Overview of Deepfake Detection Based on Deep Learning
Deep learning (DL) has become one of the most influential fields in computer science, directly affecting today's human life and society. Like every other technological innovation in history, deep learning has been used for some illegal purposes. Deepfakes are such a deep learning application. Hundreds of studies have been conducted in the past few years to invent and optimize various Deepfake detection using AI. This article mainly discusses how to detect Deepfake.
To deal with deepfakes, deep learning methods as well as machine learning (non-deep learning) methods have been developed to detect them. Deep learning models need to consider a large number of parameters, so a large amount of data is required to train such models. This is exactly why DL methods have higher performance and accurate results compared to non-DL methods.
What is Deepfake Detection
Most deepfake generators will leave some traces during the deepfake process. These changes in deepfake videos can be classified as spatial inconsistencies: incompatibilities that occur within individual frames of the video and temporal inconsistencies: incompatible features that appear in the sequence of video frames.
Spatial inconsistencies include areas of the face that are incompatible with the background of the video frame, resolution changes, and partially rendered organ and skin textures (which may not render all human features of the face correctly). Most common deepfake generators are unable to render features such as blinks and teeth. And sometimes white strips are used to replace the teeth that are even visible to the naked eye on the still frame (below).
Temporal inconsistencies include abnormal eye blinks, head postures, facial movements, and brightness changes in video frame sequences.
These traces, both spatial and temporal, left by deepfake generators can be identified by deepfake detectors made from deep neural networks (DNN). The widespread use of familiar generative adversarial networks (GANs) in deepfake generators challenges the balance between fake detection and generation.
Deepfake Detection
Deepfake detector is a binary classification system that determines whether input digital media is real or fake. Deepfake detection is not performed by a single black-box-like module, but consists of several other modules and steps that work together to provide detection results. Common steps in Deepfake detection are as follows [2].
- Deepfake Input from digital media.
- Preprocessing includes face detection and enhancement.
