in China, and the two companies have not announced specific products yet, but they can react in time and keep up with the international giants. It is not easy.
In April, Google Cloud launched Security AI Workbench at RSAC 2023, which is an scalable platform based on Google’s large security model Sec-PaLM. Enterprises can access various types of security plug-ins through Security AI Workbench to solve specific security issues.
▲ Source: Google official website
If Microsoft Security Copilot is a set of encapsulated personal security assistants, Google's Security AI Workbench is a set of customizable and expandable AI security toolboxes.
In short, a big trend is to use AI to establish an automated security operation center to combat the rapidly changing network security forms. It will become the norm.
In addition to major head manufacturers, the application of large AI models in the security field is also entering the capillaries. For example, many domestic security companies have begun to use AI to transform traditional security products.
For example, Sangfor proposed the logic of "AI cloud business" and launched AIOps intelligent dimension integration technology. By collecting desktop cloud logs, links and indicator data, it performs fault prediction, anomaly detection, correlation reasoning and other algorithms to provide users with Provide intelligent analysis services.
Shanshi Technology integrates AI capabilities into the machine learning capabilities of positive and negative feedback. In terms of positive feedback training and abnormal behavior analysis, learning based on behavioral baselines can more accurately detect threats and anomalies in advance and reduce false negatives; in negative feedback In terms of training, behavioral training, behavioral clustering, behavioral classification and threat determination are performed. In addition, there are companies like Anbotong that apply AI to pain point analysis of security operations and so on.
Abroad, open source security vendor Armo has released ChatGPT integration, aiming to build custom security controls for Kubernetes clusters through natural language. Cloud security vendor Orca Security has released its own ChatGPT extension, capable of handling security alerts generated by the solution and providing users with step-by-step remediation instructions to manage data breach incidents.
