


Hallucination? Musk's TruthGPT can't handle it either! OpenAI co-founder says it's complicated
Last month, Musk frantically called for a 6-month suspension of super AI research and development.
Before long, Lao Ma could no longer sit still and officially announced the launch of an AI platform called TruthGPT.
Musk once said that TruthGPT will be the "largest truth-seeking artificial intelligence" that will try to understand the nature of the universe.
He emphasized that an artificial intelligence that cares about understanding the universe is unlikely to exterminate humanity because we are an interesting part of the universe.
However, no language model can handle "illusion" so far.
Recently, the co-founder of OpenAI explained why the realization of TruthGPT’s lofty ideals is so difficult.
TruthGPT ideal is a bubble?
The TruthGPT that Musk’s X.AI wants to build is an honest language model.
In doing so, we will directly target ChatGPT.
Because, previously, AI systems like ChatGPT often produced classic hallucination cases such as erroneous output, and even supported reports of certain political beliefs.
Although ChatGPT allows users to have more control over language models to solve problems, "illusion" is still a core problem that OpenAI, Google, and Musk's artificial intelligence companies must deal with in the future.
OpenAI co-founder and researcher John Schulman discusses these challenges and how to deal with them in his talk "RL and Truthfulness – Towards TruthGPT".
#Why are there "hallucinations"?
According to Schulman, hallucinations can be roughly divided into two types:
1. "Pattern completion behavior", that is, the language model cannot express itself Uncertainty, the inability to question premises in a prompt, or to continue from a previous mistake.
2. The model guesses incorrectly.
Since the language model represents a knowledge graph that contains facts from the training data in its own network, fine-tuning can be understood as learning a function that operates on that knowledge graph And output token prediction.
For example, a fine-tuning dataset might contain the question "What is the genre of Star Wars?" and the answer "Science Fiction."
If this information is already in the original training data, i.e. it is part of the knowledge graph, then the model will not learn new information; One behavior - outputting the correct answer. This kind of fine-tuning is also called "behavioral cloning".
But the problem is, if the question about "What is the name of Han Solo's spin-off movie" appears in the fine-tuning data set.
But if the answer "Solo" is not part of the original training data set (nor part of the knowledge graph), the network will learn to answer even if it does not know the answer.
Teach the network to make up answers—that is, to create “hallucinations”—by fine-tuning answers that are actually correct but not in the knowledge graph. Conversely, training with incorrect answers causes the network to withhold information.
Thus, behavioral cloning should ideally always be based on network knowledge, but this knowledge is often unknown to the human workers who create or evaluate the dataset, such as instruction tuning.
According to Schulman, this problem also exists when other models create fine-tuned data sets, as is the case with the Alpaca formula.
He predicted that smaller networks with smaller knowledge graphs would not only learn to use the output of ChatGPT to give answers and follow instructions, but also learn to hallucinate more frequently.
How does OpenAI combat hallucinations?
First of all, for simple questions, the language model can predict whether it knows the answer in most cases, and can also express uncertainty.
Therefore, Schulman said that when fine-tuning the data set, the model must learn how to express uncertainty, how to deal with situations where the premise is changed, and when errors are acknowledged.
Instances of these situations should be fed to the model and let them learn.
But the models are still poorly trained in timing, that is, they don’t know when to perform these operations.
Schulman said that this is where reinforcement learning (RL) comes into play. For example, Reinforcement Learning with Human Feedback (RLHF).
Applying RL, the model can learn "behavior boundaries" and learn when to perform what behavior.
Another difficulty is the ability to retrieve and cite sources.
The question is, with the ability to copy behavior and RLHF, why does ChatGPT still hallucinate?
The reason lies in the difficulty of the problem itself.
While the above method works well for short questions and answers, other problems arise with long format settings common in ChatGPT.
On the one hand, a completely wrong answer is unlikely. In most cases, wrong and right are mixed together.
In extreme cases, it may be just an error in 100 lines of code.
