


From BERT to ChatGPT, a hundred-page review summarizes the evolution history of pre-trained large models
All success has its traces, and ChatGPT is no exception.
Not long ago, Turing Award winner Yann LeCun was put on the hot search list because of his harsh evaluation of ChatGPT.
In his view, “As far as the underlying technology is concerned, ChatGPT has no special innovation,” nor is it “anything revolutionary.” Many research labs are using the same technology and doing the same work. What’s more, ChatGPT and the GPT-3 behind it are in many ways composed of multiple technologies developed over many years by multiple parties and are the result of decades of contributions by different people. Therefore, LeCun believes that ChatGPT is not so much a scientific breakthrough as it is a decent engineering example.
"Whether ChatGPT is revolutionary" is a controversial topic. But there is no doubt that it is built on the basis of many technologies accumulated previously. For example, the core Transformer was proposed by Google a few years ago, and Transformer was inspired by Bengio's work on the concept of attention. If we go back further, we can also link to research from even earlier decades.
Of course, the public may not be able to appreciate this step-by-step feeling. After all, not everyone will read the papers one by one. But for technicians, it is still very helpful to understand the evolution of these technologies.
In a recent review article, researchers from Michigan State University, Beijing Aeronautical University, Lehigh University and other institutions carefully combed this field There are hundreds of papers, mainly focusing on pre-training basic models in the fields of text, image and graph learning, which are well worth reading. Professor at Duke University, Academician of the Canadian Academy of EngineeringPei Jian,Distinguished Professor of Computer Science Department, University of Illinois at ChicagoYu Shilun(Philip S. Yu) , Salesforce AI Research Vice PresidentXiong CaimingDu is one of the authors of the paper.
Paper link: https://arxiv.org/pdf/2302.09419.pdf
Thesis table of contents is as follows:
On overseas social platforms, DAIR.AI co-founder Elvis S. recommended this review and won one More than a thousand likes.
Introduction