- Feature extraction of processed frames.
- Classification/Detection.
- Authenticity of the output image.
A typical DL-based deepfake detector contains 3 main components to perform the above tasks.
- Preprocessing module.
- Feature extraction module.
- Evaluator module (deep learning classifier model).
The main steps will be explained in detail next: data preprocessing, feature extraction and detection/classification process.
Data Preprocessing
After the data collection phase, the data should be preprocessed before the training and testing steps for deepfake detection. Data preprocessing is done automatically using available libraries such as OpenCV python, MTCNN and YOLO etc.
Data augmentation also plays a crucial role in improving the performance of deepfake detectors. Augmentation techniques such as rescaling (stretching), shear mapping, scaling augmentation, rotation, brightness changes, and horizontal/vertical flipping can be applied to increase the generalization of the dataset [3].
The first step in data preprocessing is to extract individual frames from the video clip. After extracting the frames, you need to detect faces from the extracted video frames. Since facial regions often appear anomalies, selecting only facial regions helps the feature extraction model focus only on the region of interest (ROI), thus saving the computational cost for full-frame scanning. Once facial regions are detected, they are cropped from the rest of the background of the frame and follow a series of steps to make them available for model training and testing. Another reason to crop facial regions is to make all input images to the model the same size.
Feature extraction
The frames preprocessed in the previous step will be sent to the feature extractor. Most feature extractors are based on convolutional neural networks (CNN). Some recent studies have demonstrated the effectiveness and efficiency improvement of the application of capsule networks in the feature extraction process, which is a new trend.
The feature extractor extracts the spatial features available on the preprocessed video frames. Feature extraction can extract visual features, local features/facial landmarks, such as the position of eyes, nose, mouth, dynamics of mouth shape, blinking and other biological features. The extracted feature vectors are then sent to the classifier network to output decisions.
Classification
