


Can you tell if you have diarrhea by listening to the sound of defecation? After listening to 350 toilet sounds, this AI recognition accuracy reached 98%
Produced by Big Data Digest
Author: Caleb
Feces can be said to be a great "treasure" excreted by humans.
The shape of stool can not only help judge a person's health, but may even leak your personal information. This is enough to show that stool also plays a very important role in the field of AI. Role.
Now, there is new research showing that the sound of defecation can also be used as medical evidence. It seems that the research on stool is getting more and more complicated.
Researchers affiliated with the Georgia Institute of Technology in the United States published a paper The feces thesis: Using machine learning to detect diarrhea. The paper proposed a machine learning model that uses a machine installed in the toilet. Sensors are used to record the sound of defecation to determine whether a person has diarrhea symptoms.
Paper link:
https://asa.scitation.org/doi/10.1121/10.0015504
It seems that this study not only has a smell, but also a sound~
The accuracy is 98%, but the algorithm listened to 350 defecation sounds
We know that cholera, a bacterial disease that causes diarrhea, affects millions of people every year and causes about 150,000 deaths.
But while early detection and alerting health professionals can effectively mitigate the impact of cholera, monitoring similar intestinal diseases remains a sensitive issue.
So, researchers proposed a new detection method, the sound of defecation.
Install a sensor in the toilet and a built-in microphone to record the sound of defecation. Then machine learning can analyze the sound and use an indicator light to indicate the presence of diarrhea.
When analyzing the sound, the system will first convert the sound of defecation into a An image called a spectrogram. Depending on the situation, sounds and spectrograms have different characteristics. For example, urinating produces a consistent tone, while bowel movements have a specific tone, and diarrhea is more random.
The generated spectrum images are then sent to machine learning algorithms to classify based on different features.
To train the algorithm, the researchers used 350 audio clips from YouTube and the sound database Soundsnap toilet-related sound data, covering standard defecation, diarrhea, urination, and gas.