Pre-trained basic model (PFM) is an important part of artificial intelligence in the big data era. The name "Basic Model" comes from a review published by Percy Liang, Li Feifei and others - "On the Opportunities and Risks of Foundation Models", which is a general term for a type of model and its functions. PFM has been extensively studied in the fields of NLP, CV, and graph learning. They show strong potential for feature representation learning in various learning tasks, such as text classification, text generation, image classification, object detection, and graph classification. Whether training on multiple tasks with large datasets or fine-tuning on small-scale tasks, PFM exhibits superior performance, which makes it possible to quickly start data processing.
PFM and pre-training
PFM is based on pre-training technology, which aims to utilize a large amount of data and tasks to Train a general model that can be easily fine-tuned in different downstream applications.
The idea of pre-training originated from transfer learning in CV tasks. But after seeing the effectiveness of this technology in the CV field, people began to use this technology to improve model performance in other fields.
When pre-training technology is applied to the NLP field, a well-trained language model can capture rich knowledge that is beneficial to downstream tasks, such as long-term dependencies, hierarchical relationships, etc. In addition, the significant advantage of pre-training in the field of NLP is that the training data can come from any unlabeled text corpus, that is, there is an almost unlimited amount of training data that can be used for the pre-training process. Early pre-training was a static technique, such as NNLM and Word2vec, but static methods are difficult to adapt to different semantic environments. Therefore, dynamic pre-training techniques have been proposed, such as BERT, XLNet, etc. Figure 1 depicts the history and evolution of PFM in the fields of NLP, CV, and GL. PFM based on pre-training techniques uses large corpora to learn common semantic representations. Following the introduction of these pioneering works, various PFMs have emerged and been applied to downstream tasks and applications.
