


Beat OpenAI again! Google releases 2 billion parameter universal model to automatically recognize and translate more than 100 languages
Last week, OpenAI released the ChatGPT API and Whisper API, which just triggered a carnival among developers.
#On March 6, Google launched a benchmark model-USM. Not only can it support more than 100 languages, but the number of parameters has also reached 2 billion.
# Of course, the model is still not open to the public, "This is very Google"!
## Simply put, the USM model covers 12 million hours of speech and 28 billion sentences. It is pre-trained on an unlabeled dataset of 300 different languages and fine-tuned on a smaller labeled training set.
Google researchers said that although the annotation training set used for fine-tuning is only 1/7 of Whisper, USM has equivalent or even better results. performance, and also the ability to efficiently adapt to new languages and data.
##Paper address: https://arxiv.org/abs/2303.01037
The results show that USM not only achieves SOTA in multilingual automatic speech recognition and speech-text translation task evaluation, but can also be actually used in YouTube subtitle generation.
#Currently, the languages that support automatic detection and translation include mainstream English, Chinese, and small languages such as Assamese.
#The most important thing is that it can also be used for real-time translation of future AR glasses demonstrated by Google at last year’s IO conference.
##Jeff Dean personally announced: Let AI support 1,000 languages
In November last year, Google first announced a new project to "develop an artificial intelligence language model that supports the 1,000 most commonly used languages in the world."
#The release of the latest model is described by Google as a "critical step" towards its goal.
# When it comes to building language models, it can be said that there are many heroes competing.
According to rumors, Google plans to showcase more than 20 products powered by artificial intelligence at this year’s annual I/O conference.
Currently, automatic speech recognition faces many challenges:
- Traditional Supervised learning methods lack scalability