According to the test results, when background noise such as voices is removed, the system's accuracy can reach 98%; when the background noise is retained, the system's accuracy is 96% .
It seems that the system can still distinguish diarrhea or non-diarrhoea relatively accurately based on the sound of defecation.
Maia Gatlin, the first author of the paper, said, “We hope that this small-footprint sensor can be installed in areas where cholera continues to be affected.” “This sensor can also be used in disaster areas (water pollution). leading to the spread of waterborne pathogens) and could even be used in nursing/hospice facilities to automatically monitor patients' bowel movements. Perhaps one day, this algorithm could be combined with existing home smart devices to monitor their own bowel movements and health."
The researchers said they hope to not only conduct research on defecation sounds in the future, but also include urination sounds in order to predict abnormal changes in various diseases.
emmm Digest bacteria just want to say: Don’t come over!
Stool research also followed closely
Just imagine, if this research could be combined with stool research, what would happen? No... no, this picture is a bit too beautiful. Digest Fungi said that he didn't dare to look at it.
But it’s true that many gastroenterologists hope to learn more by analyzing a patient’s stool and looking for abnormalities that may be signs of inflammatory bowel disease or irritable bowel syndrome. The patient's gut health.
For example, last May, Duke University developed a new technology that can automate most steps in this process within the existing toilet system. In addition, Can use AI to scan and classify flushed feces.
This is the Smart Toilet proposed by Duke University.
The system is designed to be installed in existing toilet pipes to collect excrement images. The supporting AI algorithm has been trained on more than 3,000 unique stool images. These stools are Has been classified by gastroenterologists as loose, normal, constipated and with or without blood.
The algorithm can then analyze the image itself. The algorithm accurately classified stool 85% of the time and accurately detected blood 76% of the time.