The recently popular ChatGPT is a typical case of PFM application. It is fine-tuned from the generative pre-trained transformer model GPT-3.5, which was trained using a large amount of this paper and code. Additionally, ChatGPT applies reinforcement learning from human feedback (RLHF), which has emerged as a promising way to align large-scale LMs with human intentions. The excellent performance of ChatGPT may bring about changes in the training paradigm of each type of PFM, such as the application of instruction alignment technology, reinforcement learning, prompt tuning and thinking chain, thus moving towards general artificial intelligence.
This article will focus on PFM in the fields of text, image and graph, which is a relatively mature research classification method. For text, it is a general-purpose LM used to predict the next word or character in a sequence. For example, PFM can be used in machine translation, question answering systems, topic modeling, sentiment analysis, etc. For images, it is similar to PFM on text, using huge datasets to train a large model suitable for many CV tasks. For graphs, similar pre-training ideas are used to obtain PFMs that are used in many downstream tasks. In addition to PFMs for specific data domains, this article also reviews and elaborates on some other advanced PFMs, such as PFMs for voice, video, and cross-domain data, and multimodal PFMs. In addition, a great convergence of PFMs capable of handling multi-modal tasks is emerging, which is the so-called unified PFM. The authors first define the concept of unified PFM and then review recent research on unified PFMs that have achieved SOTA (such as OFA, UNIFIED-IO, FLAVA, BEiT-3, etc.).
Based on the characteristics of existing PFM in the above three fields, the author concludes that PFM has the following two major advantages. First, to improve performance on downstream tasks, the model requires only minor fine-tuning. Second, PFM has been scrutinized in terms of quality. Instead of building a model from scratch to solve a similar problem, we can apply PFM to task-relevant data sets. The broad prospects of PFM have inspired a large amount of related work to focus on issues such as model efficiency, security, and compression.
Contribution and structure of the paper
Before the publication of this article, there have been several reviews reviewing some specific Pre-trained models in fields such as text generation, visual transformer, and target detection.