In other cases, the information is not wrong in the traditional sense but misleading. Therefore, in a system like ChatGPT, it is difficult to measure the quality of the output in terms of information content or correctness.
But this measurement is very important for RL algorithms designed to train complex behavioral boundaries.
Currently, OpenAI relies on RLHF’s ranking-based reward model, which is able to predict which of two answers it thinks is better, but does not give a valid signal as to which one is better. How much better, more informative or correct the answer is.
Schulman said it lacks the ability to provide feedback to the model to learn fine behavioral boundaries. And this kind of fine behavioral boundary is the possible way to solve the illusion.
Additionally, this process is further complicated by human error in the RLHF labeling process.
Therefore, although Schulman regards RL as one of the important ways to reduce hallucinations, he believes that there are still many unresolved problems.
Except for what the reward model mentioned above needs to look like to guide correct behavior, RLHF currently only relies on human judgment.
This may make knowledge generation more difficult. Because predictions about the future sometimes lead to less convincing presentations.
However, Schulman believes that the generation of knowledge is the next important step in language models. At the same time, he believes that the theoretical construction of problems such as predicting the future and giving inference rules is the next type of open problem that needs to be solved urgently. Sexual issues.
One possible solution, Schulman said, is to use other AI models to train language models.
OpenAI also believes that this method is very meaningful for AI alignment.
ChatGPT Architect
As the architect of ChatGPT, John Schulman joined OpenAI as one of the co-founders as early as 2015 when he was still studying for a PhD.
In an interview, Schulman explained why he joined OpenAI:
I want to do it Regarding artificial intelligence research, I think OpenAI has an ambitious mission and is committed to building general artificial intelligence.
Although, talking about AGI seemed a little crazy at the time, I thought it was reasonable to start thinking about it, and I wanted to be in a place where talking about AGI was acceptable.
In addition, according to Schulman, OpenAI’s idea of introducing human feedback reinforcement learning (RLHF) into ChatGPT can be traced back to 17 years ago.
At that time, he was also a member of OpenAI and published a paper "Deep Reinforcement Learning from Human Preferences" which mentioned this method.
##Paper address: https://arxiv.org/pdf/1706.03741.pdf
The OpenAI security team is working on this because they want to align their models with human preferences—trying to make the models actually listen to humans and try to do what humans want to do.
When GPT-3 completed training, then Schulman decided to join this trend because he saw the potential of the entire research direction.
When asked what his first reaction was when he used ChatGPT for the first time, Schulman’s words revealed “no emotion.”
I still remember that ChatGPT came out last year, which made many people’s brains explode instantly.
And no one inside OpenAI is excited about ChatGPT. Because the released ChatGPT was a weaker model based on GPT-3.5, colleagues were playing with GPT-4 at that time.
So at that time, no one at OpenAI was excited about ChatGPT because there was such a more powerful and smarter model that had already been trained.
Regarding his views on the next frontier of artificial intelligence in the future, Schulman said that AI continues to improve on more difficult tasks, and then the question arises, what should humans do? , tasks under which humans can have greater influence and do more work with the help of large models.
The above is the detailed content of Hallucination? Musk's TruthGPT can't handle it either! OpenAI co-founder says it's complicated. For more information, please follow other related articles on the PHP Chinese website!
