


WIMI Hologram (NASDAQ: WIMI) develops BCI game models and paradigms based on brain-computer interface
2023-05-15 10:48:43 Author: Song Junyi
With the rapid development of brain-computer interface (BCI) technology in recent years, scientific researchers have also integrated brain-computer interface (BCI) technology into entertainment games for in-depth research. Therefore, in addition to clinical applications, brain-computer interface (BCI) BCI) technology can also become a form of entertainment. Currently, most BCI games cannot be widely popularized due to problems such as poor control performance or easy fatigue. Over the past few years, a large number of studies have been conducted, demonstrating the increasing popularity of BCI technology in game interaction. Among the BCI games in previous studies, P300 potentials, steady-state visual evoked potentials (SSVEPs), and motor imagery (MI) were the most common games that used EEG (EEG) signals. The stability of the P300 makes it less prone to fatigue and requires no special training for the user. Therefore, WIMI Hologram (NASDAQ: WIMI) uses the P300 brain-computer interface to design a game model to explore the use of electroencephalogram (EEG) signals in actual environments for a feasible and natural game execution experience.
WIMI Hologram’s research innovation lies in the integration of BCI games and paradigm design, integrating game rules and the characteristics of the BCI system. The introduction of the convolutional neural network (CNN) algorithm can achieve high accuracy in training samples. The BCI system is not only a form of entertainment, but also increases the diversity of game operations.
In the past decade, deep learning has developed rapidly in many fields such as computer vision, natural language processing, and speech processing. In image classification, researchers have proposed some novel deep architectures that achieve high accuracy. In recent years, convolutional neural networks (CNN) have been used for P300 detection. Using CNN to identify and classify P300 EEG signals can achieve good results. In order to improve the generalization ability of the model, the state-of-the-art P300 signal classification and character recognition are implemented. On small data sets, overfitting will occur, so large data sets need to be used to train nonlinear data. At this time, CNN is very suitable. However, it is very difficult to collect large amounts of training data in practical applications. Therefore, we propose a new architecture based on convolutional neural networks (CNN) to achieve state-of-the-art P300 signal classification and character recognition on a small training dataset.
WIMI Hologram (NASDAQ:WIMI) designed a P300 BCI game model based on CNN, and proposed an algorithm based on Bayesian deep learning to solve the over-fitting problem when training on small data sets . Conclusions are drawn by successfully applying a P300-based BCI game model and demonstrating its applicability to deep learning algorithms for online BCI systems.
WIMI Hologram is based on the CNN BCI game model system framework and contains three subsystems, namely the data collection part, the data processing part and the visual and game terminals. In the data acquisition part, multi-channel scalp EEG signals are recorded using electrode caps and amplifiers. After the signal is preprocessed, the data processing process can be divided into two steps: offline training and online classification testing. Finally, the classification results are converted into operation commands and sent to the visual and game terminals. The visual and game terminal consists of two sub-steps: (1) providing visual stimulation to the user after the stimulation strategy is updated and (2) providing visual feedback (output coordinates) to the user.
EGG data collection and preprocessing
WIMI Hologram is based on the CNN BCI game model using 32-channel capacitors and amplifiers to non-invasively record EEG data, digitized at 1000 Hz and filtered using a 50 Hz notch filter. Collect all electrode data. In order to reduce the impact of filtering edge effects, the recorded data are first filtered. Band-pass filters can be used to process the EEG signal of each channel to extract key information of the P300 signal after stimulus triggering. Then, the data is downsampled. Data matrices of the same characters are stacked and averaged to reduce the signal-to-noise ratio.
CNN Architecture
WIMI WIMI Hologram After preprocessing the BCI signal based on the CNN BCI game model, the data processing part can be divided into two steps: offline training and online classification. CNNs are based on Bayesian backpropagation, a variational inference method used to learn the posterior distribution of neural network weights from which the weights in backpropagation can be sampled. For example, if we model each weight parameter with a Gaussian distribution, then the original weight value can be expressed as the mean and standard deviation of the Gaussian distribution. The posterior is then calculated via variational inference.
The convolutional layer of CNN needs to use a convolution kernel with a weight value for convolution operation. Each weight parameter in the convolution kernel is expressed in the form of a Gaussian distribution. In order to obtain a certain weight value, Gaussian distribution must be used for sampling. Therefore, in the sampling process, heavy parameterization technology is used to put the sampling process in front, so that the forward propagation of the network becomes the derivative, and the weights are updated during the back propagation process. The convolution kernel is generated by extracting specific weight values from the convolution kernel weight distribution, and then the convolution operation is performed on the receptive field.
Brain-computer interface (BCI) is an unconventional communication method that can establish a communication path between a person and the periphery. Initially, BCI technology was used in the clinical field to help patients recover and interact with each other by sending commands directly from the brain to the computer. The ability to interact with the outside world. Now in addition to clinical applications, BCI technology has also been experimented and applied in entertainment games. BCI technology is often used to provide input for games, thereby getting rid of dependence on intermediate devices (mouse, keyboard, gamepad and game controller). WIMI is based on the CNN BCI game model, forming a platform that can satisfy the interests of both healthy users and disabled users. For healthy users, BCI games are full of technology and mystery, which increases the appeal of the game and helps promote the game. For disabled users, BCI games provide them with a fair gaming platform that not only allows them to play games in the same way as healthy users, but also serves as a functional rehabilitation system to help patients with rehabilitation training. The application of BCI technology in entertainment games is a key step in pushing it from the scientific research stage to the practical application market stage.
The above is the detailed content of WIMI Hologram (NASDAQ: WIMI) develops BCI game models and paradigms based on brain-computer interface. For more information, please follow other related articles on the PHP Chinese website!

