


Google's incredible 'night vision” camera suddenly became popular! Perfect noise reduction and 3D perspective synthesis
Recently, an AI night scene shooting video from Google has gone viral!
The technology in the video is called RawNeRF, which as the name suggests is a new variant of NeRF.
NeRF is a fully connected neural network that uses 2D image information as training data to restore 3D scenes.
RawNeRF has many improvements compared to the previous NeRF. Not only does it perfectly reduce noise, but it also changes the camera perspective and adjusts focus, exposure and tone mapping. This paper by Google was published in November 2021 and included in CVPR 2022.
## Project address: https://bmild.github.io/rawnerf/
在黑夜的RawNeRFPreviously, NeRF used tone-mapped low dynamic range LDR images as input.
Google's RawNeRF instead trains directly on linear raw images, which can retain the full dynamic range of the scene.
In the field of composite views, dealing with dark photos has always been a problem.
Because in this case there is minimal detail in the image. And these images make it difficult to stitch new views together.
Fortunately, we have a new solution - using the data of the original sensor (RAW sensor data).
It's a picture like this, so we have more details.
However, there is still a problem: there is too much noise.
So we have to make a choice: less detail and less noise, or more detail and more noise .
The good news is: we can use image noise reduction technology.
It can be seen that the image effect after noise reduction is good, but when it comes to composite views, this quality is still not enough.
However, image denoising technology provides us with an idea: since we can denoise a single image, we can also denoise a group of images.
Let’s take a look at the effect of RawNeRF.
And, it has more amazing features: tone mapping the underlying data to extract more details from dark images .
For example, changing the focus of the image creates a great depth of field effect.
What’s even more amazing is that this is still real-time.
In addition, the exposure of the image will also change accordingly as the focus changes!
Next, let us take a look at five classic application scenarios of RawNeRF.
Five classic scenes
1. Image clarity
Look at this image, you Can you see the information on the street signs?
