


Text-to-image generation is one of the hottest AIGC directions in 2022 and was selected as one of the top ten scientific breakthroughs in 2022 by "Science". Recently, Google's new text-to-image generation paper "Muse: Text-To-Image Generation via Masked Generative Transformers" has attracted great attention.
- ##Paper address: https://arxiv.org/pdf/2301.00704v1.pdf
- Project address: https://muse-model.github.io/
The study proposes a A new model for text-to-image synthesis using a masked image modeling approach, where the image decoder architecture is conditioned on embeddings from a pre-trained and frozen T5-XXL Large Language Model (LLM) encoder.
Similar to Google’s previous Imagen model, this study found that tuning based on a pre-trained LLM is critical for realistic, high-quality image generation. The Muse model is built on the Transformer (Vaswani et al., 2017) architecture.
Compared with Imagen (Saharia et al., 2022) or Dall-E2 (Ramesh et al., 2022) based on the cascaded pixel-space diffusion model Compared with Muse, the efficiency is significantly improved due to the use of discrete tokens. Compared with the SOTA autoregressive model Parti (Yu et al., 2022), Muse is more efficient due to its use of parallel decoding.
Based on experimental results on TPU-v4, researchers estimate that Muse is more than 10 times faster than Imagen-3B or Parti-3B models in inference speed, and faster than Stable Diffusion v1.4 (Rombach et al., 2022) 2 times faster. Researchers believe that Muse is faster than Stable Diffusion because the diffusion model is used in Stable Diffusion v1.4, which obviously requires more iterations during inference.
On the other hand, the improvement in Muse efficiency has not resulted in a decrease in the quality of the generated images or a decrease in the model's semantic understanding of the input text prompt. This study evaluated Muse's generation results against multiple criteria, including CLIP score (Radford et al., 2021) and FID (Heusel et al., 2017). The Muse-3B model achieved a CLIP score of 0.32 and a FID score of 7.88 on the COCO (Lin et al., 2014) zero-shot validation benchmark.
