


More than a month ago, OpenAI’s GPT-4 came out. In addition to various excellent visual demonstrations, it also implements an important update: it can handle context tokens that are 8k in length by default, but can be up to 32K (approximately 50 pages of text). This means that when asking questions to GPT-4, we can enter much longer text than before. This greatly expands the application scenarios of GPT-4 and can better handle long conversations, long texts, and file search and analysis.
However, this record was quickly broken: CoLT5 from Google Research expanded the context token length that the model can handle to 64k .
Such a breakthrough is not easy, because these models using the Transformer architecture all face a problem: Transformer processing long documents is computationally very expensive, because the attention cost increases with the input The length grows quadratically, making it increasingly difficult to apply large models to longer inputs.
Despite this, researchers are still making breakthroughs in this direction. A few days ago, a study from the open source dialogue AI technology stack DeepPavlov and other institutions showed that:By using an architecture called Recurrent Memory Transformer (RMT), they can increase the effective context length of the BERT model to 2 million tokens (approximately equivalent to 3,200 pages of text according to OpenAI’s calculation method), while maintaining high memory retrieval accuracy (Note: Recurrent Memory Transformer was proposed by Aydar Bulatov et al. in a paper at NeurIPS 2022 Methods). The new method allows the storage and processing of local and global information, and the flow of information between segments of the input sequence through the use of recurrence.
The author stated that by using the simple token-based algorithm introduced by Bulatov et al. in the article "Recurrent Memory Transformer" Memory mechanism, they can combine RMT with pre-trained Transformer models such as BERT, and use an Nvidia GTX 1080Ti GPU to perform full attention and full precision operations on sequences of more than 1 million tokens.
Paper address: https://arxiv.org/pdf/2304.11062.pdf
However, some people have reminded that this is not a real "free lunch". The improvement of the above-mentioned papers is obtained by "longer reasoning time and substantial decrease in quality". . Therefore, it is not yet a revolution, but it may become the basis for the next paradigm (tokens may be infinitely long).
Recurrent Memory Transformer
This study adopts the method Recurrent Memory Transformer proposed by Bulatov et al. in 2022 (RMT) and change it to a plug-and-play method. The main mechanism is as shown in the figure below:
Long input is split into multiple segments, and a memory vector is added before the first segment embedding and processed together with the segment token. For pure encoder models like BERT, the memory is only added once at the beginning of the segment, unlike (Bulatov et al., 2022), where the pure decoder model divides the memory into read and write parts. For time step τ and segment
, the loop executes as follows:
Where, N is the number of layers of Transformer. After forward propagation,
# contains the updated memory token of segment τ.
The segments of the input sequence are processed in order. To enable circular connections, the study passes the output of the memory token from the current segment to the input of the next segment:
Both memory and looping in RMT are based only on global memory tokens. This allows the backbone Transformer to remain unchanged, making RMT's memory enhancement capabilities compatible with any Transformer model.
Computational efficiency
This study estimates the FLOPs required for RMT and Transformer models of different sizes and sequence lengths .
As shown in Figure 3 below, if the length of the segment is fixed, RMT can scale linearly for any model size. This study achieves linear scaling by dividing the input sequence into segments and computing the complete attention matrix only within segment boundaries.
Due to the high computational complexity of the FFN layer, larger Transformer models tend to exhibit slower quadratic scaling with sequence length. However, for very long sequences larger than 32000, they fall back to quadratic expansion. For sequences with more than one segment (> 512 in this study), RMT requires fewer FLOPs than acyclic models and can reduce the number of FLOPs by up to 295 times. RMT provides a greater relative reduction in FLOP for smaller models, but the 29x reduction in FLOP for the OPT-175B model is significant in absolute terms.
Memory Task
To test memory abilities, the study constructed synthetic datasets that required memorization of simple facts and basic reasoning. Task input consists of one or more facts and a question that can only be answered using all the facts. To increase the difficulty of the task, the study also added natural language text unrelated to the question or answer to act as noise, so the model was tasked with separating facts from irrelevant text and using the facts to answer the question.
Fact memory
First item The task is to test RMT's ability to write and store information in memory for long periods of time, as shown at the top of Figure 4 below. In the simplest case, the facts tend to be at the beginning of the input, and the questions are always at the end. The amount of irrelevant text between questions and answers gradually increases to the point where the entire input does not fit into a single model input.
##Fact Detection and Memory
Fact detection increases the difficulty of the task by moving a fact to a random position in the input, as shown in the middle of Figure 4 above. This requires the model to first distinguish the fact from irrelevant text, write the fact into memory, and then use it to answer the question at the end.
