


1200 times faster! MIT develops a new generation of drug research and development AI to defeat the old model
As we all know, the entire universe is filled with countless molecules.
How many of these molecules have potentially drug-like properties that could be used to develop life-saving drugs? Is it a million? Or billions? Or trillions?
The answer is: 10 to the 60th power.
Such a huge number has greatly delayed the development of new drugs. For rapidly spreading diseases such as COVID-19, there is currently no specific drug. This is also because the types and quantities of molecules are too large, far beyond what is currently available. The range of calculations that drug design models can make.
A research team at MIT doesn’t believe this evil. It doesn’t work out, right? Then it’s okay to accelerate the previous model, right?
This acceleration is 1200 times.
They studied a geometric deep learning model called "EquiBind", which is 1,200 times faster than the previous fastest computational molecular docking model "QuickVina2-W", and successfully combined drug-like molecules Binding to proteins reduces the chance and cost of drug trial failure.
The research paper will be published at ICML 2022.
First introduction to "EquiBind"
"EquiBind" is developed based on its predecessor "EquiDock". "EquiDock" uses the late MIT The technology developed by Octavian-Eugen Ganea, an AI researcher at the college, combines two proteins. Ganea is also a co-author of the "EquiBind" paper.
Before drug development can begin, researchers must find promising drug-like molecules that can correctly bind, or "docked," to specific protein targets during the drug discovery process.
After successfully docking with the protein, combining the drug (ligand) can prevent the protein from functioning. If this happens to one of the bacteria's essential proteins, it can kill the bacteria and thus protect the body.
However, the process of drug discovery can be expensive, both from an economic and computational perspective. The research and development process often costs billions of dollars and will take more than 10 years before final approval by the FDA. Ten years of development and testing.
