Preface
After Python3.7, the contextvars
module appeared in the official library. Its main function is to provide multi-threading And the asyncio ecosystem adds context functions. Even if the program is running multiple coroutines concurrently, it can call the context variables of the program, thereby decoupling our logic.
Context can be understood as speaking for us In the process of chatting, if some words are separated from the specific context, their meaning changes, and the same is true for the running of the program. There is also its context in the thread, but it is called a stack, such as in python is saved in the thread.local variable, and the coroutine also has its own context, but it is not exposed. However, with the contextvars
module, we can use the contextvars
module to save and Read.
The advantage of using contextvars
is not only to prevent "a variable from being spread all over the world" from happening, but it can also be combined with TypeHint very well, so that your code can It is checked by mypy and IDE to make your code more suitable for engineering.
However, after using contextvars
, there will be some more hidden calls, and these hidden costs need to be solved.
Update instructions
Switch web framework
sanic
Add a self forstarlette
Write a context description that can be used for
starlette
,fastapi
Update the latest example of fast_tools.context and simply modify the text.
1. The difference between passing variables with or without context
If you have used the Flask
framework, you will know Flask
owns It has its own context function, and contextvars is very similar to it, and also adds support for asyncio context. The context of Flask
is implemented based on threading.local
. The isolation effect of threading.local
is very good, but it is only for threads. Isolate the data status between threads, and werkzeug
in order to support running in gevent
, I implemented a Local
variable, the commonly used Flask
Examples of context variablesrequest
are as follows:
from flask import Flask, request app = Flask(__name__) @app.route('/') def root(): so1n_name = request.get('so1n_name') return f'Name is {so1n_name}'
Compared with another classic web framework of Python
Djano
, it does not Context support, so the request
object can only be passed displayed, The example is as follows:
from django.http import HttpResponse def root(request): so1n_name = request.get('so1n_name') return HttpResponse(f'Name is {so1n_name}')
It can be found by comparing the above two, in Django# In ##, we need to explicitly pass a variable called request, while
Flask imports a global variable called request and uses it directly in the view to achieve the purpose of decoupling.
# 伪代码,举个例子一个request传了3个函数 from django.http import HttpResponse def is_allow(request, uid): if request.ip == '127.0.0.1' and check_permissions(uid): return True else: return False def check_permissions(request, uid): pass def root(request): user_id = request.GET.get('uid') if is_allow(request, id): return HttpResponse('ok') else return HttpResponse('error')In addition, in addition to preventing
the problem of passing a parameter for a day, some decoupling can be carried out through context. For example, one of the most classic technical business requirements is to print logs request_id, thereby facilitating link troubleshooting. At this time, if there is a context module, the reading and writing of request_id can be decoupled, such as the following example of reading and writing request_id based on the
Flask framework:
import logging from typing import Any from flask import g # type: ignore from flask.logging import default_handler # 这是一个Python logging.Filter的对象, 日志在生成之前会经过Filter步骤, 这时候我们可以为他绑定request_id变量 class RequestIDLogFilter(logging.Filter): """ Log filter to inject the current request id of the request under `log_record.request_id` """ def filter(self, record: Any) -> Any: record.request_id = g.request_id or None return record # 配置日志的format格式, 这里多配了一个request_id变量 format_string: str = ( "[%(asctime)s][%(levelname)s][%(filename)s:%(lineno)d:%(funcName)s:%(request_id)s]" " %(message)s" ) # 为flask的默认logger设置format和增加一个logging.Filter对象 default_handler.setFormatter(logging.Formatter(format_string)) default_handler.addFilter(RequestIDLogFilter()) # 该方法用于设置request_id def set_request_id() -> None: g.request_id = request.headers.get("X-Request-Id", str(uuid4())) # 初始化FLask对象, 并设置before_request app: Flask = Flask("demo") app.before_request(set_request_id)2. How to use the contextvars module