Introduction Suppose there is a farmer who daily observes the progress of crops in several weeks. He looks at the growth rates and begins to ponder about how much more taller his plants could grow in another few weeks. From th
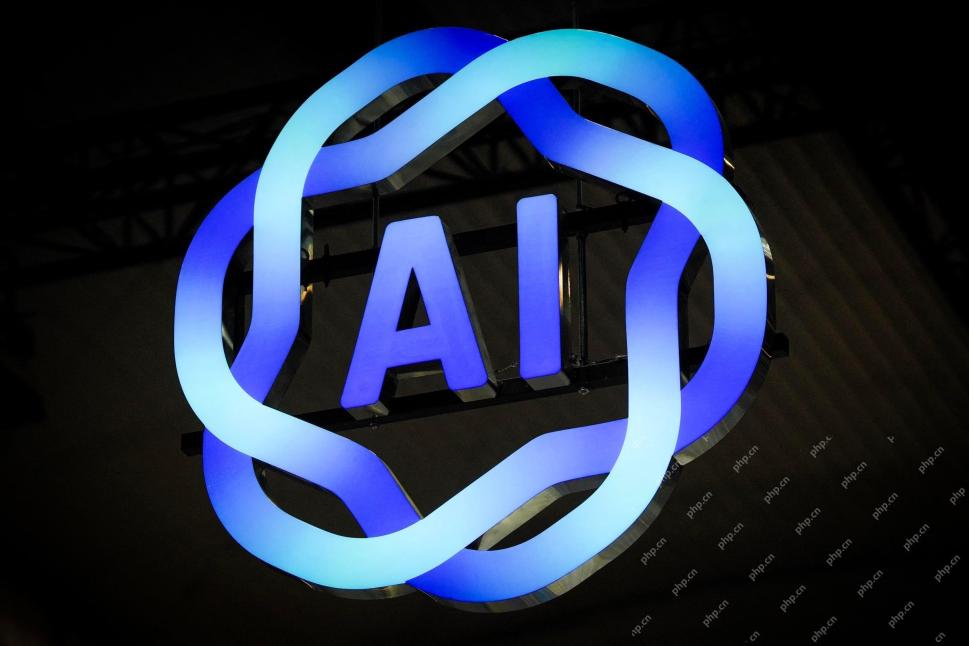
Soft AI — defined as AI systems designed to perform specific, narrow tasks using approximate reasoning, pattern recognition, and flexible decision-making — seeks to mimic human-like thinking by embracing ambiguity. But what does this mean for busine
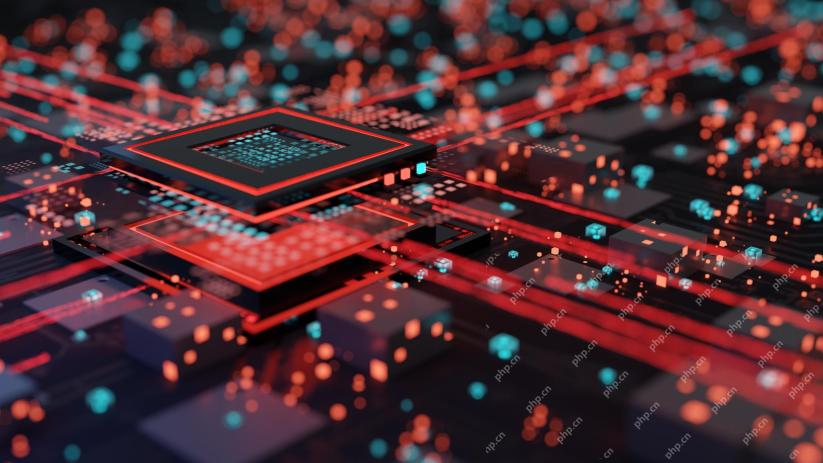
The answer is clear—just as cloud computing required a shift toward cloud-native security tools, AI demands a new breed of security solutions designed specifically for AI's unique needs. The Rise of Cloud Computing and Security Lessons Learned In th