More importantly, 90% of drugs fail after human trials because they have no effect or have too many side effects.
So one of the ways pharmaceutical companies recoup these costs is to raise the price of the drug they ultimately successfully develop.
Into “EquiBind”
Currently, the computational process for finding promising drug candidate molecules is as follows: most state-of-the-art computational models rely on a large number of Candidate samples, coupled with methods such as scoring, ranking and fine-tuning, to obtain the best "match" between ligand and protein.
Hannes Stärk, a first-year graduate student in MIT's Department of Electrical Engineering and Computer Science and the lead author of the paper, likened the typical "ligand-protein" binding method to "trying to put the key into something." Many keyholes in the lock."
# Typical models spend time scoring each "fit" before selecting the best model. In contrast, “EquiBind” does not need to know the target pocket of the protein in advance, and can directly predict the precise key position in just one step, which is called “blind docking”.
Unlike most models that require multiple attempts to find the favorable position of a ligand in a protein, “EquiBind” already has built-in geometric reasoning capabilities that help the model learn the underlying physical properties of the molecule and successfully to summarize. This allows for successful generalization to make better predictions when encountering new or unrecognizable data.
The release of these findings quickly attracted the attention of industry professionals, including Relay Therapeutics chief data officer Pat Walters.
Walters suggested that the team could try their model on an existing drug and protein used in lung cancer, leukemia and gastrointestinal tumors. Although most traditional docking methods fail to successfully bind ligands on these proteins, EquiBind succeeds.
Walters said: "EquiBind provides a unique solution to the docking problem, which combines pose prediction and binding site identification."