Here is an example, but this example also has other solutions. I just use this example to explain how to use the contextvar moduleFirst look at how the asyncio web framework passes variables when
contextvars is not used. According to the documentation of
starlette, when
contextvars is not used, # is passed ##Redis
The way to use the client instance is to save the Redis
client instance through the request.stat variable. Rewrite the code as follows: <pre class='brush:php;toolbar:false;'># demo/web_tools.py
# 通过中间件把变量给存进去
class RequestContextMiddleware(BaseHTTPMiddleware):
async def dispatch(
self, request: Request, call_next: RequestResponseEndpoint
) -> Response:
request.stat.redis = REDIS_POOL
response = await call_next(request)
return response
# demo/server.py
# 调用变量
@APP.route(&#39;/&#39;)
async def homepage(request):
# 伪代码,这里是执行redis命令
await request.stat.redis.execute()
return JSONResponse({&#39;hello&#39;: &#39;world&#39;})</pre>
The code is very simple and can run normally. , but when you refactor next time, for example, if you simply change the variable name redis to new_redis, the IDE will not recognize it and you need to change it one by one. At the same time, when writing code, the IDE will never know the type of the variable called by this method, and the IDE cannot intelligently check it for you (for example, when entering request.stat.redis., the IDE will not execute or an error will appear. , the IDE will not prompt). This is very unfavorable to the engineering of the project, and
and TypeHints
can solve this problem. said So many, let’s take a
client as an example to show how to use contextvars
in the asyncio ecosystem, and introduce TypeHints
(see the code for detailed explanation).<pre class='brush:php;toolbar:false;'># demo/context.py
# 该文件存放contextvars相关
import contextvars
if TYPE_CHECKING:
from demo.redis_dal import RDS # 这里是一个redis的封装实例
# 初始化一个redis相关的全局context
redis_pool_context = contextvars.ContextVar(&#39;redis_pool&#39;)
# 通过函数调用可以获取到当前协程运行时的context上下文
def get_redis() -> &#39;RDS&#39;:
return redis_pool_context.get()
# demo/web_tool.py
# 该文件存放starlette相关模块
from starlette.middleware.base import BaseHTTPMiddleware
from starlette.requests import Request
from starlette.middleware.base import RequestResponseEndpoint
from starlette.responses import Response
from demo.redis_dal import RDS
# 初始化一个redis客户端变量,当前为空
REDIS_POOL = None # type: Optional[RDS]
class RequestContextMiddleware(BaseHTTPMiddleware):
async def dispatch(
self, request: Request, call_next: RequestResponseEndpoint
) -> Response:
# 通过中间件,在进入路由之前,把redis客户端放入当前协程的上下文之中
token = redis_pool_context.set(REDIS_POOL)
try:
response = await call_next(request)
return response
finally:
# 调用完成,回收当前请求设置的redis客户端的上下文
redis_pool_context.reset(token)
async def startup_event() -> None:
global REDIS_POOL
REDIS_POOL = RDS() # 初始化客户端,里面通过asyncio.ensure_future逻辑延后连接
async def shutdown_event() -> None:
if REDIS_POOL:
await REDIS_POOL.close() # 关闭redis客户端
# demo/server.py
# 该文件存放starlette main逻辑
from starlette.applications import Starlette
from starlette.responses import JSONResponse
from demo.web_tool import RequestContextMiddleware
from demo.context import get_redis
APP = Starlette()
APP.add_middleware(RequestContextMiddleware)
@APP.route(&#39;/&#39;)
async def homepage(request):
# 伪代码,这里是执行redis命令
# 只要验证 id(get_redis())等于demo.web_tool里REDID_POOL的id一致,那证明contextvars可以为asyncio维护一套上下文状态
await get_redis().execute()
return JSONResponse({&#39;hello&#39;: &#39;world&#39;})</pre><h3 id="如何优雅的使用contextvars">3.如何优雅的使用contextvars</h3>
<p>从上面的示例代码来看, 使用<code>contextvar
和TypeHint
确实能让让IDE可以识别到这个变量是什么了, 但增加的代码太多了,更恐怖的是, 每多一个变量,就需要自己去写一个context,一个变量的初始化,一个变量的get函数,同时在引用时使用函数会比较别扭.
