


MIT researchers use AI to help self-driving cars avoid idling at red lights
What if drivers could schedule their trips precisely so that they went straight through the traffic lights every time?
While this might just be possible for human drivers It happens under particularly lucky circumstances, but it could also be achieved more stably by autonomous vehicles using AI to control their speed.
In a new study, scientists at the Massachusetts Institute of Technology (MIT) demonstrate a machine learning approach. The method can learn to control a fleet of autonomous vehicles to keep traffic flowing as they approach and pass through a signalized intersection.
According to simulation results, their method can reduce fuel consumption and emissions while increasing average vehicle speed. The technology will work best if all cars on the road are autonomous, but even if only 25% of cars use their control algorithms, it will still bring huge fuel and emissions benefits.
"This is a very interesting place to intervene. No one's life is better because they are stuck at an intersection. There is an expectation in many other climate change interventions quality of life difference, so there's a barrier to entry there," said Cathy Wu, senior author of the study. It is reported that he is the Gilbert W. Winslow Career Development Assistant Professor in the Department of Civil and Environmental Engineering and a member of the Institute for Data, Systems and Society (IDSS) and the Laboratory of Information and Decision Systems (LIDS).
LIDS and Vindula Jayawardana, a graduate student in the Department of Electrical Engineering and Computer Science, are both first authors of the study. The research will be presented at the European Control Conference.
Intricate Intersections
While humans may drive through green lights without thinking, intersections can occur in billions of different situations, depending on the number of lanes, the operation of the lights mode, number and speed of vehicles, presence of pedestrians and cyclists, etc.
The typical approach to solving intersection control problems is to use mathematical models to solve a simple, ideal intersection. This looks good on paper, but it's likely not to hold up in the real world, where traffic patterns are often chaotic.