Entrepreneurs and using AI and Generative AI to make their businesses better. At the same time, it is important to remember generative AI, like all technologies, is an amplifier – making the good great and the mediocre, worse. A rigorous 2024 study o
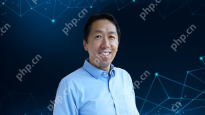
Unlock the Power of Embedding Models: A Deep Dive into Andrew Ng's New Course Imagine a future where machines understand and respond to your questions with perfect accuracy. This isn't science fiction; thanks to advancements in AI, it's becoming a r
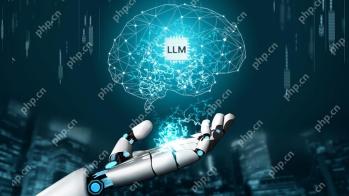
Large Language Models (LLMs) and the Inevitable Problem of Hallucinations You've likely used AI models like ChatGPT, Claude, and Gemini. These are all examples of Large Language Models (LLMs), powerful AI systems trained on massive text datasets to
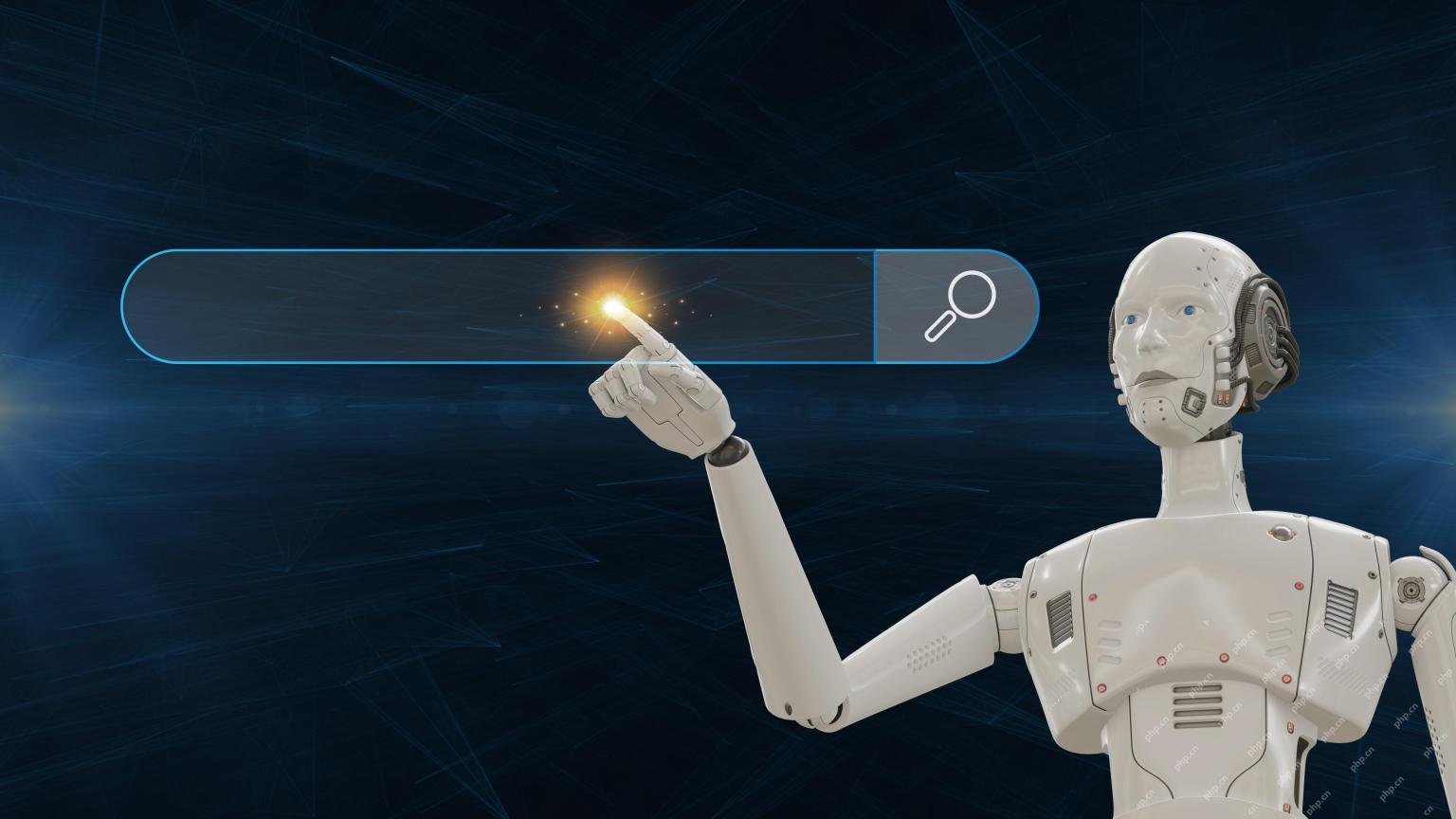
Recent research has shown that AI Overviews can cause a whopping 15-64% decline in organic traffic, based on industry and search type. This radical change is causing marketers to reconsider their whole strategy regarding digital visibility. The New
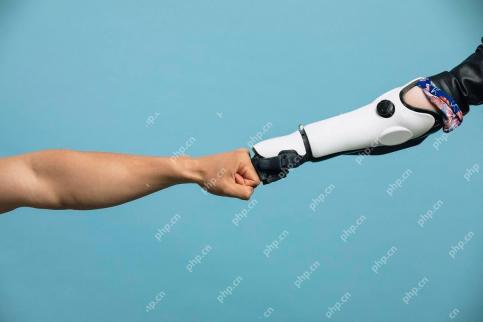
A recent report from Elon University’s Imagining The Digital Future Center surveyed nearly 300 global technology experts. The resulting report, ‘Being Human in 2035’, concluded that most are concerned that the deepening adoption of AI systems over t