自己在使用了contextvars
一段时间后,觉得这样太麻烦了,每次都要做一堆重复的操作,且平时使用最多的就是把一个实例或者提炼出Headers的参数放入contextvars中,所以写了一个封装fast_tools.context(同时兼容fastapi
和starlette
), 它能屏蔽所有与contextvars的相关逻辑,其中由ContextModel负责contextvars的set和get操作,ContextMiddleware管理contextvars的周期,HeaderHeader负责托管Headers相关的参数, 调用者只需要在ContextModel中写入自己需要的变量,引用时调用ContextModel的属性即可.
以下是调用者的代码示例, 这里的实例化变量由一个http client代替, 且都会每次请求分配一个客户端实例, 但在实际使用中并不会为每一个请求都分配一个客户端实例, 很影响性能:
import asyncio import uuid from contextvars import Context, copy_context from functools import partial from typing import Optional, Set import httpx from fastapi import FastAPI, Request, Response from fast_tools.context import ContextBaseModel, ContextMiddleware, HeaderHelper app: FastAPI = FastAPI() check_set: Set[int] = set() class ContextModel(ContextBaseModel): """ 通过该实例可以屏蔽大部分与contextvars相关的操作,如果要添加一个变量,则在该实例添加一个属性即可. 属性必须要使用Type Hints的写法,不然不会识别(强制使用Type Hints) """ # 用于把自己的实例(如上文所说的redis客户端)存放于contextvars中 http_client: httpx.AsyncClient # HeaderHepler用于把header的变量存放于contextvars中 request_id: str = HeaderHelper.i("X-Request-Id", default_func=lambda request: str(uuid.uuid4())) ip: str = HeaderHelper.i("X-Real-IP", default_func=lambda request: request.client.host) user_agent: str = HeaderHelper.i("User-Agent") async def before_request(self, request: Request) -> None: # 请求之前的钩子, 通过该钩子可以设置自己的变量 self.http_client = httpx.AsyncClient() check_set.add(id(self.http_client)) async def before_reset_context(self, request: Request, response: Optional[Response]) -> None: # 准备退出中间件的钩子, 这步奏后会清掉上下文 await self.http_client.aclose() context_model: ContextModel = ContextModel() app.add_middleware(ContextMiddleware, context_model=context_model) async def test_ensure_future() -> None: assert id(context_model.http_client) in check_set def test_run_in_executor() -> None: assert id(context_model.http_client) in check_set def test_call_soon() -> None: assert id(context_model.http_client) in check_set @app.get("/") async def root() -> dict: # 在使用asyncio.ensure_future开启另外一个子协程跑任务时, 也可以复用上下文 asyncio.ensure_future(test_ensure_future()) loop: "asyncio.AbstractEventLoop" = asyncio.get_event_loop() # 使用call_soon也能复用上下文 loop.call_soon(test_call_soon) # 使用run_in_executor也能复用上下文, 但必须使用上下文的run方法, copy_context表示复制当前的上下文 ctx: Context = copy_context() await loop.run_in_executor(None, partial(ctx.run, test_run_in_executor)) # type: ignore return { "message": context_model.to_dict(is_safe_return=True), # not return CustomQuery "client_id": id(context_model.http_client), } if __name__ == "__main__": import uvicorn # type: ignore uvicorn.run(app)
可以从例子中看到, 通过封装的上下文调用会变得非常愉快, 只要通过一两步方法就能设置好自己的上下文属性, 同时不用考虑如何编写上下文的生命周期. 另外也能通过这个例子看出, 在asyncio生态中, contextvars能运用到包括子协程, 多线程等所有的场景中.
4.contextvars的原理
在第一次使用时,我就很好奇contextvars是如何去维护程序的上下文的,好在contextvars的作者出了一个向下兼容的contextvars库,虽然他不支持asyncio,但我们还是可以通过代码了解到他的基本原理.