In this regard, Wu and Jayawardana thought from a different angle. They used a model-free technology called deep reinforcement learning to deal with this problem. Reinforcement learning is a trial and error method in which a control algorithm learns to make a series of decisions. When it finds a good sequence it is rewarded. With deep reinforcement learning, algorithms use hypotheses learned by neural networks to find shortcuts to good sequences—even if there are billions of possibilities.
This is useful for solving long-term problems like this. Wu pointed out that the control algorithm must issue more than 500 acceleration instructions to the vehicle over a long period of time. Additionally, she added: "And we have to get the sequence right before we know we've mitigated emissions well and are at the intersection at a good pace." That is, the researchers wanted the system to learn a strategy to reduce fuel consumption and limit the impact on travel time. These goals may be in conflict with each other.
"To reduce travel time, we want the car to drive fast, but to reduce emissions, we want the car to slow down or not move at all. These competing rewards can be very confusing for a learning agent," Wu said.
While addressing the generality of this problem is challenging, the researchers used a technique called reward shaping to work around it. Through reward shaping, they give the system some domain knowledge that it cannot learn on its own. In this case, they punish the system every time the vehicle comes to a complete stop so that it learns to avoid this behavior.
TRAFFIC TESTING
Once the researchers develop an effective control algorithm, they evaluate it using a traffic simulation platform with a single intersection. The control algorithm is applied to a fleet of networked autonomous vehicles that communicate with upcoming traffic lights to receive phase and timing information from the lights and observe their surroundings. This control algorithm tells each vehicle how to accelerate and decelerate.
