1. Traditional Sync syntax request example
It’s still the same. Before understanding the implementation of Async syntax, let’s start with a Sync syntax example. Now suppose there is a HTTP request, this program will obtain the corresponding response content through this request, and print it out. The code is as follows:
import socket def request(host: str) -> None: """模拟请求并打印响应体""" url: str = f"http://{host}" sock: socket.SocketType = socket.socket() sock.connect((host, 80)) sock.send(f"GET {url} HTTP/1.0rnHost: {host}rnrn".encode("ascii")) response_bytes: bytes = b"" chunk: bytes = sock.recv(4096) while chunk: response_bytes += chunk chunk = sock.recv(4096) print("n".join([i for i in response_bytes.decode().split("rn")])) if __name__ == "__main__": request("so1n.me")
Run the program, the program can output normally, the upper part prints the corresponding HTTP response Header, and the lower part prints After viewing the HTTP response body, you can see that the server asked us to re-request in the form of https. The output is as follows:
HTTP/1.1 301 Moved Permanently Server: GitHub.com Content-Type: text/html Location: https://so1n.me/ X-GitHub-Request-Id: A744:3871:4136AF:48BD9F:6188DB50 Content-Length: 162 Accept-Ranges: bytes Date: Mon, 08 Nov 2021 08:11:37 GMT Via: 1.1 varnish Age: 104 Connection: close X-Served-By: cache-qpg1272-QPG X-Cache: HIT X-Cache-Hits: 2 X-Timer: S1636359097.026094,VS0,VE0 Vary: Accept-Encoding X-Fastly-Request-ID: 22fa337f777553d33503cee5282598c6a293fb5e <html> <head><title>301 Moved Permanently</title></head> <body> <center><h1 id="Moved-Permanently">301 Moved Permanently</h1></center> <hr><center>nginx</center> </body> </html>
But this is not to say how the HTTP request is implemented. I don’t know much about it. , in this code, the default call of socket is blocked. When the thread calls connect or recv (send does not need to wait, but under high concurrency, you need to wait for drain before sending. The small demo does not need to use the drain method. ), the program will pause until the operation is completed. When many web pages are downloaded at one time, as mentioned in the previous article, most of the waiting time is spent on IO and the CPU is always idle. Although using the thread pool can solve this problem, the overhead is very high. Large, at the same time, the operating system often limits the number of threads that a process, user or machine can use, but coroutines have no such restrictions, occupy less resources, and have no system bottlenecks.
2. Asynchronous requests
Asynchronous allows a separate thread to handle concurrent operations, but as mentioned above, the socket is blocked by default, so the socket needs to be set to non Blocking, socket provides the setblocking method for developers to choose whether to block. After setting non-blocking, the connect and recv methods must also be changed.
Since there is no blocking, the program will return immediately after calling connect. However, the bottom layer of Python is C. This code will throw an exception after calling non-blocking socket.connect in C. We We need to capture it, like this:
import socket sock: socket.SocketType = socket.socket() sock.setblocking(Flase) try: sock.connect(("so1n.me", 80)) except BlockingIOError: pass