The deep learning model used for classification is often called the backbone of the deepfake detector. As the name suggests, the classification network is responsible for the most important task in the deepfake detection pipeline: namely, classifying and determining the probability of whether the input video is a deepfake. Most classifiers are binary classifiers, where the deepfakes output is (0) and the original frame output is (1).
The classifier is yet another convolutional layer (CNN) or similar deep learning architecture such as LSTM or ViT. The actual capabilities of a classification model vary depending on the DNN used. For example, the blink features extracted in the feature extractor module can be used by the LSTM module in the classification module to determine the temporal inconsistency of the frame blink pattern and determine whether the input is a Deepfake [3]. In most cases, the last layer in a deepfake detector is a fully connected layer. Since the outputs of the convolutional layers represent high-level features of the data, these outputs are flattened and concatenated to a single output layer to produce the final decision.
Summary
Over the past few years, there have been significant developments in both the creation and detection of deepfakes. Research related to deepfake detection using deep learning technology has also made great progress due to the accuracy of the results compared to non-deep learning methods. Deep neural network architectures such as CNN, RNN, ViT, and capsule networks are widely used in the implementation of deepfake detectors. A common deepfake detection pipeline consists of a data preprocessing module, a CNN-based feature extractor, and a classification module.
In addition, deepfake detection has a strong dependence on the traces left by the deepfake generator on the deepfake. Since current GAN-based deepfake generators are capable of synthesizing more realistic deepfakes with minimal inconsistencies, new methods must be developed to optimize deepfake detection. Deepfake detection methods based on deep ensemble learning techniques can be considered as modern and comprehensive methods to combat deepfakes [4]. Nonetheless, a gap for effective and efficient deepfake detectors still exists.
The above is the detailed content of Overview of Deepfake Detection Based on Deep Learning. For more information, please follow other related articles on the PHP Chinese website!
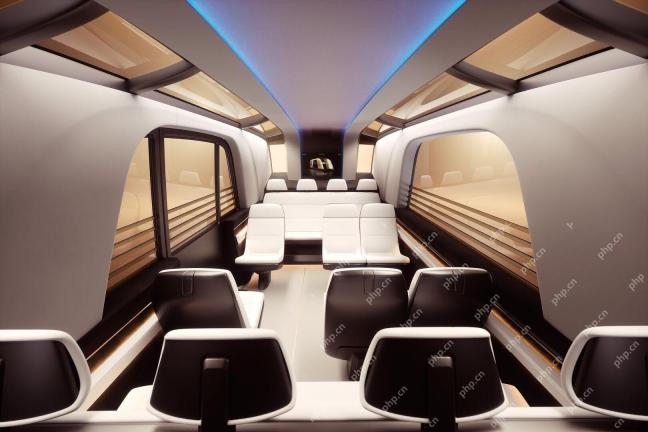
Since 2008, I've championed the shared-ride van—initially dubbed the "robotjitney," later the "vansit"—as the future of urban transportation. I foresee these vehicles as the 21st century's next-generation transit solution, surpas
