Broadcasting in NumPy is a method to perform operations on arrays of different shapes by automatically aligning them. It simplifies code, enhances readability, and boosts performance. Here's how it works: 1) Smaller arrays are padded with ones to match dimensions. 2) Compatible dimensions are identified for operation. 3) Arrays are treated as if reshaped to match each other during the operation.
Let's dive into the fascinating world of NumPy and explore the concept of "broadcasting" in its arrays. Broadcasting is a powerful feature that allows NumPy to perform operations on arrays of different shapes in a way that's both intuitive and efficient.
What is broadcasting in NumPy, and why should you care?
Broadcasting is NumPy's way of handling arrays with different shapes during arithmetic operations. It's like magic that lets you add a scalar to a matrix, or multiply two arrays of different dimensions without explicitly reshaping them. This concept is crucial because it simplifies your code, making it more readable and less prone to errors. It also leverages the efficiency of NumPy's underlying C implementation, speeding up your computations significantly.
Let's break down how broadcasting works
Imagine you want to add a 1D array to a 2D array. Normally, you'd need to reshape the 1D array to match the 2D array's dimensions. With broadcasting, NumPy does this automatically. Here's how it happens:
- If the arrays have different numbers of dimensions, the smaller array is padded with ones on its left side until both arrays have the same number of dimensions.
- Then, NumPy compares the size of each dimension. If a dimension's size is 1 in one array and matches the other array's size in that dimension, or if it's the same in both arrays, it's compatible for broadcasting.
- If all dimensions are compatible, broadcasting can proceed, and the operation is performed as if the arrays had been reshaped to match each other.
Here's a simple example to illustrate:
import numpy as np # A 2D array arr_2d = np.array([[1, 2, 3], [4, 5, 6]]) # A 1D array arr_1d = np.array([10, 20, 30]) # Broadcasting in action result = arr_2d + arr_1d print(result) # Output: # [[11 22 33] # [14 25 36]]
In this case, arr_1d
is broadcasted across arr_2d
as if it were a 2D array with shape (2, 3), where each row is [10, 20, 30]
.
Advanced broadcasting scenarios
Broadcasting isn't limited to simple cases. You can perform operations with arrays of different shapes in more complex ways. For instance, you can add a 3D array to a 2D array if the 2D array's shape matches one of the 3D array's dimensions:
arr_3d = np.array([[[1, 2], [3, 4]], [[5, 6], [7, 8]]]) arr_2d = np.array([[10, 20], [30, 40]]) result = arr_3d + arr_2d print(result) # Output: # [[[11 22] # [33 44]] # # [[15 26] # [37 48]]]
Here, arr_2d
is broadcasted along the first dimension of arr_3d
.
Common pitfalls and how to avoid them
While broadcasting is powerful, it can lead to unexpected results if you're not careful. Here are some tips to keep in mind:
-
Understand the shapes: Always check the shapes of your arrays before performing operations. Use
np.shape()
to verify. - Be aware of implicit broadcasting: Sometimes, NumPy will broadcast arrays in ways you might not expect. If your results seem off, double-check the broadcasting rules.
-
Use
np.newaxis
for explicit broadcasting: If you need to add a new dimension to an array for broadcasting, usenp.newaxis
. For example,arr[:, np.newaxis]
adds a new axis toarr
.
arr = np.array([1, 2, 3]) expanded_arr = arr[:, np.newaxis] print(expanded_arr.shape) # Output: (3, 1)
- Performance considerations: While broadcasting is efficient, it's not always the fastest approach. For very large arrays, consider using explicit reshaping or vectorization techniques.
Performance optimization and best practices
When using broadcasting, keep these optimization tips in mind:
- Minimize memory usage: Broadcasting can create temporary arrays. If memory is a concern, consider using in-place operations or explicit reshaping.
- Leverage vectorization: Broadcasting is a form of vectorization, which is generally faster than using loops. Always prefer vectorized operations when possible.
-
Profile your code: Use tools like
timeit
orcProfile
to measure the performance of your broadcasting operations. Sometimes, a different approach might be more efficient.
import numpy as np import timeit arr_large = np.random.rand(1000, 1000) scalar = 2 # Broadcasting def broadcast_add(): return arr_large + scalar # Loop-based approach def loop_add(): result = np.zeros_like(arr_large) for i in range(arr_large.shape[0]): for j in range(arr_large.shape[1]): result[i, j] = arr_large[i, j] + scalar return result # Measure performance broadcast_time = timeit.timeit(broadcast_add, number=100) loop_time = timeit.timeit(loop_add, number=100) print(f"Broadcasting time: {broadcast_time:.6f} seconds") print(f"Loop time: {loop_time:.6f} seconds")
In this example, you'll likely find that broadcasting is significantly faster than the loop-based approach, demonstrating the power of NumPy's broadcasting.
Wrapping up
Broadcasting in NumPy is a game-changer for array operations. It simplifies your code, enhances readability, and leverages NumPy's performance optimizations. By understanding and mastering broadcasting, you can write more efficient and elegant NumPy code. Just remember to be mindful of the shapes of your arrays and use the right tools to ensure your broadcasting operations work as intended. Happy coding!
The above is the detailed content of Explain the concept of 'broadcasting' in NumPy arrays.. For more information, please follow other related articles on the PHP Chinese website!
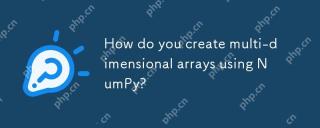
Create multi-dimensional arrays with NumPy can be achieved through the following steps: 1) Use the numpy.array() function to create an array, such as np.array([[1,2,3],[4,5,6]]) to create a 2D array; 2) Use np.zeros(), np.ones(), np.random.random() and other functions to create an array filled with specific values; 3) Understand the shape and size properties of the array to ensure that the length of the sub-array is consistent and avoid errors; 4) Use the np.reshape() function to change the shape of the array; 5) Pay attention to memory usage to ensure that the code is clear and efficient.
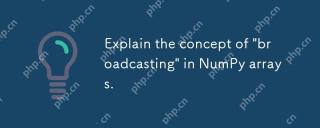
BroadcastinginNumPyisamethodtoperformoperationsonarraysofdifferentshapesbyautomaticallyaligningthem.Itsimplifiescode,enhancesreadability,andboostsperformance.Here'showitworks:1)Smallerarraysarepaddedwithonestomatchdimensions.2)Compatibledimensionsare
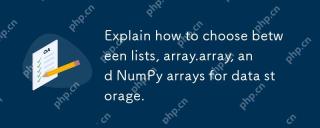
ForPythondatastorage,chooselistsforflexibilitywithmixeddatatypes,array.arrayformemory-efficienthomogeneousnumericaldata,andNumPyarraysforadvancednumericalcomputing.Listsareversatilebutlessefficientforlargenumericaldatasets;array.arrayoffersamiddlegro
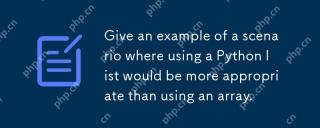
Pythonlistsarebetterthanarraysformanagingdiversedatatypes.1)Listscanholdelementsofdifferenttypes,2)theyaredynamic,allowingeasyadditionsandremovals,3)theyofferintuitiveoperationslikeslicing,but4)theyarelessmemory-efficientandslowerforlargedatasets.
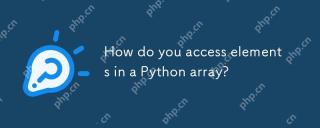
ToaccesselementsinaPythonarray,useindexing:my_array[2]accessesthethirdelement,returning3.Pythonuseszero-basedindexing.1)Usepositiveandnegativeindexing:my_list[0]forthefirstelement,my_list[-1]forthelast.2)Useslicingforarange:my_list[1:5]extractselemen
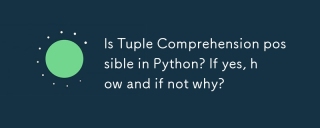
Article discusses impossibility of tuple comprehension in Python due to syntax ambiguity. Alternatives like using tuple() with generator expressions are suggested for creating tuples efficiently.(159 characters)
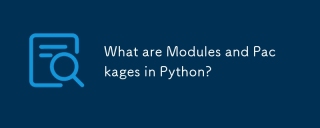
The article explains modules and packages in Python, their differences, and usage. Modules are single files, while packages are directories with an __init__.py file, organizing related modules hierarchically.
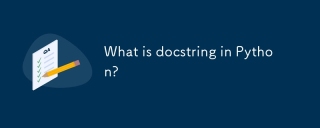
Article discusses docstrings in Python, their usage, and benefits. Main issue: importance of docstrings for code documentation and accessibility.