This requires algorithms that can use large amounts of data from different sources, enable model updates without the need for complete retraining, and be able to generalize to new languages and use cases . According to the paper, USM training uses three databases: unpaired audio data set, unpaired Text data set, paired ASR corpus. Includes YT-NTL-U (over 12 million hours of YouTube untagged audio data) and Pub-U (over 429,000 hours of speech content in 51 languages) Web-NTL (28 billion sentences in over 1140 different languages) YT-SUP and Pub-S corpora (over 10,000 hours of audio content and matching text)Fine-tuned self-supervised learning
USM uses a standard encoder-decoder structure, where the decoder can be CTC, RNN -T or LAS.
#For the encoder, USM uses Conformor, or convolution enhanced Transformer.
#The training process is divided into three stages.
#In the initial stage, unsupervised pre-training is performed using BEST-RQ (BERT-based random projection quantizer for speech pre-training). The goal is to optimize RQ.
#In the next stage, the speech representation learning model is further trained.
Use MOST (Multi-Object Supervised Pre-training) to integrate information from other text data.
The model introduces an additional encoder module that takes text as input and introduces additional layers to combine the speech encoder and text encoder output, and jointly train the model on unlabeled speech, labeled speech, and text data.
The last step is to fine-tune the ASR (automatic speech recognition) and AST (automatic speech translation) tasks. The pre-trained USM model only requires a small amount of Supervisory data can achieve good performance.
##USM overall training process
How does USM perform? Google tested it on YouTube subtitles, promotion of downstream ASR tasks, and automatic speech translation.
Performance on YouTube multi-language subtitles
Supervised YouTube data includes 73 languages, with an average of just under 3,000 hours of data per language. Despite limited supervision data, the model achieved an average word error rate (WER) of less than 30% across 73 languages, which is lower than state-of-the-art models within the United States.
In addition, Google compared it with the Whisper model (big-v2) trained with more than 400,000 hours of annotated data.
Among the 18 languages that Whisper can decode, its decoding error rate is less than 40%, while the average USM error rate is only 32.7%.
##Promotion of downstream ASR tasks