"And this method utilizes data from "The information from thousands of published crystal structures has the potential to impact the field in new ways." Stärk said: "We were surprised when all the other approaches were completely wrong or only one was right, because EquiBind was able to put it into the right pocket, and we are very excited to see this result!"
Help "EquiBind"
Although "EquiBind" has received a large number of comments from industry professionals That feedback has helped the team consider practical uses for computational models, but Stärk is still hoping to find different perspectives at the upcoming ICML in July.
Stärk said: "The feedback I am most looking forward to is suggestions on how to further improve the model."
"I would like to discuss with these researchers, tell them what I think the next steps can be, and encourage them to move forward and use the model in their own papers and methods. We have already been contacted by many researchers, Ask us if this model would be useful for their problem."
In addition, this article also commemorates Octavian-Eugen Gane, who made crucial contributions to geometric machine learning research and generously He mentored many students and was an outstanding scholar with a humble soul.
In the first half of this year, he left us forever during a hiking trip.
The above is the detailed content of 1200 times faster! MIT develops a new generation of drug research and development AI to defeat the old model. For more information, please follow other related articles on the PHP Chinese website!
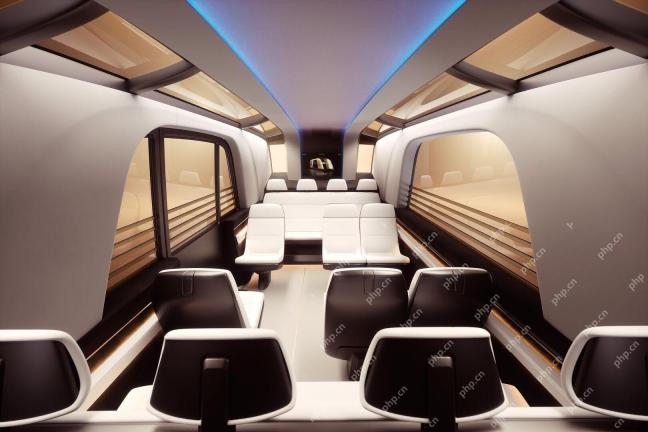
Since 2008, I've championed the shared-ride van—initially dubbed the "robotjitney," later the "vansit"—as the future of urban transportation. I foresee these vehicles as the 21st century's next-generation transit solution, surpas
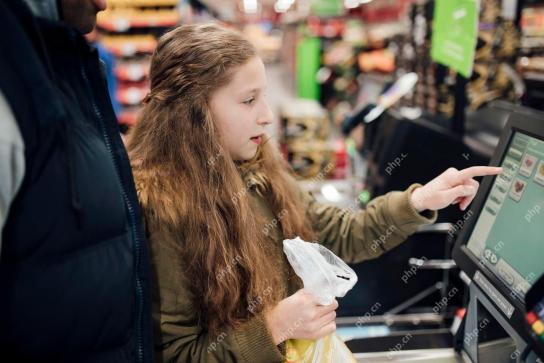
Revolutionizing the Checkout Experience Sam's Club's innovative "Just Go" system builds on its existing AI-powered "Scan & Go" technology, allowing members to scan purchases via the Sam's Club app during their shopping trip.
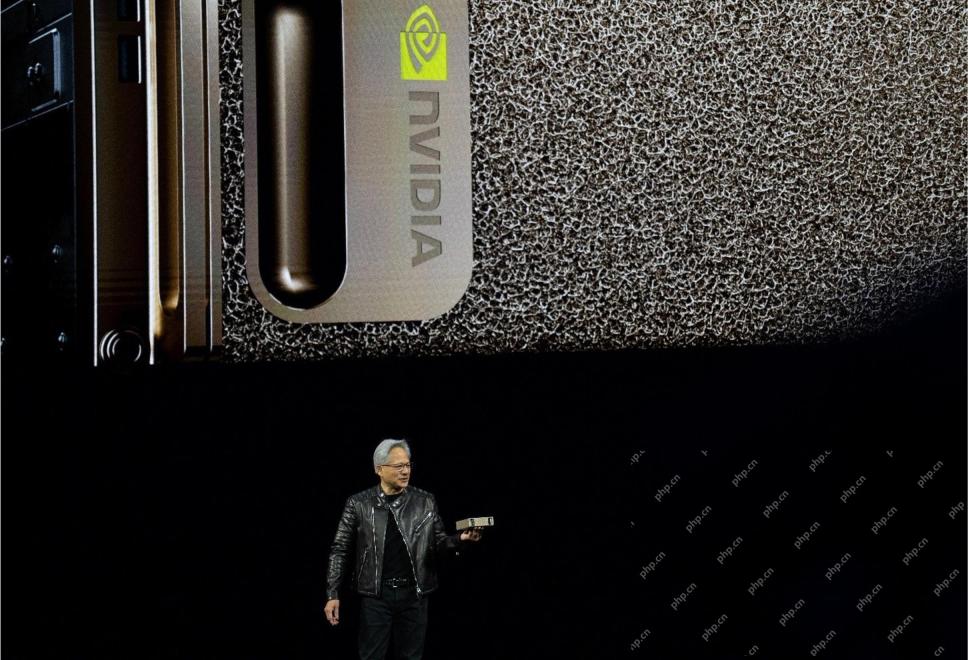