It can be seen that after RawNeRF processing, the information on the road signs is much clearer.
In the following animation, we can clearly see the difference in image synthesis between original NeRF technology and RawNeRF.
In fact, the so-called NeRF is not that old technology, it has only been 2 years...
It can be seen that RawNeRF performs very well in processing highlights. We can even see the highlight changes around the license plate in the lower right corner.
2. Specular Highlight
Specular highlight is a very difficult object to capture because it is difficult to capture when moving the camera. , they will change a lot, and the relative distance between the photos will be relatively far. These factors are huge challenges for learning algorithms.
As you can see in the picture below, the specular highlight generated by RawNeRF can be said to be quite restored.
3. Thin structure
Even in well-lit situations , the previous technology did not display the fence well.
And RawNeRF can handle night photos with a bunch of fences, and it can hold it properly.
The effect is still very good even where the fence overlaps the license plate.
4. Mirror reflection
The reflection on the road is a A more challenging specular highlight. As you can see, RawNeRF also handles it very naturally and realistically.
5. Change focus and adjust exposure
In this scene , let's try changing the perspective, constantly changing the focus, and adjusting the exposure at the same time.
In the past, to complete these tasks, we needed a collection of anywhere from 25 to 200 photos.
Now, we only need a few seconds to complete the shooting.
Of course, RawNeRF is not perfect now. We can see that there are still some differences between the RawNeRF image on the left and the real photo on the right.
However, RAWnerf has made considerable progress from a set of original images full of noise to the current effect. You know, the technology two years ago was completely unable to do this.
Benefits of RAW
To briefly review, the NeRF training pipeline receives LDR images processed by the camera, and subsequent scene reconstruction and view rendering are based on is the LDR color space. Therefore, the output of NeRF has actually been post-processed, and it is impossible to significantly modify and edit it.