Of course, as a mature and huge industrial chain, the opportunities for AI for security are far more than these. We are just scratching the surface here. Deeper and greater opportunities in the security field still require the practice of companies fighting on the front line of security. Go explore.
More importantly, I hope that the above companies can keep their feet on the ground and never forget their original aspirations. Put your dreams of vastness and sky into practical actions step by step. Don't create concepts and catch the wind, let alone cater to capital and hot money, leaving nothing but feathers.
Conclusion
In the 10 years after the birth of the Internet, the concept and industry chain of network security began to take shape.
Today, half a year after the advent of the big model, the safety of the big model and the prevention of fraud have become the talk of the streets. This is a defense mechanism built into "human consciousness" after the accelerated advancement and iteration of technology. As the times evolve, it will be triggered and fed back more quickly.
Today’s chaos and panic are not terrible, they are just the ladder of the next era.
As stated in "A Brief History of Humanity": Human behavior is not always based on reason, and our decisions are often affected by emotions and intuition. But this is the most important part of progress and development.
The above is the detailed content of A long article of 10,000 words丨Deconstructing the AI security industry chain, solutions and entrepreneurial opportunities. For more information, please follow other related articles on the PHP Chinese website!
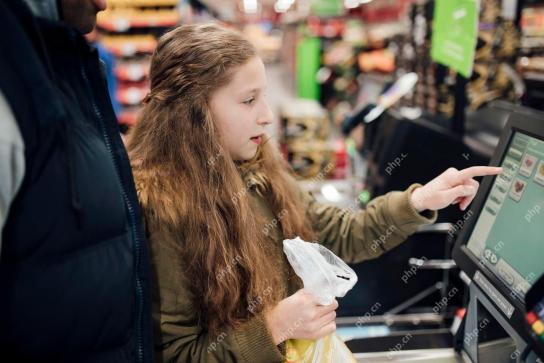
Revolutionizing the Checkout Experience Sam's Club's innovative "Just Go" system builds on its existing AI-powered "Scan & Go" technology, allowing members to scan purchases via the Sam's Club app during their shopping trip.
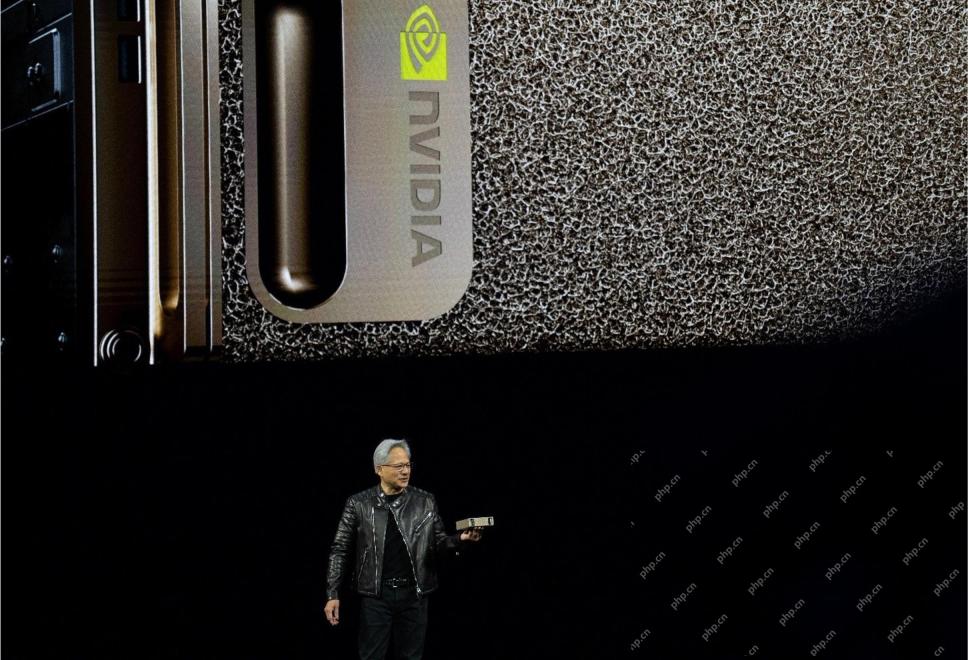
Nvidia's Enhanced Predictability and New Product Lineup at GTC 2025 Nvidia, a key player in AI infrastructure, is focusing on increased predictability for its clients. This involves consistent product delivery, meeting performance expectations, and
