When it comes to AI infrastructure, should you choose edge or cloud?
Nowadays, edge computing has been a hot topic. Hailed as the most exciting technological shift in recent years, there’s a lot of talk about its transformative power!
With increasingly powerful AI/ML algorithms redefining “intelligence” and the availability of cheaper, more powerful “edge” devices, the hype is largely real. But if you think about the history of edge computing, it's much earlier than recent interest would lead us to believe.
In fact, computing and intelligence originally started at the edge, when high-bandwidth network connectivity was almost non-existent for most applications. Even in the late 1990s, critical measurement equipment deployed remotely in factories or fields often had dedicated computing power to process incoming sensor data. However, the algorithms in these devices are only rudimentary in terms of "intelligence": primarily signal processing or data conversion.
Cloud-based computing began to gain traction in the late 2000s as network capabilities improved and connectivity increased. At the same time, powerful artificial intelligence algorithms are gaining traction as a means of unlocking meaningful information from large amounts of structured and unstructured data. In just a decade, cloud AI has become the go-to choice for AI applications.
However, the shift to the cloud also brings several issues: data upload and download costs, network reliability and data security, etc. At the same time, edge computing’s trade-offs between processing power and cost or floor space are diminishing with the rise of affordable yet powerful edge devices. It seems we are now back to considering “edge computing” as a viable and attractive option for building smart applications.
As the debate rages over which is better, edge AI or cloud AI, anyone familiar with both frameworks is likely to respond “It depends.” !". The reason is that edge and cloud infrastructure are not competing, but complementary frameworks.
Both have experienced tremendous development and refinement over the past few years, particularly as a foundation for artificial intelligence development and deployment. As with any technology choice, the choice really comes down to the specific application: goals, value drivers, economics, and any constraints on power consumption, size, and connectivity. Therefore, it’s important to understand the pros and cons of cloud and edge AI before trying to set up the right infrastructure.
Cloud-based AI is an attractive option when looking for flexibility, scalability, and ease of deployment. Today, most cloud service providers offer powerful frameworks for training and deployment of AI models and offer pay-as-you-go packages with little upfront commitment or investment. The cloud offers virtually unlimited computing and storage options, making it particularly suitable for large AI models.
But for real-time applications that require continuous evaluation of sensor or image data, this can become an unwieldy option because they must transmit data back and forth, incurring huge costs. This data transfer also makes the cloud largely unsuitable for low-latency applications that require closed-loop control or instant action.
On the other hand, edge AI is a reasonable choice for real-time data analysis for automatic alarms or closed-loop control. While edge infrastructure does require an upfront investment in edge hardware, operating costs are much lower compared to the cloud.
Today, there are a variety of edge AI hardware options available, including NPUs (Neural Processing Units), TPUs (Tensor Processing Units), and SOCs (Systems on a Chip) with dedicated AI accelerators and SoMs (systems on modules). Low-cost and low-power hardware for artificial intelligence is an active area of research and has the potential to provide better options. On the other hand, AI-based consumer applications must deal with a fairly diverse range of edge devices (phones, tablets, PCs, etc.), making edge deployment a potentially daunting prospect.
As a result, edge infrastructure may not be conducive to rapid prototyping or easy to scale. While the concept of federated learning, distributed training of AI models allows for training and deployment at the edge, the cloud remains the logical choice for training large models that require sufficient computing power.
But the solution doesn’t have to be an either/or choice. As applications transition to more microservices-based architectures, they can be broken down into smaller functions or microservices with their own specific deployment frameworks. So instead of having to choose between cloud and edge, the focus can be on using both optimally for specific applications.
For example, an application might start as a rapid prototype on the cloud. As it evolves, functions that require low latency and real-time decision-making can be moved to the edge, while those that require scale and flexibility can remain in the cloud. Model training or retraining can be managed centrally in the cloud, while some federated learning at the edge can improve accuracy locally. Likewise, sensitive data can be processed at the edge and more general data can be moved to the cloud.