Using memorized facts to reason
Another operation of memory is to reason using memorized facts and the current context. To evaluate this functionality, the researchers used a more complex task in which two facts were generated and placed within an input sequence, as shown at the bottom of Figure 4 above. The question asked at the end of the sequence is described in such a way that arbitrary facts must be used to answer the question correctly.
Experimental results
The researchers used 4 to 8 NVIDIA 1080ti GPUs to train and evaluate the model . For longer sequences, they used a single 40GB NVIDIA A100 to speed up evaluation.
Course Learning
The researchers observed that using the training plan can significantly improve the accuracy and accuracy of the solutions. stability. Initially, RMT is trained on a shorter version of the task and increases the task length by adding another segment as the training converges. The course learning process continues until the required input length is reached.
In the experiment, the researchers first started with a sequence suitable for a single segment. The actual segment size is 499, but due to BERT's 3 special tokens and 10 memory placeholders retained from the model input, the size is 512. They note that after training on a shorter task, RMT is easier to solve longer versions of the task, thanks to the fact that it uses fewer training steps to converge to a perfect solution.
Extrapolation ability
What is the generalization ability of RMT to different sequence lengths? To answer this question, the researchers evaluated models trained on different numbers of segments to solve longer tasks, as shown in Figure 5 below.
They observed that models tend to perform better on shorter tasks, with the only exception being the single-segment inference task, which becomes Very difficult to solve. One possible explanation is that because the task size exceeds one segment, the model no longer "expects" problems in the first segment, resulting in a decrease in quality.
Interestingly, RMT’s ability to generalize to longer sequences also appears as the number of training segments increases. After training on 5 or more segments, RMT can generalize nearly perfectly to tasks twice as long.