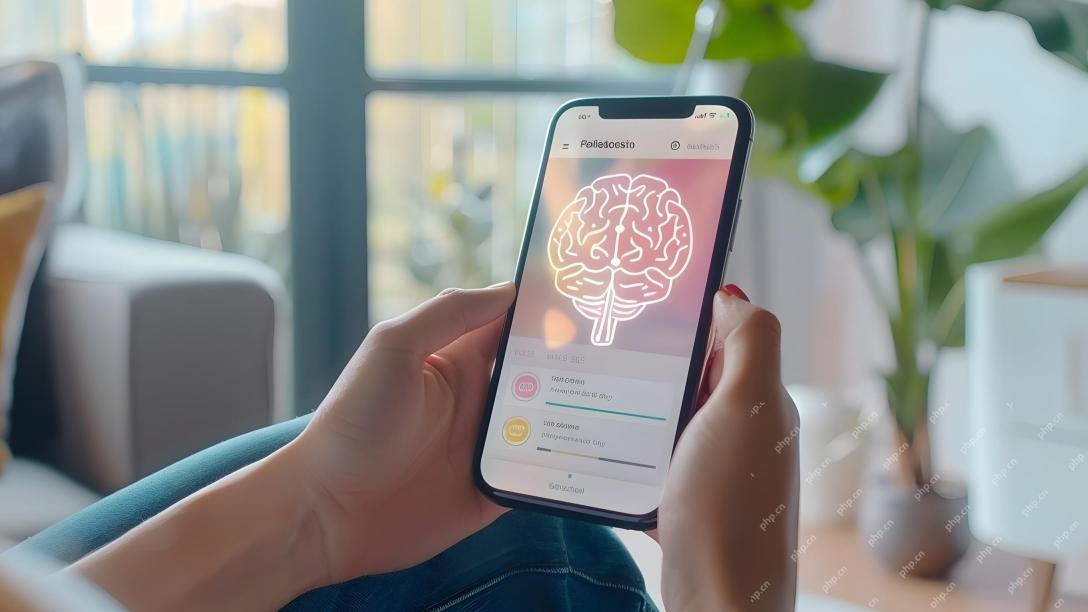
While it can’t provide the human connection and intuition of a trained therapist, research has shown that many people are comfortable sharing their worries and concerns with relatively faceless and anonymous AI bots. Whether this is always a good i
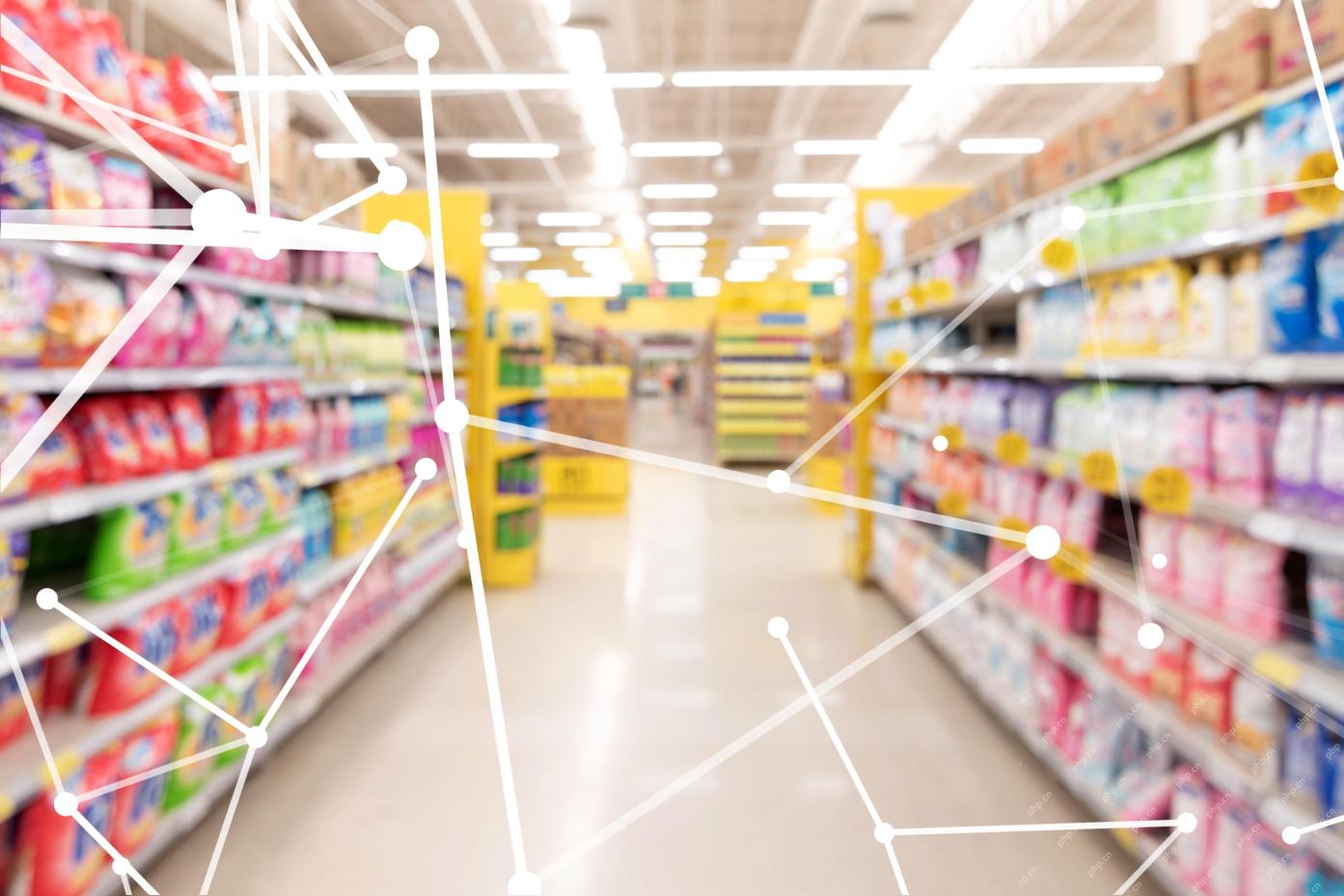
Artificial intelligence (AI), a technology decades in the making, is revolutionizing the food retail industry. From large-scale efficiency gains and cost reductions to streamlined processes across various business functions, AI's impact is undeniabl
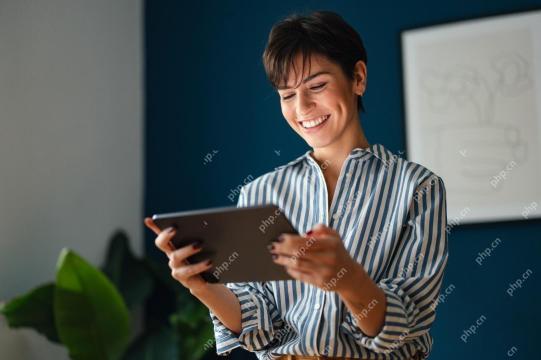