Their system did not cause any stop-and-go traffic as the vehicle approached the intersection. In the simulation, more cars passed during a single green light phase than a model simulating a human driver. When compared with other optimization methods also aimed at avoiding stop-and-go traffic, their technology resulted in greater fuel consumption and emissions reductions. If every car on the road were autonomous, their control systems could reduce fuel consumption by 18% and CO2 emissions by 25%, while driving 20% faster.
Wu said: “It’s really incredible to have a 20% to 25% reduction in fuel or emissions from one intervention. But what I find interesting, and what I really want to see, is this nonlinearity. scale. If we only control 25% of the vehicles, this gives us the benefit of 50% fuel and emissions reduction. This means we don’t have to wait until we reach 100% autonomous vehicles to benefit from this approach.”
Next, the researchers hope to study the interactive effects between multiple intersections. Additionally, they plan to explore how different intersection settings such as number of lanes, lights, timing, etc. affect travel times, emissions, and fuel consumption. Additionally, they plan to study how their control systems impact safety when autonomous vehicles share the road with human drivers.
While this work is still in its early stages, Wu believes this approach could be more feasible to implement in the near future.
The above is the detailed content of MIT researchers use AI to help self-driving cars avoid idling at red lights. For more information, please follow other related articles on the PHP Chinese website!
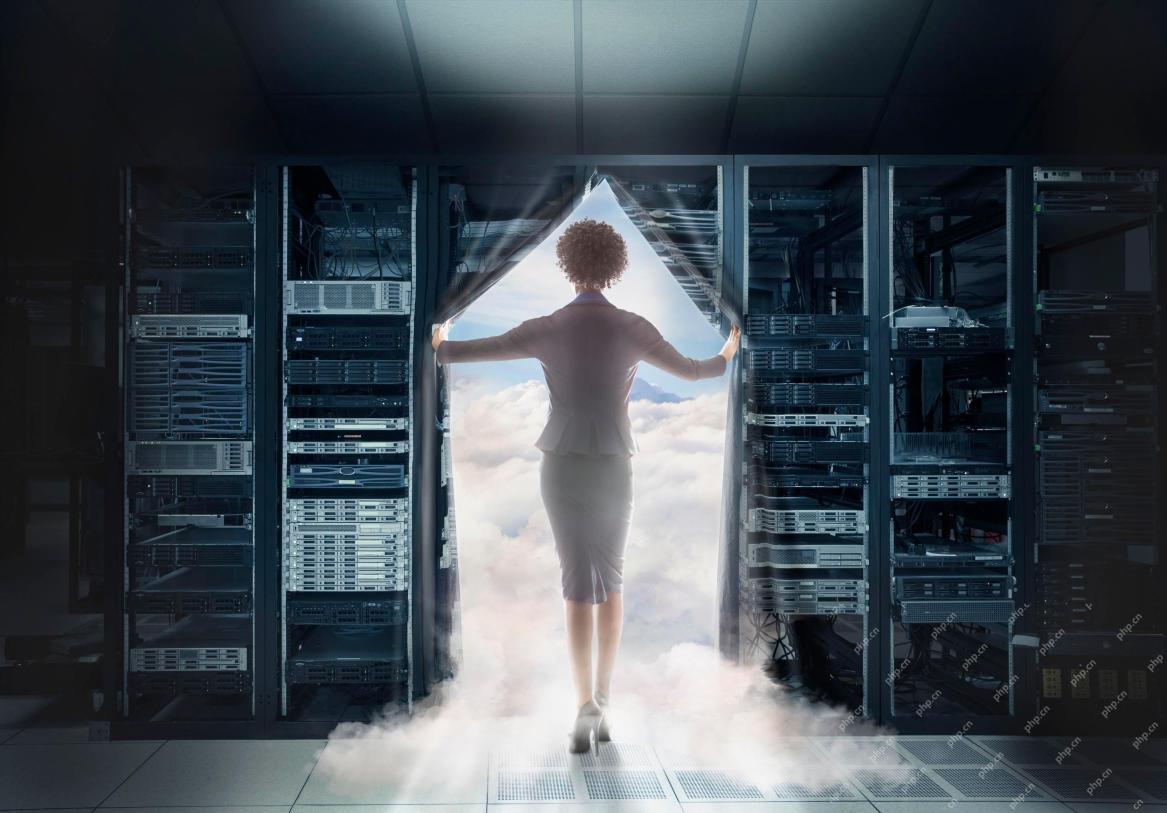
In John Rawls' seminal 1971 book The Theory of Justice, he proposed a thought experiment that we should take as the core of today's AI design and use decision-making: the veil of ignorance. This philosophy provides a simple tool for understanding equity and also provides a blueprint for leaders to use this understanding to design and implement AI equitably. Imagine that you are making rules for a new society. But there is a premise: you don’t know in advance what role you will play in this society. You may end up being rich or poor, healthy or disabled, belonging to a majority or marginal minority. Operating under this "veil of ignorance" prevents rule makers from making decisions that benefit themselves. On the contrary, people will be more motivated to formulate public