In contrast, RawNeRF is trained directly on linear raw HDR input data. The resulting rendering can be edited like any original photo, changing focus and exposure, etc.
The main benefits brought by this are two points: HDR view synthesis and noise reduction processing.
In scenes with extreme changes in brightness, a fixed shutter speed is not enough to capture the full dynamic range. The RawNeRF model can optimize both short and long exposures simultaneously to restore the full dynamic range.
For example, the large light ratio scene in (b) requires a more complex local tone mapping algorithm (such as HDR post-processing) to preserve the details of the dark parts and the outdoor scenes at the same time. highlight.
In addition, RawNeRF can also render synthetic defocus effects with correctly saturated "blurred" highlights using linear color.
In terms of image noise processing, the author further trained RawNeRF on completely unprocessed HDR linear original images to make it It becomes a "noiser" that can process dozens or even hundreds of input images.
This kind of robustness means that RawNeRF can excellently complete the task of reconstructing scenes in the dark.
For example, in (a) this night scene illuminated by only one candle, RawNeRF can extract details from the noisy raw data that would otherwise be destroyed by post-processing (b, c) .
Author introduction
The first author of the paper, Ben Mildenhall, is a researcher at Google Research Scientists working on problems in computer vision and graphics.
He received a bachelor's degree in computer science and mathematics from Stanford University in 2015, and a PhD in computer science from the University of California, Berkeley in 2020 .
The just-concluded CVPR 2022 can be said to be Ben’s highlight moment.
5 out of the 7 accepted papers won Oral, and one won the honorable mention for the best student paper.
Netizen comments
As soon as the video came out, it immediately amazed all netizens. Let's all have some fun together.
Looking at the speed of technological advancement, it won’t be long before you no longer have to worry about taking photos at night. Got~
The above is the detailed content of Google's incredible 'night vision” camera suddenly became popular! Perfect noise reduction and 3D perspective synthesis. For more information, please follow other related articles on the PHP Chinese website!