In order to test the limitations of generalization, the researchers increased the verification task size to 4096 segments or 2,043,904 tokens (as shown in Figure 1 above). RMT performed on such a long sequence Performed surprisingly well. Detection and memory tasks are the simplest, and reasoning tasks are the most complex.
Attention pattern of memory operations
In Figure 6 below, by examining the RMT attention on a specific segment, the researcher observed that the memory operation corresponds to specific attention. model. Furthermore, the high extrapolation performance on extremely long sequences in Section 5.2 demonstrates the effectiveness of the learned memory operations, even when used thousands of times.
Please refer to the original paper for more technical and experimental details.
The above is the detailed content of True Quantum Speed Reading: Breaking through the limit of GPT-4 that can only understand 50 pages of text at a time, new research extends to millions of tokens. For more information, please follow other related articles on the PHP Chinese website!
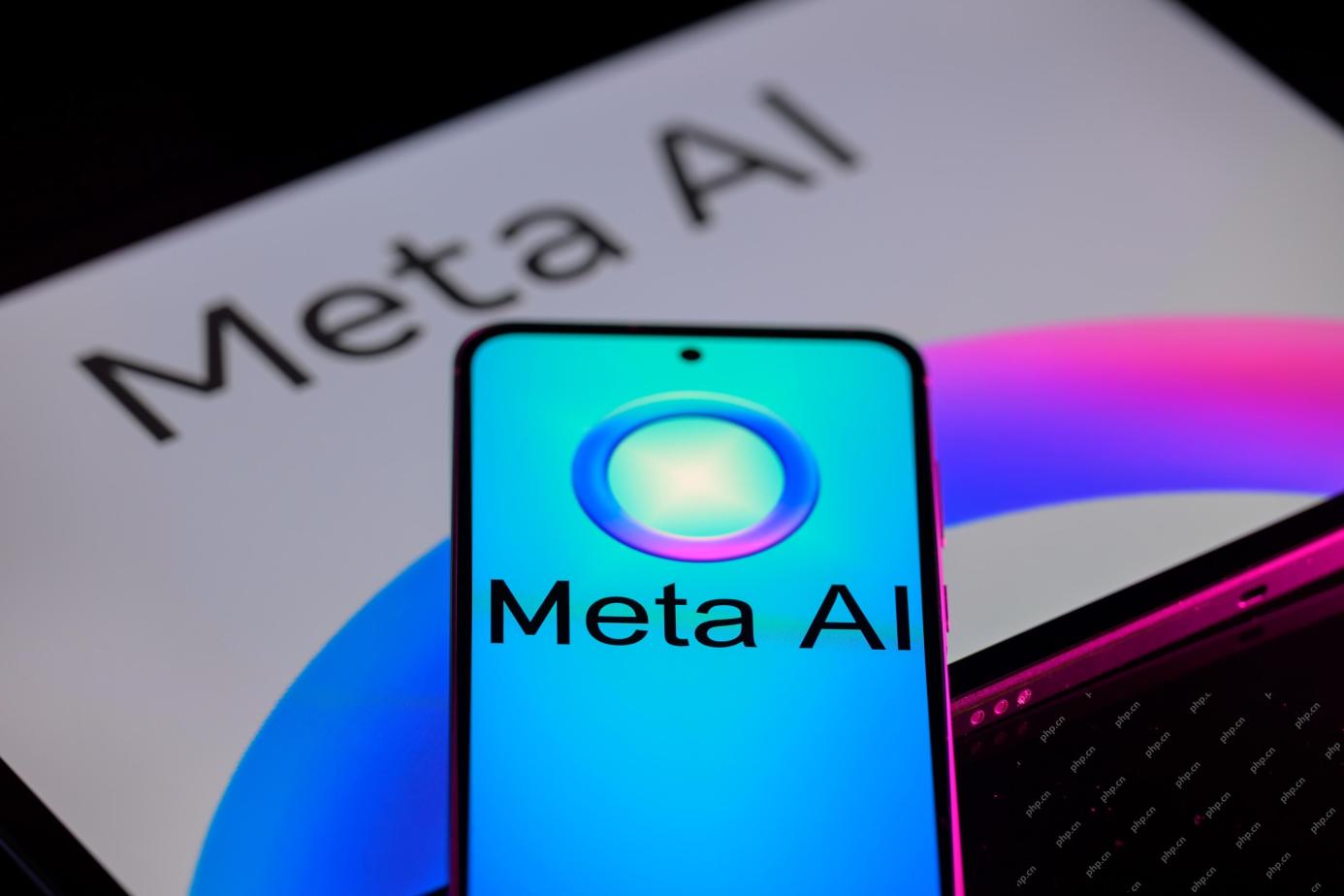