Let’s talk about it. This analysis of an innovative AI breakthrough is part of my ongoing Forbes column coverage on the latest in AI including identifying and explaining various impactful AI complexities (see the link here). In addition, for my comp
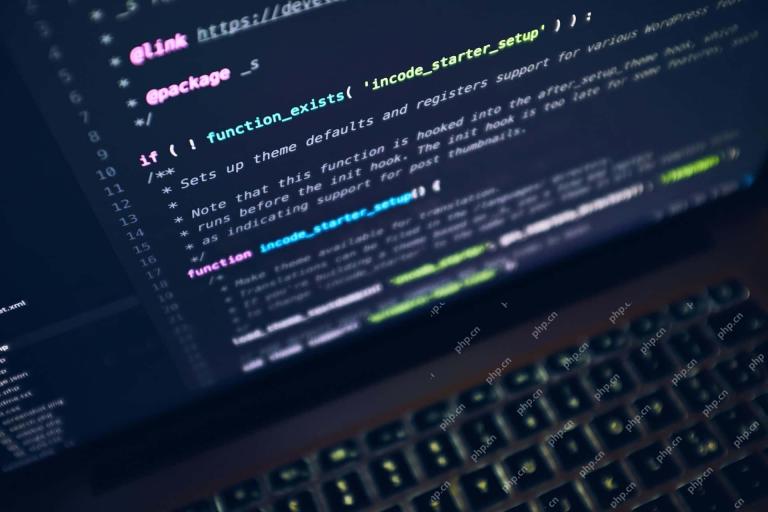
Maintaining a professional image requires occasional wardrobe updates. While online shopping is convenient, it lacks the certainty of in-person try-ons. My solution? AI-powered personalization. I envision an AI assistant curating clothing selecti
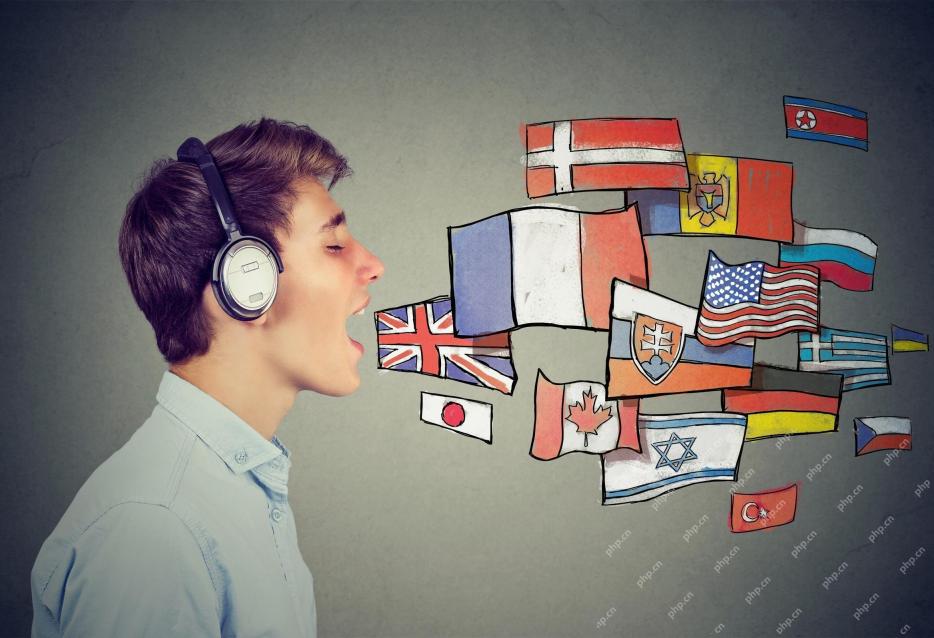