Nvidia's Enhanced Predictability and New Product Lineup at GTC 2025 Nvidia, a key player in AI infrastructure, is focusing on increased predictability for its clients. This involves consistent product delivery, meeting performance expectations, and
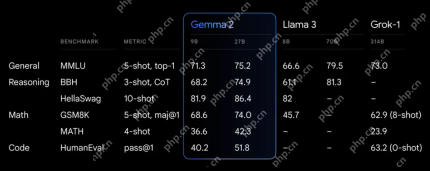
Google's Gemma 2: A Powerful, Efficient Language Model Google's Gemma family of language models, celebrated for efficiency and performance, has expanded with the arrival of Gemma 2. This latest release comprises two models: a 27-billion parameter ver
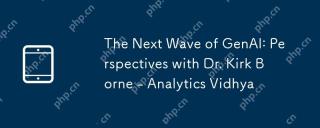
This Leading with Data episode features Dr. Kirk Borne, a leading data scientist, astrophysicist, and TEDx speaker. A renowned expert in big data, AI, and machine learning, Dr. Borne offers invaluable insights into the current state and future traje
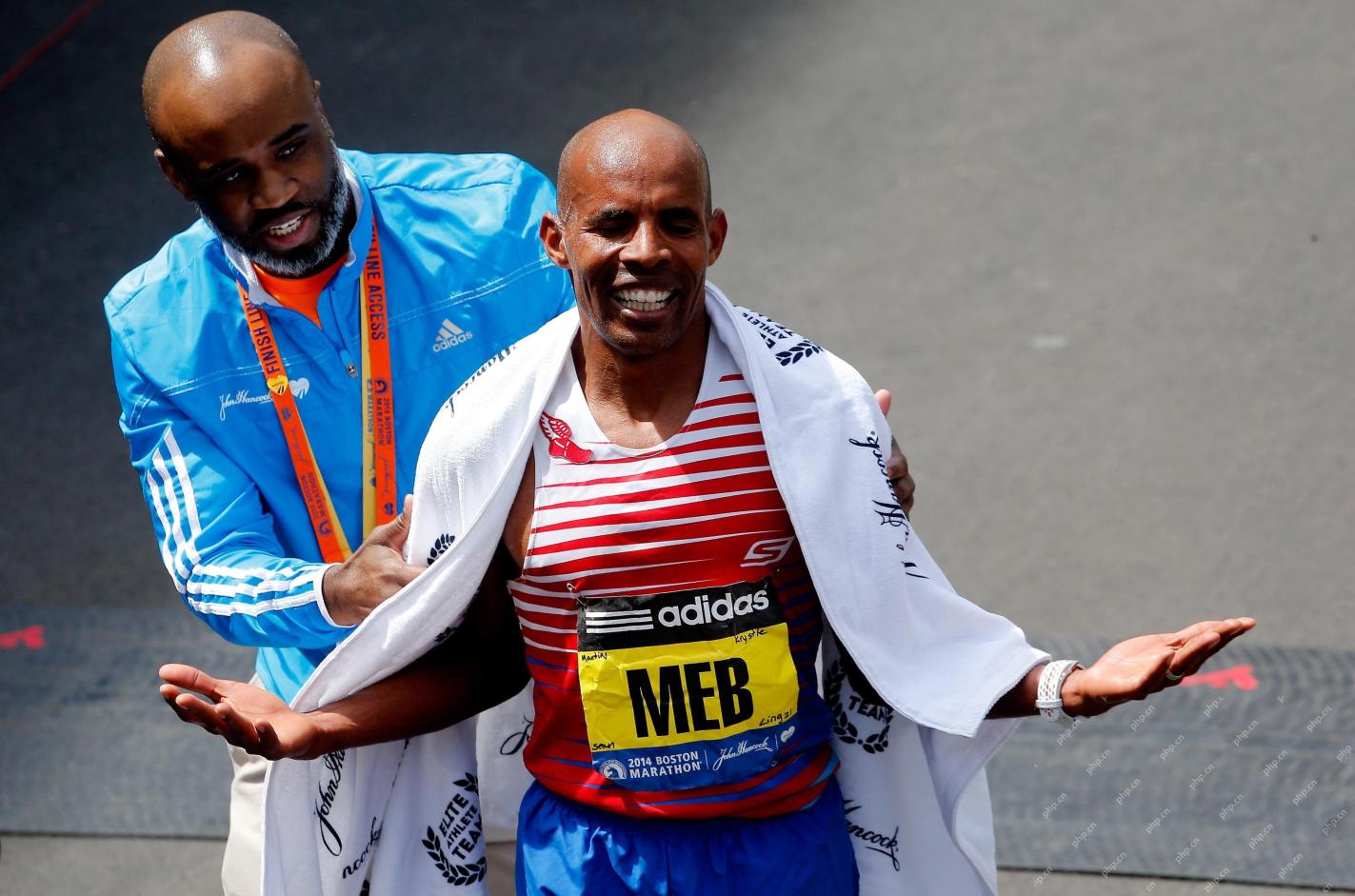
There were some very insightful perspectives in this speech—background information about engineering that showed us why artificial intelligence is so good at supporting people’s physical exercise. I will outline a core idea from each contributor’s perspective to demonstrate three design aspects that are an important part of our exploration of the application of artificial intelligence in sports. Edge devices and raw personal data This idea about artificial intelligence actually contains two components—one related to where we place large language models and the other is related to the differences between our human language and the language that our vital signs “express” when measured in real time. Alexander Amini knows a lot about running and tennis, but he still
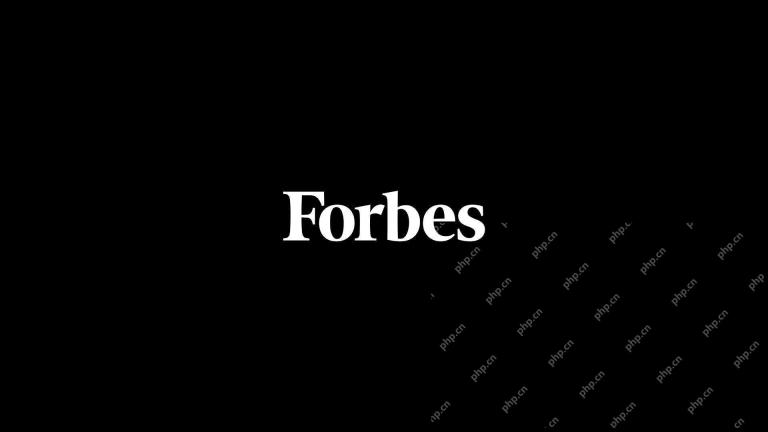
Caterpillar's Chief Information Officer and Senior Vice President of IT, Jamie Engstrom, leads a global team of over 2,200 IT professionals across 28 countries. With 26 years at Caterpillar, including four and a half years in her current role, Engst
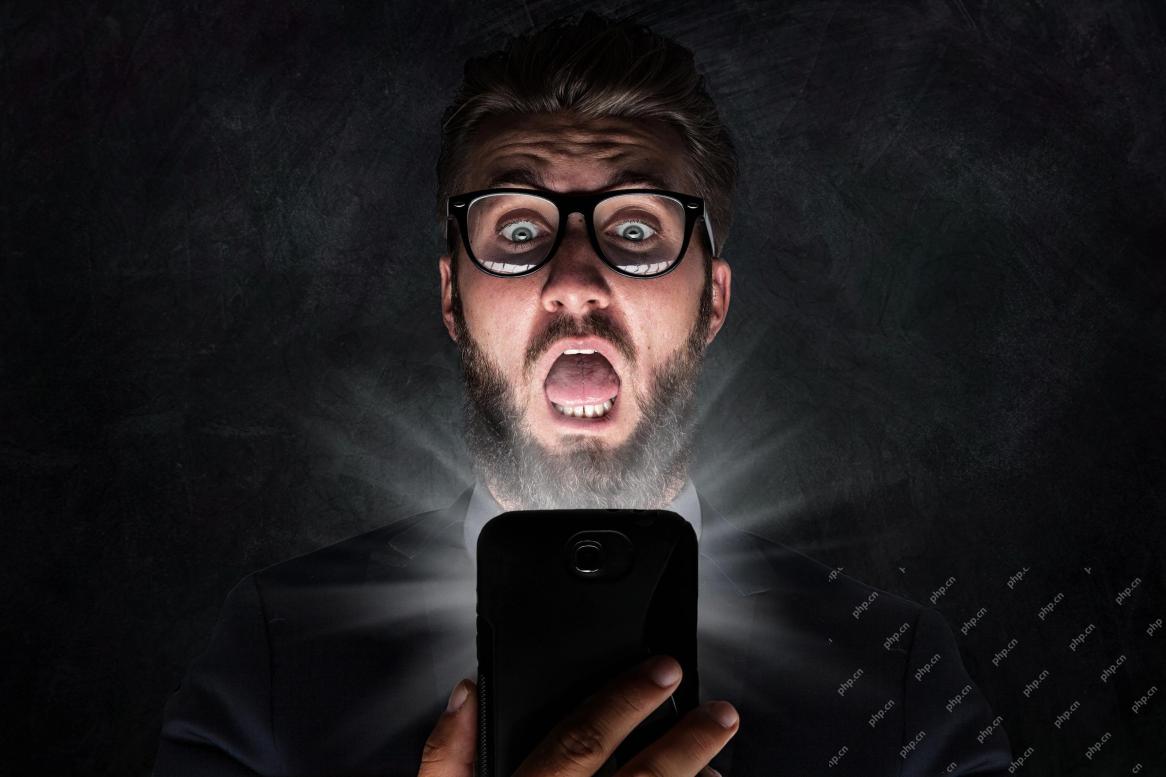
Google Photos' New Ultra HDR Tool: A Quick Guide Enhance your photos with Google Photos' new Ultra HDR tool, transforming standard images into vibrant, high-dynamic-range masterpieces. Ideal for social media, this tool boosts the impact of any photo,


