How to use KNN algorithm for classification in Python?
K nearest neighbor algorithm (KNN) is a simple and effective algorithm that can be used for classification and regression. Its basic idea is to identify the category to which a sample belongs by measuring the distance between different features. In this article, we will explore how to classify KNN in Python.
1. Prepare the data set
First, we need to prepare the data set. In this example, we will use the Iris dataset, which contains 3 different iris flowers (Setosa, Versicolour and Virginica), each with 4 features (Sepal Length, Sepal Width, Petal Length, Petal Width).
We will use the Pandas library to read and preprocess the data. First, we need to import the required libraries:
import pandas as pd import numpy as np import matplotlib.pyplot as plt
Then, we will load the dataset:
url = "https://archive.ics.uci.edu/ml/machine-learning-databases/iris/iris.data" names = ['sepal-length', 'sepal-width', 'petal-length', 'petal-width', 'class'] dataset = pd.read_csv(url, names=names)
Now that we have a dataset, we can start exploring it.
2. Data Visualization
Before classifying, we need to visualize and explore the data. We will plot a scatterplot of each feature against another feature, as well as a histogram of each feature. We can use Matplotlib library and Seaborn library for visualization.
Scatter plot between features:
import seaborn as sns sns.pairplot(dataset, hue="class")
As can be seen from this picture, the characteristics of different iris flowers are very different, which is the basis for classification.
Histogram of each feature:
dataset.hist() plt.show()
As can be seen from this figure, each feature in the data set has a different distribution, which is the basis for normalization.
3. Data preprocessing
Before classification, we need to preprocess the data. We can split the dataset into input features and output categories, and then scale the feature values to the range of 0 to 1.
First, we split the dataset into input features and output categories:
X = dataset.iloc[:, :-1].values y = dataset.iloc[:, 4].values
Then, we scale the feature values to the range of 0 to 1:
from sklearn.preprocessing import StandardScaler scaler = StandardScaler() X = scaler.fit_transform(X)
Now, we have our preprocessed dataset.
4. Split the data set
Before classification, we need to split the data set into a training set and a test set. We can do this using the train_test_split function from the Scikit-learn library.
from sklearn.model_selection import train_test_split X_train, X_test, y_train, y_test = train_test_split(X, y, test_size=0.2, random_state=0)
This will create a training set and a test set with a ratio of 80:20.
5. Training the KNN model
Now, we can start training the KNN model. We first import the KNeighborsClassifier class, create an instance, and use the fit function to fit the model.
from sklearn.neighbors import KNeighborsClassifier classifier = KNeighborsClassifier(n_neighbors=5) classifier.fit(X_train, y_train)
This will create a KNN classifier and train it to classify using the training set.
6. Prediction
Now, we can use the KNN model to predict the test set. We use the predict function to make predictions and save the results into a variable.
y_pred = classifier.predict(X_test)
7. Model Evaluation
Finally, we need to evaluate our model and determine its accuracy. We can use the confusion_matrix and classification_report functions in the Scikit-learn library to evaluate the accuracy of the model.
from sklearn.metrics import confusion_matrix, classification_report print(confusion_matrix(y_test, y_pred)) print(classification_report(y_test, y_pred))
This will output a confusion matrix and classification report showing the accuracy of our model.
Summary
Using the KNN algorithm for classification in Python requires the following steps:
1. Preparing the data set
2. Data visualization
3. Data Preprocessing
4.Split the data set
5.Train KNN model
6.Prediction
7.Model evaluation
The KNN algorithm is a simple and effective algorithm that can Used for classification and regression. Using the KNN algorithm for classification in Python requires following the above steps. At the same time, we also need to perform data visualization and preprocessing to ensure that our model can accurately classify.
The above is the detailed content of How to use KNN algorithm for classification in Python?. For more information, please follow other related articles on the PHP Chinese website!
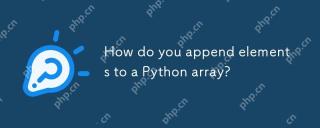
InPython,youappendelementstoalistusingtheappend()method.1)Useappend()forsingleelements:my_list.append(4).2)Useextend()or =formultipleelements:my_list.extend(another_list)ormy_list =[4,5,6].3)Useinsert()forspecificpositions:my_list.insert(1,5).Beaware
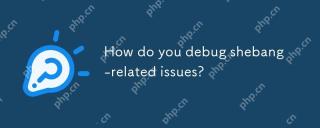
The methods to debug the shebang problem include: 1. Check the shebang line to make sure it is the first line of the script and there are no prefixed spaces; 2. Verify whether the interpreter path is correct; 3. Call the interpreter directly to run the script to isolate the shebang problem; 4. Use strace or trusts to track the system calls; 5. Check the impact of environment variables on shebang.
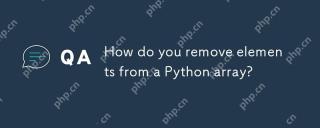
Pythonlistscanbemanipulatedusingseveralmethodstoremoveelements:1)Theremove()methodremovesthefirstoccurrenceofaspecifiedvalue.2)Thepop()methodremovesandreturnsanelementatagivenindex.3)Thedelstatementcanremoveanitemorslicebyindex.4)Listcomprehensionscr
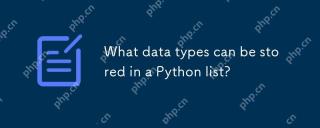
Pythonlistscanstoreanydatatype,includingintegers,strings,floats,booleans,otherlists,anddictionaries.Thisversatilityallowsformixed-typelists,whichcanbemanagedeffectivelyusingtypechecks,typehints,andspecializedlibrarieslikenumpyforperformance.Documenti
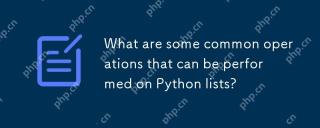
Pythonlistssupportnumerousoperations:1)Addingelementswithappend(),extend(),andinsert().2)Removingitemsusingremove(),pop(),andclear().3)Accessingandmodifyingwithindexingandslicing.4)Searchingandsortingwithindex(),sort(),andreverse().5)Advancedoperatio
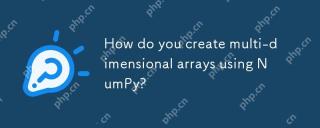
Create multi-dimensional arrays with NumPy can be achieved through the following steps: 1) Use the numpy.array() function to create an array, such as np.array([[1,2,3],[4,5,6]]) to create a 2D array; 2) Use np.zeros(), np.ones(), np.random.random() and other functions to create an array filled with specific values; 3) Understand the shape and size properties of the array to ensure that the length of the sub-array is consistent and avoid errors; 4) Use the np.reshape() function to change the shape of the array; 5) Pay attention to memory usage to ensure that the code is clear and efficient.
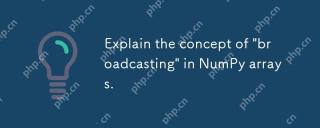
BroadcastinginNumPyisamethodtoperformoperationsonarraysofdifferentshapesbyautomaticallyaligningthem.Itsimplifiescode,enhancesreadability,andboostsperformance.Here'showitworks:1)Smallerarraysarepaddedwithonestomatchdimensions.2)Compatibledimensionsare
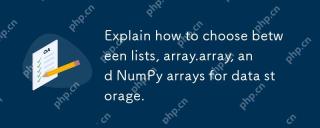
ForPythondatastorage,chooselistsforflexibilitywithmixeddatatypes,array.arrayformemory-efficienthomogeneousnumericaldata,andNumPyarraysforadvancednumericalcomputing.Listsareversatilebutlessefficientforlargenumericaldatasets;array.arrayoffersamiddlegro