Let’s take a look at the Muse generation effect:
Text-Image generation: The Muse model quickly generates high-quality images from text prompts (in On TPUv4, it takes 1.3 seconds to generate an image with 512x512 resolution, and 0.5 seconds to generate an image with 256x256 resolution). For example, generate "a bear riding a bicycle and a bird perching on the handlebars":
The Muse model generates images under text prompt conditions The token performs iterative resampling, providing users with zero-sample, mask-free editing.
Muse also offers mask-based editing, such as "There is a gazebo on the lake against the backdrop of beautiful autumn leaves."
Muse is built on a number of components, Figure 3 provides an overview of the model architecture.
Specifically, the components included are:
Pre-trained text encoder: This study found that utilizing pre-trained large language models (LLM) can improve the quality of image generation. They hypothesized that the Muse model learned to map the rich visual and semantic concepts in the LLM embeddings to the generated images. Given an input text subtitle, the study passes it through a frozen T5-XXL encoder, resulting in a 4096-dimensional sequence of language embedding vectors. These embedding vectors are linearly projected to the Transformer model.
Semantic Tokenization using VQGAN: The core component of this model is the use of semantic tokens obtained from the VQGAN model. Among them, VQGAN consists of an encoder and a decoder, and a quantization layer maps the input image to a token sequence in a learning codebook. This study all uses convolutional layers to build encoders and decoders to support encoding images of different resolutions.
Base model: The base model is a mask transformer where the inputs are embeddings and image tokens projected to T5. The study keeps all text embeddings (unmasked), randomly masks image tokens of different proportions, and replaces them with a special [mask] token.
Super-resolution model: The study found it beneficial to use a cascade of models: first a base model that generates a 16 × 16 latent map (corresponding to a 256 × 256 image), and then The basic latent map is upsampled to the super-resolution model, which is a 64 × 64 latent map (corresponding to a 512 × 512 image).