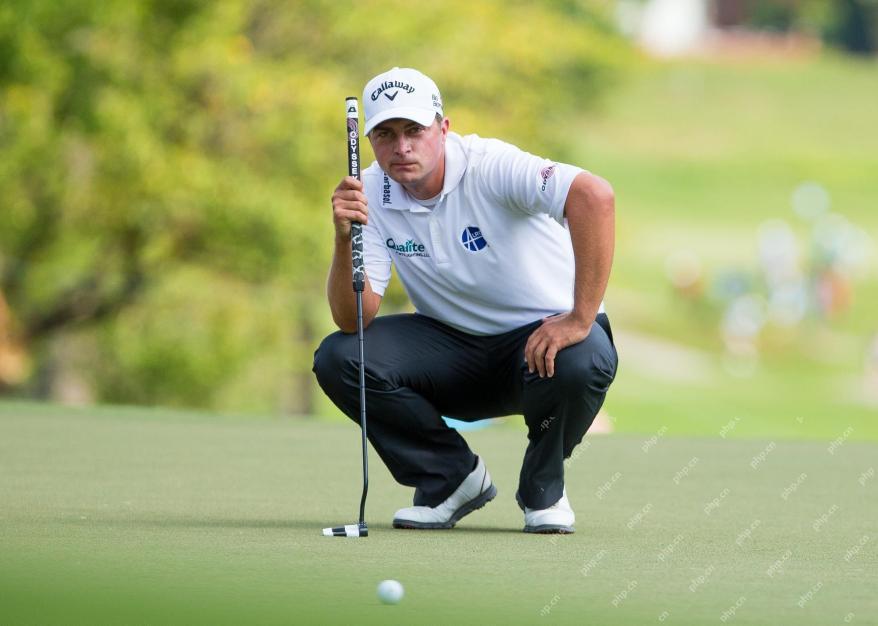
Numerous companies specialize in robotic process automation (RPA), offering bots to automate repetitive tasks—UiPath, Automation Anywhere, Blue Prism, and others. Meanwhile, process mining, orchestration, and intelligent document processing speciali
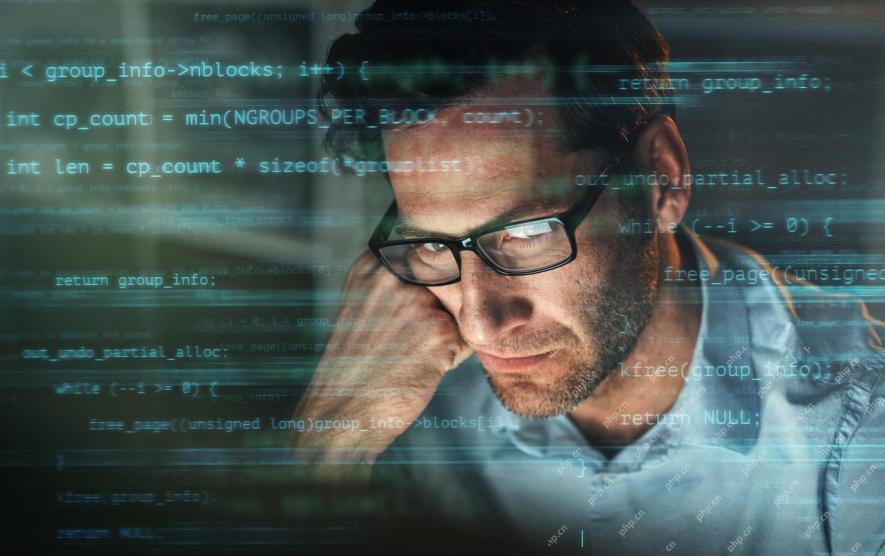
The future of AI is moving beyond simple word prediction and conversational simulation; AI agents are emerging, capable of independent action and task completion. This shift is already evident in tools like Anthropic's Claude. AI Agents: Research a
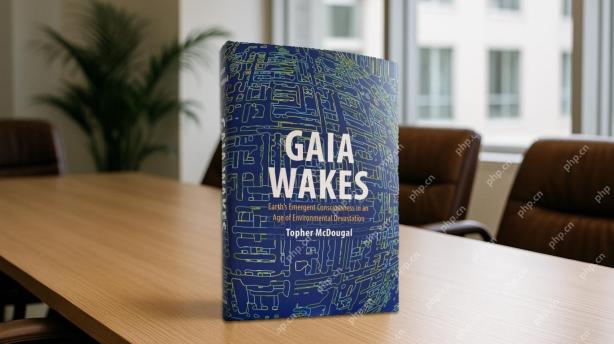
Rapid technological advancements necessitate a forward-looking perspective on the future of work. What happens when AI transcends mere productivity enhancement and begins shaping our societal structures? Topher McDougal's upcoming book, Gaia Wakes:
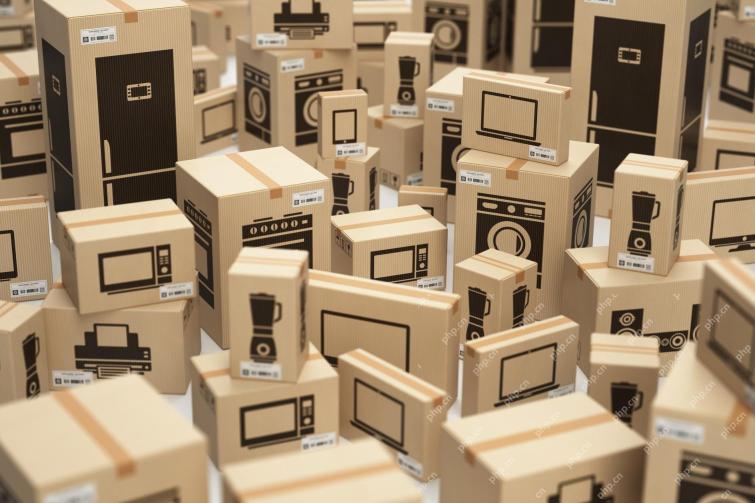
Product classification, often involving complex codes like "HS 8471.30" from systems such as the Harmonized System (HS), is crucial for international trade and domestic sales. These codes ensure correct tax application, impacting every inv
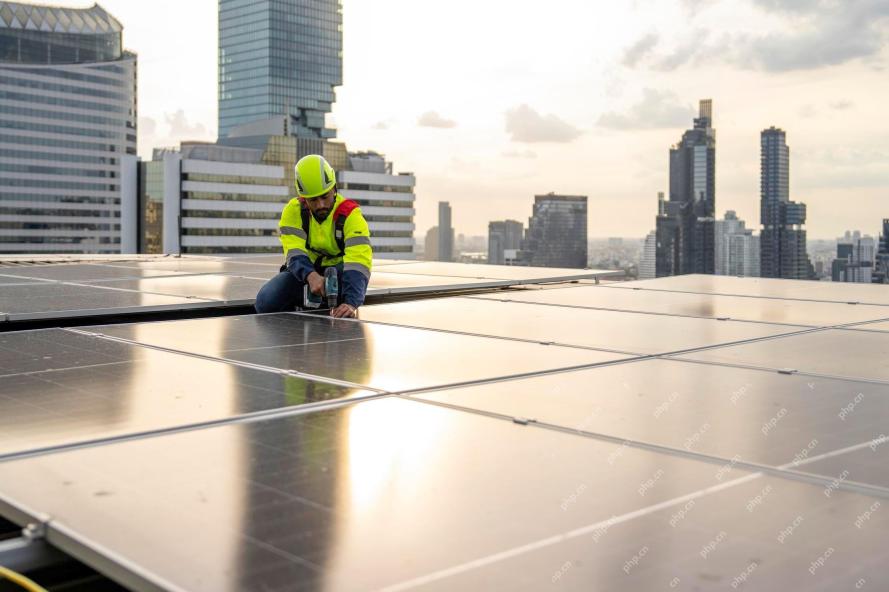
The future of energy consumption in data centers and climate technology investment This article explores the surge in energy consumption in AI-driven data centers and its impact on climate change, and analyzes innovative solutions and policy recommendations to address this challenge. Challenges of energy demand: Large and ultra-large-scale data centers consume huge power, comparable to the sum of hundreds of thousands of ordinary North American families, and emerging AI ultra-large-scale centers consume dozens of times more power than this. In the first eight months of 2024, Microsoft, Meta, Google and Amazon have invested approximately US$125 billion in the construction and operation of AI data centers (JP Morgan, 2024) (Table 1). Growing energy demand is both a challenge and an opportunity. According to Canary Media, the looming electricity
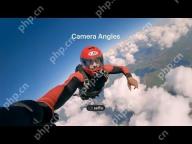