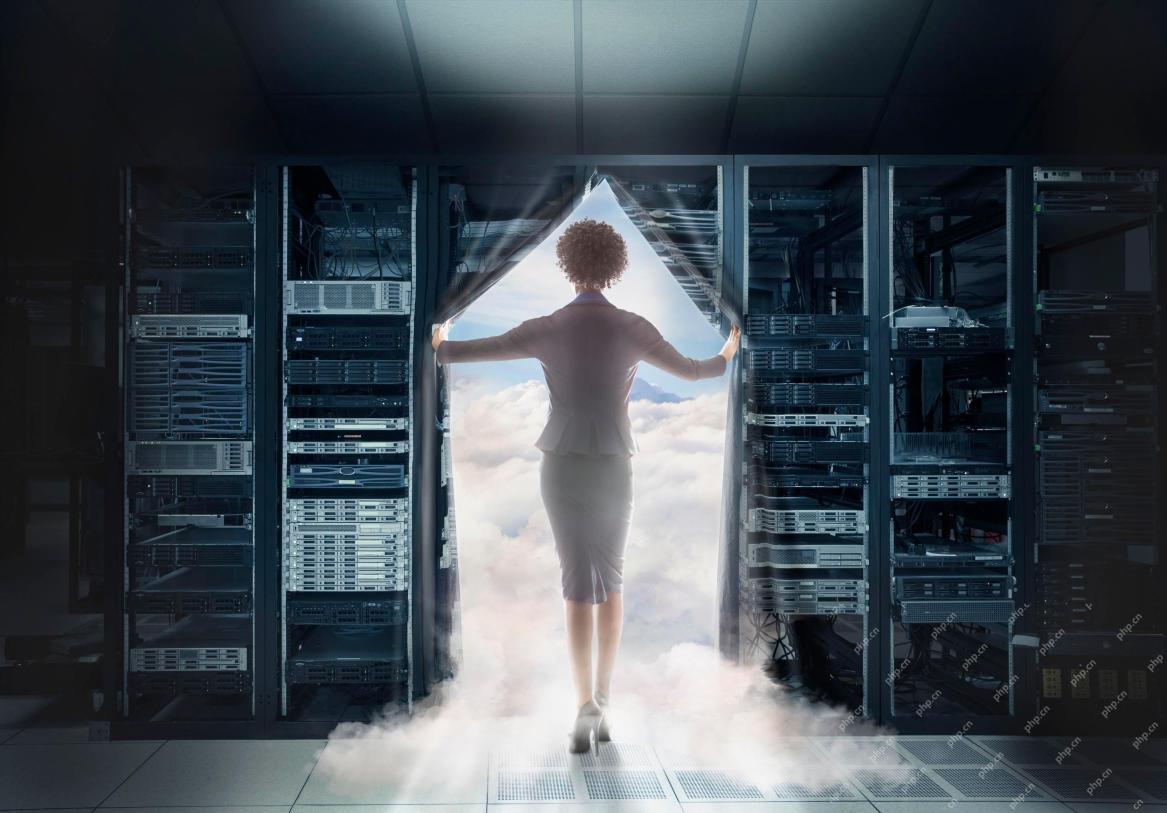
In John Rawls' seminal 1971 book The Theory of Justice, he proposed a thought experiment that we should take as the core of today's AI design and use decision-making: the veil of ignorance. This philosophy provides a simple tool for understanding equity and also provides a blueprint for leaders to use this understanding to design and implement AI equitably. Imagine that you are making rules for a new society. But there is a premise: you don’t know in advance what role you will play in this society. You may end up being rich or poor, healthy or disabled, belonging to a majority or marginal minority. Operating under this "veil of ignorance" prevents rule makers from making decisions that benefit themselves. On the contrary, people will be more motivated to formulate public
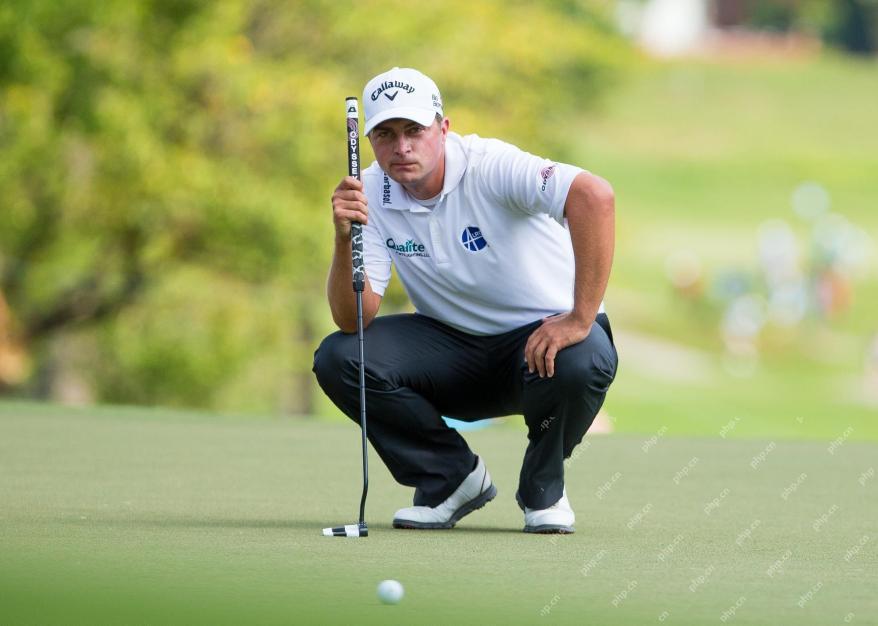
Numerous companies specialize in robotic process automation (RPA), offering bots to automate repetitive tasks—UiPath, Automation Anywhere, Blue Prism, and others. Meanwhile, process mining, orchestration, and intelligent document processing speciali
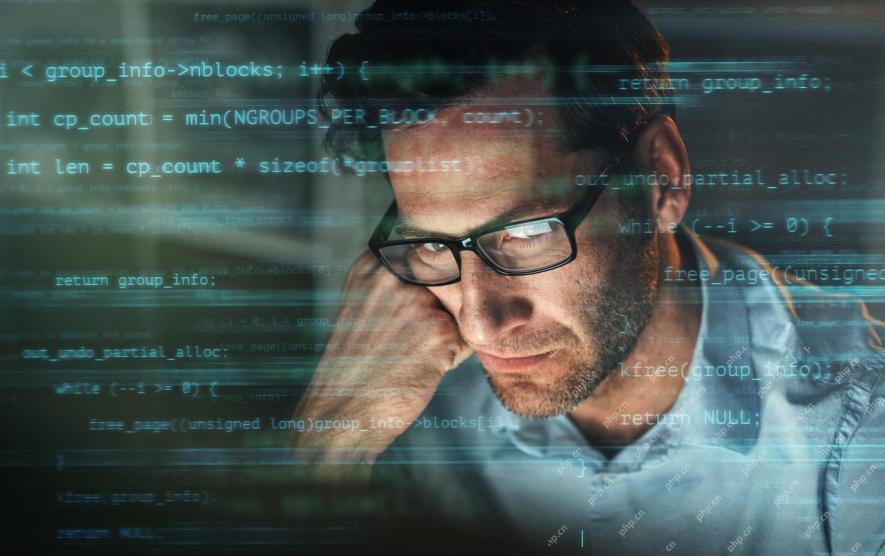
The future of AI is moving beyond simple word prediction and conversational simulation; AI agents are emerging, capable of independent action and task completion. This shift is already evident in tools like Anthropic's Claude. AI Agents: Research a
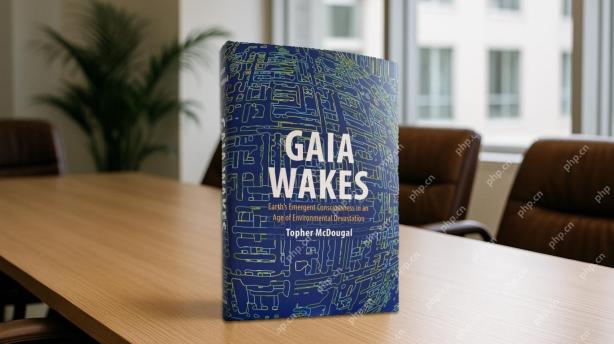
Rapid technological advancements necessitate a forward-looking perspective on the future of work. What happens when AI transcends mere productivity enhancement and begins shaping our societal structures? Topher McDougal's upcoming book, Gaia Wakes:
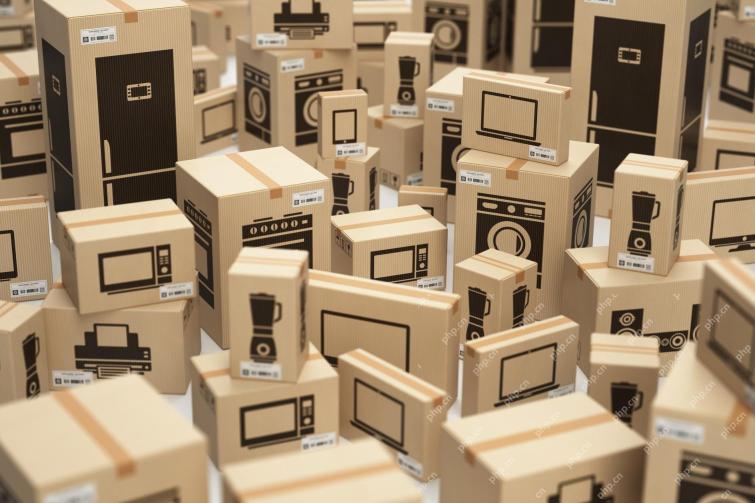
Product classification, often involving complex codes like "HS 8471.30" from systems such as the Harmonized System (HS), is crucial for international trade and domestic sales. These codes ensure correct tax application, impacting every inv
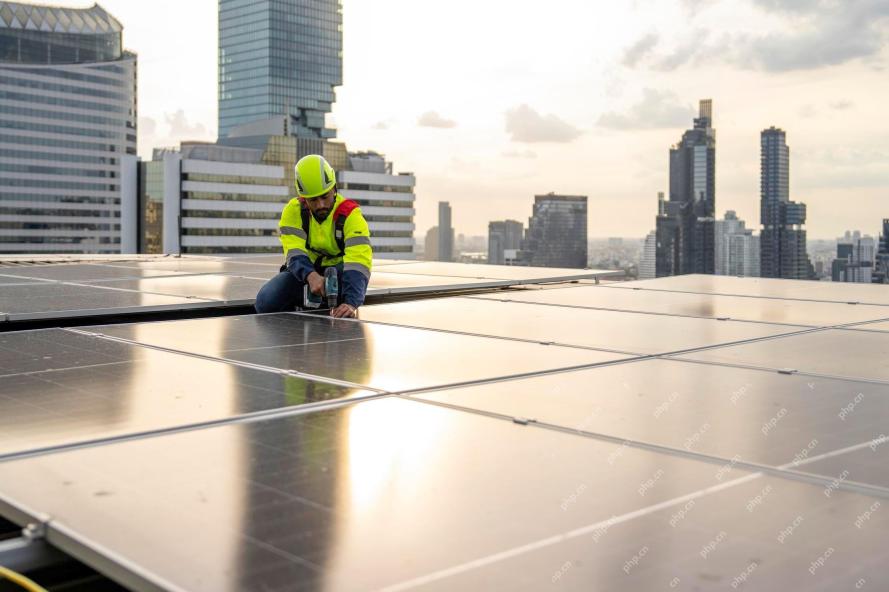