After an operation, we start to apply for establishing a connection, but we still don’t know when the connection will be established, because calling send when the connection is not established will report an error, so You can keep polling and calling send until no error is reported and it is considered successful (the real code needs to add a timeout):
while True: try: sock.send(request) break except OSError as e: pass
But this is a waste of performance to let the CPU idle, and you can't do other things during the period, just like us After ordering takeout, we kept calling to ask if the meal was ready. It was a waste of phone calls. If we had just called to tell us when the meal was ready, we would only have incurred one expense, which was very economical (this is also the case under normal circumstances). . This is when the event loop comes into play. In UNIX-like systems, there is a function called select, which can wait for an event to occur before calling the listening function. However, the initial implementation performance was not very good and was replaced by epoll on Linux. However, the interfaces are similar. The reason is that these different event loops are encapsulated in the selectors library in Python. At the same time, the best select-like function can be selected from the system through DefaultSelector. Let’s not talk about the principle of the event loop for now. The most important thing about the event loop is the two parts of its name. One is the event and the other is the cycle. In Python, events can be registered into the event loop through the following method:
def demo(): pass selector.register(fd, EVENT_WRITE, demo)
In this way, this event loop will listen to the corresponding file descriptor fd. When this file descriptor triggers a write event (EVENT_WRITE), the event loop will tell us that we can call the registered function demo. However, if you change the above code to this method to run, you will find that the program seems to end without running, but the program actually runs, but they complete the registration, and then wait for the developer to receive the event. The loop event will perform the next operation, so we only need to write the following code at the end of the code:
while True: for key, mask in selector.select(): key.data()
In this way, the program will keep running, and when the event is captured, it will be notified through the for loop We, where key.data is the callback function we registered, will notify us when an event occurs. We can get the callback function and run it. After understanding it, we can write our first concurrent program, He implemented a simple small logic of I/O multiplexing. The code and comments are as follows:
import socket from selectors import DefaultSelector, EVENT_READ, EVENT_WRITE # 选择事件循环 selector: DefaultSelector = DefaultSelector() # 用于判断是否有事件在运行 running_cnt: int = 0 def request(host: str) -> None: """模拟请求并打印响应体""" # 告诉主函数, 自己的事件还在运行 global running_cnt running_cnt += 1 # 初始化socket url: str = f"http://{host}" sock: socket.SocketType = socket.socket() sock.setblocking(False) try: sock.connect((host, 80)) except BlockingIOError: pass response_bytes: bytes = b"" def read_response() -> None: """接收响应参数, 并判断请求是否结束""" nonlocal response_bytes chunk: bytes = sock.recv(4096) print(f"recv {host} body success") if chunk: response_bytes += chunk