On publicly available datasets, USM shows lower performance on CORAAL (African American Dialect English), SpeechStew (English - United States), and FLEURS (102 languages) compared to Whisper WER, regardless of whether there is in-domain training data.
#The difference between the two models in FLEURS is particularly obvious.
##Performance on the AST task
Fine-tuning USM on the CoVoST dataset.
Divide the languages in the data set into three categories: high, medium, and low according to resource availability, and calculate the BLEU score on each category (the higher the better) , USM performs better than Whisper in every category.
Research has found that BEST-RQ pre-training is an effective way to extend speech representation learning to large data sets.
When combined with text injection in MOST, it improves the quality of downstream speech tasks, achieving state-of-the-art results on the FLEURS and CoVoST 2 benchmarks performance.
By training lightweight residual adapter modules, MOST represents the ability to quickly adapt to new domains. These remaining adapter modules only increase parameters by 2%.
Google said that currently, USM supports more than 100 languages and will expand to more than 100 languages in the future. More than 1000 languages. With this technology, it may be safe for everyone to travel around the world.
#Even, real-time translation of Google AR glasses products will attract many fans in the future.
#However, the application of this technology still has a long way to go.
#After all, in its speech at the IO conference facing the world, Google also wrote the Arabic text backwards, attracting many netizens to watch.
The above is the detailed content of Beat OpenAI again! Google releases 2 billion parameter universal model to automatically recognize and translate more than 100 languages. For more information, please follow other related articles on the PHP Chinese website!
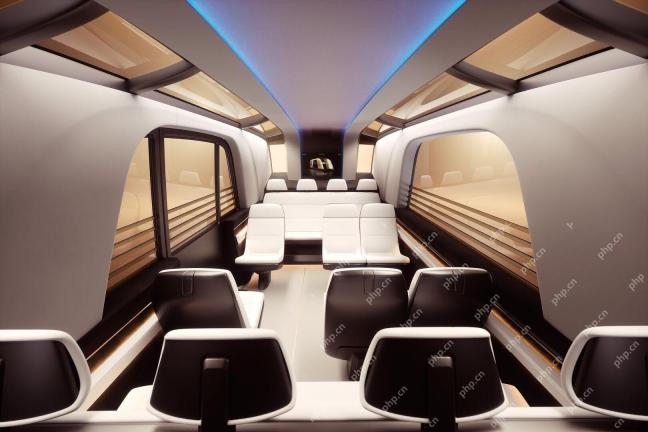
Since 2008, I've championed the shared-ride van—initially dubbed the "robotjitney," later the "vansit"—as the future of urban transportation. I foresee these vehicles as the 21st century's next-generation transit solution, surpas
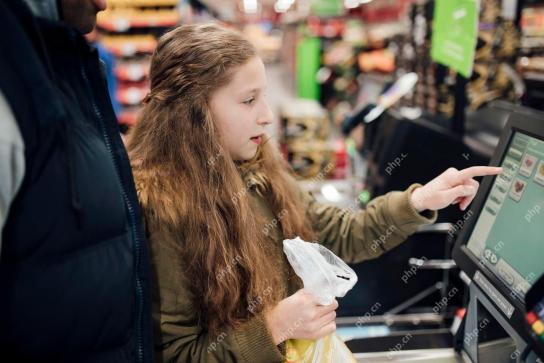
Revolutionizing the Checkout Experience Sam's Club's innovative "Just Go" system builds on its existing AI-powered "Scan & Go" technology, allowing members to scan purchases via the Sam's Club app during their shopping trip.