"On the Opportunities and Risks of Foundation Models" summarizes the opportunities and risks of foundation models. However, existing works do not achieve a comprehensive review of PFM in different domains (e.g., CV, NLP, GL, Speech, Video) on different aspects, such as pre-training tasks, efficiency, effectiveness, and privacy. In this review, the authors elaborate on the evolution of PFM in the NLP field and how pre-training has been transferred and adopted in the CV and GL fields.
Compared with other reviews, this article does not provide a comprehensive introduction and analysis of existing PFMs in all three fields. Different from the review of previous pre-trained models, the authors summarize existing models, from traditional models to PFM, as well as the latest work in three fields. Traditional models emphasize static feature learning. Dynamic PFM provides an introduction to structure, which is mainstream research.
The author further introduces some other research on PFM, including other advanced and unified PFM, model efficiency and compression, security, and privacy. Finally, the authors summarize future research challenges and open issues in different fields. They also provide a comprehensive introduction to relevant evaluation metrics and datasets in Appendices F and G.
In short, the main contributions of this article are as follows:
- To PFM Developments in NLP, CV and GL are reviewed in detail and up-to-date. In the review, the authors discuss and provide insights on the design and pre-training methods of general-purpose PFM in these three major application areas;
- summarizes the development of PFM in other multimedia areas, Such as voice and video. Additionally, the authors discuss cutting-edge topics on PFM, including unified PFM, model efficiency and compression, and security and privacy.
- Through a review of various modes of PFM in different tasks, the author discusses the main challenges and opportunities for future research on very large models in the big data era, which guides a new generation of PFM-based Collaborative and interactive intelligence.
The main contents of each chapter are as follows:
Chapter 2 of the paper introduces the general concept of PFM architecture.