else: # 没有数据代表请求结束了, 注销监听 selector.unregister(sock.fileno()) global running_cnt running_cnt -= 1 def connected() -> None: """socket建立连接时的回调""" # 取消监听 selector.unregister(sock.fileno()) print(f"{host} connect success") # 发送请求, 并监听读事件, 以及注册对应的接收响应函数 sock.send(f"GET {url} HTTP/1.0rnHost: {host}rnrn".encode("ascii")) selector.register(sock.fileno(), EVENT_READ, read_response) selector.register(sock.fileno(), EVENT_WRITE, connected) if __name__ == "__main__": # 同时多个请求 request("so1n.me") request("github.com") request("google.com") request("baidu.com") # 监听是否有事件在运行 while running_cnt > 0: # 等待事件循环通知事件是否已经完成 for key, mask in selector.select(): key.data()
This code registers four requests at nearly the same time and registers the connection callback, and then enters the event loop logic. That is to say, control is given to the event loop. Until the event loop tells the program that it has received a notification of socket establishment, the program will cancel the registered callback and then send the request, register a read event callback, and then give control to the event. Loop until the response result is received before entering the response result processing function, and the program will not exit until all response results are received. The following is the result of one of my executions:
so1n.me connect success github.com connect success google.com connect success recv google.com body success recv google.com body success baidu.com connect success recv github.com body success recv github.com body success recv baidu.com body success recv baidu.com body success recv so1n.me body success recv so1n.me body success
可以看到他们的执行顺序是随机的, 不是严格的按照so1n.me, github.com, google.com, baidu.com顺序执行, 同时他们执行速度很快, 这个程序的耗时约等于响应时长最长的函数耗时。但是可以看出, 这个程序里面出现了两个回调, 回调会让代码变得非常的奇怪, 降低可读性, 也容易造成回调地狱, 而且当回调发生报错的时候, 我们是很难知道这是由于什么导致的错误, 因为它的上下文丢失了, 这样子排查问题十分的困惑。作为程序员, 一般都不止满足于速度快的代码, 真正想要的是又快, 又能像Sync的代码一样简单, 可读性强, 也能容易排查问题的代码, 这种组合形式的代码的设计模式就叫协程。
协程出现得很早, 它不像线程一样, 被系统调度, 而是能自主的暂停, 并等待事件循环通知恢复。由于协程是软件层面实现的, 所以它的实现方式有很多种, 这里要说的是基于生成器的协程, 因为生成器跟协程一样, 都有暂停让步和恢复的方法(还可以通过throw来抛错), 同时它跟Async语法的协程很像, 通过了解基于生成器的协程, 可以了解Async的协程是如何实现的。
三.基于生成器的协程
3.1生成器
在了解基于生成器的协程之前, 需要先了解下生成器, Python的生成器函数与普通的函数会有一些不同, 只有普通函数中带有关键字yield, 那么它就是生成器函数, 具体有什么不同可以通过他们的字节码来了解:
In [1]: import dis # 普通函数 In [2]: def aaa(): pass In [3]: dis.dis(aaa) 1 0 LOAD_CONST 0 (None) 2 RETURN_VALUE # 普通函数调用函数 In [4]: def bbb(): ...: aaa() ...: In [5]: dis.dis(bbb) 2 0 LOAD_GLOBAL0 (aaa) 2 CALL_FUNCTION0 4 POP_TOP 6 LOAD_CONST 0 (None) 8 RETURN_VALUE # 普通生成器函数 In [6]: def ccc(): yield In [7]: dis.dis(ccc) 1 0 LOAD_CONST 0 (None) 2 YIELD_VALUE 4 POP_TOP 6 LOAD_CONST 0 (None) 8 RETURN_VALUE
上面分别是普通函数, 普通函数调用函数和普通生成器函数的字节码, 从字节码可以看出来, 最简单的函数只需要LOAD_CONST来加载变量None压入自己的栈, 然后通过RETURN_VALUE来返回值, 而有函数调用的普通函数则先加载变量, 把全局变量的函数aaa加载到自己的栈里面, 然后通过CALL_FUNCTION来调用函数, 最后通过POP_TOP把函数的返回值从栈里抛出来, 再把通过LOAD_CONST把None压入自己的栈, 最后返回值。而生成器函数则不一样, 它会先通过LOAD_CONST来加载变量None压入自己的栈, 然后通过YIELD_VALUE返回值, 接着通过POP_TOP弹出刚才的栈并重新把变量None压入自己的栈, 最后通过RETURN_VALUE来返回值。从字节码来分析可以很清楚的看到, 生成器能够在yield区分两个栈帧, 一个函数调用可以分为多次返回, 很符合协程多次等待的特点。
接着来看看生成器的一个使用, 这个生成器会有两次yield调用, 并在最后返回字符串'None', 代码如下:
In [8]: def demo(): ...: a = 1 ...: b = 2 ...: print('aaa', locals()) ...: yield 1 ...: print('bbb', locals()) ...: yield 2 ...: return 'None' ...: In [9]: demo_gen = demo() In [10]: demo_gen.send(None) aaa {'a': 1, 'b': 2} Out[10]: 1 In [11]: demo_gen.send(None) bbb {'a': 1, 'b': 2} Out[11]: 2 In [12]: demo_gen.send(None) --------------------------------------------------------------------------- StopIteration Traceback (most recent call last) <ipython-input-12-8f8cb075d6af> in <module> ----> 1 demo_gen.send(None) StopIteration: None
这段代码首先通过函数调用生成一个demo_gen的生成器对象, 然后第一次send调用时返回值1, 第二次send调用时返回值2, 第三次send调用则抛出StopIteration异常, 异常提示为None, 同时可以看到第一次打印aaa和第二次打印bbb时, 他们都能打印到当前的函数局部变量, 可以发现在即使在不同的栈帧中, 他们读取到当前的局部函数内的局部变量是一致的, 这意味着如果使用生成器来模拟协程时, 它还是会一直读取到当前上下文的, 非常的完美。
此外, Python还支持通过yield from语法来返回一个生成器, 代码如下:
In [1]: def demo_gen_1(): ...: for i in range(3): ...: yield i ...: In [2]: def demo_gen_2(): ...: yield from demo_gen_1() ...: In [3]: demo_gen_obj = demo_gen_2() In [4]: demo_gen_obj.send(None) Out[4]: 0 In [5]: demo_gen_obj.send(None) Out[5]: 1 In [6]: demo_gen_obj.send(None) Out[6]: 2 In [7]: demo_gen_obj.send(None) --------------------------------------------------------------------------- StopIteration Traceback (most recent call last) <ipython-input-7-f9922a2f64c9> in <module> ----> 1 demo_gen_obj.send(None) StopIteration:
通过yield from就可以很方便的支持生成器调用, 假如把每个生成器函数都当做一个协程, 那通过yield from就可以很方便的实现协程间的调用, 此外生成器的抛出异常后的提醒非常人性化, 也支持throw来抛出异常, 这样我们就可以实现在协程运行时设置异常, 比如Cancel,演示代码如下:
In [1]: def demo_exc(): ...: yield 1 ...: raise RuntimeError() ...: In [2]: def demo_exc_1(): ...: for i in range(3): ...: yield i ...: In [3]: demo_exc_gen = demo_exc() In [4]: demo_exc_gen.send(None) Out[4]: 1 In [5]: demo_exc_gen.send(None) --------------------------------------------------------------------------- RuntimeErrorTraceback (most recent call last) <ipython-input-5-09fbb75fdf7d> in <module> ----> 1 demo_exc_gen.send(None) <ipython-input-1-69afbc1f9c19> in demo_exc() 1 def demo_exc(): 2 yield 1 ----> 3 raise RuntimeError() 4 RuntimeError: In [6]: demo_exc_gen_1 = demo_exc_1() In [7]: demo_exc_gen_1.send(None)Out[7]: 0 n [8]: demo_exc_gen_1.send(None) Out[8]: 1 In [9]: demo_exc_gen_1.throw(RuntimeError) --------------------------------------------------------------------------- RuntimeErrorTraceback (most recent call last) <ipython-input-9-1a1cc55d71f4> in <module> ----> 1 demo_exc_gen_1.throw(RuntimeError) <ipython-input-2-2617b2366dce> in demo_exc_1() 1 def demo_exc_1(): 2 for i in range(3): ----> 3 yield i 4 RuntimeError:
从中可以看到在运行中抛出异常时, 会有一个非常清楚的抛错, 可以明显看出错误堆栈, 同时throw指定异常后, 会在下一处yield抛出异常(所以协程调用Cancel后不会马上取消, 而是下一次调用的时候才被取消)。
3.2用生成器实现协程
我们已经简单的了解到了生成器是非常的贴合协程的编程模型, 同时也知道哪些生成器API是我们需要的API, 接下来可以模仿Asyncio的接口来实现一个简单的协程。
首先是在Asyncio中有一个封装叫Feature, 它用来表示协程正在等待将来时的结果, 以下是我根据asyncio.Feature封装的一个简单的Feature, 它的API没有asyncio.Feature全, 代码和注释如下:
class Status: """用于判断Future状态""" pending: int = 1 finished: int = 2 cancelled: int = 3 class Future(object): def __init__(self) -> None: """初始化时, Feature处理pending状态, 等待set result""" self.status: int = Status.pending self._result: Any = None self._exception: Optional[Exception] = None self._callbacks: List[Callable[['Future'], None]] = [] def add_done_callback(self, fn: [['Future'], None]Callable) -> None: """添加完成时的回调""" self._callbacks.append(fn)def cancel(self): """取消当前的Feature""" if self.status != Status.pending: return False self.status = Status.cancelled for fn in self._callbacks: fn(self) return True def set_exception(self, exc: Exception) -> None: """设置异常""" if self.status != Status.pending: raise RuntimeError("Can not set exc") self._exception = exc self.status = Status.finished def set_result(self, result: Any) -> None: """设置结果""" if self.status != Status.pending: raise RuntimeError("Can not set result") self.status = Status.finished self._result = result for fn in self._callbacks: fn(self) def result(self): """获取结果""" if self.status == Status.cancelled: raise asyncio.CancelledError elif self.status != Status.finished: raise RuntimeError("Result is not read") elif self._exception is not None: raise self._exception return self._result def __iter__(self): """通过生成器来模拟协程, 当收到结果通知时, 会返回结果""" if self.status == Status.pending: yield self return self.result()
在理解Future时, 可以把它假想为一个状态机, 在启动初始化的时候是peding状态, 在运行的时候我们可以切换它的状态, 并且通过__iter__方法来支持调用者使用yield from Future()来等待Future本身, 直到收到了事件通知时, 可以得到结果。
但是可以发现这个Future是无法自我驱动, 调用了__iter__的程序不知道何时被调用了set_result, 在Asyncio中是通过一个叫Task的类来驱动Future, 它将一个协程的执行过程安排好, 并负责在事件循环中执行该协程。它主要有两个方法:
1.初始化时, 会先通过send方法激活生成器
2.后续被调度后马上安排下一次等待, 除非抛出StopIteration异常
还有一个支持取消运行托管协程的方法(在原代码中, Task是继承于Future, 所以Future有的它都有), 经过简化后的代码如下:
class Task: def __init__(self, coro: Generator) -> None: # 初始化状态 self.cancelled: bool = False self.coro: Generator = coro # 预激一个普通的future f: Future = Future() f.set_result(None) self.step(f) def cancel(self) -> None: """用于取消托管的coro""" self.coro.throw(asyncio.CancelledError) def step(self, f: Future) -> None: """用于调用coro的下一步, 从第一次激活开始, 每次都添加完成时的回调, 直到遇到取消或者StopIteration异常""" try: _future = self.coro.send(f.result()) except asyncio.CancelledError: self.cancelled = True return except StopIteration: return _future.add_done_callback(self.step)
这样Future和Task就封装好了, 可以简单的试一试效果如何:
In [2]:def wait_future(f: Future, flag_int: int) -> Generator[Future, None, None]: ...:result = yield from f ...:print(flag_int, result) ...: ...:future: Future = Future() ...:for i in range(3): ...:coro = wait_future(future, i) ...:# 托管wait_future这个协程, 里面的Future也会通过yield from被托管 ...:Task(coro) ...: ...:print('ready') ...:future.set_result('ok') ...: ...:future = Future() ...:Task(wait_future(future, 3)).cancel() ...:ready 0 ok 1 ok 2 ok --------------------------------------------------------------------------- CancelledErrorTraceback (most recent call last) <ipython-input-2-2d1b04db2604> in <module> 12 13 future = Future() ---> 14 Task(wait_future(future, 3)).cancel() <ipython-input-1-ec3831082a88> in cancel(self) 81 82 def cancel(self) -> None: ---> 83 self.coro.throw(asyncio.CancelledError) 84 85 def step(self, f: Future) -> None: <ipython-input-2-2d1b04db2604> in wait_future(f, flag_int) 1 def wait_future(f: Future, flag_int: int) -> Generator[Future, None, None]: ----> 2 result = yield from f 3 print(flag_int, result) 4 5 future: Future = Future() <ipython-input-1-ec3831082a88> in __iter__(self) 68 """通过生成器来模拟协程, 当收到结果通知时, 会返回结果""" 69 if self.status == Status.pending: ---> 70 yield self 71 return self.result() 72 CancelledError:
这段程序会先初始化Future, 并把Future传给wait_future并生成生成器, 再交由给Task托管, 预激, 由于Future是在生成器函数wait_future中通过yield from与函数绑定的, 真正被预激的其实是Future的__iter__方法中的yield self, 此时代码逻辑会暂停在yield self并返回。在全部预激后, 通过调用Future的set_result方法, 使Future变为结束状态, 由于set_result会执行注册的回调, 这时它就会执行托管它的Task的step方法中的send方法, 代码逻辑回到Future的__iter__方法中的yield self, 并继续往下走, 然后遇到return返回结果, 并继续走下去, 从输出可以发现程序封装完成且打印了ready后, 会依次打印对应的返回结果, 而在最后一个的测试cancel方法中可以看到,Future抛出异常了, 同时这些异常很容易看懂, 能够追随到调用的地方。