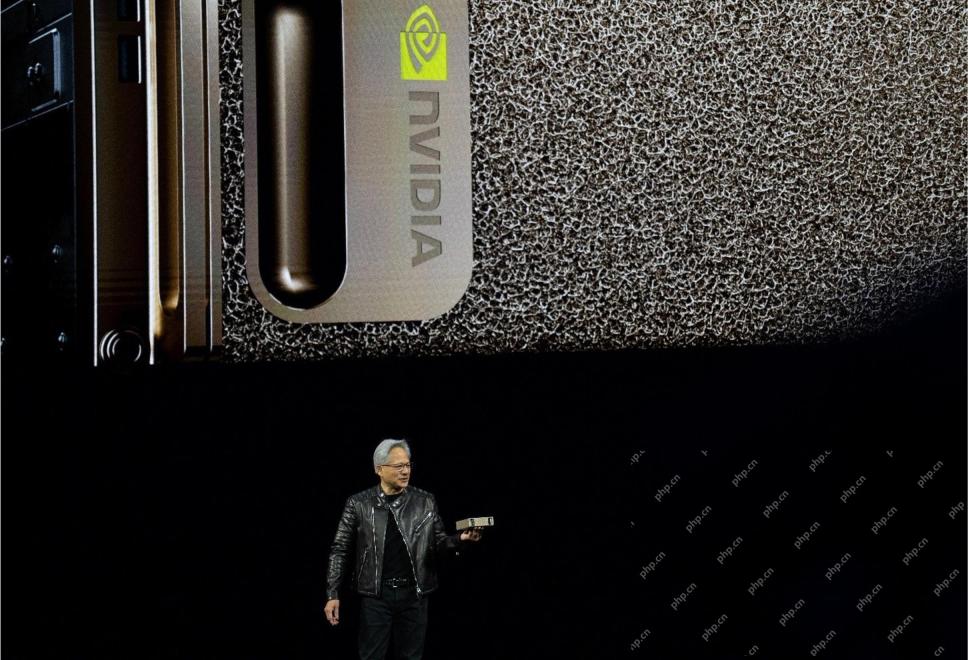
Nvidia's Enhanced Predictability and New Product Lineup at GTC 2025 Nvidia, a key player in AI infrastructure, is focusing on increased predictability for its clients. This involves consistent product delivery, meeting performance expectations, and
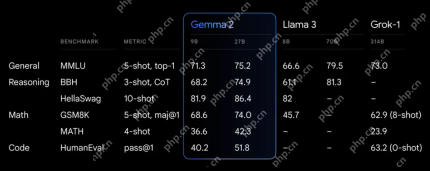
Google's Gemma 2: A Powerful, Efficient Language Model Google's Gemma family of language models, celebrated for efficiency and performance, has expanded with the arrival of Gemma 2. This latest release comprises two models: a 27-billion parameter ver
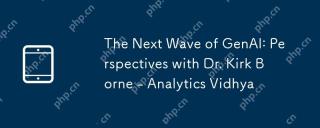
This Leading with Data episode features Dr. Kirk Borne, a leading data scientist, astrophysicist, and TEDx speaker. A renowned expert in big data, AI, and machine learning, Dr. Borne offers invaluable insights into the current state and future traje
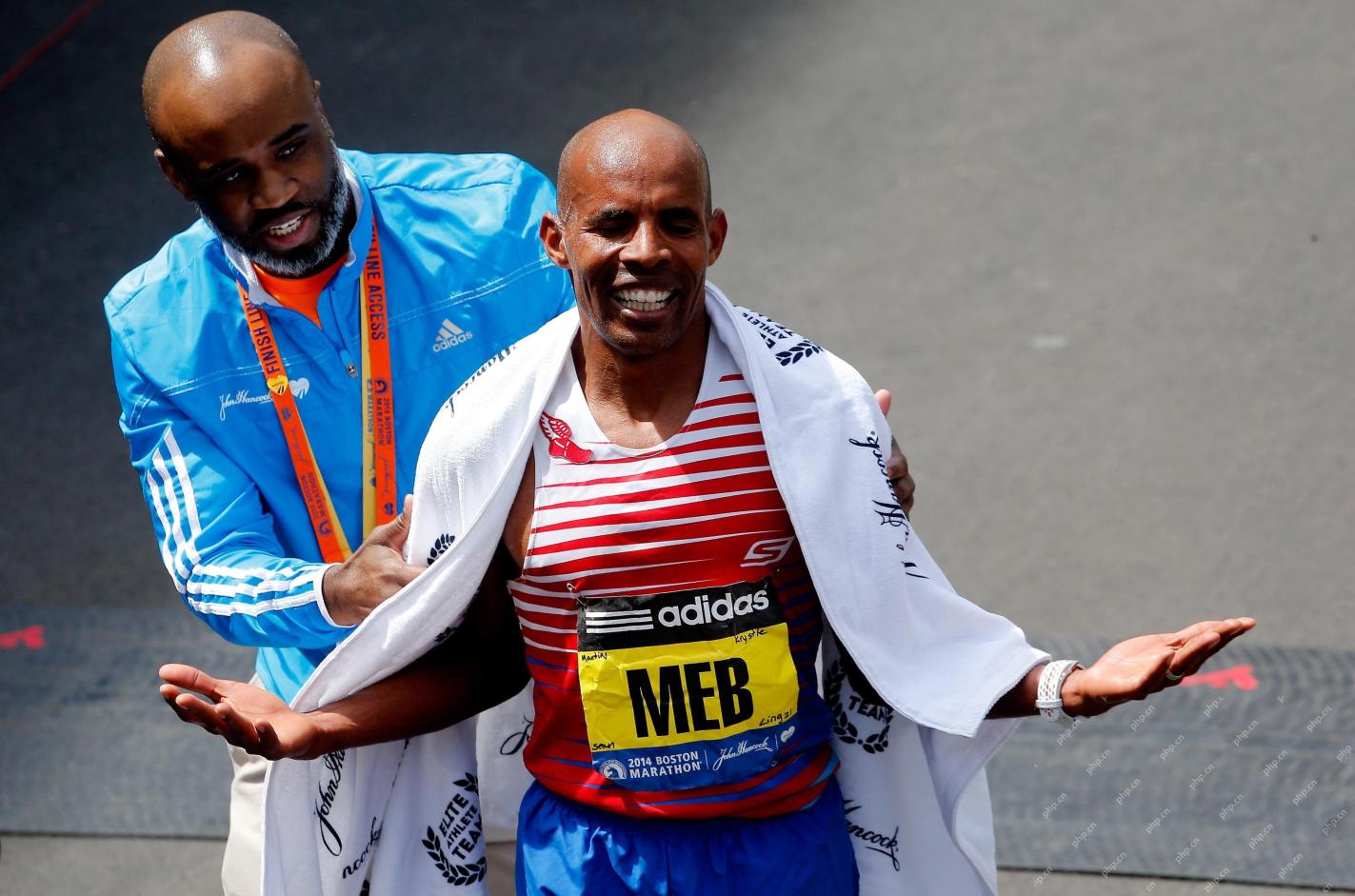
There were some very insightful perspectives in this speech—background information about engineering that showed us why artificial intelligence is so good at supporting people’s physical exercise. I will outline a core idea from each contributor’s perspective to demonstrate three design aspects that are an important part of our exploration of the application of artificial intelligence in sports. Edge devices and raw personal data This idea about artificial intelligence actually contains two components—one related to where we place large language models and the other is related to the differences between our human language and the language that our vital signs “express” when measured in real time. Alexander Amini knows a lot about running and tennis, but he still
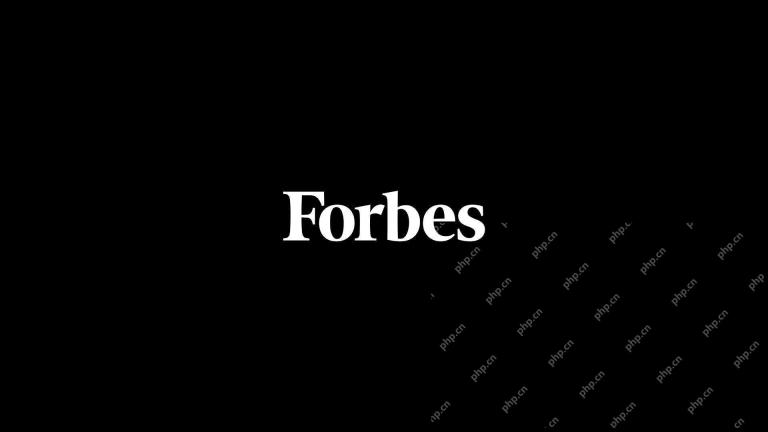
Caterpillar's Chief Information Officer and Senior Vice President of IT, Jamie Engstrom, leads a global team of over 2,200 IT professionals across 28 countries. With 26 years at Caterpillar, including four and a half years in her current role, Engst
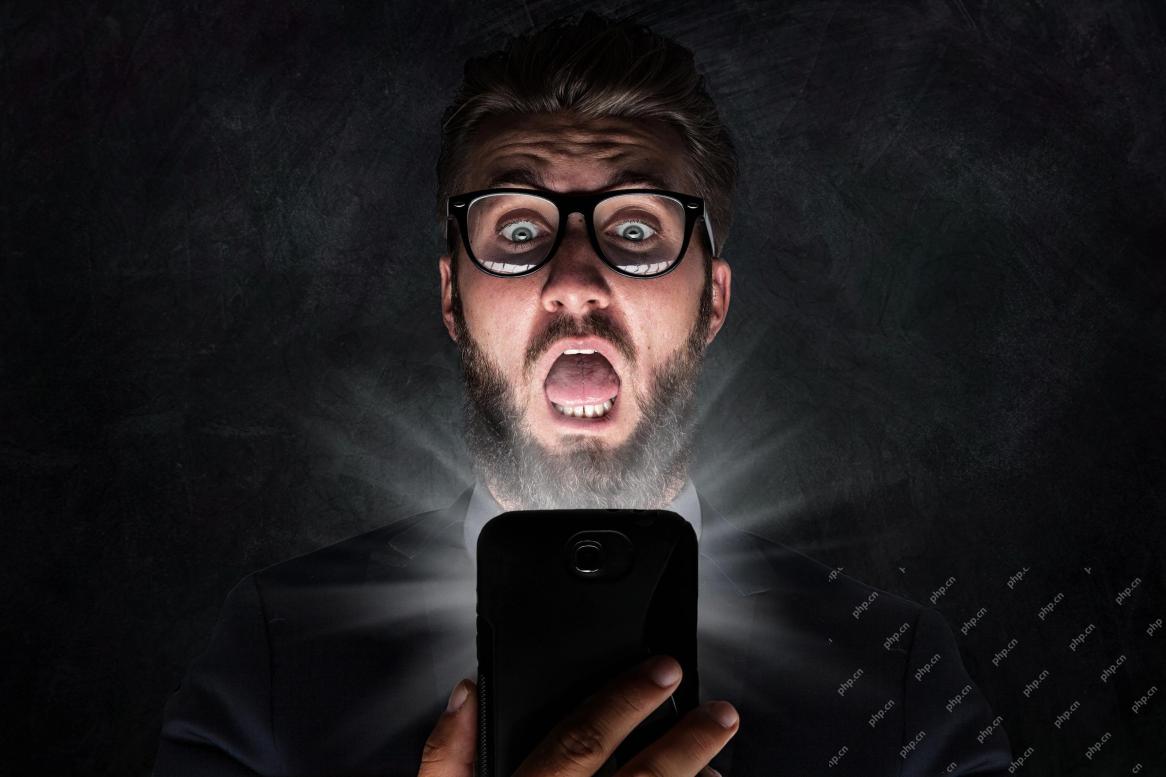
Google Photos' New Ultra HDR Tool: A Quick Guide Enhance your photos with Google Photos' new Ultra HDR tool, transforming standard images into vibrant, high-dynamic-range masterpieces. Ideal for social media, this tool boosts the impact of any photo,