Hot AI Tools

Undresser.AI Undress
AI-powered app for creating realistic nude photos

AI Clothes Remover
Online AI tool for removing clothes from photos.

Undress AI Tool
Undress images for free

Clothoff.io
AI clothes remover

AI Hentai Generator
Generate AI Hentai for free.

Hot Article

Hot Tools

Zend Studio 13.0.1
Powerful PHP integrated development environment

DVWA
Damn Vulnerable Web App (DVWA) is a PHP/MySQL web application that is very vulnerable. Its main goals are to be an aid for security professionals to test their skills and tools in a legal environment, to help web developers better understand the process of securing web applications, and to help teachers/students teach/learn in a classroom environment Web application security. The goal of DVWA is to practice some of the most common web vulnerabilities through a simple and straightforward interface, with varying degrees of difficulty. Please note that this software
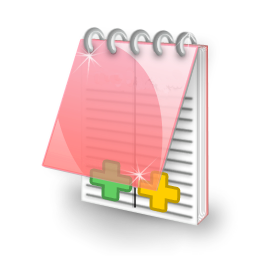
EditPlus Chinese cracked version
Small size, syntax highlighting, does not support code prompt function
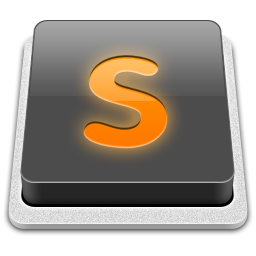
SublimeText3 Mac version
God-level code editing software (SublimeText3)

Safe Exam Browser
Safe Exam Browser is a secure browser environment for taking online exams securely. This software turns any computer into a secure workstation. It controls access to any utility and prevents students from using unauthorized resources.