4.1 ContextMeta,ContextVarMeta和TokenMeta
代码仓中有ContextMeta
,ContextVarMeta
和TokenMeta
这几个对象, 它们的功能都是防止用户来继承Context
,ContextVar
和Token
,原理都是通过元类来判断类名是否是自己编写类的名称,如果不是则抛错.
class ContextMeta(type(collections.abc.Mapping)): # contextvars.Context is not subclassable. def __new__(mcls, names, bases, dct): cls = super().__new__(mcls, names, bases, dct) if cls.__module__ != 'contextvars' or cls.__name__ != 'Context': raise TypeError("type 'Context' is not an acceptable base type") return cls
4.2 Token
上下文的本质是一个堆栈, 每次set一次对象就向堆栈增加一层数据, 每次reset就是pop掉最上层的数据, 而在Contextvars
中, 通过Token
对象来维护堆栈之间的交互.
class Token(metaclass=TokenMeta): MISSING = object() def __init__(self, context, var, old_value): # 分别存放上下文变量, 当前set的数据以及上次set的数据 self._context = context self._var = var self._old_value = old_value self._used = False @property def var(self): return self._var @property def old_value(self): return self._old_value def __repr__(self): r = '<Token ' if self._used: r += ' used' r += ' var={!r} at {:0x}>'.format(self._var, id(self)) return r
可以看到Token
的代码很少, 它只保存当前的context
变量, 本次调用set的数据和上一次被set的旧数据. 用户只有在调用contextvar.context
后才能得到Token
, 返回的Token
可以被用户在调用context后, 通过调用context.reset(token)来清空保存的上下文,方便本次context的变量能及时的被回收, 回到上上次的数据.
4.3 全局唯一context
前面说过, Python中由threading.local()
负责每个线程的context, 协程属于线程的’子集’,所以contextvar直接基于threading.local()
生成自己的全局context. 从他的源代码可以看到, _state
就是threading.local()
的引用, 并通过设置和读取_state
的context
属性来写入和读取当前的上下文, copy_context
调用也很简单, 同样也是调用到threading.local()
API.
def copy_context(): return _get_context().copy() def _get_context(): ctx = getattr(_state, 'context', None) if ctx is None: ctx = Context() _state.context = ctx return ctx def _set_context(ctx): _state.context = ctx _state = threading.local()
关于threading.local()
,虽然不是本文重点,但由于contextvars
是基于threading.local()
进行封装的,所以还是要明白threading.local()
的原理,这里并不直接通过源码分析, 而是做一个简单的示例解释.
在一个线程里面使用线程的局部变量会比直接使用全局变量的性能好,因为局部变量只有线程自己能看见,不会影响其他线程,而全局变量的修改必须加锁, 性能会变得很差, 比如下面全局变量的例子:
pet_dict = {} def get_pet(pet_name): return pet_dict[pet_name] def set_pet(pet_name): return pet_dict[pet_name]
这份代码就是模仿一个简单的全局变量调用, 如果是多线程调用的话, 那就需要加锁啦, 每次在读写之前都要等到持有锁的线程放弃了锁后再去竞争, 而且还可能污染到了别的线程存放的数据.
而线程的局部变量则是让每个线程有一个自己的pet_dict
, 假设每个线程调用get_pet
,set_pet
时,都会把自己的pid传入进来, 那么就可以避免多个线程去同时竞争资源, 同时也不会污染到别的线程的数据, 那么代码可以改为这样子:
pet_dict = {} def get_pet(pet_name, pid): return pet_dict[pid][pet_name] def set_pet(pet_name, pid): return pet_dict[pid][pet_name]
不过这样子使用起来非常方便, 同时示例例子没有对异常检查和初始化等处理, 如果值比较复杂, 我们还要维护异常状况, 这样太麻烦了.
这时候threading.local()
就应运而生了,他负责帮我们处理这些维护的工作,我们只要对他进行一些调用即可,调用起来跟单线程调用一样简单方便, 应用threading.local()
后的代码如下:
import threading thread_local=threading.local() def get_pet(pet_name): return thread_local[pet_name] def set_pet(pet_name): return thread_local[pet_name]
可以看到代码就像调用全局变量一样, 但是又不会产生竞争状态。
4.4contextvar自己封装的Context
contextvars
自己封装的Context比较简单, 这里只展示他的两个核心方法(其他的魔术方法就像dict
的魔术方法一样):
class Context(collections.abc.Mapping, metaclass=ContextMeta): def __init__(self): self._data = immutables.Map() self._prev_context = None def run(self, callable, *args, **kwargs): if self._prev_context is not None: raise RuntimeError( 'cannot enter context: {} is already entered'.format(self)) self._prev_context = _get_context() try: _set_context(self) return callable(*args, **kwargs) finally: _set_context(self._prev_context) self._prev_context = None def copy(self): new = Context() new._data = self._data return new
首先, 在__init__
方法可以看到self._data,这里使用到了一个叫immutables.Map()的不可变对象,并对immutables.Map()进行一些封装,所以context可以看成一个不可变的dict。这样可以防止调用copy方法后得到的上下文的变动会影响到了原本的上下文变量。
查看immutables.Map()的示例代码可以看到,每次对原对象的修改时,原对象并不会发生改变,并会返回一个已经发生改变的新对象.