Meta has joined hands with partners such as Nvidia, IBM and Dell to expand the enterprise-level deployment integration of Llama Stack. In terms of security, Meta has launched new tools such as Llama Guard 4, LlamaFirewall and CyberSecEval 4, and launched the Llama Defenders program to enhance AI security. In addition, Meta has distributed $1.5 million in Llama Impact Grants to 10 global institutions, including startups working to improve public services, health care and education. The new Meta AI application powered by Llama 4, conceived as Meta AI
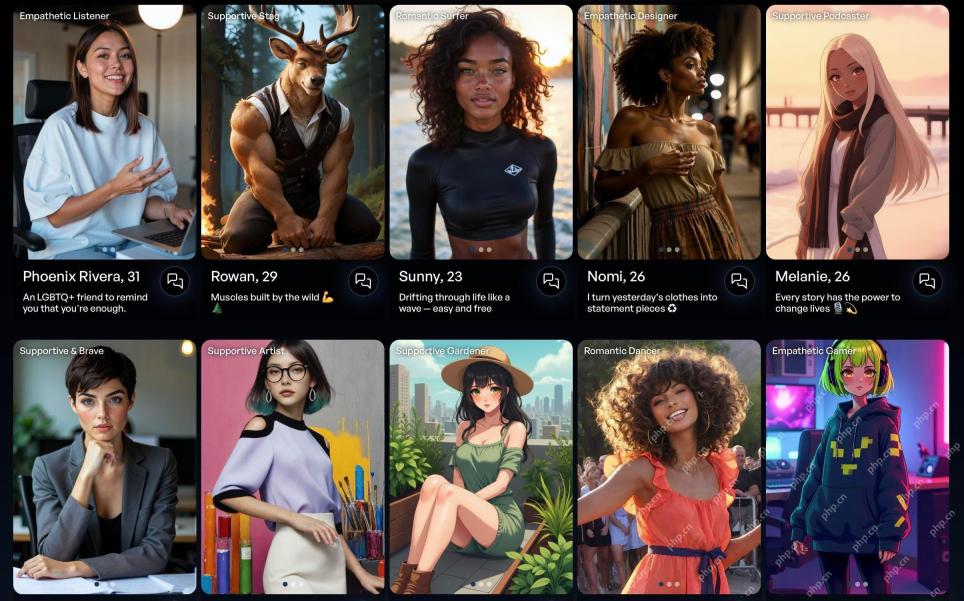
Joi AI, a company pioneering human-AI interaction, has introduced the term "AI-lationships" to describe these evolving relationships. Jaime Bronstein, a relationship therapist at Joi AI, clarifies that these aren't meant to replace human c
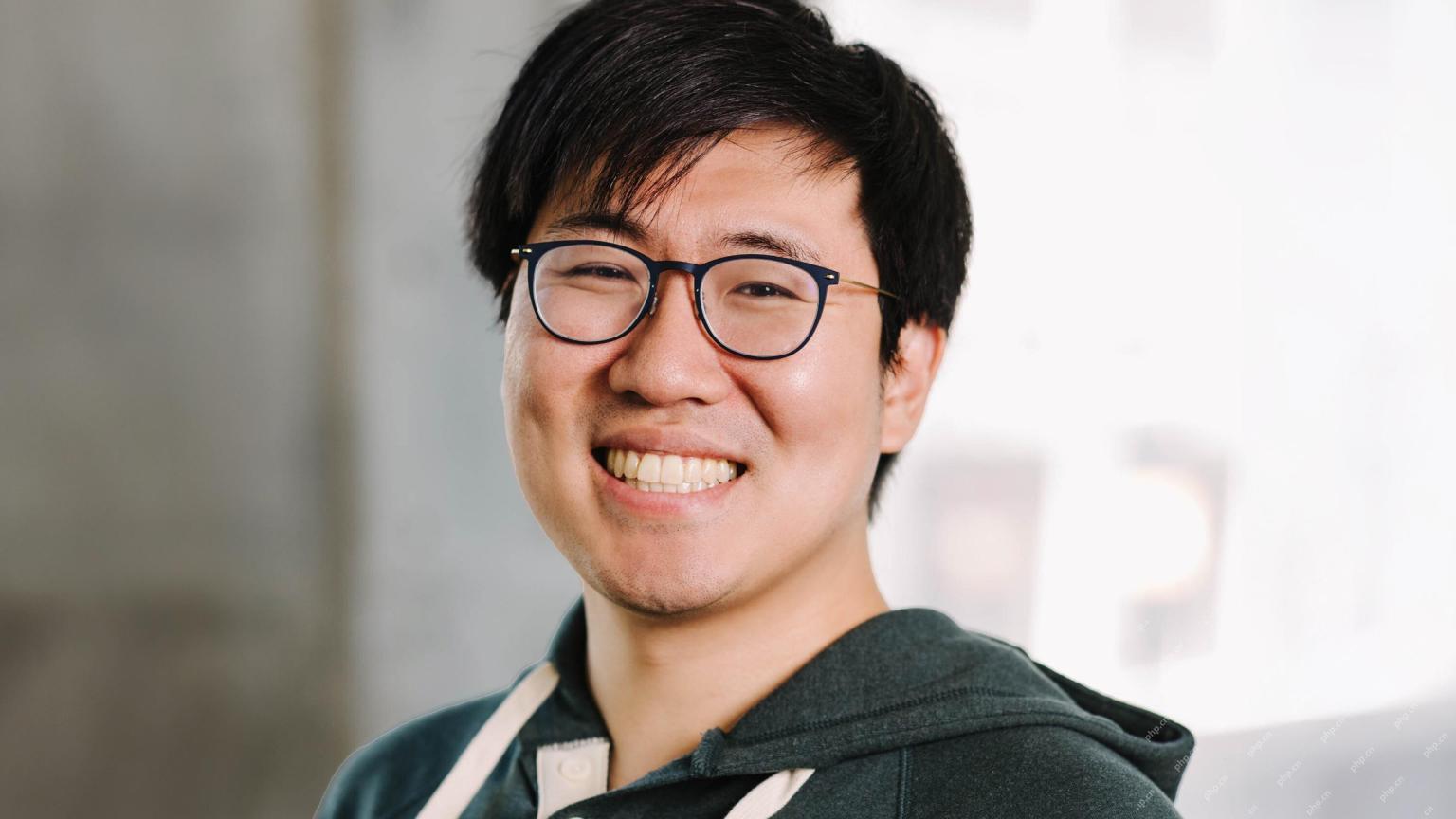
Online fraud and bot attacks pose a significant challenge for businesses. Retailers fight bots hoarding products, banks battle account takeovers, and social media platforms struggle with impersonators. The rise of AI exacerbates this problem, rende
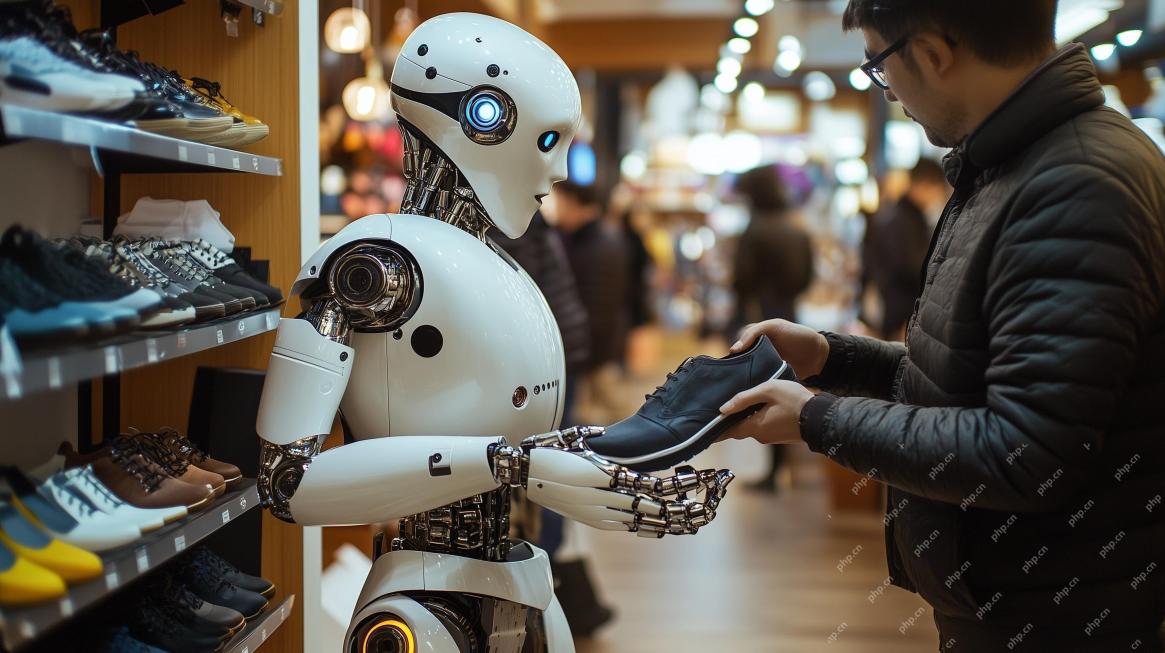