Organizations, developers, and practitioners would be better off thinking of cloud and edge not as distinct alternatives, but as a continuum from edge to cloud, with many different infrastructure options in between. This includes different types of edges – operational edge, network edge, mobile endpoints, etc., as well as different types of distributed processing on the network – private clouds, public clouds, cloudlets, fog computing, etc.
While complexity can be a challenge, finding the right combination of technologies begins to offer organizations a unique opportunity to maximize the value of AI while minimizing costs and risks.
The above is the detailed content of When it comes to AI infrastructure, should you choose edge or cloud?. For more information, please follow other related articles on the PHP Chinese website!
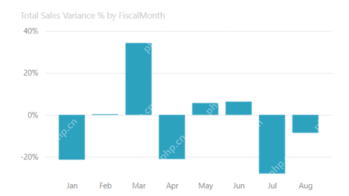
Harnessing the Power of Data Visualization with Microsoft Power BI Charts In today's data-driven world, effectively communicating complex information to non-technical audiences is crucial. Data visualization bridges this gap, transforming raw data i
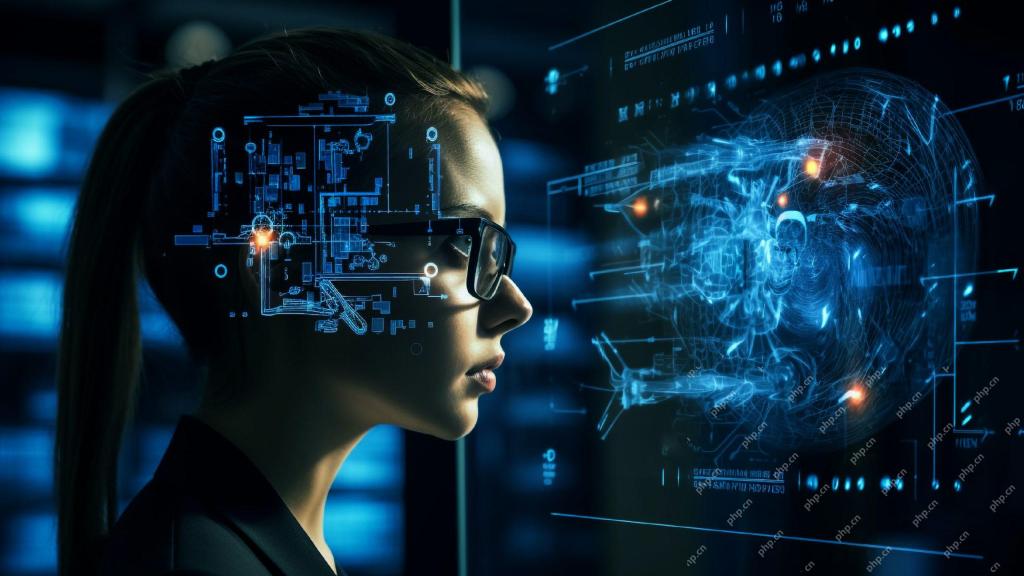
Expert Systems: A Deep Dive into AI's Decision-Making Power Imagine having access to expert advice on anything, from medical diagnoses to financial planning. That's the power of expert systems in artificial intelligence. These systems mimic the pro
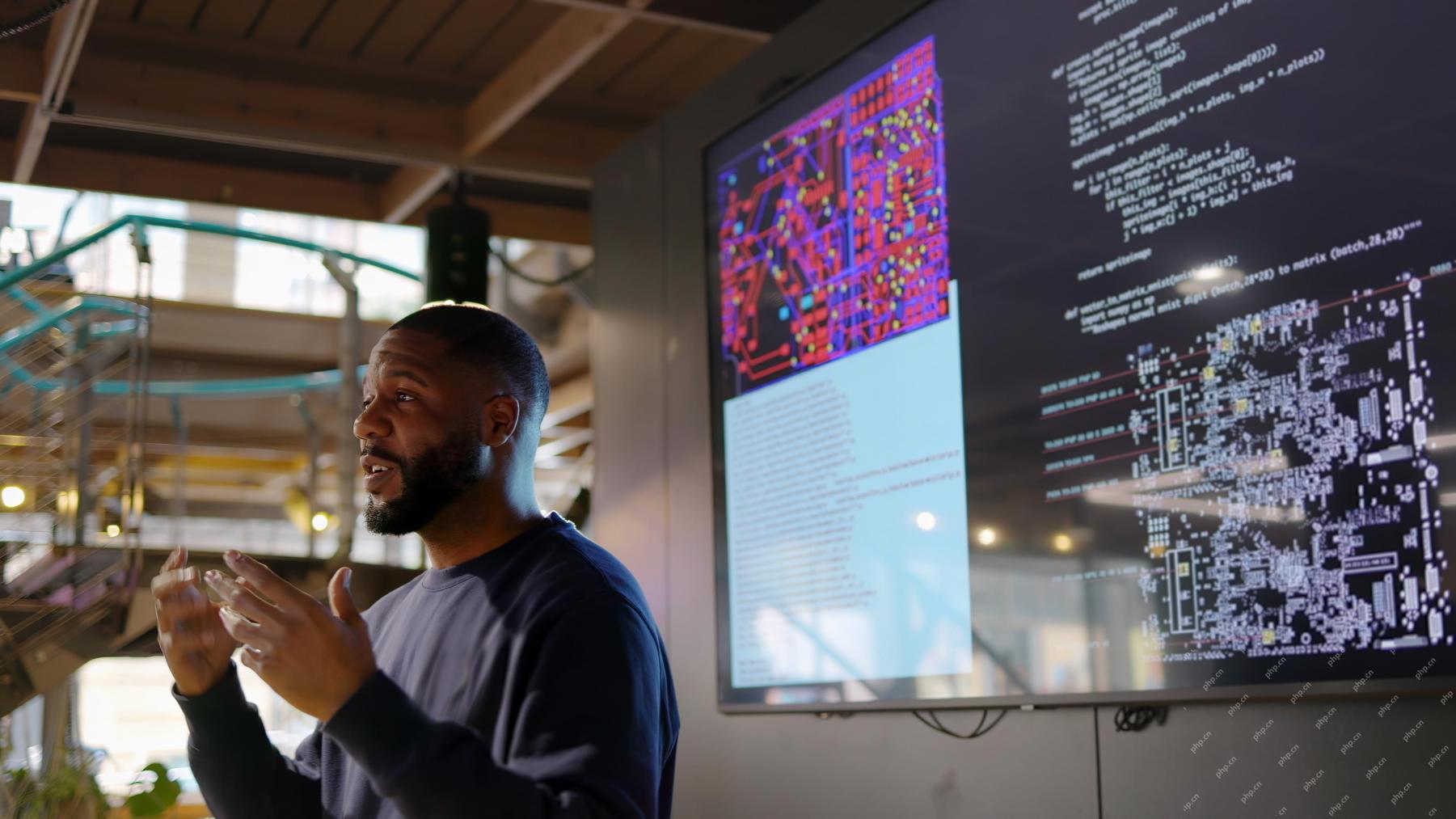
First of all, it’s apparent that this is happening quickly. Various companies are talking about the proportions of their code that are currently written by AI, and these are increasing at a rapid clip. There’s a lot of job displacement already around