Google Translate adds language learning function According to Android Authority, app expert AssembleDebug has found that the latest version of the Google Translate app contains a new "practice" mode of testing code designed to help users improve their language skills through personalized activities. This feature is currently invisible to users, but AssembleDebug is able to partially activate it and view some of its new user interface elements. When activated, the feature adds a new Graduation Cap icon at the bottom of the screen marked with a "Beta" badge indicating that the "Practice" feature will be released initially in experimental form. The related pop-up prompt shows "Practice the activities tailored for you!", which means Google will generate customized
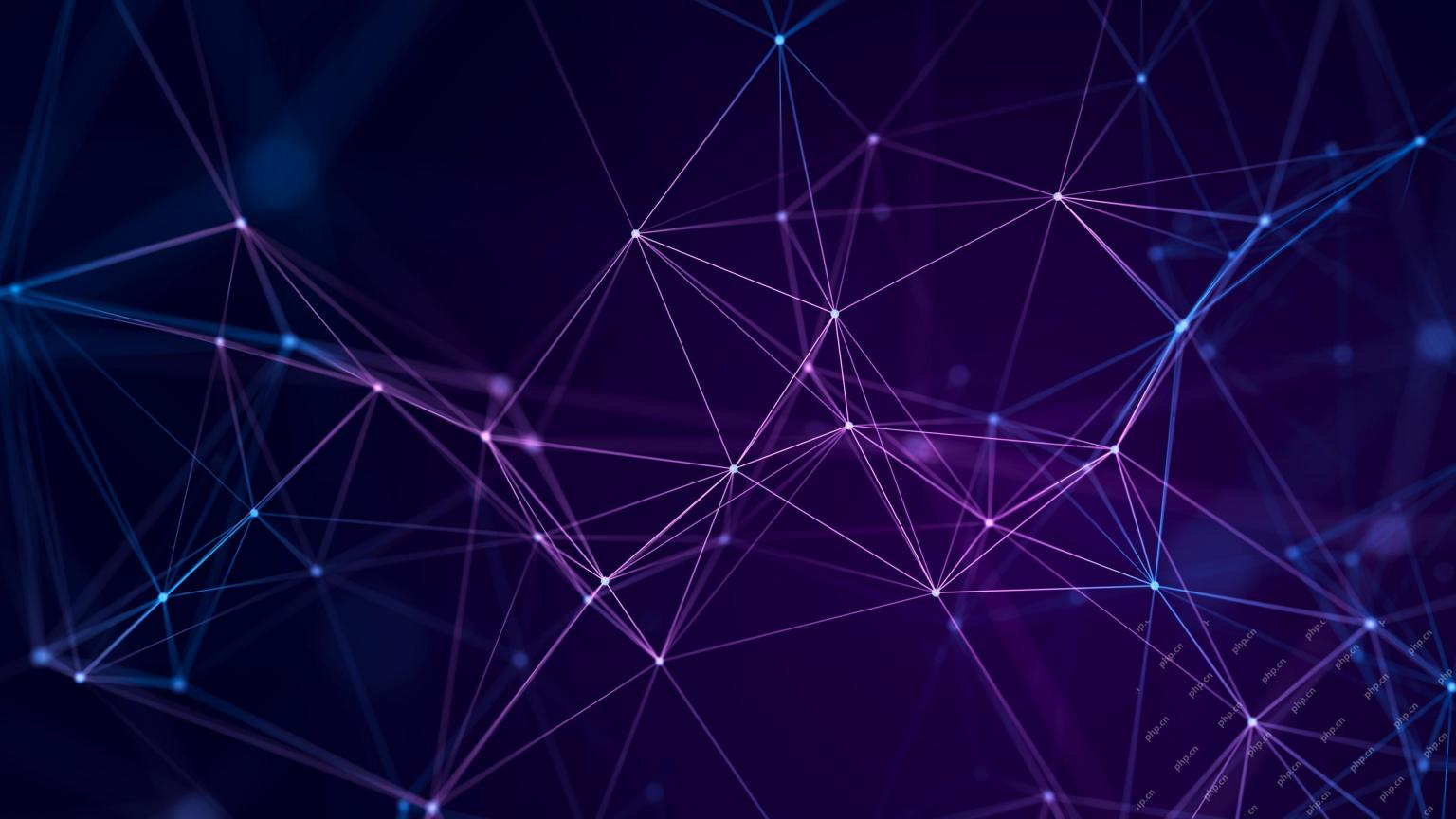
MIT researchers are developing NANDA, a groundbreaking web protocol designed for AI agents. Short for Networked Agents and Decentralized AI, NANDA builds upon Anthropic's Model Context Protocol (MCP) by adding internet capabilities, enabling AI agen
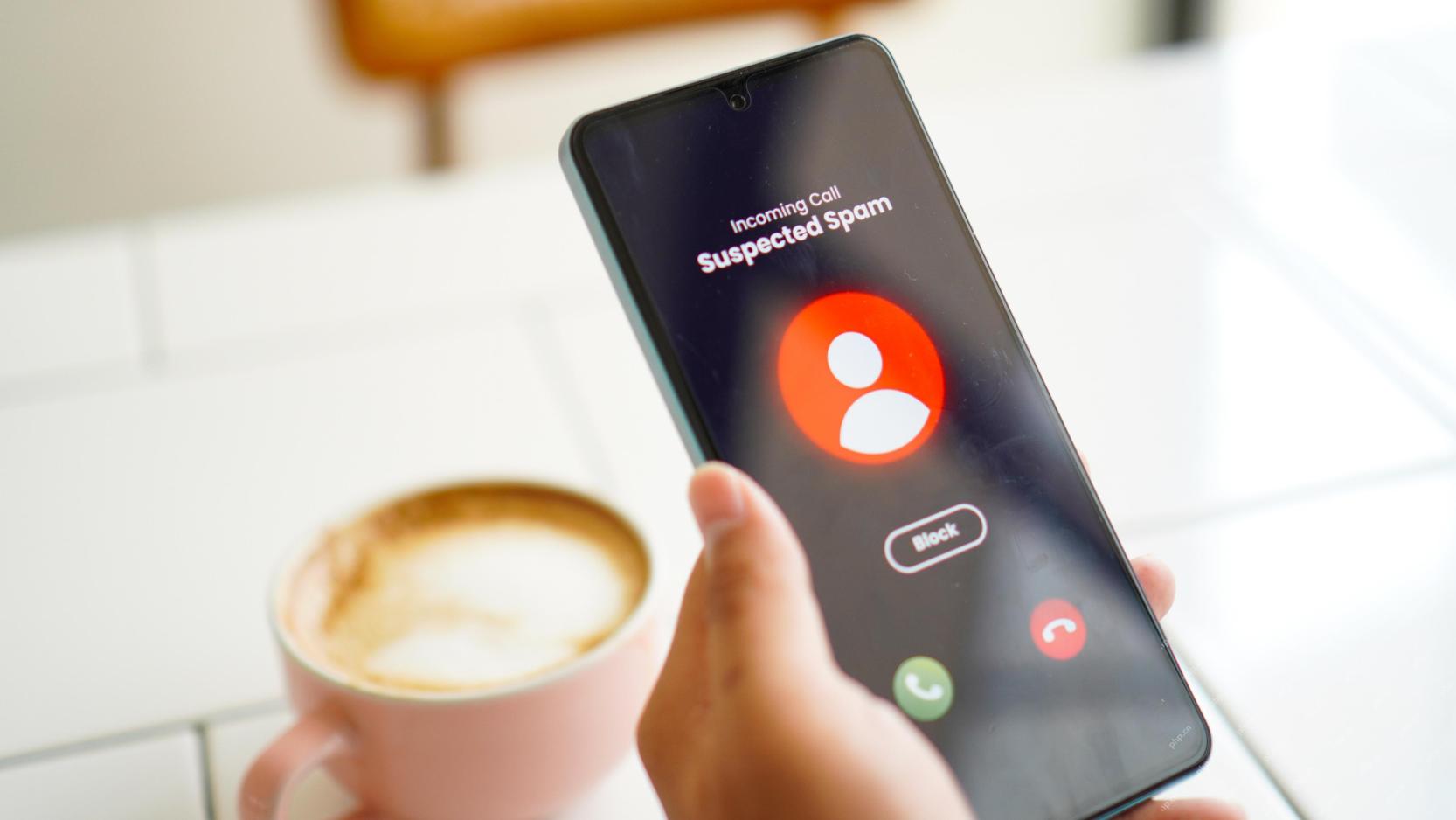
Meta's Latest Venture: An AI App to Rival ChatGPT Meta, the parent company of Facebook, Instagram, WhatsApp, and Threads, is launching