Hot AI Tools

Undresser.AI Undress
AI-powered app for creating realistic nude photos

AI Clothes Remover
Online AI tool for removing clothes from photos.

Undress AI Tool
Undress images for free

Clothoff.io
AI clothes remover

Video Face Swap
Swap faces in any video effortlessly with our completely free AI face swap tool!

Hot Article

Hot Tools

MantisBT
Mantis is an easy-to-deploy web-based defect tracking tool designed to aid in product defect tracking. It requires PHP, MySQL and a web server. Check out our demo and hosting services.

MinGW - Minimalist GNU for Windows
This project is in the process of being migrated to osdn.net/projects/mingw, you can continue to follow us there. MinGW: A native Windows port of the GNU Compiler Collection (GCC), freely distributable import libraries and header files for building native Windows applications; includes extensions to the MSVC runtime to support C99 functionality. All MinGW software can run on 64-bit Windows platforms.

SublimeText3 English version
Recommended: Win version, supports code prompts!

PhpStorm Mac version
The latest (2018.2.1) professional PHP integrated development tool
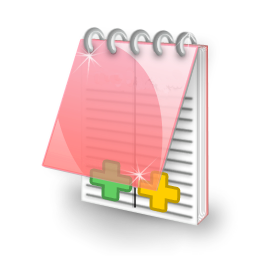
EditPlus Chinese cracked version
Small size, syntax highlighting, does not support code prompt function