Hot AI Tools

Undresser.AI Undress
AI-powered app for creating realistic nude photos

AI Clothes Remover
Online AI tool for removing clothes from photos.

Undress AI Tool
Undress images for free

Clothoff.io
AI clothes remover

Video Face Swap
Swap faces in any video effortlessly with our completely free AI face swap tool!

Hot Article

Hot Tools

SublimeText3 Chinese version
Chinese version, very easy to use

mPDF
mPDF is a PHP library that can generate PDF files from UTF-8 encoded HTML. The original author, Ian Back, wrote mPDF to output PDF files "on the fly" from his website and handle different languages. It is slower than original scripts like HTML2FPDF and produces larger files when using Unicode fonts, but supports CSS styles etc. and has a lot of enhancements. Supports almost all languages, including RTL (Arabic and Hebrew) and CJK (Chinese, Japanese and Korean). Supports nested block-level elements (such as P, DIV),
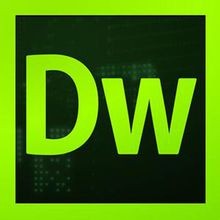
Dreamweaver CS6
Visual web development tools
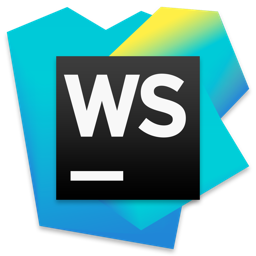
WebStorm Mac version
Useful JavaScript development tools

Safe Exam Browser
Safe Exam Browser is a secure browser environment for taking online exams securely. This software turns any computer into a secure workstation. It controls access to any utility and prevents students from using unauthorized resources.