Chapters 3, 4, and 5 summarize existing PFMs in the fields of NLP, CV, and GL, respectively.
#Chapter 6 and 7 introduce other cutting-edge research in PFM, including cutting-edge and unified PFM, model efficiency and compression, and security and privacy.
Chapter 8 summarizes the main challenges of PFM. Chapter 9 summarizes the full text.
The above is the detailed content of From BERT to ChatGPT, a hundred-page review summarizes the evolution history of pre-trained large models. For more information, please follow other related articles on the PHP Chinese website!
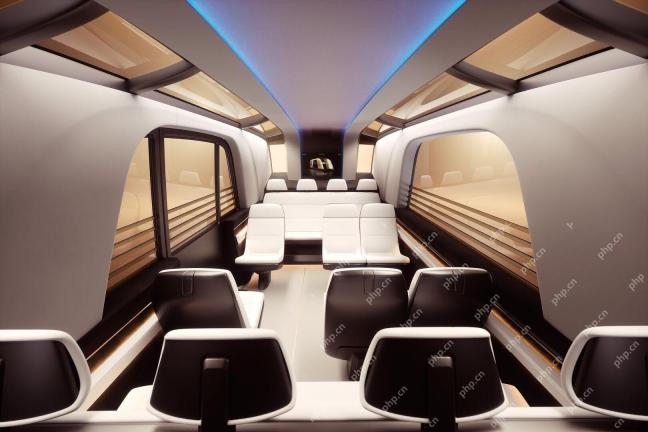
Since 2008, I've championed the shared-ride van—initially dubbed the "robotjitney," later the "vansit"—as the future of urban transportation. I foresee these vehicles as the 21st century's next-generation transit solution, surpas
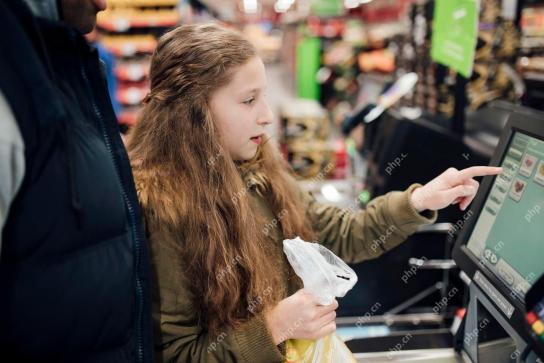
Revolutionizing the Checkout Experience Sam's Club's innovative "Just Go" system builds on its existing AI-powered "Scan & Go" technology, allowing members to scan purchases via the Sam's Club app during their shopping trip.
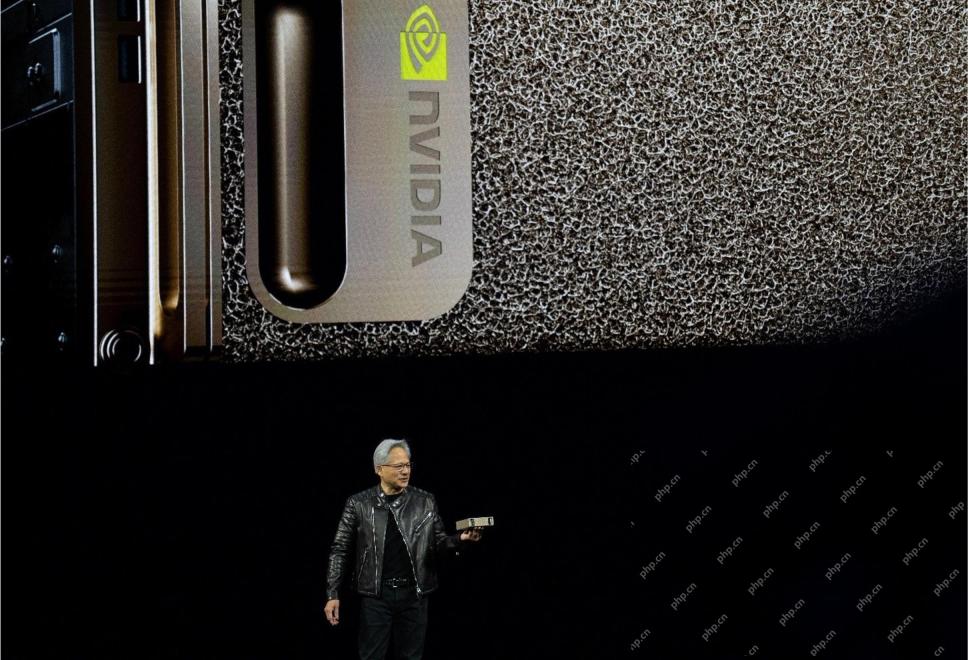
Nvidia's Enhanced Predictability and New Product Lineup at GTC 2025 Nvidia, a key player in AI infrastructure, is focusing on increased predictability for its clients. This involves consistent product delivery, meeting performance expectations, and
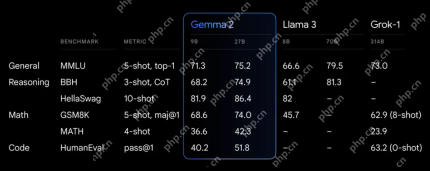
Google's Gemma 2: A Powerful, Efficient Language Model Google's Gemma family of language models, celebrated for efficiency and performance, has expanded with the arrival of Gemma 2. This latest release comprises two models: a 27-billion parameter ver
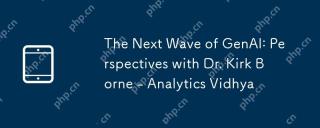
This Leading with Data episode features Dr. Kirk Borne, a leading data scientist, astrophysicist, and TEDx speaker. A renowned expert in big data, AI, and machine learning, Dr. Borne offers invaluable insights into the current state and future traje
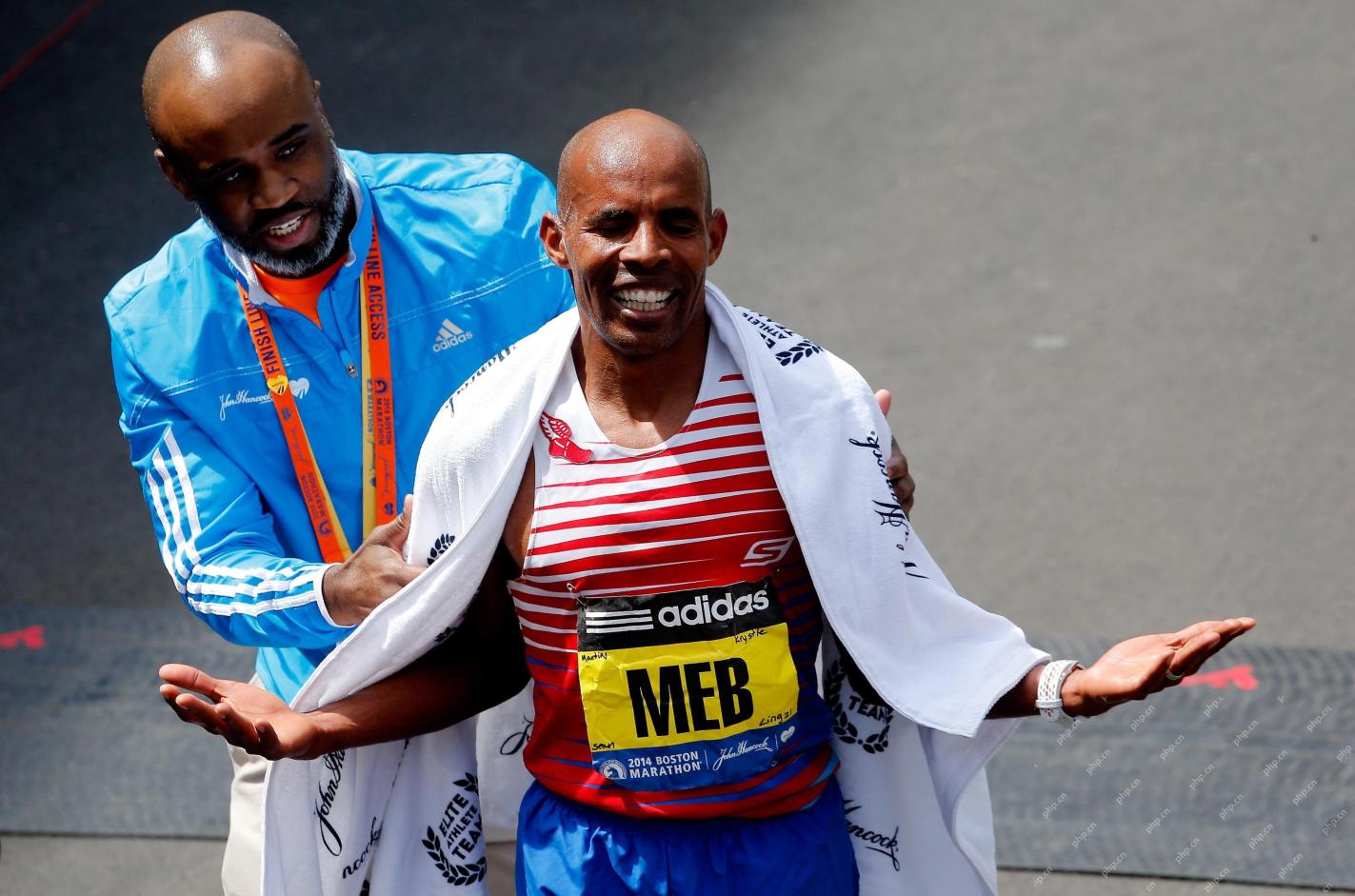