a new AI-powered application. This standalone app, Meta AI, aims to compete directly with OpenAI's ChatGPT. Lever
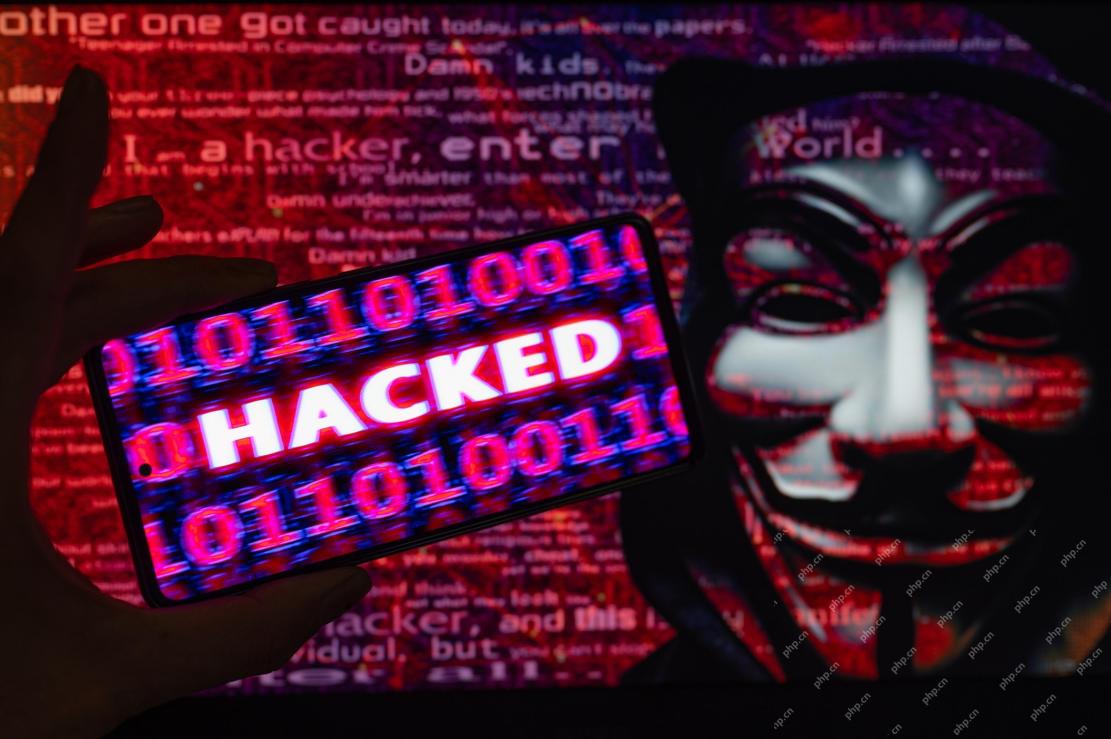
Navigating the Rising Tide of AI Cyber Attacks Recently, Jason Clinton, CISO for Anthropic, underscored the emerging risks tied to non-human identities—as machine-to-machine communication proliferates, safeguarding these "identities" become


Hot AI Tools

Undresser.AI Undress
AI-powered app for creating realistic nude photos

AI Clothes Remover
Online AI tool for removing clothes from photos.

Undress AI Tool
Undress images for free

Clothoff.io
AI clothes remover

Video Face Swap
Swap faces in any video effortlessly with our completely free AI face swap tool!

Hot Article

Hot Tools

SublimeText3 Linux new version
SublimeText3 Linux latest version
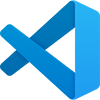
VSCode Windows 64-bit Download
A free and powerful IDE editor launched by Microsoft
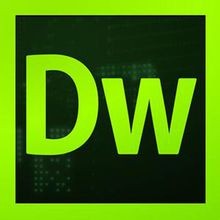
Dreamweaver CS6
Visual web development tools
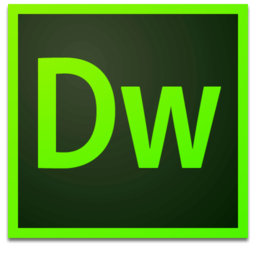
Dreamweaver Mac version
Visual web development tools
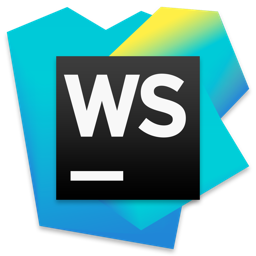
WebStorm Mac version
Useful JavaScript development tools