The future of energy consumption in data centers and climate technology investment This article explores the surge in energy consumption in AI-driven data centers and its impact on climate change, and analyzes innovative solutions and policy recommendations to address this challenge. Challenges of energy demand: Large and ultra-large-scale data centers consume huge power, comparable to the sum of hundreds of thousands of ordinary North American families, and emerging AI ultra-large-scale centers consume dozens of times more power than this. In the first eight months of 2024, Microsoft, Meta, Google and Amazon have invested approximately US$125 billion in the construction and operation of AI data centers (JP Morgan, 2024) (Table 1). Growing energy demand is both a challenge and an opportunity. According to Canary Media, the looming electricity
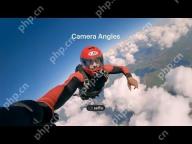
Generative AI is revolutionizing film and television production. Luma's Ray 2 model, as well as Runway's Gen-4, OpenAI's Sora, Google's Veo and other new models, are improving the quality of generated videos at an unprecedented speed. These models can easily create complex special effects and realistic scenes, even short video clips and camera-perceived motion effects have been achieved. While the manipulation and consistency of these tools still need to be improved, the speed of progress is amazing. Generative video is becoming an independent medium. Some models are good at animation production, while others are good at live-action images. It is worth noting that Adobe's Firefly and Moonvalley's Ma
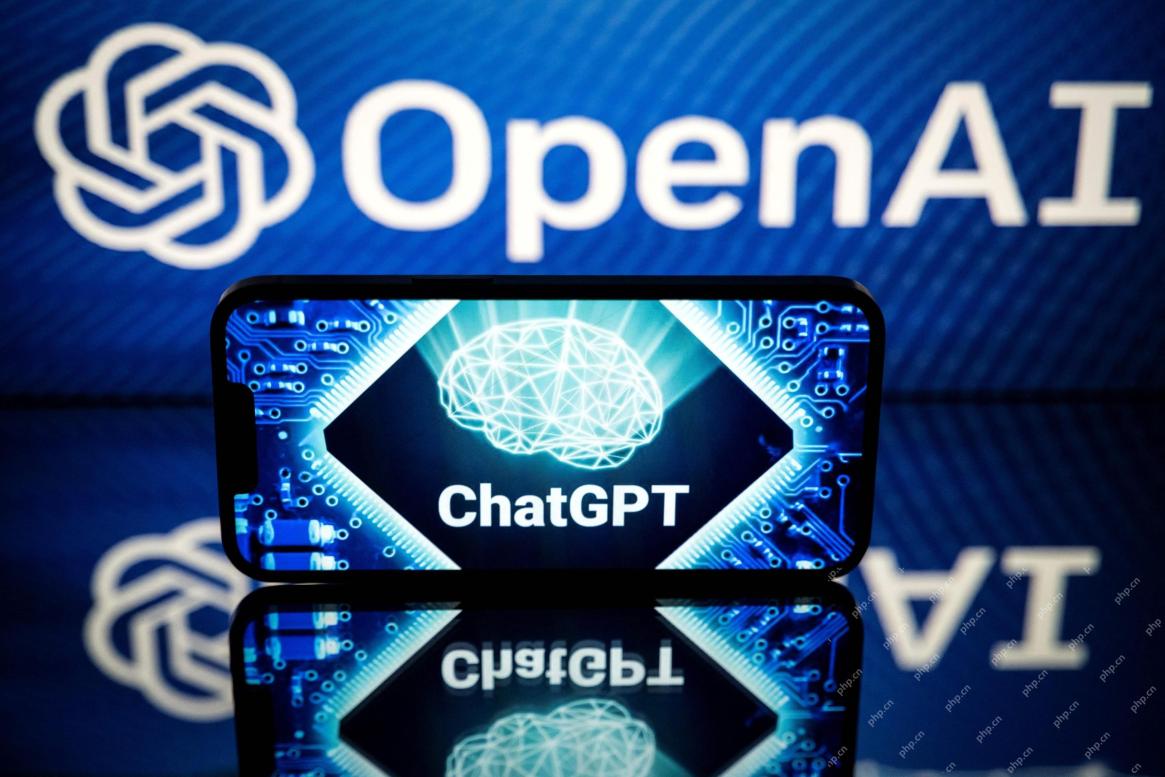
ChatGPT user experience declines: is it a model degradation or user expectations? Recently, a large number of ChatGPT paid users have complained about their performance degradation, which has attracted widespread attention. Users reported slower responses to models, shorter answers, lack of help, and even more hallucinations. Some users expressed dissatisfaction on social media, pointing out that ChatGPT has become “too flattering” and tends to verify user views rather than provide critical feedback. This not only affects the user experience, but also brings actual losses to corporate customers, such as reduced productivity and waste of computing resources. Evidence of performance degradation Many users have reported significant degradation in ChatGPT performance, especially in older models such as GPT-4 (which will soon be discontinued from service at the end of this month). this