Decoder fine-tuning: To further improve the model’s ability to generate fine details, this study increases the capacity of the VQGAN decoder by adding more residual layers and channels while maintaining the encoder Capacity remains unchanged. The new decoder layer is then fine-tuned while freezing the VQGAN encoder weights, codebook and transformer (i.e. base model and super-resolution model).
In addition to the above components, Muse also includes variable mask ratio components, iterative parallel decoding components during inference, etc.
Experiments and results
As shown in the table below, Muse shortens the inference time compared with other models.
The following table shows the FID and CLIP scores measured by different models on zero-shot COCO:
As shown in the table below, Muse (632M (base) 268M (super-res) parameter model) obtained a SOTA FID score of 6.06 when trained and evaluated on the CC3M dataset.
The following figure is an example of results generated by Muse, Imagen, and DALL-E 2 under the same prompt.
Interested readers can read the original text of the paper to learn more about the research details.
The above is the detailed content of The inference speed is 2 times faster than Stable Diffusion. Generating and repairing images can be done with one Google model, realizing new SOTA.. For more information, please follow other related articles on the PHP Chinese website!
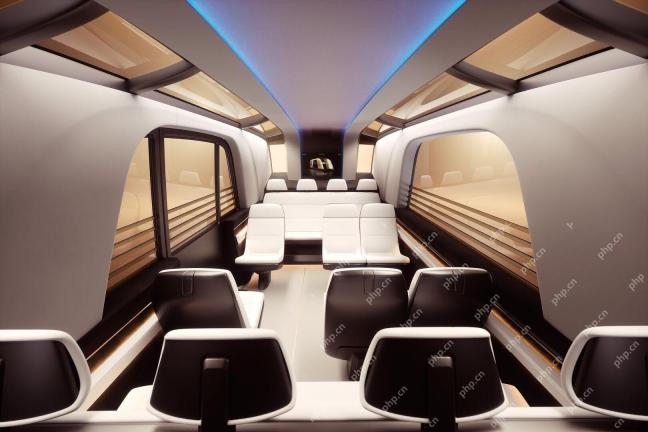
Since 2008, I've championed the shared-ride van—initially dubbed the "robotjitney," later the "vansit"—as the future of urban transportation. I foresee these vehicles as the 21st century's next-generation transit solution, surpas
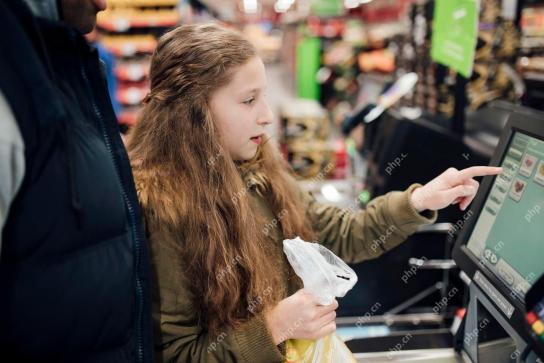
Revolutionizing the Checkout Experience Sam's Club's innovative "Just Go" system builds on its existing AI-powered "Scan & Go" technology, allowing members to scan purchases via the Sam's Club app during their shopping trip.
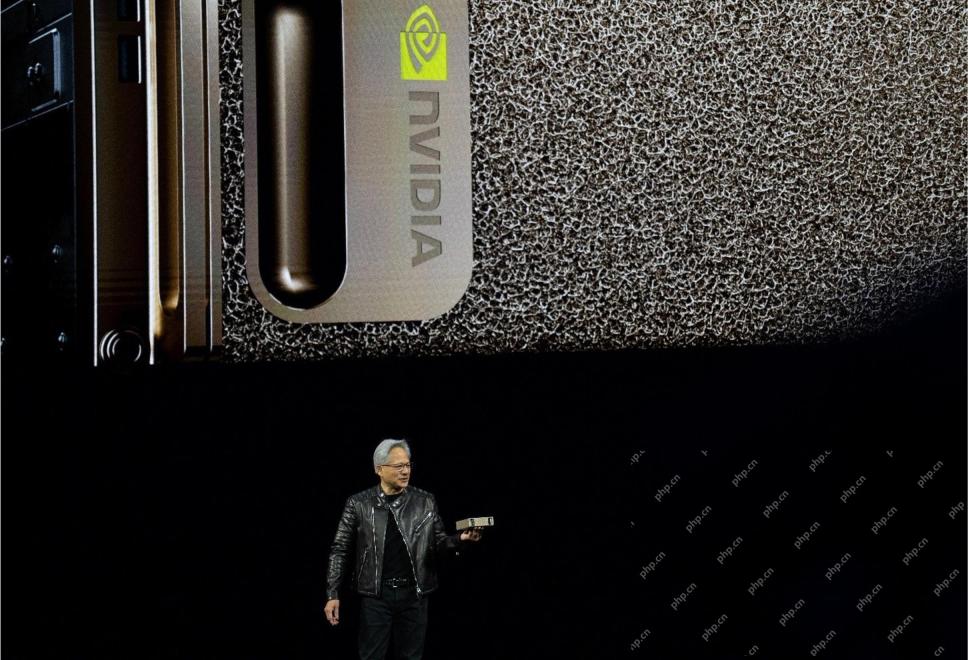