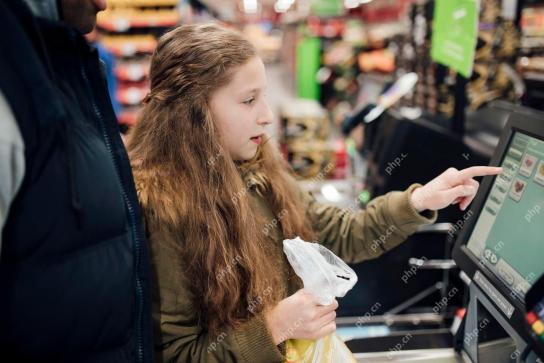
Revolutionizing the Checkout Experience Sam's Club's innovative "Just Go" system builds on its existing AI-powered "Scan & Go" technology, allowing members to scan purchases via the Sam's Club app during their shopping trip.
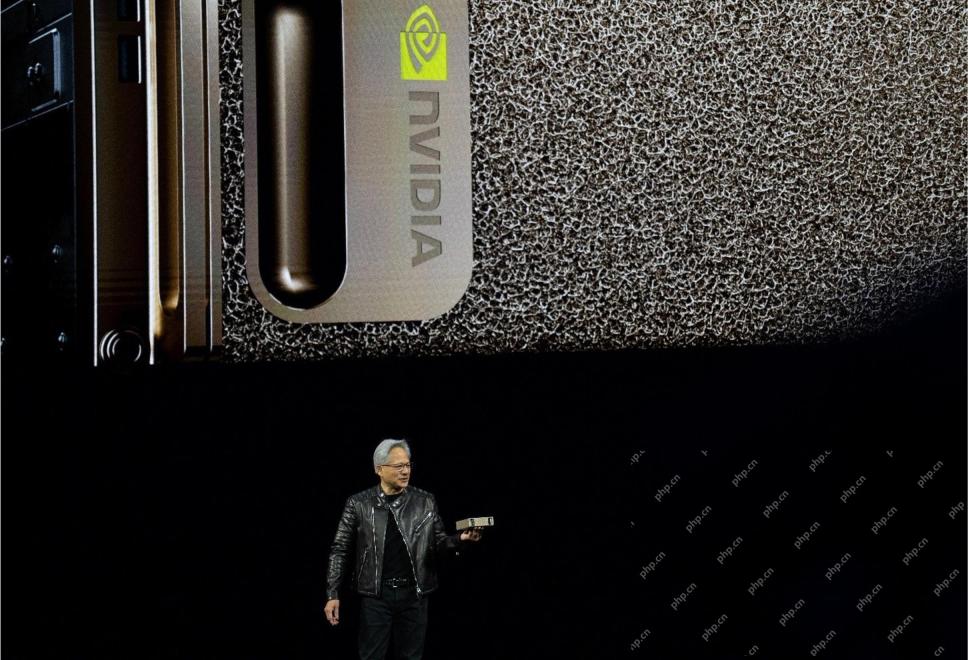
Nvidia's Enhanced Predictability and New Product Lineup at GTC 2025 Nvidia, a key player in AI infrastructure, is focusing on increased predictability for its clients. This involves consistent product delivery, meeting performance expectations, and
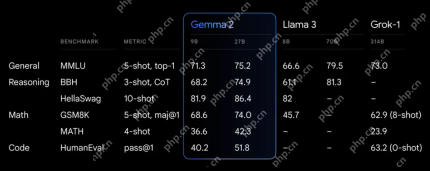
Google's Gemma 2: A Powerful, Efficient Language Model Google's Gemma family of language models, celebrated for efficiency and performance, has expanded with the arrival of Gemma 2. This latest release comprises two models: a 27-billion parameter ver
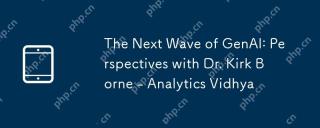
This Leading with Data episode features Dr. Kirk Borne, a leading data scientist, astrophysicist, and TEDx speaker. A renowned expert in big data, AI, and machine learning, Dr. Borne offers invaluable insights into the current state and future traje
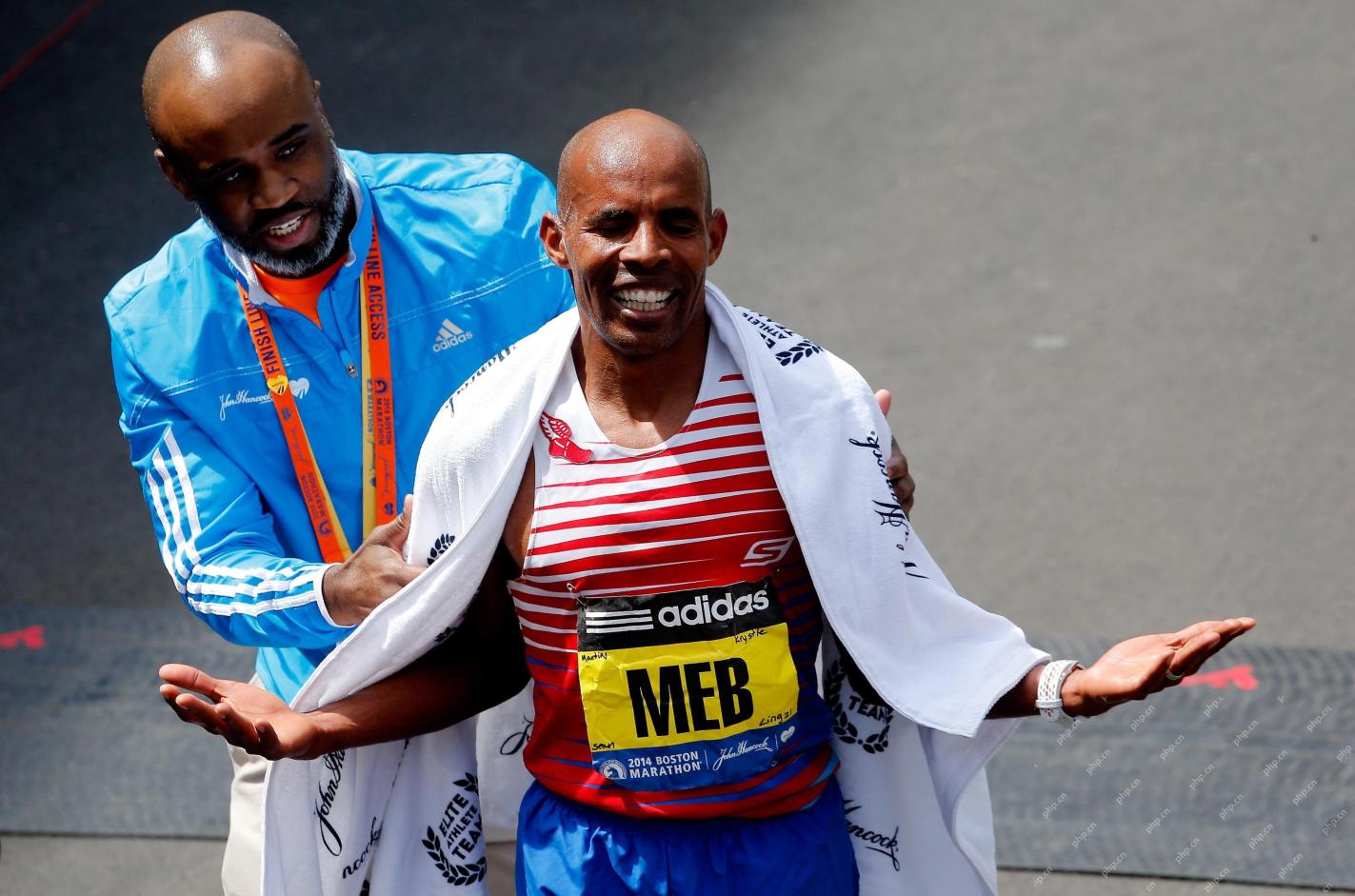
There were some very insightful perspectives in this speech—background information about engineering that showed us why artificial intelligence is so good at supporting people’s physical exercise. I will outline a core idea from each contributor’s perspective to demonstrate three design aspects that are an important part of our exploration of the application of artificial intelligence in sports. Edge devices and raw personal data This idea about artificial intelligence actually contains two components—one related to where we place large language models and the other is related to the differences between our human language and the language that our vital signs “express” when measured in real time. Alexander Amini knows a lot about running and tennis, but he still
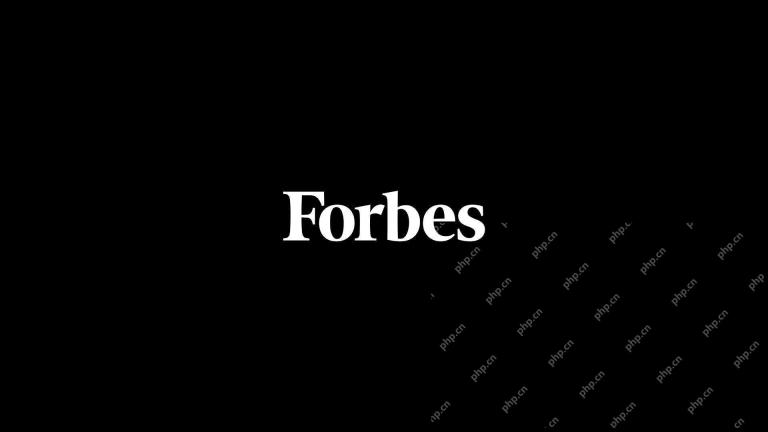
Caterpillar's Chief Information Officer and Senior Vice President of IT, Jamie Engstrom, leads a global team of over 2,200 IT professionals across 28 countries. With 26 years at Caterpillar, including four and a half years in her current role, Engst
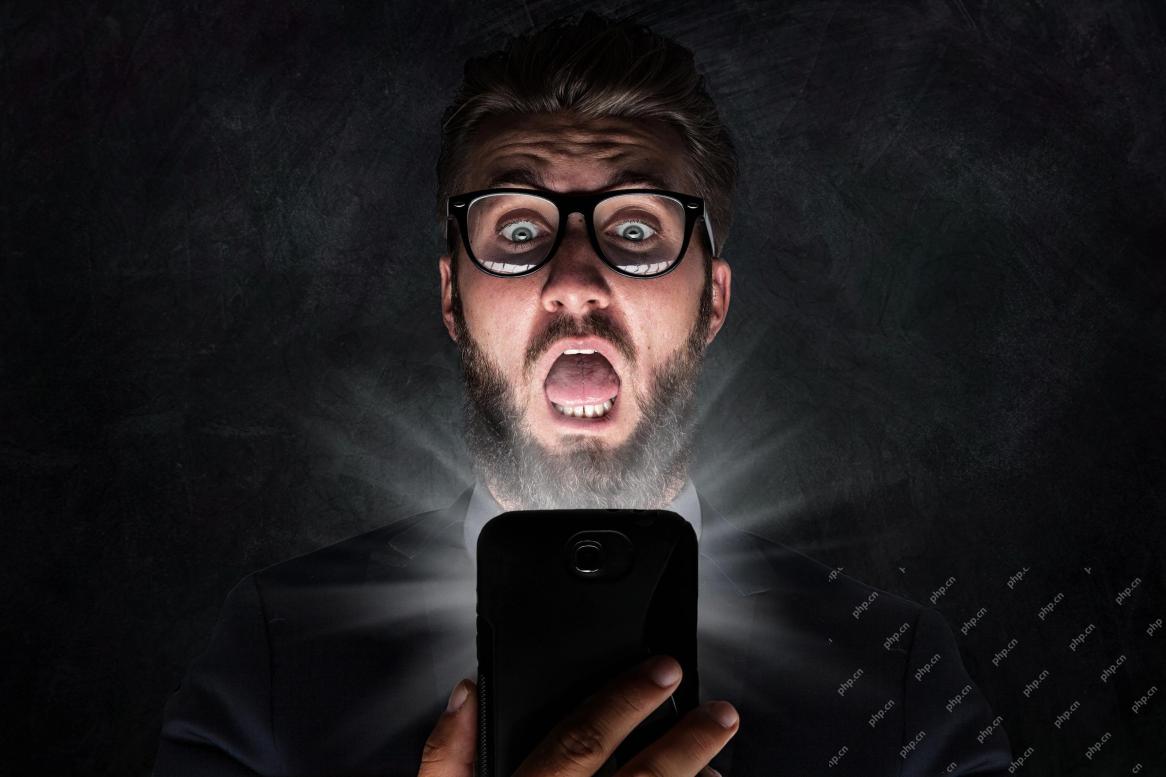
Google Photos' New Ultra HDR Tool: A Quick Guide Enhance your photos with Google Photos' new Ultra HDR tool, transforming standard images into vibrant, high-dynamic-range masterpieces. Ideal for social media, this tool boosts the impact of any photo,