Hot AI Tools

Undresser.AI Undress
AI-powered app for creating realistic nude photos

AI Clothes Remover
Online AI tool for removing clothes from photos.

Undress AI Tool
Undress images for free

Clothoff.io
AI clothes remover

Video Face Swap
Swap faces in any video effortlessly with our completely free AI face swap tool!

Hot Article

Hot Tools

PhpStorm Mac version
The latest (2018.2.1) professional PHP integrated development tool
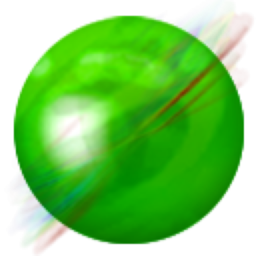
ZendStudio 13.5.1 Mac
Powerful PHP integrated development environment
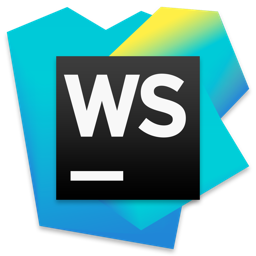
WebStorm Mac version
Useful JavaScript development tools

Safe Exam Browser
Safe Exam Browser is a secure browser environment for taking online exams securely. This software turns any computer into a secure workstation. It controls access to any utility and prevents students from using unauthorized resources.

Notepad++7.3.1
Easy-to-use and free code editor