Nvidia's Enhanced Predictability and New Product Lineup at GTC 2025 Nvidia, a key player in AI infrastructure, is focusing on increased predictability for its clients. This involves consistent product delivery, meeting performance expectations, and
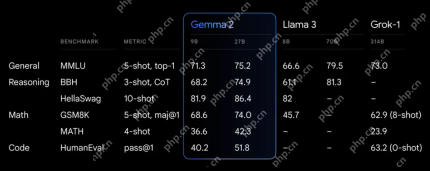
Google's Gemma 2: A Powerful, Efficient Language Model Google's Gemma family of language models, celebrated for efficiency and performance, has expanded with the arrival of Gemma 2. This latest release comprises two models: a 27-billion parameter ver
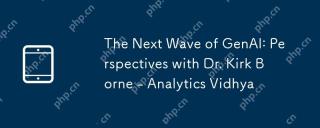
This Leading with Data episode features Dr. Kirk Borne, a leading data scientist, astrophysicist, and TEDx speaker. A renowned expert in big data, AI, and machine learning, Dr. Borne offers invaluable insights into the current state and future traje
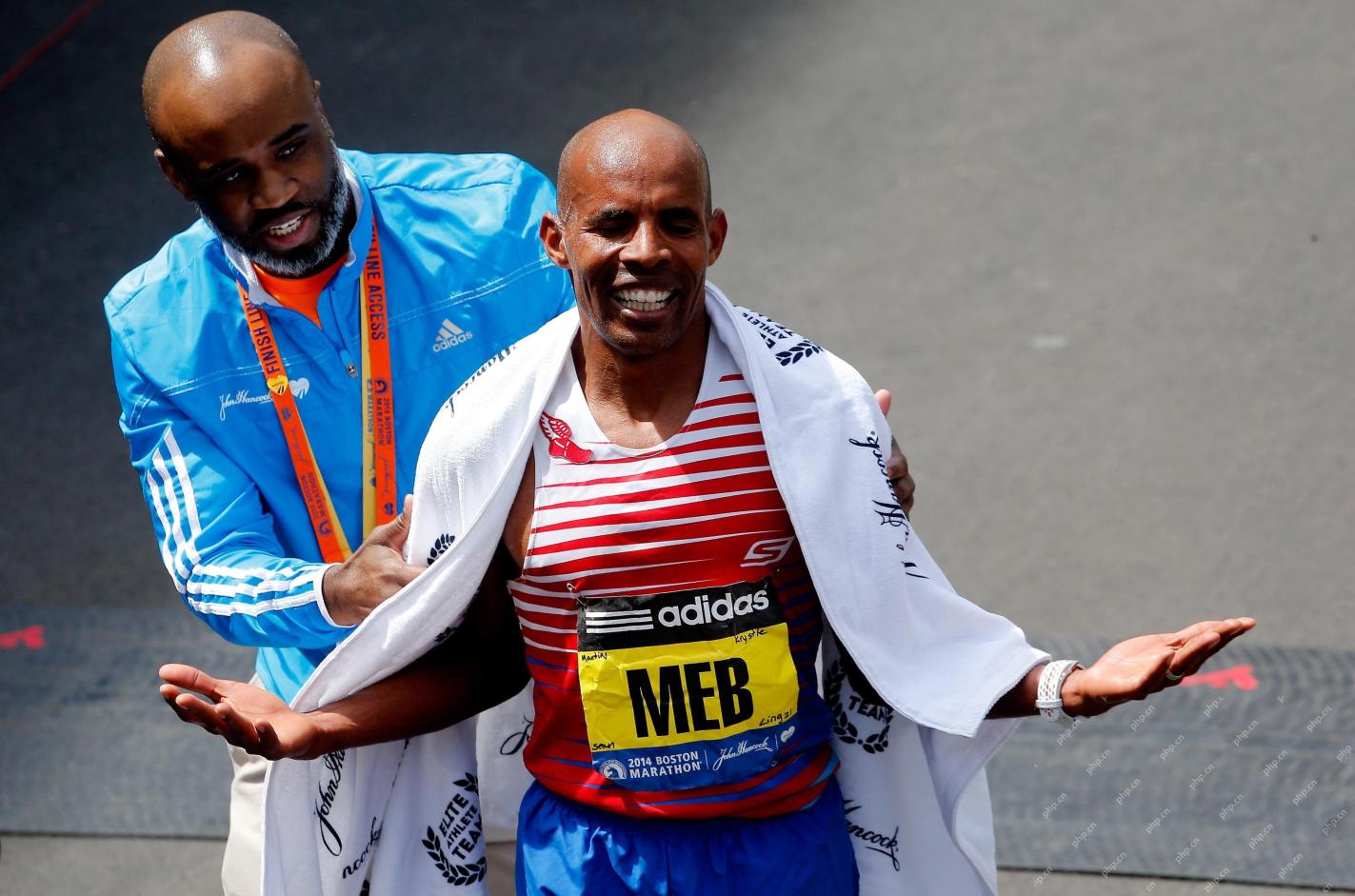
There were some very insightful perspectives in this speech—background information about engineering that showed us why artificial intelligence is so good at supporting people’s physical exercise. I will outline a core idea from each contributor’s perspective to demonstrate three design aspects that are an important part of our exploration of the application of artificial intelligence in sports. Edge devices and raw personal data This idea about artificial intelligence actually contains two components—one related to where we place large language models and the other is related to the differences between our human language and the language that our vital signs “express” when measured in real time. Alexander Amini knows a lot about running and tennis, but he still
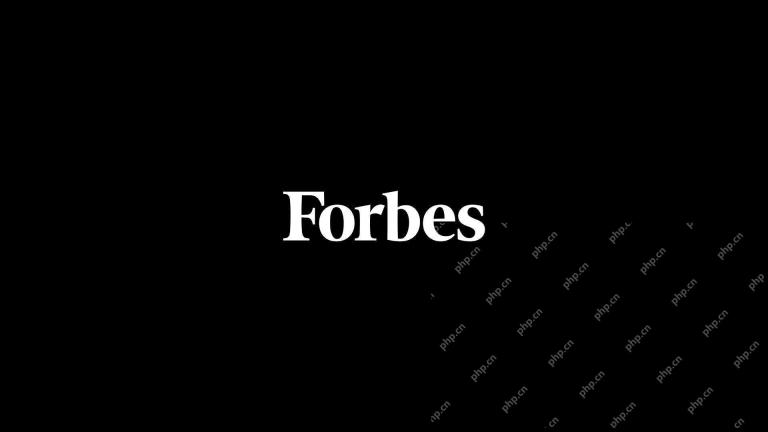
Caterpillar's Chief Information Officer and Senior Vice President of IT, Jamie Engstrom, leads a global team of over 2,200 IT professionals across 28 countries. With 26 years at Caterpillar, including four and a half years in her current role, Engst
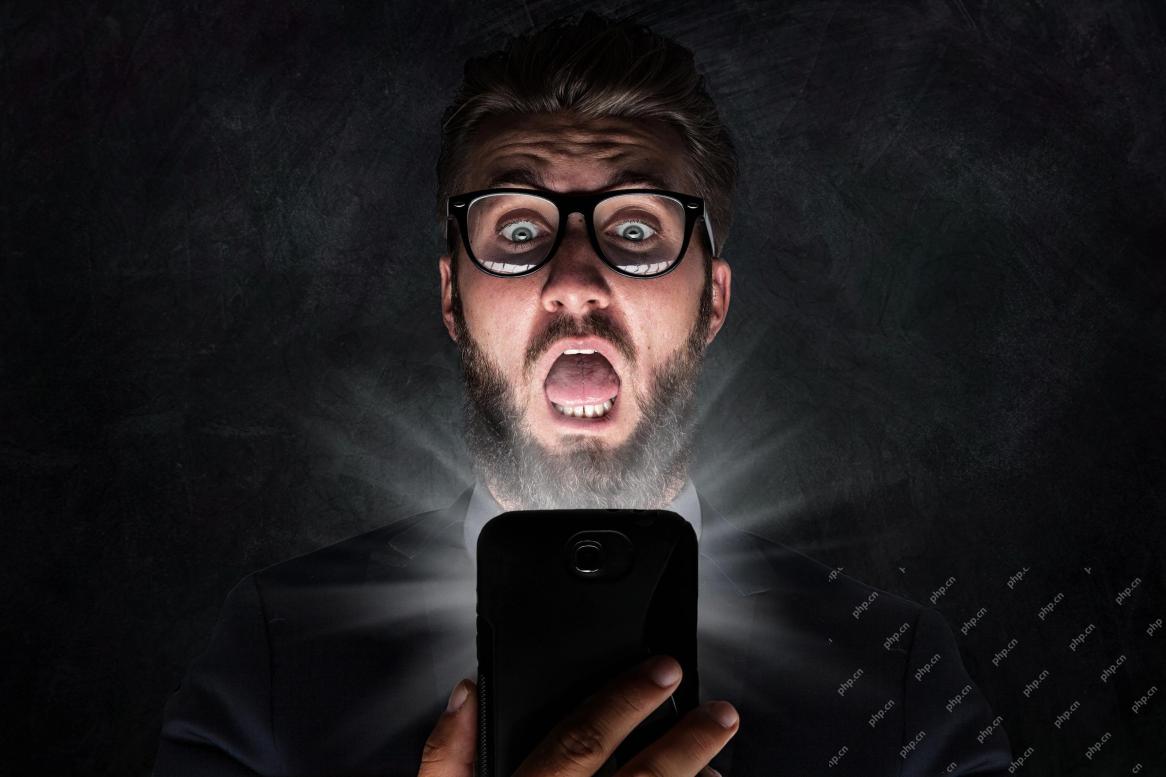
Google Photos' New Ultra HDR Tool: A Quick Guide Enhance your photos with Google Photos' new Ultra HDR tool, transforming standard images into vibrant, high-dynamic-range masterpieces. Ideal for social media, this tool boosts the impact of any photo,