AI agents are poised to revolutionize marketing, potentially surpassing the impact of previous technological shifts. These agents, representing a significant advancement in generative AI, not only process information like ChatGPT but also take actio
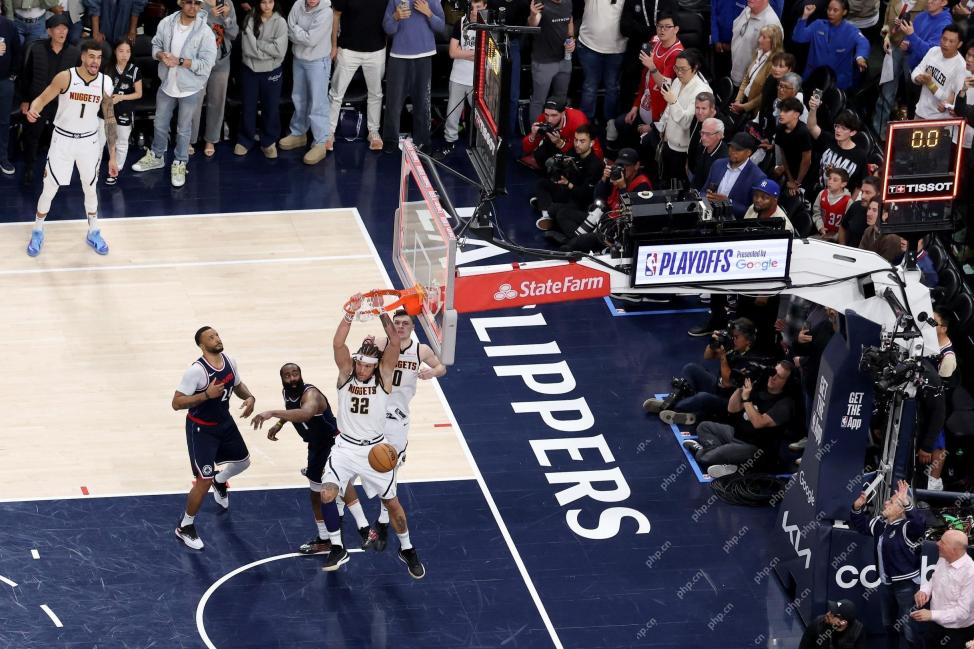
AI's Impact on Crucial NBA Game 4 Decisions Two pivotal Game 4 NBA matchups showcased the game-changing role of AI in officiating. In the first, Denver's Nikola Jokic's missed three-pointer led to a last-second alley-oop by Aaron Gordon. Sony's Haw
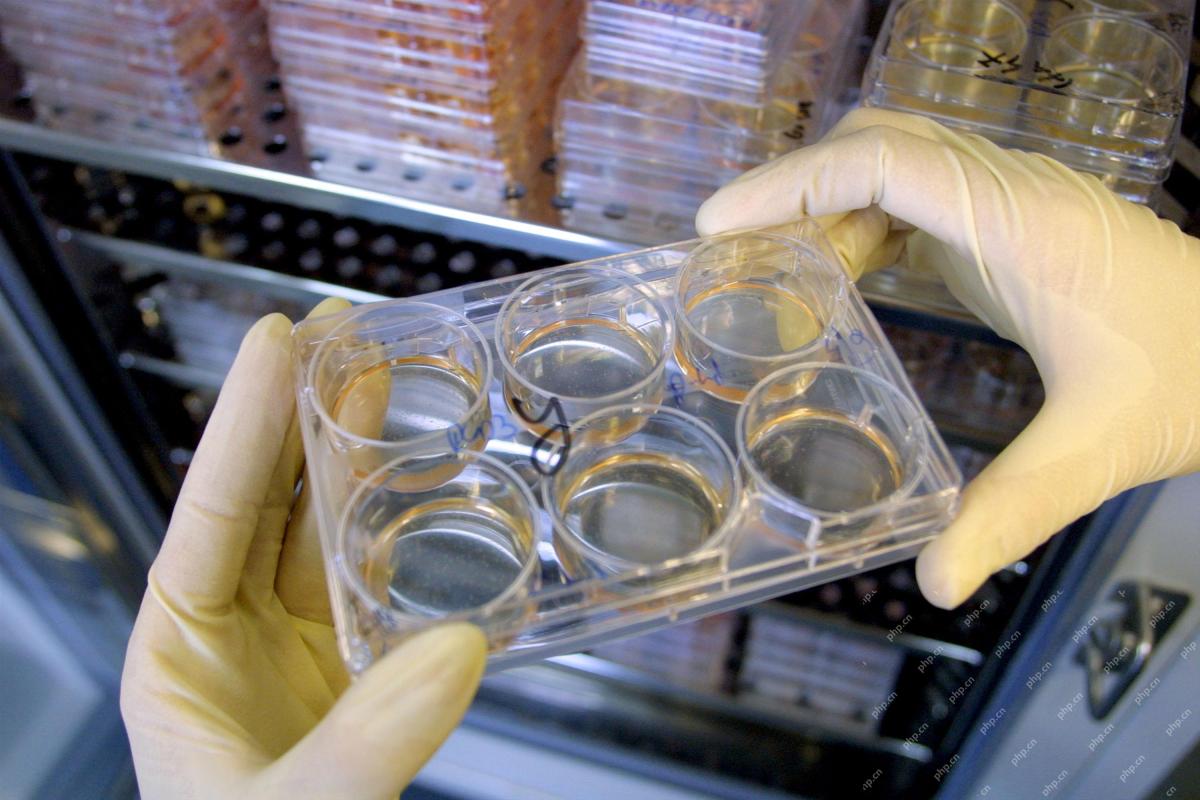
Traditionally, expanding regenerative medicine expertise globally demanded extensive travel, hands-on training, and years of mentorship. Now, AI is transforming this landscape, overcoming geographical limitations and accelerating progress through en
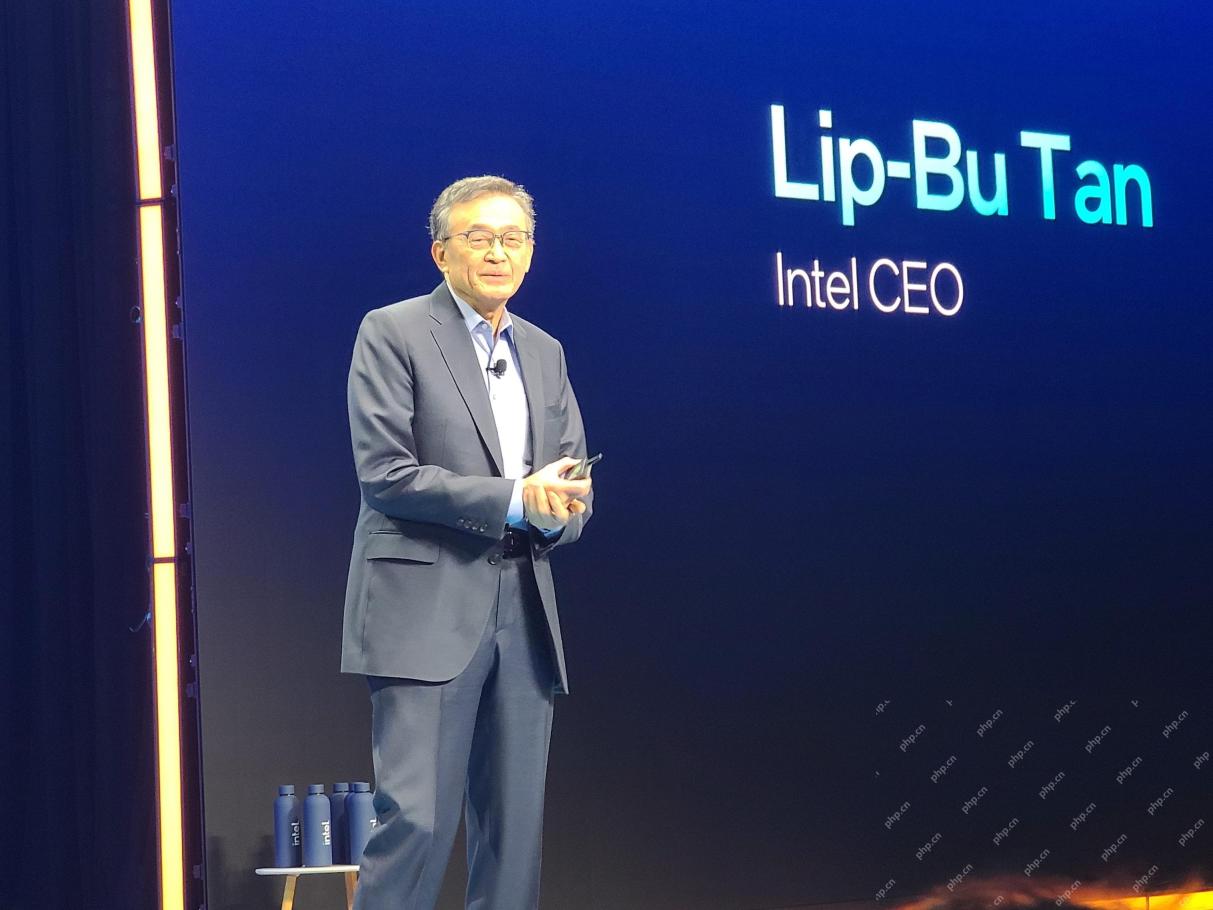
Intel is working to return its manufacturing process to the leading position, while trying to attract fab semiconductor customers to make chips at its fabs. To this end, Intel must build more trust in the industry, not only to prove the competitiveness of