Hot AI Tools

Undresser.AI Undress
AI-powered app for creating realistic nude photos

AI Clothes Remover
Online AI tool for removing clothes from photos.

Undress AI Tool
Undress images for free

Clothoff.io
AI clothes remover

Video Face Swap
Swap faces in any video effortlessly with our completely free AI face swap tool!

Hot Article

Hot Tools

Atom editor mac version download
The most popular open source editor

SublimeText3 Linux new version
SublimeText3 Linux latest version

mPDF
mPDF is a PHP library that can generate PDF files from UTF-8 encoded HTML. The original author, Ian Back, wrote mPDF to output PDF files "on the fly" from his website and handle different languages. It is slower than original scripts like HTML2FPDF and produces larger files when using Unicode fonts, but supports CSS styles etc. and has a lot of enhancements. Supports almost all languages, including RTL (Arabic and Hebrew) and CJK (Chinese, Japanese and Korean). Supports nested block-level elements (such as P, DIV),

Zend Studio 13.0.1
Powerful PHP integrated development environment

SecLists
SecLists is the ultimate security tester's companion. It is a collection of various types of lists that are frequently used during security assessments, all in one place. SecLists helps make security testing more efficient and productive by conveniently providing all the lists a security tester might need. List types include usernames, passwords, URLs, fuzzing payloads, sensitive data patterns, web shells, and more. The tester can simply pull this repository onto a new test machine and he will have access to every type of list he needs.