Generative AI is revolutionizing film and television production. Luma's Ray 2 model, as well as Runway's Gen-4, OpenAI's Sora, Google's Veo and other new models, are improving the quality of generated videos at an unprecedented speed. These models can easily create complex special effects and realistic scenes, even short video clips and camera-perceived motion effects have been achieved. While the manipulation and consistency of these tools still need to be improved, the speed of progress is amazing. Generative video is becoming an independent medium. Some models are good at animation production, while others are good at live-action images. It is worth noting that Adobe's Firefly and Moonvalley's Ma
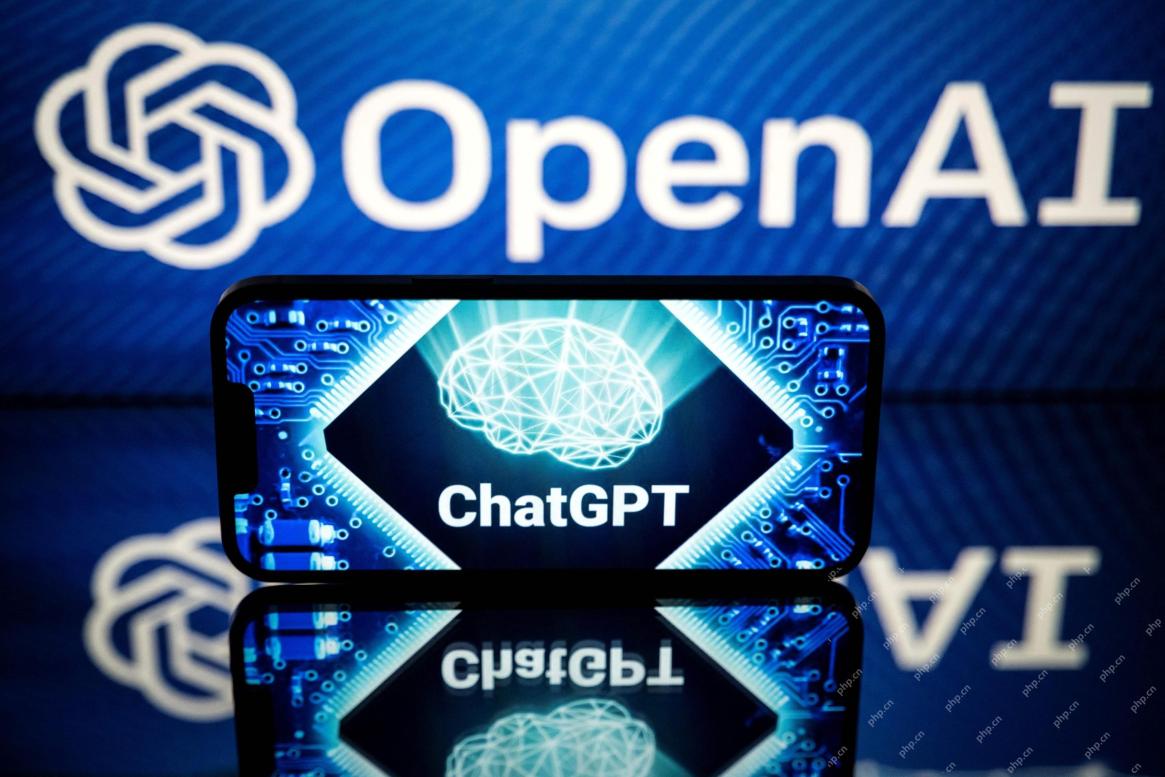
ChatGPT user experience declines: is it a model degradation or user expectations? Recently, a large number of ChatGPT paid users have complained about their performance degradation, which has attracted widespread attention. Users reported slower responses to models, shorter answers, lack of help, and even more hallucinations. Some users expressed dissatisfaction on social media, pointing out that ChatGPT has become “too flattering” and tends to verify user views rather than provide critical feedback. This not only affects the user experience, but also brings actual losses to corporate customers, such as reduced productivity and waste of computing resources. Evidence of performance degradation Many users have reported significant degradation in ChatGPT performance, especially in older models such as GPT-4 (which will soon be discontinued from service at the end of this month). this


Hot AI Tools

Undresser.AI Undress
AI-powered app for creating realistic nude photos

AI Clothes Remover
Online AI tool for removing clothes from photos.

Undress AI Tool
Undress images for free

Clothoff.io
AI clothes remover

Video Face Swap
Swap faces in any video effortlessly with our completely free AI face swap tool!

Hot Article

Hot Tools

Atom editor mac version download
The most popular open source editor
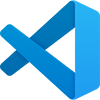
VSCode Windows 64-bit Download
A free and powerful IDE editor launched by Microsoft

Zend Studio 13.0.1
Powerful PHP integrated development environment

SublimeText3 English version
Recommended: Win version, supports code prompts!

Notepad++7.3.1
Easy-to-use and free code editor