Sonia Grego, the study's lead researcher, said: "We are optimistic about patients' willingness to use this technology because it can be installed in the toilet pipes and patients do not need to do anything other than flush. This is very important for patients. This is especially useful for patients who may not be able to report their condition, such as those living in long-term care facilities." Deborah Fisher, lead author of the study, said: "Typically, the stomach Enterologists must rely on patients' self-reported information about their stools to help determine the cause of their gastrointestinal health problems, which can be highly unreliable. Patients often cannot remember what their stools looked like or how often they had a bowel movement, which can be It is part of the standard monitoring process. Smart Toilet technology will allow us to collect the long-term information needed to more accurately and timely diagnose chronic gastrointestinal diseases."
I didn’t expect that one day, The poop is really rolled up.
Related reports:
https://www.itmedia.co.jp/news/articles/2212/22/news067.html
https://www.eurekalert.org/news-releases/972604
https://medicalxpress.com/news/2022-12-feces-thesis-machine-diarrhea.html
https://www.cnbeta.com.tw/articles/science/1132335.htm
The above is the detailed content of Can you tell if you have diarrhea by listening to the sound of defecation? After listening to 350 toilet sounds, this AI recognition accuracy reached 98%. For more information, please follow other related articles on the PHP Chinese website!
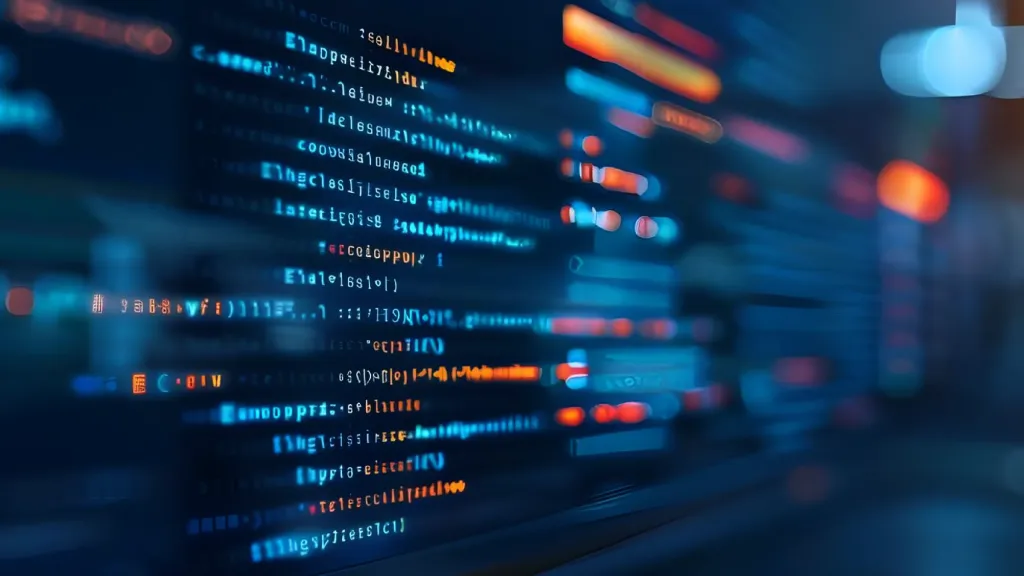
Running large language models at home with ease: LM Studio User Guide In recent years, advances in software and hardware have made it possible to run large language models (LLMs) on personal computers. LM Studio is an excellent tool to make this process easy and convenient. This article will dive into how to run LLM locally using LM Studio, covering key steps, potential challenges, and the benefits of having LLM locally. Whether you are a tech enthusiast or are curious about the latest AI technologies, this guide will provide valuable insights and practical tips. Let's get started! Overview Understand the basic requirements for running LLM locally. Set up LM Studi on your computer
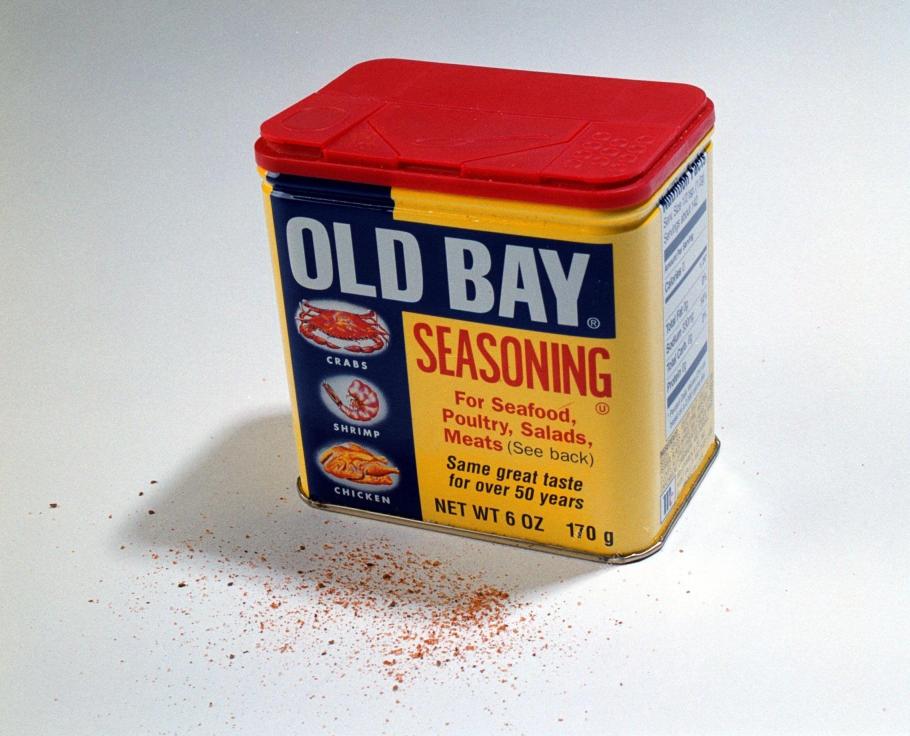
Guy Peri is McCormick’s Chief Information and Digital Officer. Though only seven months into his role, Peri is rapidly advancing a comprehensive transformation of the company’s digital capabilities. His career-long focus on data and analytics informs
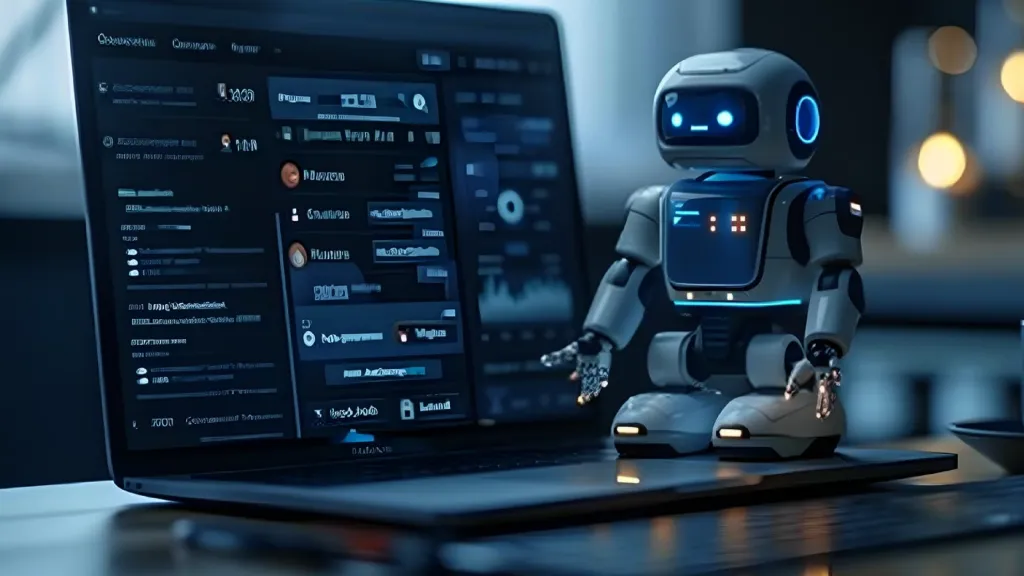