Hot AI Tools

Undresser.AI Undress
AI-powered app for creating realistic nude photos

AI Clothes Remover
Online AI tool for removing clothes from photos.

Undress AI Tool
Undress images for free

Clothoff.io
AI clothes remover

Video Face Swap
Swap faces in any video effortlessly with our completely free AI face swap tool!

Hot Article

Hot Tools

PhpStorm Mac version
The latest (2018.2.1) professional PHP integrated development tool
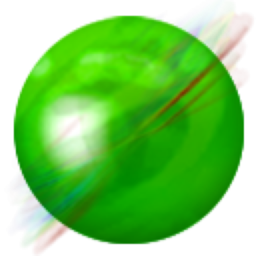
ZendStudio 13.5.1 Mac
Powerful PHP integrated development environment
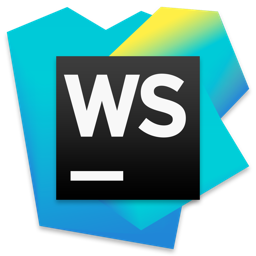
WebStorm Mac version
Useful JavaScript development tools

Safe Exam Browser
Safe Exam Browser is a secure browser environment for taking online exams securely. This software turns any computer into a secure workstation. It controls access to any utility and prevents students from using unauthorized resources.

Notepad++7.3.1
Easy-to-use and free code editor