Hot AI Tools

Undresser.AI Undress
AI-powered app for creating realistic nude photos

AI Clothes Remover
Online AI tool for removing clothes from photos.

Undress AI Tool
Undress images for free

Clothoff.io
AI clothes remover

Video Face Swap
Swap faces in any video effortlessly with our completely free AI face swap tool!

Hot Article

Hot Tools

Atom editor mac version download
The most popular open source editor

SublimeText3 Linux new version
SublimeText3 Linux latest version

mPDF
mPDF is a PHP library that can generate PDF files from UTF-8 encoded HTML. The original author, Ian Back, wrote mPDF to output PDF files "on the fly" from his website and handle different languages. It is slower than original scripts like HTML2FPDF and produces larger files when using Unicode fonts, but supports CSS styles etc. and has a lot of enhancements. Supports almost all languages, including RTL (Arabic and Hebrew) and CJK (Chinese, Japanese and Korean). Supports nested block-level elements (such as P, DIV),

Zend Studio 13.0.1
Powerful PHP integrated development environment

SecLists
SecLists is the ultimate security tester's companion. It is a collection of various types of lists that are frequently used during security assessments, all in one place. SecLists helps make security testing more efficient and productive by conveniently providing all the lists a security tester might need. List types include usernames, passwords, URLs, fuzzing payloads, sensitive data patterns, web shells, and more. The tester can simply pull this repository onto a new test machine and he will have access to every type of list he needs.