现在Future和Task正常运行了, 可以跟我们一开始执行的程序进行整合, 代码如下:
class HttpRequest(object): def __init__(self, host: str): """初始化变量和sock""" self._host: str = host global running_cnt running_cnt += 1 self.url: str = f"http://{host}" self.sock: socket.SocketType = socket.socket() self.sock.setblocking(False) try: self.sock.connect((host, 80)) except BlockingIOError: pass def read(self) -> Generator[Future, None, bytes]: """从socket获取响应数据, 并set到Future中, 并通过Future.__iter__方法或得到数据并通过变量chunk_future返回""" f: Future = Future() selector.register(self.sock.fileno(), EVENT_READ, lambda: f.set_result(self.sock.recv(4096))) chunk_future = yield from f selector.unregister(self.sock.fileno()) return chunk_future# type: ignore def read_response(self) -> Generator[Future, None, bytes]: """接收响应参数, 并判断请求是否结束""" response_bytes: bytes = b"" chunk = yield from self.read() while chunk: response_bytes += chunk chunk = yield from self.read() return response_bytes def connected(self) -> Generator[Future, None, None]: """socket建立连接时的回调""" # 取消监听 f: Future = Future() selector.register(self.sock.fileno(), EVENT_WRITE, lambda: f.set_result(None)) yield f selector.unregister(self.sock.fileno()) print(f"{self._host} connect success") def request(self) -> Generator[Future, None, None]: # 发送请求, 并监听读事件, 以及注册对应的接收响应函数 yield from self.connected() self.sock.send(f"GET {self.url} HTTP/1.0rnHost: {self._host}rnrn".encode("ascii")) response = yield from self.read_response() print(f"request {self._host} success, length:{len(response)}") global running_cnt running_cnt -= 1 if __name__ == "__main__": # 同时多个请求 Task(HttpRequest("so1n.me").request()) Task(HttpRequest("github.com").request()) Task(HttpRequest("google.com").request()) Task(HttpRequest("baidu.com").request()) # 监听是否有事件在运行 while running_cnt > 0: # 等待事件循环通知事件是否已经完成 for key, mask in selector.select(): key.data()
这段代码通过Future和生成器方法尽量的解耦回调函数, 如果忽略了HttpRequest中的connected和read方法则可以发现整段代码跟同步的代码基本上是一样的, 只是通过yield和yield from交出控制权和通过事件循环恢复控制权。同时通过上面的异常例子可以发现异常排查非常的方便, 这样一来就没有了回调的各种糟糕的事情, 开发者只需要按照同步的思路进行开发即可, 不过我们的事件循环是一个非常简单的事件循环例子, 同时对于socket相关都没有进行封装, 也缺失一些常用的API, 而这些都会被Python官方封装到Asyncio这个库中, 通过该库, 我们可以近乎完美的编写Async语法的代码。
The above is the detailed content of What exactly is going on with coroutines in Python?. For more information, please follow other related articles on the PHP Chinese website!
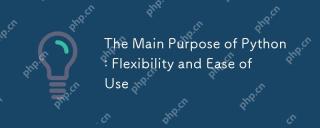
Python's flexibility is reflected in multi-paradigm support and dynamic type systems, while ease of use comes from a simple syntax and rich standard library. 1. Flexibility: Supports object-oriented, functional and procedural programming, and dynamic type systems improve development efficiency. 2. Ease of use: The grammar is close to natural language, the standard library covers a wide range of functions, and simplifies the development process.
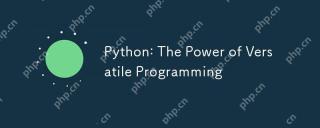
Python is highly favored for its simplicity and power, suitable for all needs from beginners to advanced developers. Its versatility is reflected in: 1) Easy to learn and use, simple syntax; 2) Rich libraries and frameworks, such as NumPy, Pandas, etc.; 3) Cross-platform support, which can be run on a variety of operating systems; 4) Suitable for scripting and automation tasks to improve work efficiency.