map2 = map.set('a', 10) print(map, map2) # will print: # <immutables.Map({'a': 1, 'b': 2})> # <immutables.Map({'a': 10, 'b': 2})> map3 = map2.delete('b') print(map, map2, map3) # will print: # <immutables.Map({'a': 1, 'b': 2})> # <immutables.Map({'a': 10, 'b': 2})> # <immutables.Map({'a': 10})>
此外,context还有一个叫run
的方法, 上面在执行loop.run_in_executor
时就用过run
方法, 目的就是可以产生一个新的上下文变量给另外一个线程使用, 同时这个新的上下文变量跟原来的上下文变量是一致的.
执行run的时候,可以看出会copy一个新的上下文来调用传入的函数, 由于immutables.Map
的存在, 函数中对上下文的修改并不会影响旧的上下文变量, 达到进程复制数据时的写时复制的目的. 在run
方法的最后, 函数执行完了会再次set旧的上下文, 从而完成一次上下文切换.
def run(self, callable, *args, **kwargs): # 已经存在旧的context,抛出异常,防止多线程循环调用 if self._prev_context is not None: raise RuntimeError( 'cannot enter context: {} is already entered'.format(self)) self._prev_context = _get_context() # 保存当前的context try: _set_context(self) # 设置新的context return callable(*args, **kwargs) # 执行函数 finally: _set_context(self._prev_context) # 设置为旧的context self._prev_context = None
4.5 ContextVar
我们一般在使用contextvars模块时,经常使用的就是ContextVar
这个类了,这个类很简单,主要提供了set–设置值,get–获取值,reset–重置值三个方法, 从Context
类中写入和获取值, 而set和reset的就是通过上面的token类进行交互的.
set – 为当前上下文设置变量
def set(self, value): ctx = _get_context() # 获取当前上下文对象`Context` data = ctx._data try: old_value = data[self] # 获取Context旧对象 except KeyError: old_value = Token.MISSING # 获取不到则填充一个object(全局唯一) updated_data = data.set(self, value) # 设置新的值 ctx._data = updated_data return Token(ctx, self, old_value) # 返回带有旧值的token
get – 从当前上下文获取变量
def get(self, default=_NO_DEFAULT): ctx = _get_context() # 获取当前上下文对象`Context` try: return ctx[self] # 返回获取的值 except KeyError: pass if default is not _NO_DEFAULT: return default # 返回调用get时设置的值 if self._default is not _NO_DEFAULT: return self._default # 返回初始化context时设置的默认值 raise LookupError # 都没有则会抛错
reset – 清理本次用到的上下文数据
def reset(self, token): if token._used: # 判断token是否已经被使用 raise RuntimeError("Token has already been used once") if token._var is not self: # 判断token是否是当前contextvar返回的 raise ValueError( "Token was created by a different ContextVar") if token._context is not _get_context(): # 判断token的上下文是否跟contextvar上下文一致 raise ValueError( "Token was created in a different Context") ctx = token._context if token._old_value is Token.MISSING: # 如果没有旧值则删除该值 ctx._data = ctx._data.delete(token._var) else: # 有旧值则当前contextvar变为旧值 ctx._data = ctx._data.set(token._var, token._old_value) token._used = True # 设置flag,标记token已经被使用了
则此,contextvar的原理了解完了,接下来再看看他是如何在asyncio运行的.