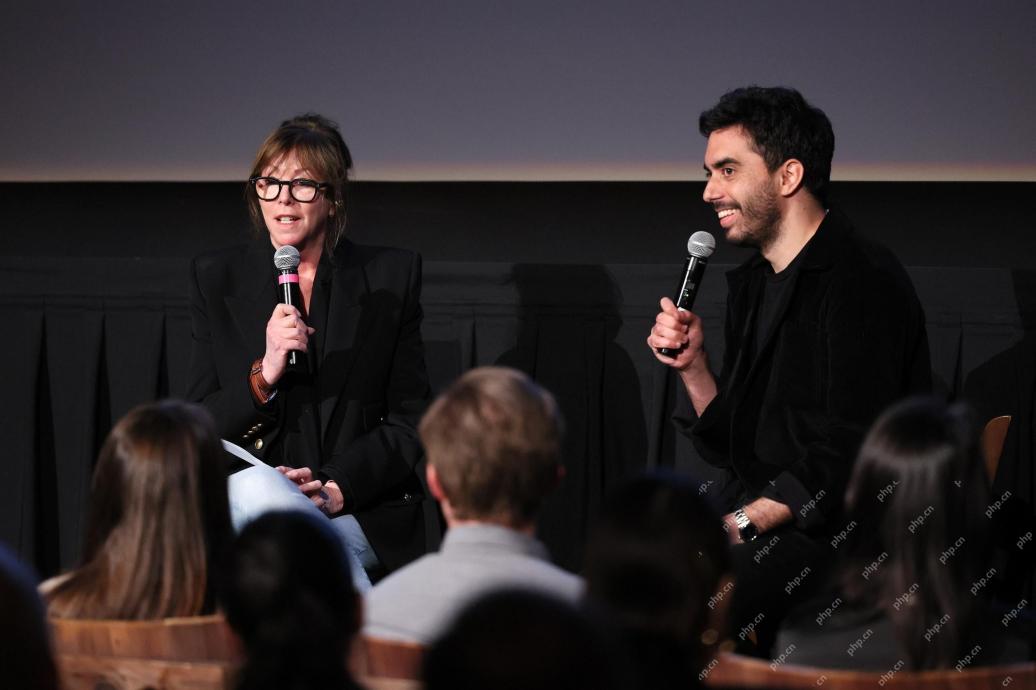
The film industry, alongside all creative sectors, from digital marketing to social media, stands at a technological crossroad. As artificial intelligence begins to reshape every aspect of visual storytelling and change the landscape of entertainment
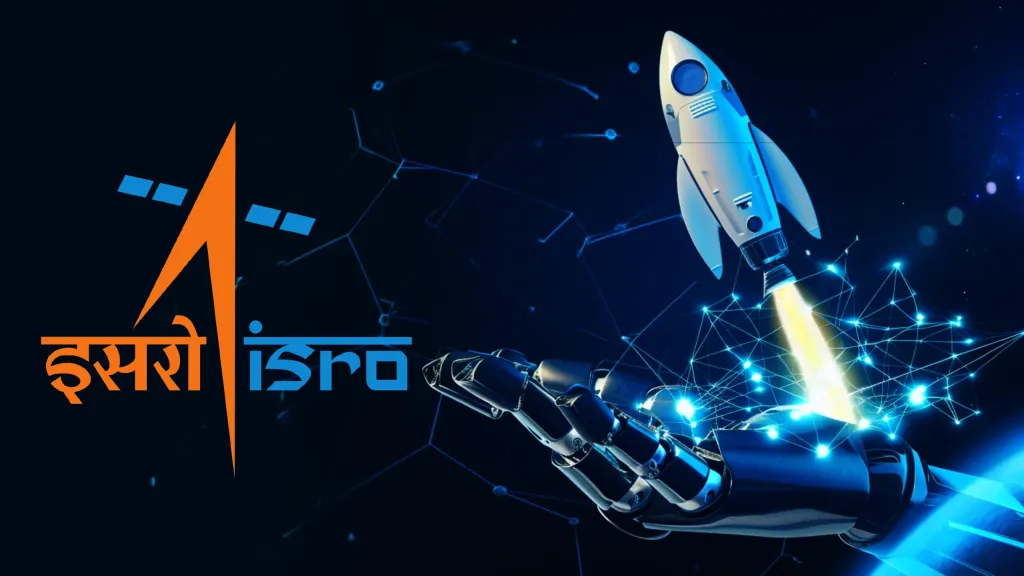
ISRO's Free AI/ML Online Course: A Gateway to Geospatial Technology Innovation The Indian Space Research Organisation (ISRO), through its Indian Institute of Remote Sensing (IIRS), is offering a fantastic opportunity for students and professionals to
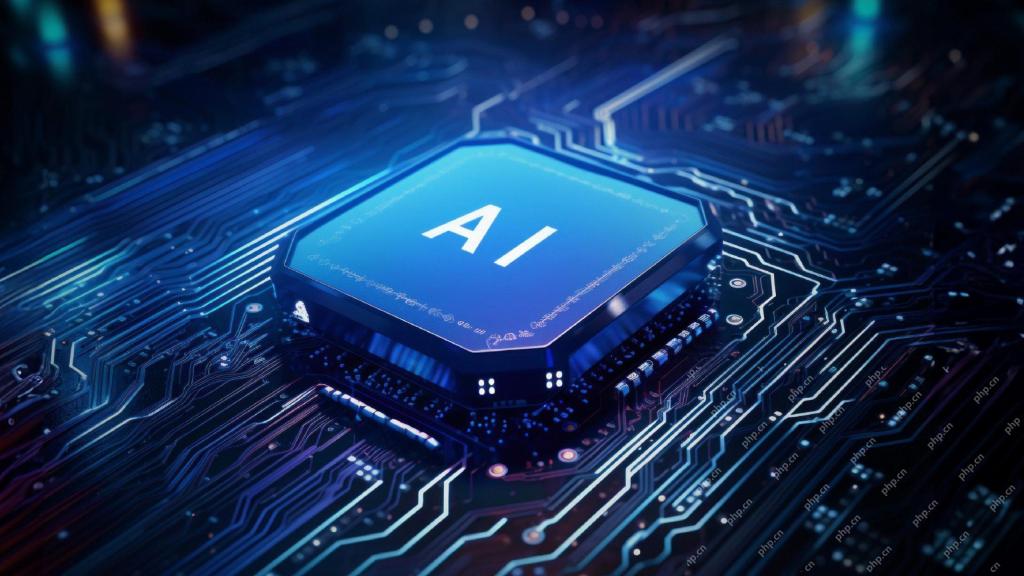