Introduction Artificial intelligence (AI) is evolving to understand not just words, but also emotions, responding with a human touch. This sophisticated interaction is crucial in the rapidly advancing field of AI and natural language processing. Th
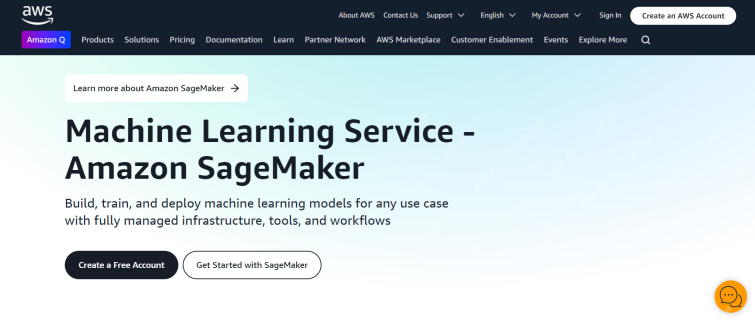
Introduction In today's data-centric world, leveraging advanced AI technologies is crucial for businesses seeking a competitive edge and enhanced efficiency. A range of powerful tools empowers data scientists, analysts, and developers to build, depl
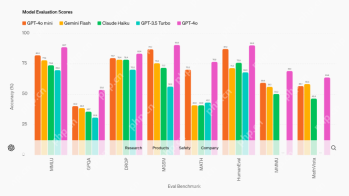
This week's AI landscape exploded with groundbreaking releases from industry giants like OpenAI, Mistral AI, NVIDIA, DeepSeek, and Hugging Face. These new models promise increased power, affordability, and accessibility, fueled by advancements in tr
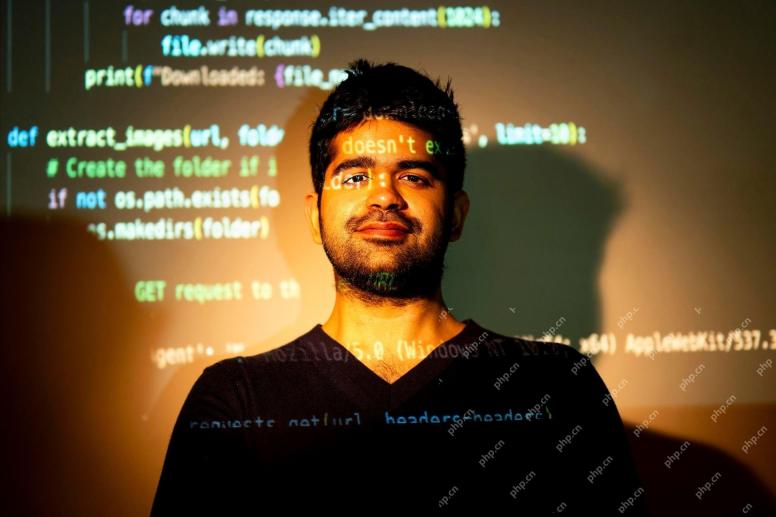
But the company’s Android app, which offers not only search capabilities but also acts as an AI assistant, is riddled with a host of security issues that could expose its users to data theft, account takeovers and impersonation attacks from malicious
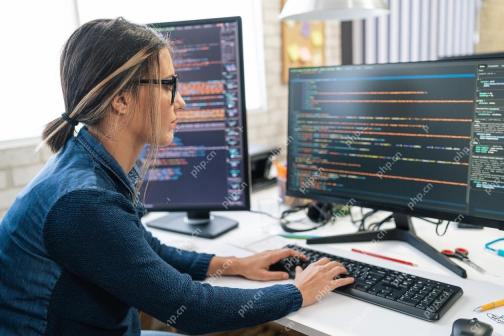
You can look at what’s happening in conferences and at trade shows. You can ask engineers what they’re doing, or consult with a CEO. Everywhere you look, things are changing at breakneck speed. Engineers, and Non-Engineers What’s the difference be
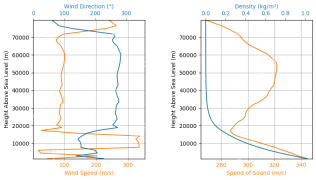
Simulate Rocket Launches with RocketPy: A Comprehensive Guide This article guides you through simulating high-power rocket launches using RocketPy, a powerful Python library. We'll cover everything from defining rocket components to analyzing simula


Hot AI Tools

Undresser.AI Undress
AI-powered app for creating realistic nude photos

AI Clothes Remover
Online AI tool for removing clothes from photos.

Undress AI Tool
Undress images for free

Clothoff.io
AI clothes remover

Video Face Swap
Swap faces in any video effortlessly with our completely free AI face swap tool!

Hot Article

Hot Tools

SublimeText3 English version
Recommended: Win version, supports code prompts!
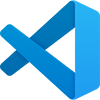
VSCode Windows 64-bit Download
A free and powerful IDE editor launched by Microsoft

SAP NetWeaver Server Adapter for Eclipse
Integrate Eclipse with SAP NetWeaver application server.

SublimeText3 Linux new version
SublimeText3 Linux latest version
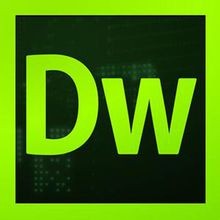
Dreamweaver CS6
Visual web development tools