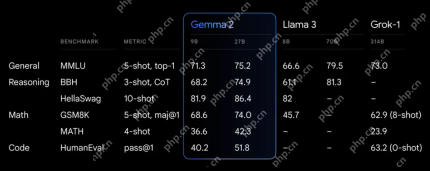
Google's Gemma 2: A Powerful, Efficient Language Model Google's Gemma family of language models, celebrated for efficiency and performance, has expanded with the arrival of Gemma 2. This latest release comprises two models: a 27-billion parameter ver
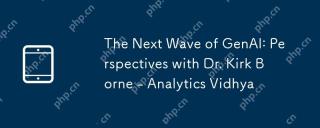
This Leading with Data episode features Dr. Kirk Borne, a leading data scientist, astrophysicist, and TEDx speaker. A renowned expert in big data, AI, and machine learning, Dr. Borne offers invaluable insights into the current state and future traje
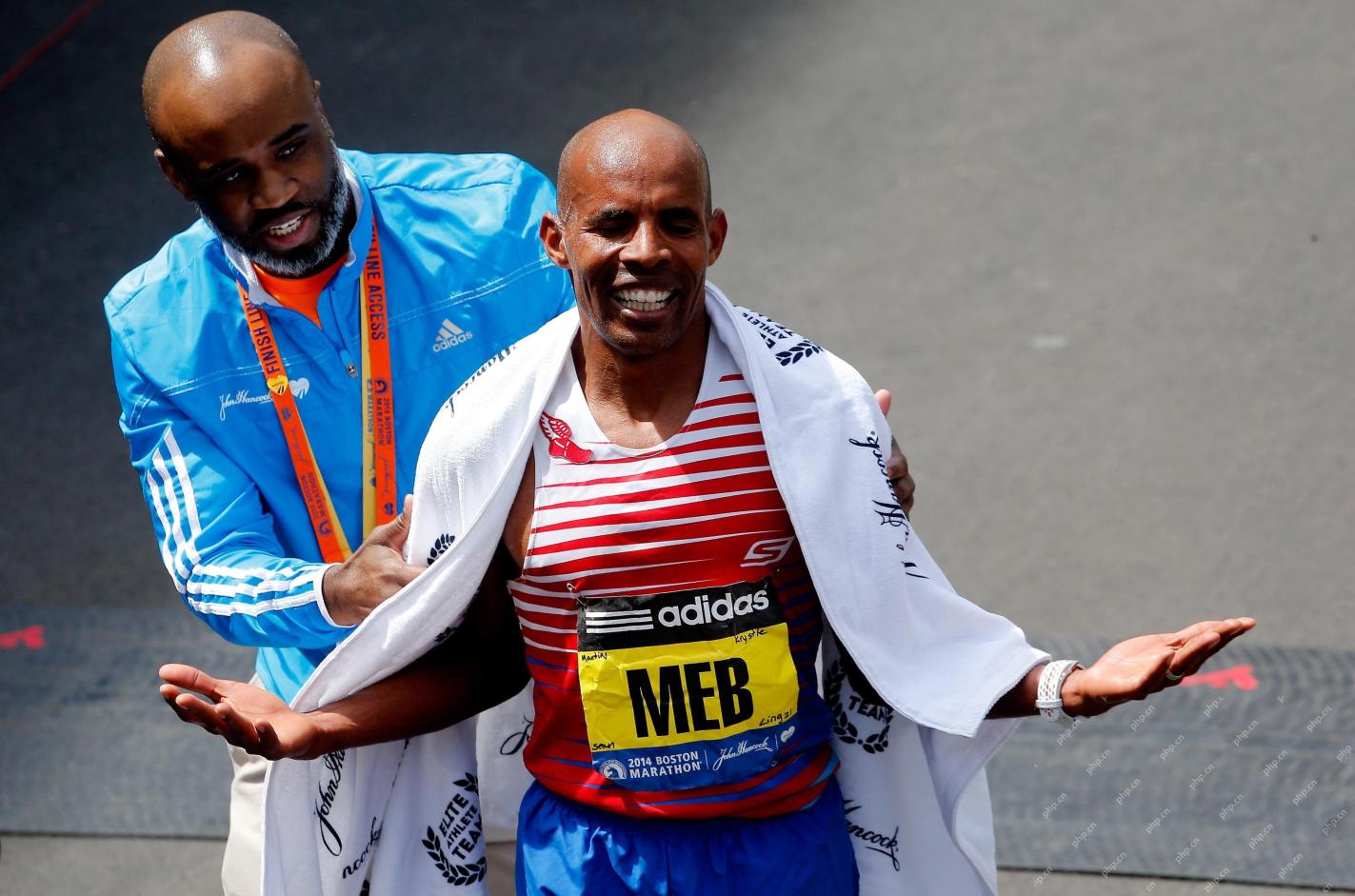
There were some very insightful perspectives in this speech—background information about engineering that showed us why artificial intelligence is so good at supporting people’s physical exercise. I will outline a core idea from each contributor’s perspective to demonstrate three design aspects that are an important part of our exploration of the application of artificial intelligence in sports. Edge devices and raw personal data This idea about artificial intelligence actually contains two components—one related to where we place large language models and the other is related to the differences between our human language and the language that our vital signs “express” when measured in real time. Alexander Amini knows a lot about running and tennis, but he still
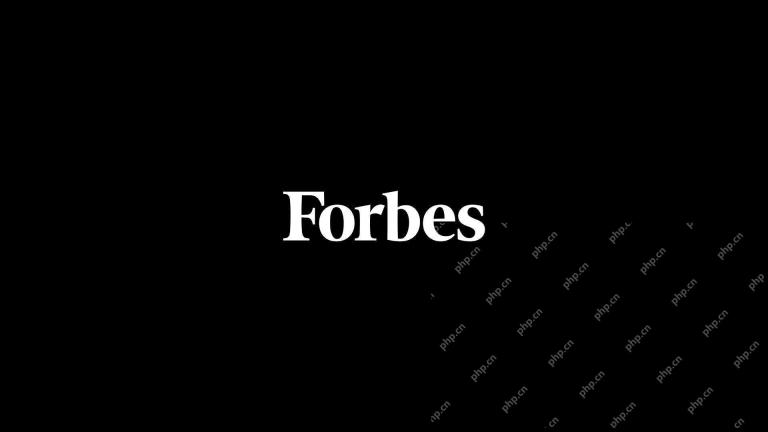
Caterpillar's Chief Information Officer and Senior Vice President of IT, Jamie Engstrom, leads a global team of over 2,200 IT professionals across 28 countries. With 26 years at Caterpillar, including four and a half years in her current role, Engst
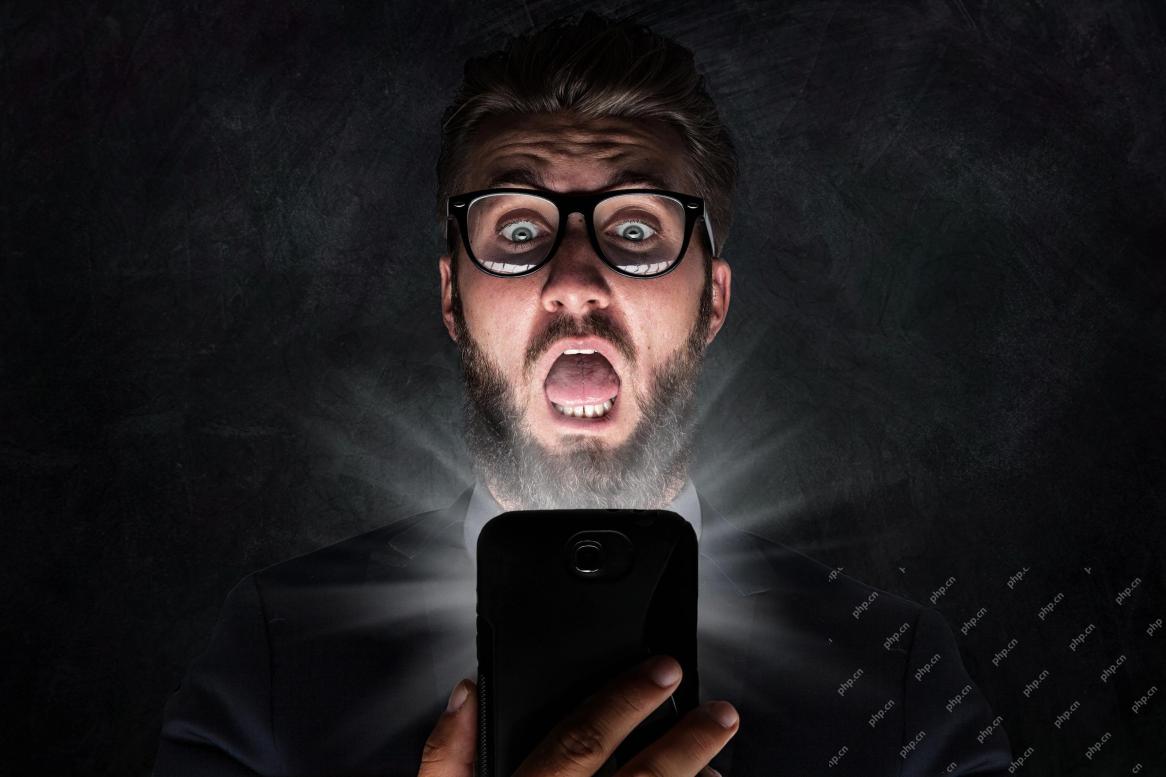
Google Photos' New Ultra HDR Tool: A Quick Guide Enhance your photos with Google Photos' new Ultra HDR tool, transforming standard images into vibrant, high-dynamic-range masterpieces. Ideal for social media, this tool boosts the impact of any photo,
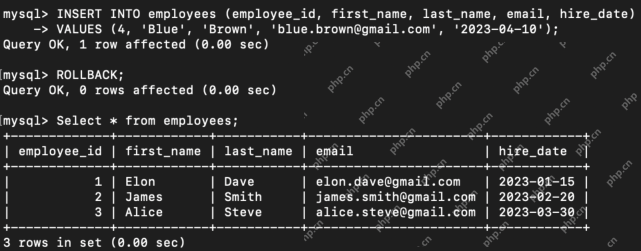
Introduction Transaction Control Language (TCL) commands are essential in SQL for managing changes made by Data Manipulation Language (DML) statements. These commands allow database administrators and users to control transaction processes, thereby