5.contextvars asyncio
由于向下兼容的contextvars
并不支持asyncio, 所以这里通过aiotask-context的源码简要的了解如何在asyncio中如何获取和设置context。
5.1在asyncio中获取context
相比起contextvars复杂的概念,在asyncio中,我们可以很简单的获取到当前协程的task, 然后通过task就可以很方便的获取到task的context了,由于Pyhon3.7对asyncio的高级API 重新设计,所以可以看到需要对获取当前task进行封装
PY37 = sys.version_info >= (3, 7) if PY37: def asyncio_current_task(loop=None): """Return the current task or None.""" try: return asyncio.current_task(loop) except RuntimeError: # simulate old behaviour return None else: asyncio_current_task = asyncio.Task.current_task
不同的版本有不同的获取task方法, 之后我们就可以通过调用asyncio_current_task().context
即可获取到当前的上下文了…
5.2 对上下文的操作
同样的,在得到上下文后, 我们这里也需要set, get, reset的操作,不过十分简单, 类似dict一样的操作即可, 它没有token的逻辑:
set
def set(key, value): """ Sets the given value inside Task.context[key]. If the key does not exist it creates it. :param key: identifier for accessing the context dict. :param value: value to store inside context[key]. :raises """ current_task = asyncio_current_task() if not current_task: raise ValueError(NO_LOOP_EXCEPTION_MSG.format(key)) current_task.context[key] = value
get
def get(key, default=None): """ Retrieves the value stored in key from the Task.context dict. If key does not exist, or there is no event loop running, default will be returned :param key: identifier for accessing the context dict. :param default: None by default, returned in case key is not found. :return: Value stored inside the dict[key]. """ current_task = asyncio_current_task() if not current_task: raise ValueError(NO_LOOP_EXCEPTION_MSG.format(key)) return current_task.context.get(key, default)
clear – 也就是contextvar.ContextVars
中的reset
def clear(): """ Clear the Task.context. :raises ValueError: if no current task. """ current_task = asyncio_current_task() if not current_task: raise ValueError("No event loop found") current_task.context.clear()
5.2 copying_task_factory和chainmap_task_factory
在Python的更高级版本中,已经支持设置context了,所以这两个方法可以不再使用了.他们最后都用到了task_factory
的方法.task_factory
简单说就是创建一个新的task,再通过工厂方法合成context,最后把context设置到task
def task_factory(loop, coro, copy_context=False, context_factory=None): """ By default returns a task factory that uses a simple dict as the task context, but allows context creation and inheritance to be customized via ``context_factory``. """ # 生成context工厂函数 context_factory = context_factory or partial( dict_context_factory, copy_context=copy_context) # 创建task, 跟asyncio.ensure_future一样 task = asyncio.tasks.Task(coro, loop=loop) if task._source_traceback: del [-1] # 获取task的context try: context = asyncio_current_task(loop=loop).context except AttributeError: context = None # 从context工厂中处理context并赋值在task task.context = context_factory(context) return task
aiotask-context
提供了两个对context处理的函数dict_context_factory
和chainmap_context_factory
.在aiotask-context
中,context是一个dict对象,dict_context_factory
可以选择赋值或者设置新的context
def dict_context_factory(parent_context=None, copy_context=False): """A traditional ``dict`` context to keep things simple""" if parent_context is None: # initial context return {} else: # inherit context new_context = parent_context if copy_context: new_context = deepcopy(new_context) return new_context
chainmap_context_factory
与dict_context_factory
的区别就是在合并context而不是直接继承.同时借用ChainMap
保证合并context后,还能同步context的改变
def chainmap_context_factory(parent_context=None): """ A ``ChainMap`` context, to avoid copying any data and yet preserve strict one-way inheritance (just like with dict copying) """ if parent_context is None: # initial context return ChainMap() else: # inherit context if not isinstance(parent_context, ChainMap): # if a dict context was previously used, then convert # (without modifying the original dict) parent_context = ChainMap(parent_context) return parent_context.new_child()
The above is the detailed content of How to use contextvars module in python. For more information, please follow other related articles on the PHP Chinese website!
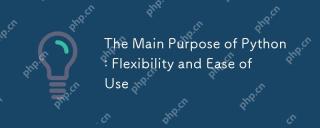
Python's flexibility is reflected in multi-paradigm support and dynamic type systems, while ease of use comes from a simple syntax and rich standard library. 1. Flexibility: Supports object-oriented, functional and procedural programming, and dynamic type systems improve development efficiency. 2. Ease of use: The grammar is close to natural language, the standard library covers a wide range of functions, and simplifies the development process.