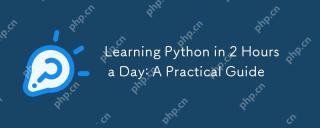
Yes, learn Python in two hours a day. 1. Develop a reasonable study plan, 2. Select the right learning resources, 3. Consolidate the knowledge learned through practice. These steps can help you master Python in a short time.
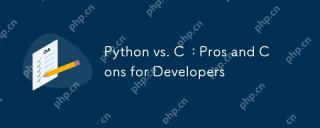
Python is suitable for rapid development and data processing, while C is suitable for high performance and underlying control. 1) Python is easy to use, with concise syntax, and is suitable for data science and web development. 2) C has high performance and accurate control, and is often used in gaming and system programming.
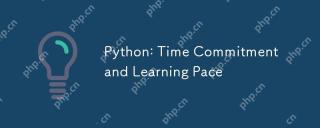
The time required to learn Python varies from person to person, mainly influenced by previous programming experience, learning motivation, learning resources and methods, and learning rhythm. Set realistic learning goals and learn best through practical projects.
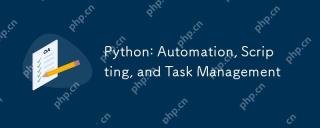
Python excels in automation, scripting, and task management. 1) Automation: File backup is realized through standard libraries such as os and shutil. 2) Script writing: Use the psutil library to monitor system resources. 3) Task management: Use the schedule library to schedule tasks. Python's ease of use and rich library support makes it the preferred tool in these areas.
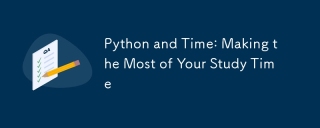
To maximize the efficiency of learning Python in a limited time, you can use Python's datetime, time, and schedule modules. 1. The datetime module is used to record and plan learning time. 2. The time module helps to set study and rest time. 3. The schedule module automatically arranges weekly learning tasks.
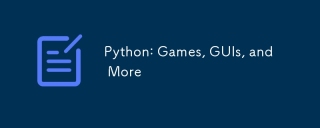
Python excels in gaming and GUI development. 1) Game development uses Pygame, providing drawing, audio and other functions, which are suitable for creating 2D games. 2) GUI development can choose Tkinter or PyQt. Tkinter is simple and easy to use, PyQt has rich functions and is suitable for professional development.


Hot AI Tools

Undresser.AI Undress
AI-powered app for creating realistic nude photos

AI Clothes Remover
Online AI tool for removing clothes from photos.

Undress AI Tool
Undress images for free

Clothoff.io
AI clothes remover

AI Hentai Generator
Generate AI Hentai for free.

Hot Article

Hot Tools

SublimeText3 English version
Recommended: Win version, supports code prompts!

SecLists
SecLists is the ultimate security tester's companion. It is a collection of various types of lists that are frequently used during security assessments, all in one place. SecLists helps make security testing more efficient and productive by conveniently providing all the lists a security tester might need. List types include usernames, passwords, URLs, fuzzing payloads, sensitive data patterns, web shells, and more. The tester can simply pull this repository onto a new test machine and he will have access to every type of list he needs.

SAP NetWeaver Server Adapter for Eclipse
Integrate Eclipse with SAP NetWeaver application server.
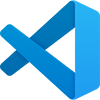
VSCode Windows 64-bit Download
A free and powerful IDE editor launched by Microsoft
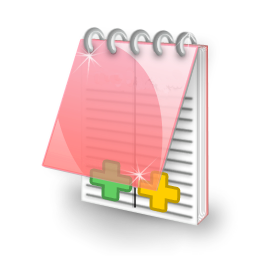
EditPlus Chinese cracked version
Small size, syntax highlighting, does not support code prompt function