Local Search Algorithms: A Comprehensive Guide Planning a large-scale event requires efficient workload distribution. When traditional approaches fail, local search algorithms offer a powerful solution. This article explores hill climbing and simul
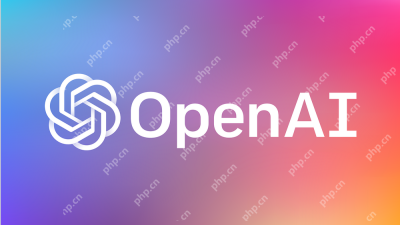
The release includes three distinct models, GPT-4.1, GPT-4.1 mini and GPT-4.1 nano, signaling a move toward task-specific optimizations within the large language model landscape. These models are not immediately replacing user-facing interfaces like
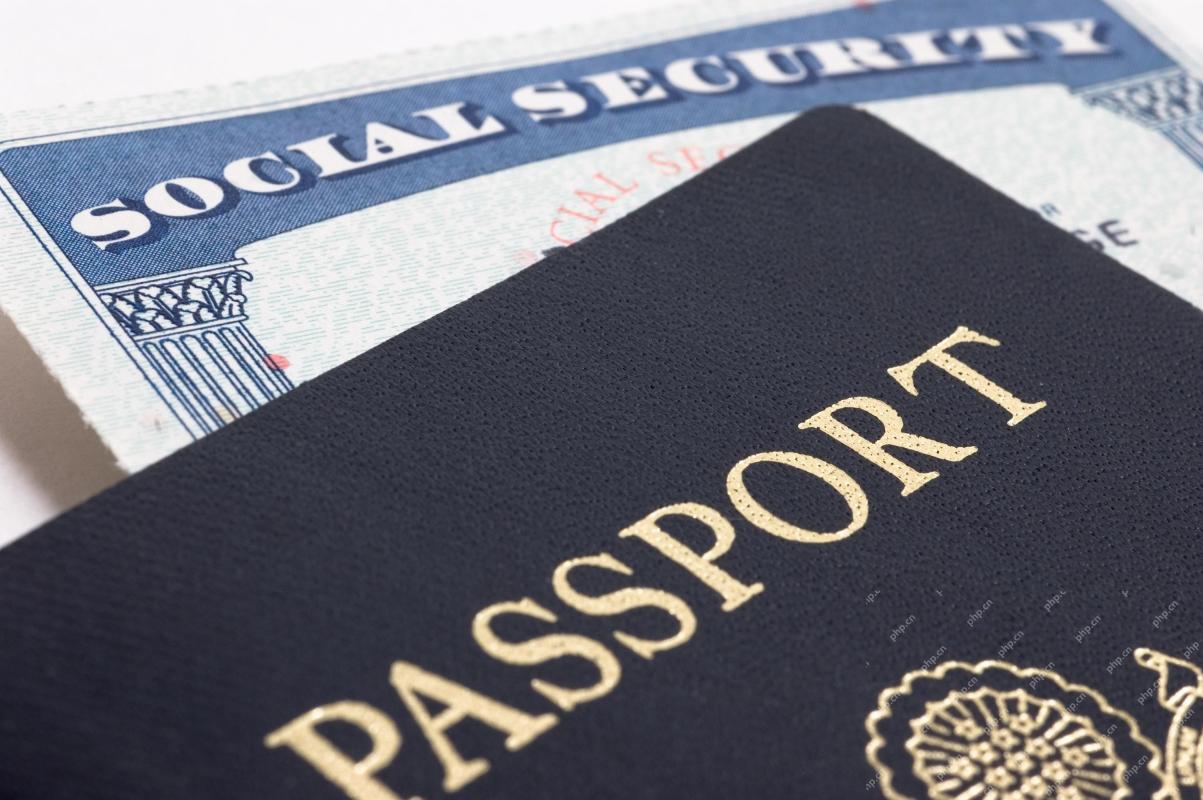
Chip giant Nvidia said on Monday it will start manufacturing AI supercomputers— machines that can process copious amounts of data and run complex algorithms— entirely within the U.S. for the first time. The announcement comes after President Trump si


Hot AI Tools

Undresser.AI Undress