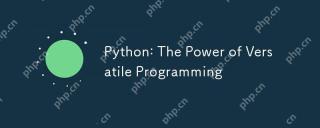
Python is highly favored for its simplicity and power, suitable for all needs from beginners to advanced developers. Its versatility is reflected in: 1) Easy to learn and use, simple syntax; 2) Rich libraries and frameworks, such as NumPy, Pandas, etc.; 3) Cross-platform support, which can be run on a variety of operating systems; 4) Suitable for scripting and automation tasks to improve work efficiency.
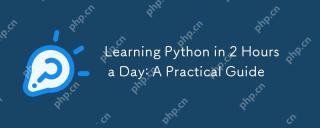
Yes, learn Python in two hours a day. 1. Develop a reasonable study plan, 2. Select the right learning resources, 3. Consolidate the knowledge learned through practice. These steps can help you master Python in a short time.
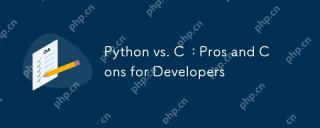
Python is suitable for rapid development and data processing, while C is suitable for high performance and underlying control. 1) Python is easy to use, with concise syntax, and is suitable for data science and web development. 2) C has high performance and accurate control, and is often used in gaming and system programming.
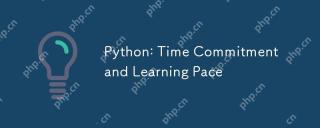
The time required to learn Python varies from person to person, mainly influenced by previous programming experience, learning motivation, learning resources and methods, and learning rhythm. Set realistic learning goals and learn best through practical projects.
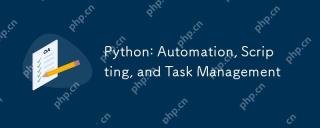
Python excels in automation, scripting, and task management. 1) Automation: File backup is realized through standard libraries such as os and shutil. 2) Script writing: Use the psutil library to monitor system resources. 3) Task management: Use the schedule library to schedule tasks. Python's ease of use and rich library support makes it the preferred tool in these areas.
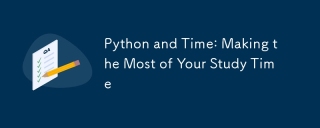
To maximize the efficiency of learning Python in a limited time, you can use Python's datetime, time, and schedule modules. 1. The datetime module is used to record and plan learning time. 2. The time module helps to set study and rest time. 3. The schedule module automatically arranges weekly learning tasks.
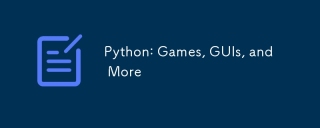
Python excels in gaming and GUI development. 1) Game development uses Pygame, providing drawing, audio and other functions, which are suitable for creating 2D games. 2) GUI development can choose Tkinter or PyQt. Tkinter is simple and easy to use, PyQt has rich functions and is suitable for professional development.


Hot AI Tools

Undresser.AI Undress
AI-powered app for creating realistic nude photos

AI Clothes Remover
Online AI tool for removing clothes from photos.

Undress AI Tool
Undress images for free

Clothoff.io
AI clothes remover

AI Hentai Generator
Generate AI Hentai for free.

Hot Article

Hot Tools

MinGW - Minimalist GNU for Windows
This project is in the process of being migrated to osdn.net/projects/mingw, you can continue to follow us there. MinGW: A native Windows port of the GNU Compiler Collection (GCC), freely distributable import libraries and header files for building native Windows applications; includes extensions to the MSVC runtime to support C99 functionality. All MinGW software can run on 64-bit Windows platforms.
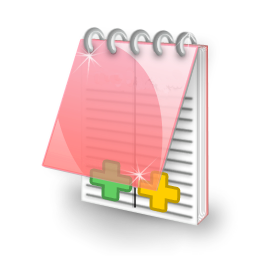
EditPlus Chinese cracked version
Small size, syntax highlighting, does not support code prompt function

SublimeText3 Chinese version
Chinese version, very easy to use

SublimeText3 Linux new version
SublimeText3 Linux latest version

Zend Studio 13.0.1
Powerful PHP integrated development environment