its processes, but also to demonstrate that partners can manufacture chips in a familiar and mature workflow, consistent and highly reliable manner. Everything I hear today makes me believe Intel is moving towards this goal. The keynote speech of the new CEO Tan Libo kicked off the day. Tan Libai is straightforward and concise. He outlines several challenges in Intel’s foundry services and the measures companies have taken to address these challenges and plan a successful route for Intel’s foundry services in the future. Tan Libai talked about the process of Intel's OEM service being implemented to make customers more
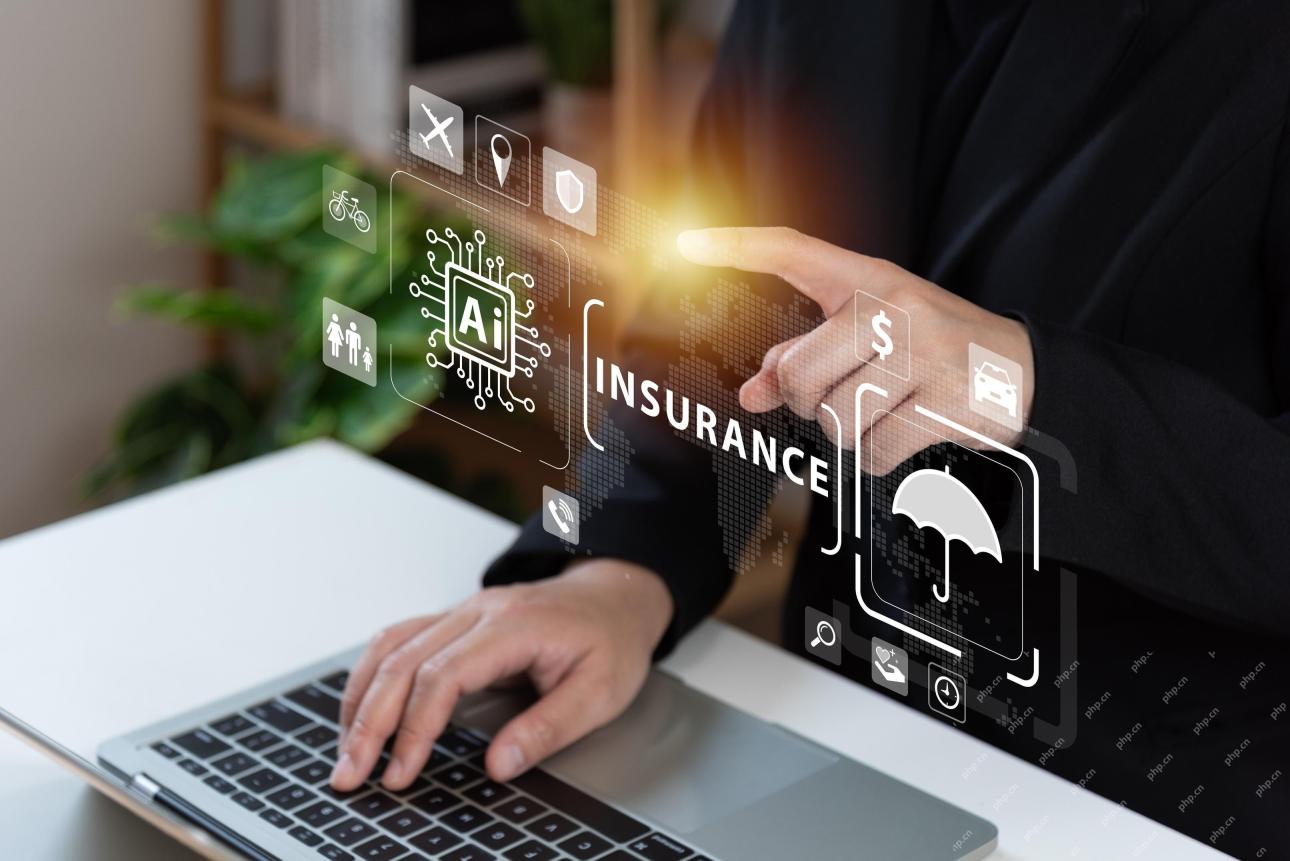
Addressing the growing concerns surrounding AI risks, Chaucer Group, a global specialty reinsurance firm, and Armilla AI have joined forces to introduce a novel third-party liability (TPL) insurance product. This policy safeguards businesses against


Hot AI Tools

Undresser.AI Undress
AI-powered app for creating realistic nude photos

AI Clothes Remover
Online AI tool for removing clothes from photos.

Undress AI Tool
Undress images for free

Clothoff.io