AI-powered app for creating realistic nude photos

AI Clothes Remover
Online AI tool for removing clothes from photos.

Undress AI Tool
Undress images for free

Clothoff.io
AI clothes remover

AI Hentai Generator
Generate AI Hentai for free.

Hot Article

Hot Tools
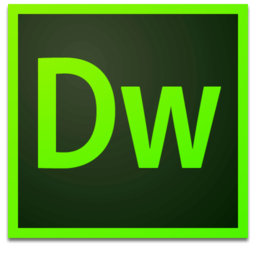
Dreamweaver Mac version
Visual web development tools
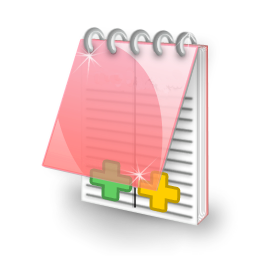
EditPlus Chinese cracked version
Small size, syntax highlighting, does not support code prompt function

Atom editor mac version download
The most popular open source editor
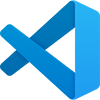
VSCode Windows 64-bit Download
A free and powerful IDE editor launched by Microsoft
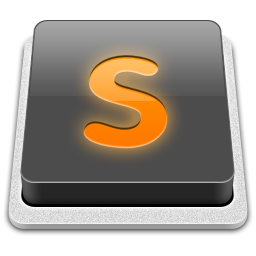
SublimeText3 Mac version
God-level code editing software (SublimeText3)