Hot AI Tools

Undresser.AI Undress
AI-powered app for creating realistic nude photos

AI Clothes Remover
Online AI tool for removing clothes from photos.

Undress AI Tool
Undress images for free

Clothoff.io
AI clothes remover

Video Face Swap
Swap faces in any video effortlessly with our completely free AI face swap tool!

Hot Article

Hot Tools

SublimeText3 English version
Recommended: Win version, supports code prompts!

mPDF
mPDF is a PHP library that can generate PDF files from UTF-8 encoded HTML. The original author, Ian Back, wrote mPDF to output PDF files "on the fly" from his website and handle different languages. It is slower than original scripts like HTML2FPDF and produces larger files when using Unicode fonts, but supports CSS styles etc. and has a lot of enhancements. Supports almost all languages, including RTL (Arabic and Hebrew) and CJK (Chinese, Japanese and Korean). Supports nested block-level elements (such as P, DIV),
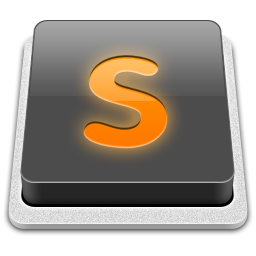
SublimeText3 Mac version
God-level code editing software (SublimeText3)

MinGW - Minimalist GNU for Windows
This project is in the process of being migrated to osdn.net/projects/mingw, you can continue to follow us there. MinGW: A native Windows port of the GNU Compiler Collection (GCC), freely distributable import libraries and header files for building native Windows applications; includes extensions to the MSVC runtime to support C99 functionality. All MinGW software can run on 64-bit Windows platforms.

Atom editor mac version download
The most popular open source editor