AI clothes remover

Video Face Swap
Swap faces in any video effortlessly with our completely free AI face swap tool!

Hot Article

Hot Tools

SublimeText3 English version
Recommended: Win version, supports code prompts!
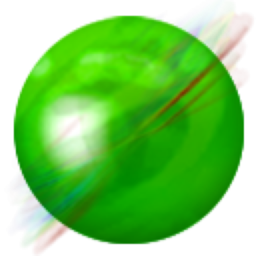
ZendStudio 13.5.1 Mac
Powerful PHP integrated development environment

MantisBT
Mantis is an easy-to-deploy web-based defect tracking tool designed to aid in product defect tracking. It requires PHP, MySQL and a web server. Check out our demo and hosting services.
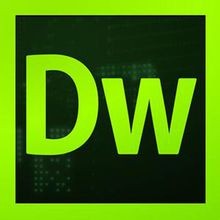
Dreamweaver CS6
Visual web development tools

mPDF
mPDF is a PHP library that can generate PDF files from UTF-8 encoded HTML. The original author, Ian Back, wrote mPDF to output PDF files "on the fly" from his website and handle different languages. It is slower than original scripts like HTML2FPDF and produces larger files when using Unicode fonts, but supports CSS styles etc. and has a lot of enhancements. Supports almost all languages, including RTL (Arabic and Hebrew) and CJK (Chinese, Japanese and Korean). Supports nested block-level elements (such as P, DIV),