Hot AI Tools

Undresser.AI Undress
AI-powered app for creating realistic nude photos

AI Clothes Remover
Online AI tool for removing clothes from photos.

Undress AI Tool
Undress images for free

Clothoff.io
AI clothes remover

Video Face Swap
Swap faces in any video effortlessly with our completely free AI face swap tool!

Hot Article

Hot Tools

Atom editor mac version download
The most popular open source editor
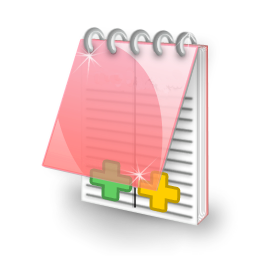
EditPlus Chinese cracked version
Small size, syntax highlighting, does not support code prompt function
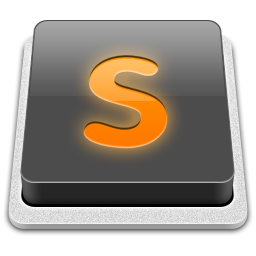
SublimeText3 Mac version
God-level code editing software (SublimeText3)

Safe Exam Browser
Safe Exam Browser is a secure browser environment for taking online exams securely. This software turns any computer into a secure workstation. It controls access to any utility and prevents students from using unauthorized resources.

SAP NetWeaver Server Adapter for Eclipse
Integrate Eclipse with SAP NetWeaver application server.