There were some very insightful perspectives in this speech—background information about engineering that showed us why artificial intelligence is so good at supporting people’s physical exercise. I will outline a core idea from each contributor’s perspective to demonstrate three design aspects that are an important part of our exploration of the application of artificial intelligence in sports. Edge devices and raw personal data This idea about artificial intelligence actually contains two components—one related to where we place large language models and the other is related to the differences between our human language and the language that our vital signs “express” when measured in real time. Alexander Amini knows a lot about running and tennis, but he still
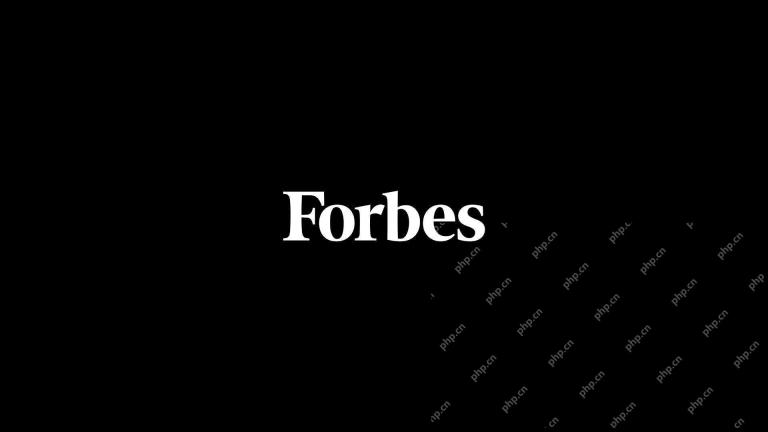
Caterpillar's Chief Information Officer and Senior Vice President of IT, Jamie Engstrom, leads a global team of over 2,200 IT professionals across 28 countries. With 26 years at Caterpillar, including four and a half years in her current role, Engst
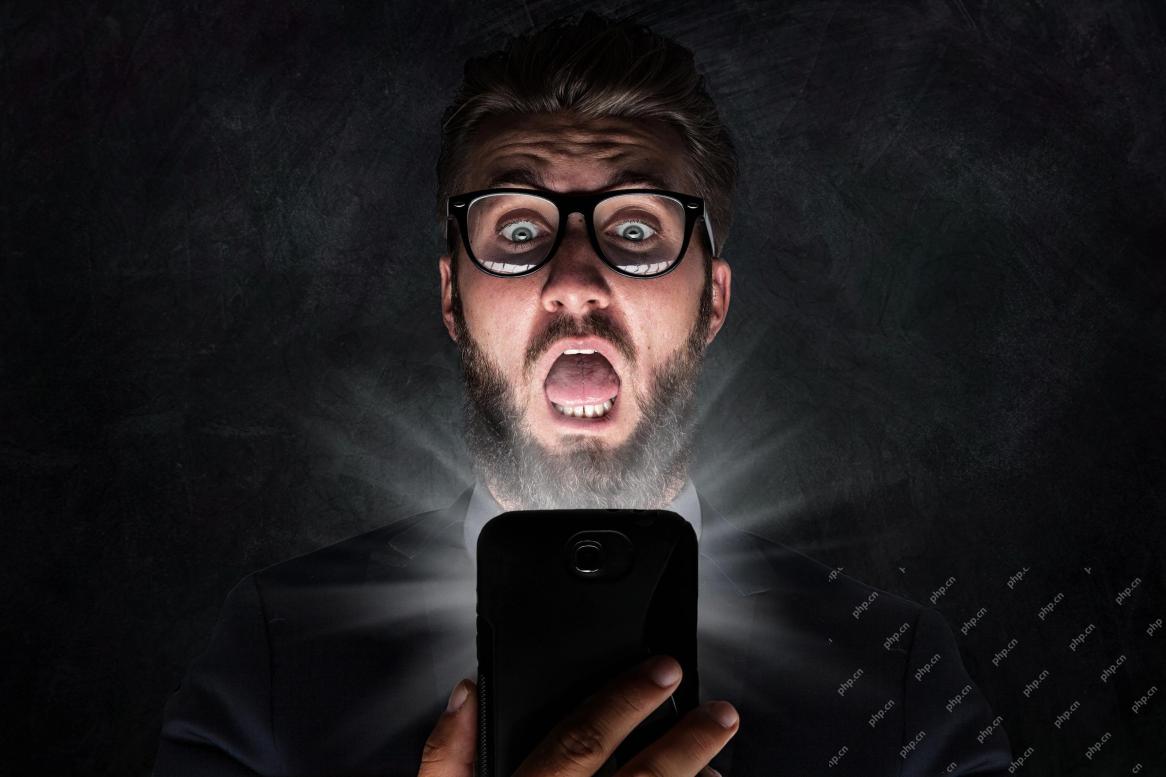
Google Photos' New Ultra HDR Tool: A Quick Guide Enhance your photos with Google Photos' new Ultra HDR tool, transforming standard images into vibrant, high-dynamic-range masterpieces. Ideal for social media, this tool boosts the impact of any photo,


Hot AI Tools

Undresser.AI Undress
AI-powered app for creating realistic nude photos

AI Clothes Remover
Online AI tool for removing clothes from photos.

Undress AI Tool
Undress images for free

Clothoff.io
AI clothes remover

Video Face Swap
Swap faces in any video effortlessly with our completely free AI face swap tool!

Hot Article

Hot Tools
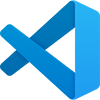
VSCode Windows 64-bit Download
A free and powerful IDE editor launched by Microsoft

DVWA
Damn Vulnerable Web App (DVWA) is a PHP/MySQL web application that is very vulnerable. Its main goals are to be an aid for security professionals to test their skills and tools in a legal environment, to help web developers better understand the process of securing web applications, and to help teachers/students teach/learn in a classroom environment Web application security. The goal of DVWA is to practice some of the most common web vulnerabilities through a simple and straightforward interface, with varying degrees of difficulty. Please note that this software

PhpStorm Mac version
The latest (2018.2.1) professional PHP integrated development tool

SublimeText3 English version
Recommended: Win version, supports code prompts!

Atom editor mac version download
The most popular open source editor